Exploring the Potential of ChatGPT in Disease Risk Prediction: Advancements in Life Sciences Technology
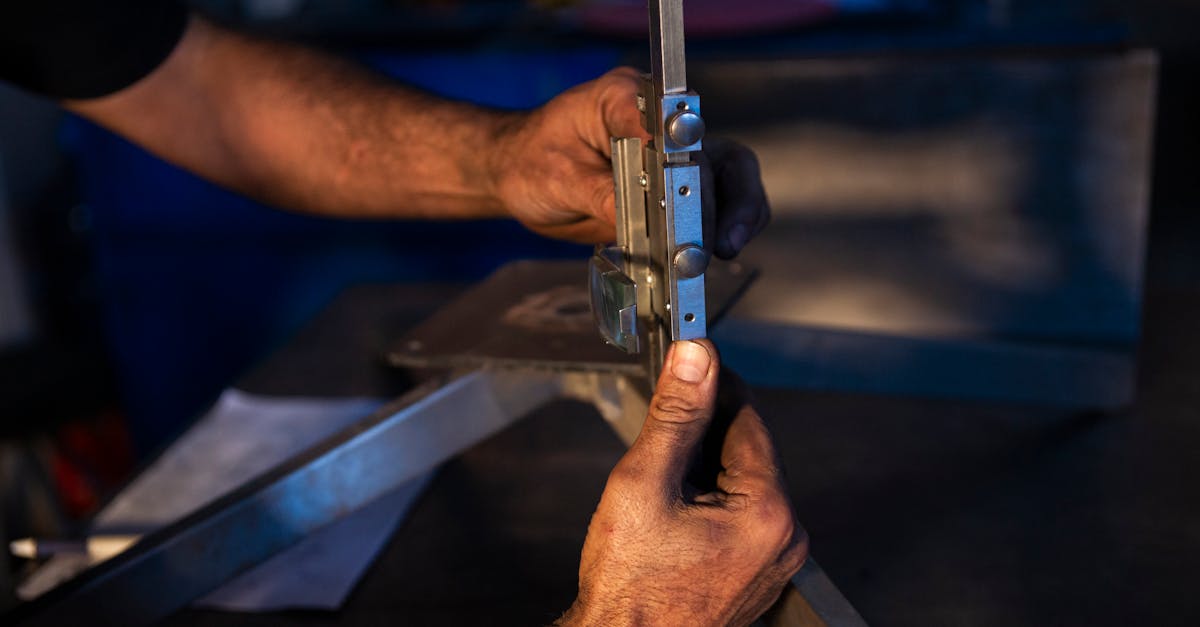
In recent years, significant advancements have been made in the field of life sciences, particularly in the area of disease risk prediction. With the integration of technology, researchers and healthcare professionals are now able to analyze patient data and genetic information to predict the risk of developing certain diseases, including cancer or cardiovascular disorders.
The use of technology in disease risk prediction has greatly revolutionized the healthcare industry. By leveraging the power of advanced algorithms and data analytics, medical professionals can make more informed decisions and provide personalized care to patients. This enables early detection and intervention, ultimately improving patient outcomes and reducing healthcare costs.
The process of disease risk prediction begins with the collection and analysis of patient data, which includes medical history, lifestyle factors, and demographic information. This data is then combined with genetic information obtained through genetic testing or sequencing. The combination of these datasets allows for a comprehensive understanding of an individual's susceptibility to certain diseases.
Life sciences technology plays a crucial role in processing and analyzing these vast amounts of data. Machine learning algorithms are used to identify patterns and relationships within the data, which can then be used to predict disease risk. These algorithms are trained using large datasets that contain both healthy individuals and those who have already developed the disease, enabling them to make accurate predictions for new individuals.
One of the key benefits of disease risk prediction technology is its ability to identify individuals who are at a high risk of developing certain diseases. This allows healthcare professionals to implement preventive measures, such as lifestyle changes or early screenings, to reduce the likelihood of disease development. By intervening early, the progression of the disease can be slowed down or even prevented entirely.
Furthermore, disease risk prediction technology can also aid in the development of personalized treatment plans. By understanding an individual's risk factors, healthcare professionals can tailor treatment approaches to suit their specific needs. This can lead to more effective and targeted treatments, minimizing adverse effects and improving patient outcomes.
Although disease risk prediction technology has shown great promise, it is important to note that it is not foolproof. Genetic and environmental factors play a significant role in disease development, and predictions may not always be accurate. Additionally, ethical considerations, such as data privacy and informed consent, must be taken into account when implementing these technologies.
In conclusion, the integration of technology in life sciences has revolutionized disease risk prediction. By analyzing patient data and genetic information, healthcare professionals can predict the risk of developing certain diseases, enabling early detection and personalized treatment approaches. This technology holds great potential for improving patient outcomes and reducing healthcare costs, but it must be used responsibly and in conjunction with other healthcare practices.
Comments:
Thank you all for reading my article on 'Exploring the Potential of ChatGPT in Disease Risk Prediction: Advancements in Life Sciences Technology'. I hope you found it informative and thought-provoking. I'm looking forward to your comments and insights!
Great article, Taren! The potential of ChatGPT in disease risk prediction is truly fascinating. It opens up new possibilities in the field of life sciences. I'm excited to see how this technology develops further.
Thank you, Laura! I agree, the advancements in life sciences technology are continuously pushing the boundaries. The applications of ChatGPT in disease risk prediction could have a significant impact on healthcare outcomes if properly developed and implemented.
I'm a bit skeptical about relying solely on ChatGPT for disease risk prediction. While it has potential, how can we ensure its accuracy and reliability? Human error is already a concern in such predictions, and AI systems are not immune to biases.
Valid concerns, Michael. The accuracy and reliability of ChatGPT in disease risk prediction are indeed critical factors that need to be thoroughly addressed. Validation, robust training, and addressing biases are important areas of focus to ensure trustworthy results.
I share your skepticism, Michael. Trusting AI systems with critical healthcare predictions is a huge responsibility, and we need robust validation and regulatory frameworks in place to ensure their reliability.
Indeed, Hannah. Trust is a crucial aspect, and it must be earned through transparency, rigorous validation, and regulatory oversight. The reliability of AI systems for healthcare predictions should be thoroughly evaluated before their widespread adoption.
I think ChatGPT can be a valuable tool in disease risk prediction, but it should be used in combination with other established methods. It can assist healthcare professionals in making informed decisions but shouldn't replace human judgement entirely.
Absolutely, Emily. ChatGPT should complement healthcare professionals rather than replace them. It has the potential to assist in analysis, provide additional insights, and contribute to the decision-making process. Collaborative efforts are the way to go.
I'm concerned about the ethical implications of using ChatGPT in disease risk prediction. How can we ensure patient privacy and prevent misuse of personal health data?
Ethical considerations are crucial, Chris. Privacy and data security must be prioritized when using ChatGPT for disease risk prediction. Proper policies, regulations, and informed consent protocols should be in place to safeguard patient information and prevent misuse.
This article opens up exciting possibilities for the future of healthcare. ChatGPT could potentially revolutionize disease risk prediction and improve preventive care strategies. However, we must remain cautious and diligent in its development and implementation.
I find it fascinating how machine learning and natural language processing technologies have advanced. Applying them to disease risk prediction can help identify patterns and correlations that humans might overlook. It's a promising area to explore further.
It's important to consider potential biases in the training data for ChatGPT. If the data used contains biases, it could lead to skewed predictions and discriminatory outcomes. Diversity and inclusivity should be prioritized when training these models.
You're absolutely right, David. Biases in training data can propagate into the model's predictions, leading to unintended discriminatory effects. Ensuring diverse and representative datasets and implementing bias mitigation techniques is crucial for ethical use.
ChatGPT has the potential to empower individuals by providing personalized disease risk predictions. It could help in raising awareness and promoting proactive healthcare behaviors. However, clear communication and interpretation of the results will be crucial to avoid unnecessary anxiety.
I'm curious about the scalability of ChatGPT for widespread disease risk prediction. What challenges do you foresee in implementing this technology on a larger scale?
Scalability is indeed a challenge, Daniel. As the user base grows, maintaining the system's performance and response times will be crucial. Additionally, collecting enough high-quality training data to cover a wide range of diseases and demographics would be another hurdle.
I have concerns about the transparency and interpretability of ChatGPT's predictions. Can users truly understand the reasoning behind the risk assessments and have confidence in the system's predictions?
Transparency and interpretability are legitimate concerns, Oliver. Ensuring that the predictions provided by ChatGPT can be explained and understood by users is crucial. Researchers are actively working on making AI systems more transparent and interpretable to build trust.
I believe ChatGPT's success in disease risk prediction heavily relies on the quality and diversity of the data it learns from. How can we ensure the datasets used for training adequately represent the population's demographics and healthcare scenarios?
You raise an excellent point, Sophia. Ensuring that the training datasets encompass diverse demographics and healthcare scenarios is crucial to avoid biased predictions that might not generalize well. Ongoing efforts are being made to improve dataset representativeness in AI applications.
Although ChatGPT's potential for disease risk prediction is exciting, we should always prioritize human expertise and judgment in healthcare decision-making. AI systems should aid professionals, not replace their expertise and experience.
Well said, Joshua. AI systems like ChatGPT have the potential to complement and enhance healthcare decision-making by providing valuable insights. Human expertise, empathy, and critical thinking are irreplaceable in ensuring the best possible outcomes for patients.
I'm concerned about the overreliance on AI in healthcare. While ChatGPT can assist in disease risk prediction, we must be cautious not to substitute it for thorough medical examination and consultation.
Valid concern, Liam. ChatGPT should be seen as a supporting tool rather than a replacement for comprehensive medical examination and consultation. It can provide additional insights but must be used responsibly and in conjunction with human expertise.
I agree, Liam. It's important for patients to have a holistic approach to their healthcare. While ChatGPT can be helpful, it should not be solely relied upon. Encouraging regular check-ups and consultations with healthcare professionals is essential.
The potential of ChatGPT in disease risk prediction is exciting, but we shouldn't overlook the challenges of integrating it into the existing healthcare infrastructure. Ensuring compatibility, security, and smooth adoption is crucial for its success.
Absolutely, Adam. Integration into the healthcare infrastructure is indeed a challenge. Compatibility, security, and regulatory considerations, along with proper training and support for healthcare professionals, are key for a successful adoption and implementation.
ChatGPT's potential in disease risk prediction is undeniable! It has the ability to analyze vast amounts of data quickly and can assist in identifying potential risks. Proper development, validation, and collaboration with healthcare providers will be crucial for its success.
I'm fascinated by the potential of ChatGPT, but I'm concerned about potential biases in its predictions. How can we ensure inclusivity and fairness when implementing this technology in disease risk prediction?
Valid concern, Olivia. To ensure inclusivity and fairness, it's important to have diverse representation in both the development teams and the datasets used for training. Additionally, implementing rigorous evaluation processes with fairness metrics is critical to detect and mitigate biases.
Excellent article, Taren! The potential applications of ChatGPT in disease risk prediction are vast. I'm excited to see how this technology can benefit patients and healthcare providers alike.
Really interesting read, Taren! ChatGPT can revolutionize disease risk prediction and pave the way for personalized healthcare interventions. Looking forward to future advancements in this field!
I believe ChatGPT in disease risk prediction can help in resource allocation and preventive measures. By identifying high-risk individuals, healthcare resources can be distributed more effectively, potentially reducing the burden on the system.
You make a great point, Matthew. ChatGPT can assist in prioritizing resources and implementing preventive measures. By identifying high-risk individuals early on, timely interventions can be made, potentially improving healthcare outcomes and reducing costs.
I'm curious about the computational resources required for running ChatGPT on a large scale. Are there any limitations or challenges associated with the system's computational requirements?
Great question, Noah. Running ChatGPT on a large scale can be computationally demanding. Adequate computational resources and optimization techniques are necessary to ensure smooth performance and response times, especially when handling a high volume of user inquiries.
The potential of ChatGPT in disease risk prediction is exciting, but we must consider the digital divide. Not everyone has equal access to healthcare technologies. How can we ensure equitable distribution and accessibility of ChatGPT in healthcare?
You raise an important concern, Ella. Ensuring equitable distribution and accessibility of healthcare technologies like ChatGPT is crucial. Collaborative efforts, public-private partnerships, and initiatives to bridge the digital divide are necessary for its widespread and equitable adoption.