Exploring the Potential of Gemini in Facilitating Discovery of Protein-Protein Interactions in Technology
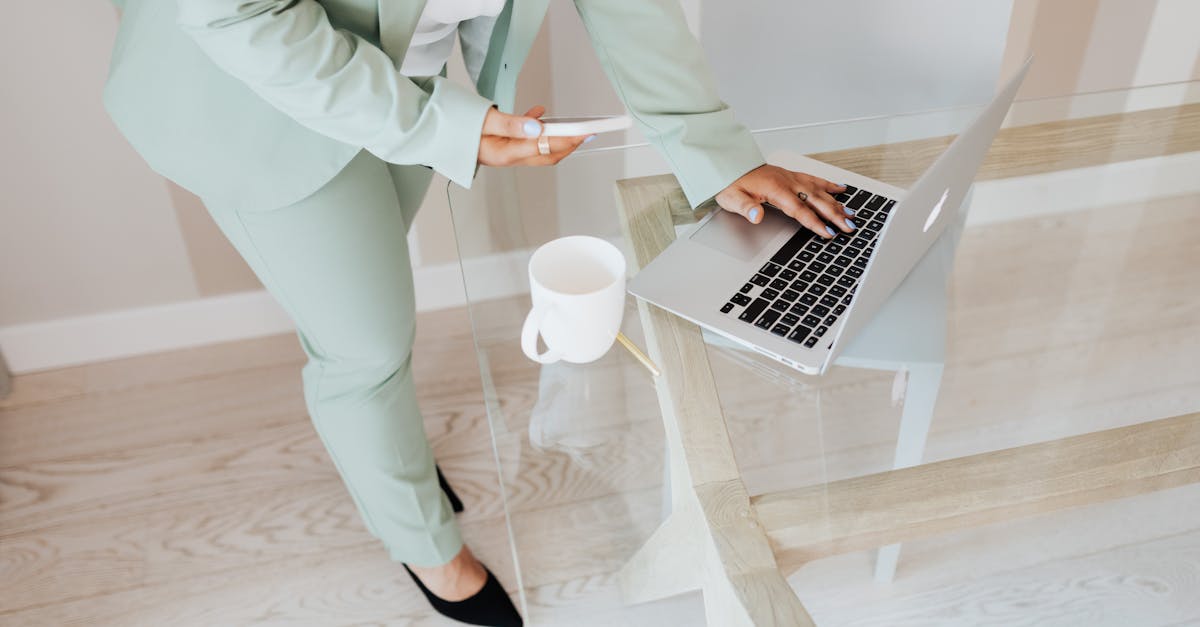
Protein-protein interactions (PPIs) play a vital role in understanding the mechanisms behind various biological processes and diseases. The identification of PPIs has traditionally been a laborious process involving experimental techniques such as yeast two-hybrid assays or co-immunoprecipitation followed by mass spectrometry. However, advancements in technology have opened up new possibilities, and recently, artificial intelligence-based approaches have gained prominence in facilitating the discovery of PPIs.
One such technology, Gemini, a language model developed by Google, has demonstrated great potential in assisting biologists and researchers in exploring PPIs. Gemini is an AI program capable of engaging in natural language conversations. By leveraging its capabilities, researchers can interact with Gemini using input queries related to protein interactions and extract valuable information.
The benefits of using Gemini in exploring PPIs are numerous. Firstly, it provides a powerful tool for hypothesis generation. By conversing with Gemini and asking specific questions, researchers can explore PPIs that were previously unknown or not extensively studied. This enables the discovery of new connections and potential mechanisms in biological systems.
Moreover, Gemini can assist in interpreting experimental data by providing insights and suggestions. Researchers can input experimental results or observations and seek explanations or additional information from Gemini. This can potentially save time and resources by generating preliminary interpretations, which can then be further validated through experiments.
Furthermore, Gemini can help researchers stay up-to-date with the latest developments in the field of PPIs. By asking Gemini about recent publications, conferences, or even specific research groups, researchers can quickly access relevant information, stay informed, and collaborate with other experts in the field.
It is important to note that while Gemini provides valuable assistance, it should be used as a complementary tool and not a replacement for experimental validation. The predictions and information provided by Gemini should be critically evaluated and further investigated through traditional experimental techniques to ensure their accuracy and reliability.
In conclusion, the potential of Gemini in facilitating the discovery of PPIs in technology is immense. Its ability to engage in natural language conversations and provide valuable insights makes it a valuable tool for researchers exploring protein interactions. By leveraging Gemini, researchers can generate hypotheses, interpret experimental data, and stay informed about the latest developments in the field. As technology continues to advance, AI-based approaches like Gemini will undoubtedly play a significant role in furthering our understanding of PPIs and accelerating scientific discovery.
Comments:
Thank you all for your comments on my article! I appreciate the engagement.
Great article, Ryan! I found the potential of Gemini in facilitating discovery of protein-protein interactions fascinating. It could have a significant impact on the field of technology.
I agree, Emily. The ability of Gemini to generate hypotheses and provide valuable insights can definitely contribute to advancing our understanding of protein-protein interactions.
It's interesting to see how artificial intelligence can be applied to such complex problems. However, I wonder about the limitations and potential biases of Gemini in this context.
That's a valid concern, Alex. Gemini, like all AI models, can indeed have limitations and biases. In the case of protein-protein interactions, it's crucial to thoroughly validate and verify the generated hypotheses.
I have some experience in protein research, and I think Gemini can provide a valuable starting point for exploring new avenues and generating novel hypotheses. However, it should always be followed by rigorous experimental procedures.
Absolutely, Natalie! Gemini can serve as a tool to spark new ideas and guide research directions, but validating the hypotheses through experiments is essential.
I'm curious about the scalability of using Gemini for this purpose. Can it handle the vast amount of data and complexity involved in studying protein-protein interactions?
Scalability is indeed a challenge, Samuel. Currently, Gemini's capabilities may be more suitable for exploratory research and generating focused hypotheses rather than analyzing large-scale datasets.
This article got me thinking about potential ethical considerations of using AI in scientific research. We should ensure that it is used responsibly and doesn't replace the human element of expertise.
You raise an important point, Sophia. AI should complement human expertise, not replace it. Proper oversight and collaboration between AI systems and domain experts are crucial for responsible usage.
I'm impressed by the possibilities of Gemini! It could support scientists who don't have domain-specific expertise in protein-protein interactions and help bridge knowledge gaps.
Absolutely, Daniel! Gemini has the potential to democratize access to preliminary insights and enable researchers from various domains to contribute to the study of protein-protein interactions.
I wonder if Gemini could be trained on more specialized datasets to improve its accuracy and generate more relevant hypotheses in the context of protein-protein interactions.
That's a great suggestion, Emma! Fine-tuning Gemini on domain-specific datasets could enhance its performance and make it more applicable to the study of protein-protein interactions.
Kudos to the author for exploring such an innovative application of AI! It's refreshing to see novel uses of technology in scientific domains.
Thank you, Oliver! The potential of AI in scientific research is immense, and I'm glad to contribute to its exploration.
I'm intrigued by the potential of Gemini for drug discovery. It could help identify possible interactions between drugs and proteins, potentially leading to more efficient pharmaceutical development.
You're absolutely right, Sophie! Gemini can have applications in multiple areas, including drug discovery. Its ability to generate hypotheses can aid in identifying potential drug targets and interactions.
While the potential of Gemini is exciting, we should also consider the limitations of using AI models. They are only as good as the data they are trained on. How can we ensure biases in the data won't affect the generated hypotheses?
That's a crucial concern, Grace. Addressing biases in data is crucial for ensuring the integrity of AI-generated hypotheses. Careful curation and evaluation of training data, as well as ongoing monitoring, are necessary to mitigate bias.
I'm curious about potential privacy issues when using Gemini for scientific research. What measures can be taken to protect sensitive data when using AI models?
Privacy is indeed important, Elijah. When using AI models, researchers must follow stringent privacy protocols, anonymize data, and ensure secure storage and usage to protect sensitive information.
I can see Gemini becoming a valuable tool for collaboration among researchers. It could facilitate knowledge-sharing and foster interdisciplinary cooperation.
Absolutely, Brandon! Gemini's collaborative potential can bridge gaps between disciplines, facilitate knowledge transfer, and enable researchers to pool their expertise.
The use of AI models like Gemini brings up questions about intellectual property and ownership of the generated insights. How can these concerns be addressed?
You raise an important point, Victoria. Clear guidelines and agreements should be in place to address intellectual property rights, ensuring fair attribution and collaboration when using AI models in research.
This article made me consider the potential impact of Gemini on the pace of scientific discoveries. With its ability to generate hypotheses, it could accelerate the process of exploring new insights.
Indeed, Liam! Gemini has the potential to speed up the early stages of research by quickly exploring various hypotheses, allowing researchers to focus their efforts on the most promising avenues.
Are there any examples where Gemini has been applied to protein-protein interactions? If so, I'd love to learn more about the outcomes and any challenges faced.
Great question, Chloe! While Gemini is a relatively new AI model, there have been initial explorations of applying it to protein-protein interactions, primarily as a tool for hypothesis generation. Ongoing research is being conducted to evaluate its outcomes and address potential challenges.
I always appreciate articles that shed light on the practical applications of AI in scientific domains. It's exciting to witness the continuous advancements in this field.
Thank you, Eva! AI's potential impact on science is vast, and it's essential to explore and evaluate its applications in diverse domains to harness its benefits fully.
One concern that comes to mind is the interpretability of results generated by Gemini. How can we ensure that the hypotheses it generates are understandable and explainable?
Interpretability is indeed an important aspect, Lucas. Efforts are underway to develop techniques that provide explanations for AI model outputs, ensuring greater transparency and enabling researchers to understand how the hypotheses are generated.
I applaud the potential of Gemini in accelerating scientific discoveries. It could be a game-changer, especially when it comes to exploring complex biological systems and identifying protein interactions.
Thank you, Olivia! The complexity of biological systems makes them a suitable domain for AI assistance, and Gemini's potential in aiding the exploration of protein interactions is exciting.
As promising as Gemini is, I believe it should be used as a tool alongside human experts rather than a standalone solution. The collaboration between AI and scientists can yield more reliable and impactful results.
I completely agree, Jackson! The combination of AI and human expertise creates a powerful synergy, enabling us to leverage the strengths of both for more robust and reliable scientific outcomes.
I'm excited to see how Gemini and similar AI models will evolve in the future. The advancements in this field have the potential to revolutionize scientific research.
Indeed, Isabella! The continuous development and refinement of AI models like Gemini hold great promise for transforming scientific research and accelerating discoveries.
This article has sparked my curiosity about the potential challenges faced when applying Gemini to protein-protein interactions. Are there any specific hurdles that need to be addressed?
Great question, Ethan! Some challenges include the integrity of generated hypotheses, ensuring scalability, addressing biases, and fine-tuning the model on domain-specific datasets. Ongoing research and collaborations aim to tackle these hurdles.
I appreciate the author's emphasis on the importance of experimental validation. While Gemini can generate hypotheses, it's through experimentation that we can truly verify and gain confidence in its predictions.
Absolutely, Lily! Experimental validation remains a crucial step to confirm and refine the hypotheses generated by Gemini, ensuring the reliability and accuracy of the findings.
I'm impressed by the potential of AI models to contribute to scientific discoveries. Gemini's ability to generate hypotheses effectively makes it a valuable tool for researchers working on protein-protein interactions.
Thank you, Noah! AI models have indeed become powerful allies for scientists. The ability of Gemini to generate hypotheses can aid in guiding research efforts and accelerating the discovery of protein-protein interactions.
I'm curious about the collaboration between AI models like Gemini and other emerging technologies, such as virtual reality or augmented reality, to enhance scientific research experiences. Is there any ongoing exploration in that direction?
That's an interesting idea, Ava! While I'm not aware of specific ongoing research in that direction, the combination of AI models and emerging immersive technologies could definitely enhance the research experience, enabling intuitive data exploration and manipulation.
As exciting as Gemini's potential is, it's important to be mindful of the ethical implications and societal impact of its usage. Responsible AI practices should always be followed to avoid unintended consequences.
You're absolutely right, Sophia! Ethical considerations and responsible usage of AI models should be at the forefront of scientific research. Ensuring transparency, fairness, and addressing potential biases are crucial in harnessing AI's potential for positive impact.
Thank you all for taking the time to read my article on the potential of Gemini in facilitating the discovery of protein-protein interactions in technology. I'm excited to hear your thoughts and engage in a discussion!
Great article, Ryan! It's fascinating to see how AI technologies like Gemini can bring innovation to the field of protein-protein interactions. I'm curious about the specific applications you foresee for Gemini in this context?
Thanks, Mary! The potential applications of Gemini in this area are numerous. One key area is in assisting biologists and researchers in predicting novel protein-protein interactions, which could lead to the discovery of new therapeutic targets. Gemini can also aid in the interpretation of complex experimental data and provide insights for experimental design. Overall, it has the potential to accelerate the discovery process and drive scientific breakthroughs.
Hi Ryan! This is an intriguing idea. However, I'm concerned about the limitations of using Gemini for such tasks. How can we ensure the accuracy and reliability of the predicted protein-protein interactions?
Hi Alex! Valid concern indeed. While Gemini can provide valuable insights, it's crucial to have validation and experimental confirmation for the predicted interactions. Integrating Gemini with existing experimental methods and leveraging multiple AI models for validation can help mitigate any potential inaccuracies.
Interesting article, Ryan! I wonder if Gemini can also be used to uncover previously unknown protein functions based on their interactions with other proteins.
Hi Sarah! Absolutely, Gemini can play an essential role in uncovering new protein functions. By analyzing the interactions between proteins, we can gain insights into their potential roles and cellular processes. This can support the discovery of protein functions that were previously unknown or poorly understood, contributing to our understanding of biological systems.
Nice article, Ryan! One concern I have is the interpretability of Gemini's predictions. How do we ensure that the generated explanations for protein-protein interactions are easily understandable and transparent?
Hi Mark! Valid point. Interpretable explanations are crucial, especially in scientific domains. We can address this challenge by incorporating techniques like attention mechanisms, highlighting the key components of the interaction, or using explainable AI approaches to provide insights into the Gemini's decision-making process. Making the explanations more transparent would greatly enhance the utility and trust in the system.
Great work, Ryan! Do you think Gemini could eventually replace traditional experimental methods in studying protein-protein interactions, or would it rather serve as a complementary tool?
Hi Emily! Thank you! While Gemini is promising, it's unlikely to replace traditional experimental methods entirely. Instead, it can serve as a powerful complementary tool to augment human intelligence. By combining the strengths of AI with experimental techniques, we can accelerate the research process, generate hypotheses, and guide experimental design more efficiently.
Ryan, congratulations on the article! I'm wondering how Gemini can handle the vast complexity of protein-protein interactions. Can it effectively handle large-scale datasets and provide meaningful insights?
Thank you, Michael! Gemini can indeed handle large-scale datasets. By training on extensive databases and integrating multiple knowledge resources, it can capture the complexity of protein-protein interactions. It can then provide meaningful insights and predictions, facilitating the exploration of complex biological systems even on a large scale.
I enjoyed reading your article, Ryan! What are your thoughts on potential ethical concerns associated with using AI like Gemini in scientific research and its impact on human researchers?
Hi Daniel! Ethical concerns are essential to address when deploying AI in scientific research. While AI can augment human researchers, it's crucial to ensure transparency, fairness, and accountability in its use. Researchers should be aware of AI's limitations, interpret its outputs critically, and actively monitor and validate its predictions. Collaboration between AI and human experts can help address these concerns and leverage the full potential of such technologies.
Fascinating read, Ryan! How do you envision the future development of Gemini and its potential role in advancing the understanding of protein-protein interactions?
Hi Sophia! The future of Gemini holds immense promise. It will likely undergo further advancements, incorporating domain-specific knowledge, and benefiting from improved training data. Intelligent integration with experimental data and interaction databases can enhance its accuracy and reliability. With these advancements, Gemini can better assist researchers in the discovery of novel protein-protein interactions, unravel complex biological mechanisms, and drive advancements in biotechnology and medicine.
Thanks for sharing this article, Ryan! How does Gemini handle the inherent noise and uncertainty in experimental data, particularly in the context of protein-protein interactions?
Hi Tom! Coping with noise and uncertainty is crucial in the analysis of experimental data. Gemini can handle these challenges by leveraging probabilistic reasoning and employing techniques such as Bayesian inference to quantify uncertainty. By contextualizing the noise and understanding its implications, Gemini can generate predictions and insights that take the noise into account, aiding in dealing with the inherent uncertainties of experimental data.
Great article, Ryan! What are the current limitations of Gemini in its application to protein-protein interaction studies?
Thanks, Linda! While promising, Gemini still has some limitations. One challenge is the lack of sufficient high-quality training data, especially when dealing with rare or unexplored protein interactions. Additionally, the interpretability of the AI-generated insights and managing potential biases are areas that require further research and development. Nonetheless, ongoing advancements in AI and collaborative efforts can help overcome these limitations, ensuring its effective utilization in protein-protein interaction studies.
Ryan, your article is thought-provoking! How do you see the integration of Gemini with existing protein-protein interaction databases and resources?
Hi David! Integrating Gemini with existing databases and resources is a crucial step towards its practical use. By combining AI-generated insights with curated protein-protein interaction databases, we can improve the reliability and accuracy of the predictions. Moreover, integrating Gemini with resources like protein ontologies and functional databases could enhance our understanding of protein-protein interactions and shed light on their roles in biological systems.
Well-written article, Ryan! Are there any legal or privacy implications that need to be considered when using AI like Gemini in protein-protein interaction studies?
Hi Lisa! Legal and privacy implications are always important to consider. When applying AI like Gemini, researchers must ensure compliance with data protection and privacy regulations. Access to sensitive or personally identifiable information needs to be handled carefully, employing adequate anonymization and security measures. Strict adherence to ethical guidelines and policies can help address these concerns and ensure responsible and privacy-conscious use of AI in protein-protein interaction studies.
Interesting topic, Ryan! How can Gemini contribute to the discovery of potential drug targets in protein-protein interactions?
Hi Olivia! Gemini can play a significant role in identifying potential drug targets by predicting novel protein-protein interactions implicated in specific diseases or pathologies. By analyzing the interactions within disease-related networks, Gemini can identify likely candidate proteins involved in these interactions. These proteins can then be further explored as potential drug targets, providing valuable insights for therapeutic development.
Ryan, great article! What are the main challenges in training Gemini for protein-protein interaction studies, and how can these be addressed?
Thanks, Chris! One of the main challenges lies in obtaining high-quality training data that adequately covers the vast landscape of protein-protein interactions. Efforts in curating and expanding publicly available databases specific to protein-protein interactions can address this data limitation. Additionally, collaborations between AI researchers and domain experts can help in integrating the relevant biological context into the training process, ensuring that Gemini captures the nuances and specificities of protein interactions accurately.
Ryan, excellent insights! What are the computational requirements for deploying Gemini in protein-protein interaction studies on a large scale?
Hi Grace! Deploying Gemini on a large scale requires significant computational resources. Training and fine-tuning the model can be computationally intensive, and inference for complex protein-protein interaction predictions might require high-performance computing systems. However, with advancements in hardware and optimization techniques, the computational requirements can be efficiently managed, making it feasible to integrate Gemini into large-scale protein-protein interaction studies.
Great piece, Ryan! How can Gemini be validated and benchmarked regarding its performance in predicting protein-protein interactions?
Thanks, Sophie! Validating and benchmarking Gemini's performance is crucial to ensure its reliability. One approach is to use experimentally validated protein-protein interaction datasets for testing its predictions against known interactions. Additionally, metrics like precision, recall, and F1 score can be employed to assess the model's performance. Model comparison against existing state-of-the-art methods can also help evaluate its predictive capabilities and identify areas for improvement.
Hi Ryan! Are there any specific design considerations when using Gemini to predict protein-protein interactions, such as addressing biases or training on diverse protein families?
Hi Samuel! Design considerations are indeed important. It's crucial to ensure a balanced representation of protein families during training to avoid biases towards commonly studied proteins. By training on diverse protein families and incorporating unbiased datasets, we can increase the model's ability to generalize and predict interactions beyond the well-studied proteins. Additionally, techniques like active learning can be employed to selectively prioritize or correct biases during the model's training process.
Ryan, interesting article! How do you envision the collaboration between human researchers and AI models like Gemini in the future?
Hi Sophia! Collaboration between human researchers and AI models like Gemini holds immense potential. Human researchers can provide critical domain knowledge, interpret and validate the model's predictions, and effectively incorporate AI-generated insights into the research process. In turn, Gemini can augment human intelligence, improve research efficiency, and provide new perspectives and hypotheses for further investigation. Successful collaboration between humans and AI can lead to accelerated scientific discoveries and advancements.
Ryan, great article! Could Gemini be adapted to study other types of molecular interactions, such as protein-nucleic acid interactions?
Hi Ella! Absolutely! Gemini can be adapted to study other molecular interactions beyond protein-protein interactions. By training the model with relevant datasets, it can learn to analyze and predict protein-nucleic acid interactions or interactions between other biomolecules. Expanding its capabilities to study diverse molecular interactions can contribute to our understanding of complex biological phenomena and facilitate various applications in biotechnology and medicine.
Impressive work, Ryan! How does Gemini handle the vast amount of existing literature and incorporate it into its predictions in the field of protein-protein interactions?
Thanks, John! Gemini can leverage the vast amount of existing literature and scientific knowledge through pre-training on large text corpora. By integrating this knowledge into its understanding of protein-protein interactions, Gemini can generate insights and predictions that align with existing scientific literature. However, it's important to note that the model's outputs require careful evaluation, as they might not always align perfectly with the latest research findings and methodologies.
Ryan, your article is intriguing! How can Gemini potentially contribute to the study of protein-protein interactions in the context of rare diseases or lesser-explored biological systems?
Hi David! Gemini can play a significant role in the study of protein-protein interactions in the context of rare diseases or lesser-explored biological systems. By training on diverse datasets or incorporating transfer learning techniques from related domains, such as well-studied diseases or model organisms, Gemini can generate hypotheses and predictions specific to these contexts. This can shed light on protein interactions that might be unique to such cases, aiding in the understanding and potential treatment of rare diseases.
Great article, Ryan! How can the outputs from Gemini be integrated with other computational methods or experimental techniques to enhance the discovery of protein-protein interactions?
Thanks, Jessica! Integration of Gemini's outputs with other computational methods and experimental techniques is crucial for enhancing the discovery of protein-protein interactions. For example, the predictions made by Gemini can be validated through targeted experimental verification or by leveraging other computational models specifically designed for protein-protein interaction analysis. Such integration can provide a holistic approach towards uncovering novel interactions and building a comprehensive understanding of biological networks.
Ryan, your article is fascinating! How do you see the potential adoption and readiness of Gemini in the biological research community?
Hi Emma! Adoption of Gemini and similar AI technologies in the biological research community depends on multiple factors. Ensuring its readiness requires addressing concerns related to interpretability, validation, and incorporation into existing research practices. Collaborative efforts between AI researchers, biologists, and bioinformaticians can contribute to improving and demonstrating the effectiveness of Gemini, fostering trust and confidence within the research community. Demonstrating its utility through successful case studies and continuous improvements can drive its adoption in the study of protein-protein interactions.
Fantastic article, Ryan! Do you think Gemini can eventually be extended to predict more complex biological interactions beyond just protein-protein interactions?
Hi Sophia! Definitely! Gemini's potential can extend beyond protein-protein interactions. With advancements in AI and the availability of diverse datasets, it can be adapted to predict complex biological interactions involving proteins, nucleic acids, carbohydrates, and other biomolecules. By training the model accordingly and incorporating domain-specific knowledge, we can leverage the power of AI to gain insights into various types of biological interactions, accelerating our understanding of complex biological systems.
Thank you all for your insightful comments and questions! I appreciate your engagement and enthusiasm in exploring the potential of Gemini in facilitating the discovery of protein-protein interactions. Your feedback and ideas contribute to the ongoing research and development in this area. If you have any further thoughts or queries, feel free to share!