Exploring the Potential of ChatGPT in Peptide Design: Revolutionizing Peptides Technology
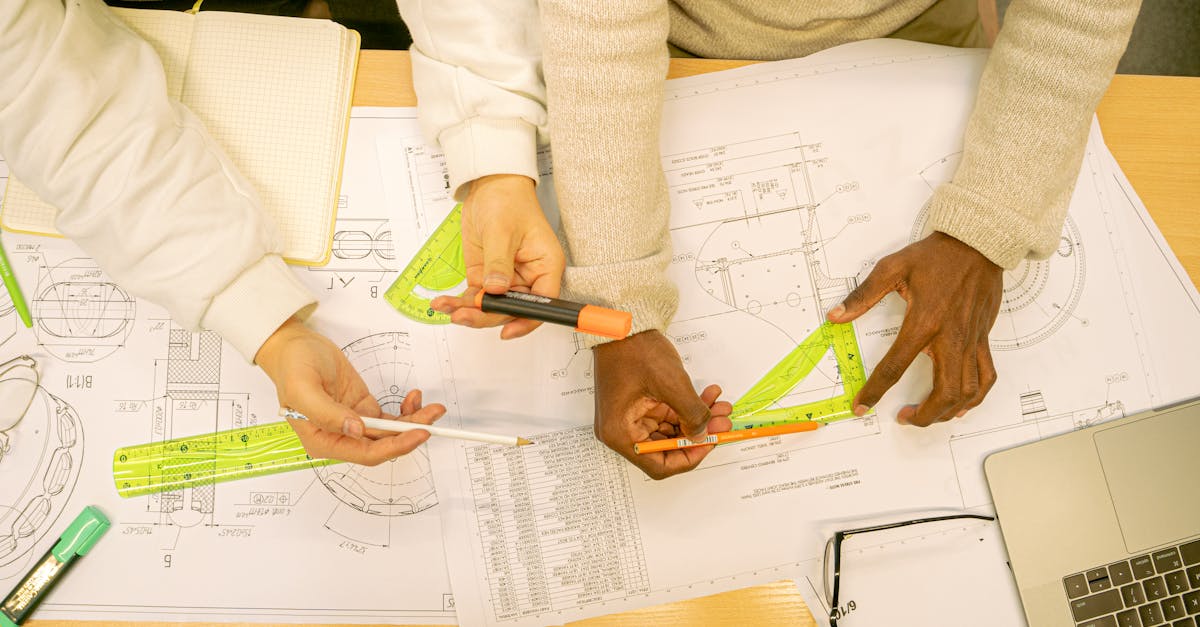
Peptides, short chains of amino acids, have gained significant attention in various fields, including medicine, biotechnology, and materials science. Due to their diverse chemical properties and ability to interact with biological systems, peptides have become promising candidates for drug development, biomaterials design, and more. However, designing new peptides with specific functional traits can be a time-consuming and challenging task.
The Role of AI in Peptide Design
Artificial Intelligence (AI) has revolutionized various industries, and it can also play a crucial role in peptide design. By integrating AI algorithms, researchers can harness the power of machine learning and computational modeling to expedite the process of designing new peptides.
Algorithm Development and Training
AI algorithms can be developed and trained using large datasets of known peptides and their associated properties or functionalities. These datasets allow the algorithms to learn patterns and correlations between peptide sequences and their desired traits. For example, if researchers want to design a peptide with improved stability, they can use a dataset of stable peptides to train the AI algorithm to recognize sequence characteristics that contribute to stability.
Predictive Modeling and Optimization
Once the AI algorithm is trained, it can predict the properties or functionalities of new peptide sequences. Researchers can input desired traits and the AI algorithm will generate peptide sequences that are likely to possess those traits. This predictive modeling approach eliminates the need for extensive trial-and-error experimentation and significantly accelerates the peptide design process.
Benefits of AI in Peptide Design
The integration of AI in peptide design offers several advantages:
Rapid Generation of Peptide Candidates
AI algorithms can quickly generate numerous peptide sequences based on defined traits. Researchers can then focus on analyzing and evaluating these candidate peptides, saving time and resources compared to traditional manual design approaches.
Exploration of Unexplored Sequence Space
AI algorithms can explore vast sequence space, allowing researchers to identify novel peptide sequences with desired traits that may not have been considered otherwise. This enables the discovery of peptides with improved functionality and opens up new possibilities for peptide-based applications.
Reduction of Experimental Trials
By utilizing AI algorithms, researchers can reduce the number of experimental trials required to develop a peptide with specific functionalities. This not only accelerates the design process but also minimizes the costs associated with extensive laboratory experimentation.
Challenges and Future Directions
While AI integration in peptide design holds immense promise, there are still challenges to be addressed. The quality and availability of peptide datasets for algorithm training, the complexity of peptide behavior, and the interpretation of machine learning results are among the obstacles that researchers need to overcome.
In the future, AI algorithms can be further improved by incorporating additional features such as novel representations of peptide structures or incorporating quantum chemistry-based predictions. This integration of various computational approaches has the potential to enhance the accuracy and efficiency of peptide design even further.
Conclusion
The integration of AI in peptide design brings unprecedented opportunities for streamlining the research process and accelerating the discovery of novel peptides with desired traits and functionalities. By leveraging the power of computational modeling, researchers can generate peptide candidates rapidly, explore unexplored sequence space, and reduce the number of experimental trials. While challenges remain, continuous advancements in AI and peptide research will pave the way for groundbreaking applications in medicine, biotechnology, and beyond.
Comments:
Thank you all for taking the time to read my article on exploring the potential of ChatGPT in peptide design. I'm excited to discuss this revolutionary technology with you!
This is a fascinating topic, Gabriel! I believe the application of ChatGPT in peptide design has the potential to significantly advance the field. The combination of AI and biotechnology is truly captivating.
I agree, Olivia. The ability to leverage AI to design peptides opens up new possibilities for developing targeted drugs and therapies.
However, I wonder about the limitations of ChatGPT in this context. Can it accurately predict the properties and interactions of peptides?
Great question, Sophie. While ChatGPT shows promise in peptide design, it's important to recognize that it may not always accurately predict the desired properties. It still requires fine-tuning and validation.
Gabriel, have there been any successful applications of ChatGPT in real peptide design projects so far?
There have been some notable successes, Sophie. ChatGPT has aided in generating peptide candidates with desired activities and improved selectivity in drug design projects. However, it's still an ongoing area of research and development.
Gabriel, do you foresee any challenges in convincing the scientific community to embrace AI-augmented peptide design?
Certainly, Nathan. While the potential is immense, gaining acceptance from the scientific community for new methodologies always comes with challenges. Rigorous validation and benchmarking against existing methods will be necessary.
Agreed, Gabriel. Validation is key to ensure the reliability and accuracy of AI-driven design methods.
Interesting, Gabriel. The versatility of AI-guided peptide design is truly remarkable!
You're right, Gabriel. Combining expertise from various domains will lead to responsible and impactful integration of AI in peptide design.
Absolutely, Sophie. Collaboration and interdisciplinary efforts can ensure the effective and safe utilization of AI in complex scientific domains like peptide design.
Gabriel, have there been any instances where ChatGPT's predictions have surpassed existing peptide design approaches?
Sophie, despite the limitations, I believe ChatGPT can serve as a valuable tool for exploring and generating peptide candidates. It can efficiently assist researchers in the initial stages of design.
You're right, Olivia. ChatGPT can aid in the design process by suggesting various possibilities, complementing existing approaches and accelerating initial exploration.
I think you're right, Sophie. AI is a powerful tool, but it's crucial to validate and refine its output before implementing it in real-world scenarios.
As a researcher in the field, I find ChatGPT intriguing. It could potentially speed up the peptide design process and aid in exploring novel sequences. Looking forward to seeing how this technology evolves!
I'm curious about the ethical considerations associated with AI-driven peptide design. For instance, should there be a regulatory framework to ensure safety and prevent misuse?
You bring up an important point, Emily. As with any new technology, ethical considerations are crucial. The development of regulations to ensure responsible use and prevent misuse should go hand in hand with the advancement of AI in peptide design.
Absolutely, Gabriel. Ethical considerations should be at the forefront to mitigate potential risks and ensure the responsible development and use of AI in peptide design.
I fully agree, Emily. We must proactively address ethical concerns to prevent unintended consequences.
Sophie and Emily, thank you for emphasizing the importance of ethics. The responsible integration of AI in peptide design must be a collective effort.
Gabriel, what are some potential applications of AI-guided peptide design in other areas apart from drug development?
Great question, Olivia. AI-augmented peptide design can have applications in agricultural science, material science, and even food industry, where tailored peptide sequences can enhance manufacturing processes and product properties.
Thank you for sharing, Gabriel. The potential impact of AI in diverse fields is exciting to envision.
Gabriel, what are the challenges in curating diverse peptide datasets, and how can we overcome them?
Thank you for the insights, Gabriel. Collaboration and shared resources are key to ensure a comprehensive dataset for training AI models in peptide design.
I'm also curious about the training data. How large and diverse is the dataset used to train ChatGPT for peptide design?
The training data for ChatGPT in peptide design consists of sequences and associated properties from various peptide databases. It's important to curate diverse and high-quality datasets to ensure broad applicability.
Are there any significant challenges that need to be overcome before AI-driven peptide design can become more widely adopted?
Certainly, Nathan. One of the challenges involves exploring the interpretability of AI models to understand the rationale behind their predictions. Additionally, increasing the model's efficiency and scalability are areas of active research.
Thank you, Gabriel. Overcoming these challenges will be crucial for the successful integration of AI in peptide design.
Gabriel, thank you for shedding light on the potential of ChatGPT in peptide design. It's exciting to witness the intersection of AI and biotechnology in advancing therapeutic approaches.
You're welcome, Sarah. The collaboration between AI and biotechnology indeed holds great promise, and I appreciate your enthusiasm!
Absolutely, Gabriel. The possibilities that lie ahead are truly remarkable, and I'm eagerly following the advancements in this field.
Validation and benchmarking against existing methods are crucial. We should ensure that AI is enhancing the peptide design process without compromising reliability.
Indeed, the scientific community demands robust validation of AI-driven methods to gain confidence in their reliability.
Curating diverse peptide datasets can be challenging due to the vast number of potential sequences. Collaboration between researchers, data sharing initiatives, and automated tools for data collection can help overcome these challenges.
Ethics should be at the core of AI development. It's essential to consider both the potential benefits and risks associated with this technology.
There have been cases where ChatGPT has suggested unique peptide sequences that showed promising results in initial studies. However, further validation and comparison with existing approaches are needed to establish its superiority.
Gabriel, thank you for introducing us to the potential of AI in peptide design. It's exciting to witness the advancements in this field!
You're welcome, Michael. I'm glad you find it exciting. The continuous progress in AI-driven peptide design opens up new opportunities for innovative drug development.
Indeed, Gabriel. The marriage of AI and biotechnology has the potential to revolutionize the pharmaceutical industry.
Thanks for clarifying, Gabriel. It'll be interesting to see how ChatGPT's predictions evolve and its potential impact on peptide design strategies.
Validation and reliability are indeed critical, David. AI should enhance our capabilities while maintaining accuracy and reproducibility.
Gabriel, can ChatGPT also be used to optimize existing peptides in terms of functionality and properties?
Absolutely, Michael. ChatGPT can offer insights and suggestions for optimizing existing peptides by exploring sequence modifications and predicting their potential effects on functionality and properties.
That's impressive, Gabriel. The ability to optimize existing peptides holds immense promise in various fields, from drug development to materials science.
Indeed, Michael. AI-guided optimization of existing peptides can unlock new possibilities and improve the effectiveness of peptide-based therapies.