Exploring the Potential of ChatGPT in Text-to-Speech Conversion for Computational Linguistics
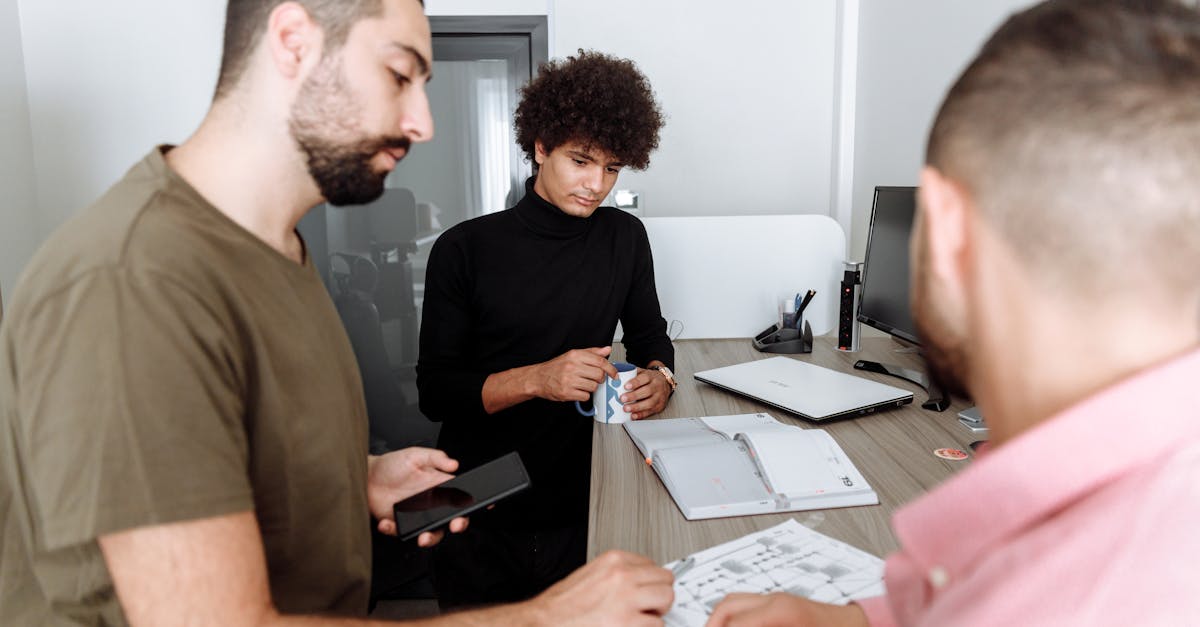
Computational Linguistics is an interdisciplinary field that combines principles of linguistics, computer science, and artificial intelligence to study human language and develop technologies that enable computers to process and understand natural language.
One of the key applications of Computational Linguistics is text-to-speech conversion, which involves converting written text into spoken words. This technology has made significant advancements in recent years, with the development of more sophisticated algorithms and neural network models.
Text-to-Speech Conversion with ChatGPT-4
ChatGPT-4 is a state-of-the-art language generation model developed by OpenAI. It is designed to generate conversational text that closely resembles human language. With its advanced natural language processing capabilities, ChatGPT-4 can produce high-quality text that can be converted to speech in the most human-like manner.
The usage of ChatGPT-4 in text-to-speech conversion offers several benefits. Firstly, it allows for the creation of more engaging and interactive user experiences. Applications that rely on written text can now provide audio output, making them more accessible to users with visual impairments or those who prefer audio content.
Moreover, ChatGPT-4's ability to generate natural-sounding speech can greatly enhance various applications, including voice assistants, customer service chatbots, virtual reality simulations, audiobook production, and language learning platforms. With the integration of text-to-speech conversion, these applications can provide a more immersive and realistic experience for users.
The Advancements in Text-to-Speech Technology
The field of text-to-speech conversion has evolved significantly over the years, driven by improvements in both speech synthesis techniques and the underlying computational models. Traditional methods, such as formant synthesis and concatenative synthesis, have been largely replaced by more advanced approaches, such as parametric synthesis and neural network-based models.
Parametric synthesis models use mathematical functions to generate speech signals based on linguistic and acoustic parameters. These models can generate speech that closely resembles human speech, but they often require significant manual tuning and may lack naturalness. However, recent advancements in neural network-based models, particularly using deep learning techniques, have revolutionized text-to-speech conversion.
Deep learning models, such as WaveNet and Tacotron, have shown remarkable progress in generating high-quality speech from text inputs. These models use neural networks to learn the complex relationships between text and speech, enabling them to produce more natural and expressive audio output. They are trained on large-scale datasets, allowing them to learn the intricacies of speech production and capture the nuances of human language.
The Future of Text-to-Speech Conversion
The continuous advancements in Computational Linguistics and text-to-speech technology hold great promise for the future. As researchers and engineers refine existing models and develop new techniques, we can expect even more accurate, natural, and emotionally expressive speech synthesis systems.
Furthermore, the integration of text-to-speech conversion technology with other AI-powered systems, such as machine translation, voice recognition, and sentiment analysis, will enable a wide range of applications that can understand, process, and generate spoken language effectively.
In conclusion, Computational Linguistics has greatly advanced the field of text-to-speech conversion. With ChatGPT-4's capability to generate human-like text, we can now convert that text into speech, improving the accessibility and user experience of various applications. As technology continues to evolve, we can expect further breakthroughs in text-to-speech conversion, ultimately bringing us closer to a more seamless interaction between humans and machines.
Comments:
Thank you all for taking the time to read and comment on my article! I'm excited to engage in this discussion.
Great article, Carine! I found your exploration of ChatGPT's potential in text-to-speech conversion fascinating.
I agree, Hannah. Carine, your insights about computational linguistics were quite enlightening.
I really enjoyed reading this article, Carine. It made me think about the possibilities that ChatGPT opens up for natural language processing.
Thank you, Hannah, Mark, and Emma, for your positive feedback! I'm thrilled that you found the article thought-provoking.
Carine, your article provides a clear overview of ChatGPT's application in the field of computational linguistics.
I found the section on ChatGPT's limitations and challenges quite interesting, Carine. It's important to consider the potential drawbacks.
Thank you, Liam and Sophia, for your kind words! Liam, I'm glad you found the overview helpful. Sophia, you're right, we must be aware of the limitations as well.
Carine, I appreciate your article's focus on the practical applications of ChatGPT in text-to-speech conversion. It gives a practical perspective.
Thank you, Oliver. I wanted to emphasize the real-world implications of ChatGPT's potential in computational linguistics.
Thinking about the future, Carine, do you see a time when ChatGPT's text-to-speech conversion could replace human voice actors in certain scenarios?
Oliver, while it's difficult to predict, ChatGPT has the potential to automate certain aspects of voice acting. However, the unique qualities of human voice actors may always have a place in certain scenarios.
Carine, apart from ethical guidelines, should society engage in a broader conversation about the impact of AI like ChatGPT in text-to-speech conversion?
Sophia, absolutely! Broader societal discussions are necessary to collectively shape the ethical and responsible deployment of AI, including its impact on text-to-speech conversion.
Carine, the involvement of diverse stakeholders will be crucial to avoid biases and ensure equitable representation in ChatGPT's text-to-speech conversion.
Sophia, diversity in stakeholder involvement is vital for building AI systems that are fair, unbiased, and serve everyone while minimizing potential risks.
Carine, it's wonderful how advancements in speech synthesis benefit both existing methods and AI models like ChatGPT, fostering overall progress.
That's true, Oliver! The advancements in both traditional methods and emerging AI models create a mutually beneficial environment, propelling the field forward.
As a computational linguistics student, Carine, your article gave me valuable insights into the practical uses of ChatGPT's text-to-speech conversion.
Carine, I must say your article got me excited about the possibilities of ChatGPT in speech synthesis for my research!
I found your article very well-researched, Carine. I appreciated the inclusion of real-world examples showcasing ChatGPT's potential.
Sophie, I'm glad the article resonated with you as a computational linguistics student. Nathan, that's fantastic! I hope ChatGPT proves useful for your research. Zara, I think including examples helps illustrate the practicality.
Carine, excellent article! I liked how you discussed the ethical considerations surrounding ChatGPT's deployment.
Thank you, Max. It's crucial to address ethical concerns when leveraging AI technologies like ChatGPT.
Carine, your article provided a comprehensive analysis of the use of ChatGPT in text-to-speech conversion. Well done!
Thank you, Lucy! I wanted to cover all the important aspects to provide a thorough understanding of ChatGPT's potential.
Carine, do you think the use of ChatGPT for text-to-speech conversion could surpass existing methods in terms of accuracy and naturalness?
Ryan, while ChatGPT has shown promising results, surpassing existing methods may require further advancements. However, it has the potential to bridge the gap.
Carine, have there been any real-world applications of ChatGPT's text-to-speech conversion in computational linguistics so far?
Olivia, while the technology is still in development, researchers have started exploring its applications, such as assisting people with speech disorders.
Carine, how can we address the limitations you discussed and improve the performance of ChatGPT in text-to-speech conversion?
Emily, tackling the limitations involves refining the training process, incorporating domain-specific data, and implementing user feedback loops.
Carine, I'm curious, do you think ChatGPT's text-to-speech conversion capabilities could impact industries like audiobook production?
Tom, absolutely! ChatGPT could potentially automate parts of the audiobook production process, making it more efficient and cost-effective.
Carine, how can we ensure AI deployment in text-to-speech conversion adheres to ethical principles?
Alex, it's crucial to establish guidelines, conduct rigorous testing, and involve diverse stakeholders to ensure AI is deployed responsibly and respects ethical principles.
Carine, it would be interesting to know how existing speech synthesis methods are adapting to the advancements like ChatGPT.
Tom, existing methods are also being improved, learning from models like ChatGPT and finding ways to integrate their strengths into established frameworks.
Carine, your article highlights the importance of inclusive development and ethical considerations in ChatGPT's text-to-speech conversion.
Thank you, Tom. It's crucial for the AI community and developers to prioritize inclusive development practices and address ethical considerations.
Carine, have there been any studies comparing ChatGPT's text-to-speech conversion with other existing methods?
Emily, while there are ongoing studies, it's still an emerging field. However, initial results indicate the potential of ChatGPT's text-to-speech conversion in certain domains.
Carine, it's fascinating to think about the possibilities of ChatGPT's text-to-speech conversion in different industries, like video game development.
Indeed, Nathan. ChatGPT's capabilities could streamline voiceover work in video game development, reducing production time and costs.
Carine, I couldn't agree more. Inclusive development practices will lead to AI systems that empower individuals and respect diverse needs.
Absolutely, Emily! By embracing inclusivity, we can ensure that AI systems like ChatGPT's text-to-speech conversion serve everyone and contribute to a more equitable future.
Carine, your article succeeded in sparking interesting discussions on ChatGPT's text-to-speech conversion. It's been a pleasure to participate.
Thank you, Emily. I'm delighted that the article fostered engaging discussions, and I appreciate your active participation.
Thanks for sharing, Carine. I'm excited to see how ChatGPT's text-to-speech capabilities find practical use in various domains.
You're welcome, Olivia. The potential applications are indeed exciting, and I believe we'll witness further advancements in the coming years.
Carine, it's inspiring how ChatGPT can assist those with speech disorders. The impact it could have on accessibility is remarkable.
Absolutely, Emma! The accessibility aspect is one of the most promising aspects of ChatGPT's text-to-speech conversion.
Carine, incorporating user feedback loops sounds like an effective approach. It could help refine the system's performance in different contexts.
Sophie, user feedback is invaluable for system improvement and addressing user-specific requirements. It plays a crucial role in enhancing ChatGPT's performance.
Carine, your article provided valuable insights into the potential of ChatGPT in text-to-speech conversion. It was an enlightening read.
I'm glad you found it enlightening, Sophie! It was my aim to offer insights into ChatGPT's application in computational linguistics.
Carine, thank you once again for sharing your expertise on ChatGPT's potential in computational linguistics. I look forward to future articles.
You're welcome, Sophie. It was a pleasure to share my insights. I'm excited to continue exploring and writing about AI's advancements in the future.
Ryan, accuracy and naturalness in text-to-speech conversion depend on factors like data quality and system optimization. ChatGPT has the potential to improve both.
James, that makes sense. It'll be interesting to see how the technology progresses and its impact on this field.
James, implementing user feedback and continuous learning will likely play a vital role in improving the accuracy and naturalness of ChatGPT's text-to-speech conversion.
Liam, I agree. Continuous improvement through feedback iterations is crucial for refining AI systems like ChatGPT's text-to-speech conversion.
Liam and James, the collaboration between users and developers would ensure the practicality and effectiveness of ChatGPT's text-to-speech conversion.
Emma, exactly! Collaborative efforts help tailor AI systems to meet user needs and maximize their benefits.
Carine, involving users in the development process can help identify system biases and ensure the inclusivity of ChatGPT's text-to-speech conversion.
Lucy, user involvement during development is essential for addressing biases, ensuring inclusivity, and creating a system that serves a wide range of users.