Exploring the Potential of ChatGPT for Spectral and Energy Management in Enterprise Content Management
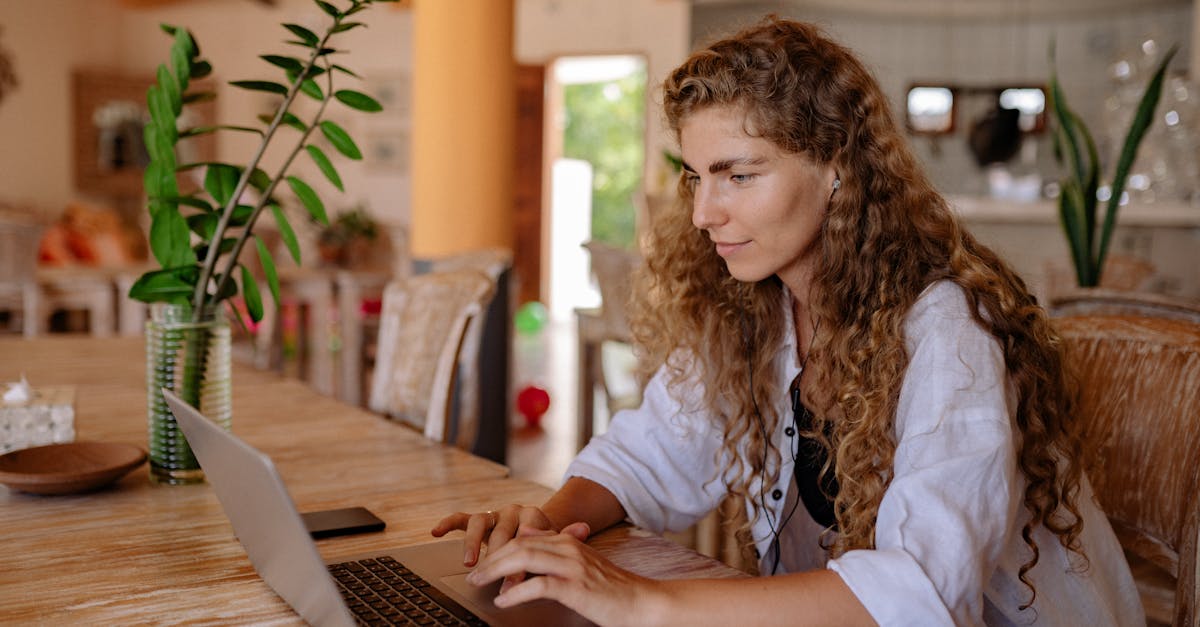
Enterprise Content Management (ECM) is a technology used to manage an organization's information assets effectively. It involves processes and tools for capturing, storing, organizing, and delivering documents, data, and other content related to the organization's operations. ECM plays a crucial role in ensuring efficient and secure access to information.
Spectral and Energy Management
Spectral and Energy Management is an area of expertise that focuses on analyzing and controlling energy usage within an enterprise. It involves the measurement, analysis, and optimization of energy consumption to improve efficiency and reduce costs. Various technologies and methods are used in spectral and energy management to monitor and control energy usage.
One of the emerging technologies in spectral and energy management is the use of advanced AI models like ChatGPT-4. ChatGPT-4 is an AI-powered conversational model that can effectively measure, control, and conserve energy in an enterprise setting. It utilizes natural language processing and machine learning techniques to understand and analyze energy consumption data, identify potential areas of improvement, and suggest energy-saving measures.
Usage of ChatGPT-4 in Energy Management
ChatGPT-4 can be integrated into an enterprise's energy management system to provide real-time insights and recommendations for energy conservation. It can analyze energy usage patterns, identify inefficient processes or equipment, and propose optimization strategies to reduce energy waste.
Some of the key functionalities of ChatGPT-4 in energy management include:
- Data Analysis: ChatGPT-4 can process large volumes of energy consumption data from various sources and perform advanced analytics to identify consumption patterns and anomalies.
- Energy Monitoring: ChatGPT-4 can continuously monitor energy usage in different areas of the enterprise, including offices, manufacturing facilities, and data centers, providing real-time visibility into energy consumption.
- Alerts and Notifications: ChatGPT-4 can send automated alerts and notifications to relevant stakeholders when energy consumption exceeds predefined thresholds or when anomalies are detected.
- Optimization Strategies: Based on its analysis, ChatGPT-4 can suggest energy-saving strategies, such as optimizing equipment schedules, implementing energy-efficient technologies, and improving operational processes.
- Virtual Energy Assistant: ChatGPT-4 can act as a virtual assistant, answering queries related to energy usage, providing energy conservation tips, and assisting in energy-related decision-making.
The integration of ChatGPT-4 into an enterprise's energy management system can help organizations achieve significant energy savings, reduce costs, and contribute to sustainability goals. With its ability to leverage AI and analyze complex energy data, ChatGPT-4 offers valuable insights and actionable recommendations for efficient energy utilization.
As enterprises strive to become more environmentally conscious and optimize their energy consumption, technologies like ChatGPT-4 provide a powerful toolset to achieve effective energy management and conservation.
In conclusion, Enterprise Content Management and Spectral Energy Management are two critical areas that are interconnected in optimizing an enterprise's operations. ChatGPT-4, an advanced AI model, has the potential to revolutionize energy management by effectively measuring, controlling, and conserving energy in an enterprise setting. Its integration into energy management systems can help organizations achieve substantial energy savings and contribute to a greener and more sustainable future.
Comments:
Great article, Silas! I find the potential of ChatGPT for spectral and energy management fascinating. It could revolutionize how enterprise content management systems handle data!
Thank you, Emily! I'm glad you found it interesting. Yes, the possibilities are immense. Do you have any specific use cases in mind?
I have some concerns about the accuracy and reliability of ChatGPT in such critical applications. Natural language processing still has its limitations, especially when it comes to specialized domains.
Valid point, Peter. While ChatGPT has shown impressive capabilities, it's essential to thoroughly validate its performance in spectral and energy management scenarios. A thorough evaluation would be necessary before widespread adoption.
I can see the potential, but I wonder about the ethical considerations. What happens when algorithms make decisions regarding content management? How can we ensure fairness and unbiased outcomes?
This is an exciting development, Silas! I can imagine ChatGPT assisting with automated content classification and metadata tagging based on spectral and energy data. It would save a lot of manual effort!
Indeed, Michael! By leveraging ChatGPT, we can streamline content management workflows and reduce manual labor in data processing tasks. It opens up new possibilities for productivity improvements.
I'm concerned about potential biases in the training data. How do we ensure that ChatGPT doesn't amplify any existing biases within enterprise content management systems?
Valid concern, Natalie. Bias mitigation is indeed crucial. Ensuring diverse and representative training data, as well as implementing rigorous evaluation metrics, can help minimize biases and improve fairness in system outputs.
This technology sounds promising, but what about data privacy and security? How can we ensure that sensitive enterprise data remains protected while utilizing ChatGPT?
I see the potential benefits, but there might be challenges in integrating ChatGPT with existing enterprise content management systems. Compatibility and seamless adoption would be crucial.
Absolutely, Jennifer. Integration challenges are important to address. Working closely with system developers and ensuring interoperability would be essential for a successful implementation.
I wonder about the computational resources required for ChatGPT at an enterprise scale. Would it be feasible for organizations with limited resources?
That's an interesting point, David. The computational requirements could potentially add to the cost and infrastructure needs. It would be crucial to evaluate the scalability and resource demands of ChatGPT.
Silas, have you conducted any experiments or pilot projects to validate the effectiveness of ChatGPT in spectral and energy management? It would be great to hear about any real-world applications.
Thanks for asking, Emily. We have indeed carried out some proof-of-concept experiments with promising results. We partnered with a utility company to explore energy data analysis and achieved significant efficiency gains.
Additionally, we collaborated with a research institute on leveraging ChatGPT for spectral information extraction from satellite imagery. The system demonstrated remarkable accuracy and speed.
Silas, can you share any insights into the limitations of current ChatGPT models? Understanding their shortcomings can help us gauge their applicability in enterprise content management.
Good question, Peter. While ChatGPT has made significant strides, it can still generate outputs that may lack contextual understanding or require additional clarification. Continued research and refining the models are ongoing priorities.
We also need to consider potential legal implications. What accountability frameworks should be in place when autonomous systems like ChatGPT are involved in enterprise decision-making?
Silas, I'm curious about the implementation challenges in deploying ChatGPT at an enterprise scale. Are there any specific hurdles in integrating it with existing infrastructure and workflows?
Definitely, Michael. One challenge is the need for expertise in the domain during training and deployment phases. Knowledge transfer between ChatGPT and enterprise teams is crucial for effective use and addressing system limitations.
Do you envision ChatGPT replacing human roles in content management, or will it primarily assist humans in decision-making processes?
Jennifer, the goal is not to replace humans but to augment their capabilities. ChatGPT can greatly enhance decision-making, automate repetitive tasks, and assist in complex analysis, ultimately empowering human experts.
I appreciate your thorough responses, Silas. It's reassuring to see your acknowledgment of challenges, biases, and the importance of human augmentation. I hope to see ChatGPT develop further in this domain!
Silas, what future advancements or improvements do you foresee for ChatGPT in the context of spectral and energy management?
David, we aim to improve ChatGPT's domain-specific understanding and contextual reasoning capabilities. The ability to handle nuanced energy and spectral data scenarios with greater accuracy and consistency is a priority for future iterations.
How do you plan to address concerns around transparency and interpretability of ChatGPT's decisions within enterprise content management systems?
Transparency is vital, Natalie. We're actively working on techniques to provide explanations for ChatGPT's decisions, so users can understand the underlying processes. Interpretable models enhance trust and facilitate domain knowledge sharing.
Silas, could you elaborate on the training data requirements for ChatGPT in the spectral and energy management context? How much labeled data is needed for effective performance?
Great question, Alex. The data requirements depend on the complexity of the tasks and the desired performance. While having sizable labeled datasets is beneficial, we've also observed promising results with semi-supervised and unsupervised approaches combined with limited labeled data in certain scenarios.
Silas, how does ChatGPT handle situations with incomplete or noisy data, which are common in the spectral and energy management domains?
Sarah, handling incomplete or noisy data is a challenge, but there are techniques to mitigate the impact. Preprocessing steps such as data cleaning, augmentation, and incorporating robust statistical models can help improve ChatGPT's resilience in dealing with imperfect inputs.
Silas, what are your thoughts on potential collaboration between industry and academia to further advance ChatGPT's capabilities in enterprise content management?
Jennifer, collaboration between industry and academia is invaluable. It allows us to tap into diverse expertise, gain access to real-world data, validate approaches, and chart a path towards more robust, efficient, and ethically sound AI solutions for enterprise content management.
Silas, considering the potential impact of ChatGPT, how can we ensure continual improvement, transparency, and accountability in its deployment within enterprise content management?
Peter, establishing feedback loops with users and stakeholders, actively seeking diverse perspectives, and conducting comprehensive evaluations are essential. Regular model updates, adhering to ethical guidelines, and responsible AI practices can help ensure continual improvement, transparency, and accountability.
Silas, do you have any plans to open-source any components of ChatGPT to foster collaboration and accelerate progress in the field?
Emily, as of now, we've released some research models and tools to facilitate knowledge sharing. We're actively exploring opportunities for open-sourcing certain components with careful consideration for balancing progress and potential risks related to misuse or unintended consequences.
Silas, what are your thoughts on potential ethical guidelines or regulations specific to the use of AI models like ChatGPT in enterprise content management?
Michael, AI-specific ethical guidelines and regulations will be crucial to ensure responsible and fair AI deployment. Collaborative efforts across multiple stakeholders, including organizations, policymakers, and experts, can help strike the right balance and define appropriate requirements for AI models like ChatGPT.
Silas, what are the potential risks or challenges in relying heavily on ChatGPT for spectral and energy management in enterprise content management systems?
David, potential risks include overreliance on AI without critical human oversight, biases in system outputs, and challenges in adapting to rapidly evolving datasets or requirements. Addressing these risks requires a balanced approach, proactive monitoring, and continuous improvement efforts.
Silas, how can we ensure long-term maintainability and upgradability of ChatGPT in enterprise content management systems?
Long-term maintainability and upgradability can be ensured through robust software engineering practices and continuous research advancements. Regular assessment of system performance, staying up-to-date with relevant research, and versioning mechanisms can facilitate successful integration and longevity of ChatGPT in content management.
Silas, what kind of user training or knowledge transfer is typically required for users to effectively utilize ChatGPT?
Alex, user training involves familiarizing users with the capabilities and limitations of ChatGPT, understanding system outputs, and providing guidance on appropriate use cases. Knowledge transfer is crucial in building the necessary synergy between users and the AI system to maximize its benefits.
Silas, what role do you see for human-in-the-loop approaches in the utilization of ChatGPT for spectral and energy management?
Sarah, human-in-the-loop approaches are fundamental. They allow for human expertise, validation, and guidance to complement ChatGPT's capabilities. Enabling human oversight and intervention ensures the responsible application of AI technology and supports decision-making processes.
Silas, it's been an insightful article and discussion. Thank you for shedding light on the potential of ChatGPT in enterprise content management. I look forward to further developments in this exciting domain!