Gemini: Revolutionizing Technology Categorization through Conversational AI
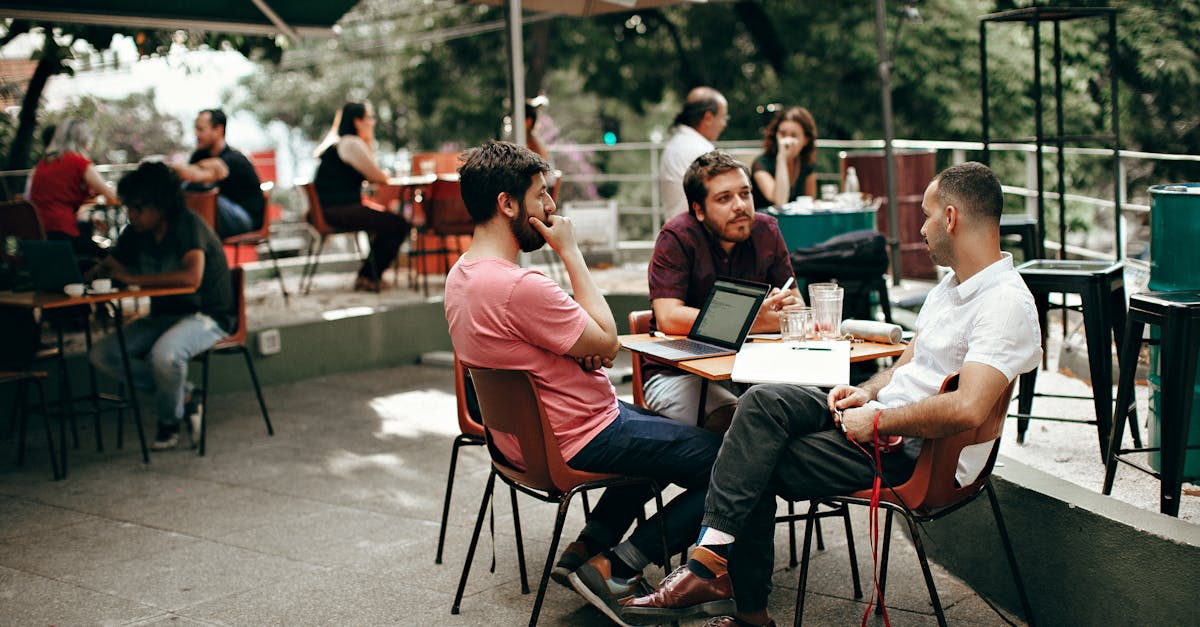
Conversational AI has taken the tech industry by storm, and one of the most notable advancements in this field is Gemini. Developed by Google, Gemini is an advanced language model that has revolutionized technology categorization.
The Power of AI in Technology Categorization
Technology categorization plays a crucial role in various domains, including research, customer support, and content management systems. Traditionally, technology categorization has been performed manually, which is time-consuming and prone to errors. However, with the advent of conversational AI, this process has become much more efficient and accurate.
Gemini is built on Google's powerful LLM model, which stands for Generative Pre-trained Transformer. LLM is a state-of-the-art language model that has been trained on a vast amount of data from the internet. With its advanced natural language processing capabilities, LLM can understand and generate human-like text.
How Gemini Transforms Technology Categorization
Using Gemini, technology categorization can be done through a simple conversation with the AI model. Instead of manually going through each piece of technology and categorizing it, users can now interact with Gemini and let it handle the categorization process.
The conversational nature of Gemini makes the categorization process more intuitive and user-friendly. Users can ask questions, provide descriptions, and discuss various aspects of the technology they want to categorize. Gemini responds in a conversational manner, providing suggestions and insights based on its extensive knowledge base.
The flexibility of Gemini allows it to adapt to different use cases and industries. Whether it's categorizing programming languages, software frameworks, or hardware components, Gemini can handle a wide range of technology-related categorization tasks.
Benefits of Gemini in Technology Categorization
Gemini brings several benefits to technology categorization:
- Time-saving: Gemini eliminates the need for manual categorization, saving valuable time for businesses and researchers.
- Accuracy: With its extensive training on a diverse dataset, Gemini offers more accurate categorization results compared to traditional methods.
- Scalability: Gemini can handle a large number of technology categorization tasks simultaneously, making it suitable for enterprises with vast tech inventories.
- User-friendly: The conversational interface of Gemini makes it accessible to users without technical expertise, enabling wider adoption across various industries.
The Future of Gemini
As Gemini continues to evolve, it holds immense potential for technology categorization. Google is constantly working on improving the model's capability to provide more accurate suggestions and insights. With its ability to understand natural language text, it can better adapt to user queries and enhance the categorization process further.
The technology industry is evolving at a rapid pace, and having an advanced AI model like Gemini can significantly streamline the categorization process. With its conversational AI approach, Gemini is transforming the way technology is classified and organized.
Comments:
Thank you all for reading my article! I'm excited to hear your thoughts on Gemini and its potential in technology categorization.
Great article, Jeremy! Gemini definitely seems like a game-changer for technology categorization. The ability to have conversational AI that understands context is impressive.
I agree, Emily! Gemini's conversational capabilities make it stand out. It could greatly enhance the accuracy and efficiency of technology categorization.
The potential for Gemini is immense, no doubt. But how does it handle complex technical jargon and specific terminology?
That's a great question, Michael. Gemini is trained on a vast amount of internet text, so it has exposure to technical jargon. However, it may still struggle with highly specialized or domain-specific terms.
I'm curious to know how Gemini differs from traditional algorithms used for technology categorization. Could you explain that, Jeremy?
Of course, Natalie. Traditional algorithms rely on predefined rules, patterns, or statistical models. Gemini, on the other hand, uses deep learning techniques and is trained to generate human-like responses based on context, making it more adaptable and able to handle diverse inputs.
Jeremy, do you have any insights into how Gemini can be implemented in real-world technology categorization tasks?
Absolutely, Sarah. Gemini can be integrated into existing technology categorization systems as an additional step to refine results. It can also assist in user support, answering queries related to technology categorization, and ensuring better user experiences.
While the idea of Gemini sounds promising, what are some potential challenges or limitations we should consider?
A valid concern, Robert. Gemini may generate plausible-sounding but incorrect responses. It could also have difficulty handling ambiguous queries. Close supervision and iterative improvements are necessary to address these challenges.
I'm concerned about bias. Gemini learns from internet text which can be biased. Is there any mechanism to mitigate this?
You raise an important point, Julia. Google is working to improve system behavior and address biases. They use reinforcement learning from human feedback (RLHF) to reduce biases, and they actively seek user feedback for improvements.
I can see the potential of Gemini in technology categorization, but how does it perform when dealing with a wide range of languages?
Good question, David. Gemini has been primarily trained on English text, so it performs best with that language. However, Google is working on expanding its language capabilities in the future.
I think the ability of Gemini to generate human-like responses is impressive. But how can we ensure the responses are accurate and reliable?
Valid concern, Melissa. Google uses techniques like RLHF and human reviewers to train and fine-tune Gemini, ensuring more accurate and reliable responses over time.
Do you have any metrics or studies to demonstrate the effectiveness of Gemini in technology categorization?
Yes, William. Google evaluates Gemini through benchmark datasets and internal metrics. They also conduct studies and gather user feedback to understand its performance and make iterative improvements.
Gemini could be a valuable tool in technology categorization, but I'm concerned about its potential misuse. How does Google address this?
Misuse prevention is a priority for Google, Olivia. They have safety mitigations in place and have put mechanisms to address inappropriate behavior. They are also actively seeking feedback from the user community to identify and improve any risks.
Jeremy, could you provide some examples of how Gemini has already been used in technology categorization projects?
Certainly, Eric. Gemini has been used to improve search functions on websites, assist in software documentation, and enhance natural language understanding for technology support systems. These are just a few examples of its applications.
I assume Gemini requires significant computing power and resources. Is it accessible to small businesses or individual developers?
Initially, Gemini required substantial resources, Jennifer. But Google has made progress in reducing costs and making it more accessible. They offer both free and paid plans to cater to a wide range of users.
This article has convinced me of the potential of Gemini in technology categorization. I'm excited to explore its capabilities further!
That's great to hear, Ryan! I hope you find valuable applications for Gemini in your technology categorization projects.
The advancements in conversational AI with Gemini are fascinating. I wonder how it compares to other AI models in the industry?
Indeed, Paul. Gemini has made significant strides, but there are other impressive models in the industry as well. Gemini focuses on being a useful tool for developers, leveraging its conversational AI capabilities for technology categorization.
Would Gemini be able to filter and categorize technology-related articles or blog posts effectively?
Absolutely, Michelle. Gemini can assist in filtering and categorizing technology-related articles or blog posts based on their content. Its conversational abilities make it adaptable to specific requirements.
Gemini sounds impressive, but can it handle real-time interactions and provide instant responses?
Good point, Sophia. Gemini's response time depends on the context and complexity of the query. While it can handle near-real-time interactions, instant responses might not always be possible.
Jeremy, what are your thoughts on the future developments and improvements of Gemini?
Great question, Mark. Google is actively working on refining Gemini based on user feedback, expanding its capabilities to more languages, and further reducing biases. The future looks promising with continuous improvements.
Can Gemini be integrated into existing technology support chatbots or customer service systems?
Definitely, Amy. Gemini can be integrated with existing technology support chatbots or customer service systems to enhance their capabilities. It can provide more accurate and human-like responses to user queries.
Are there any limitations on the length or complexity of queries that Gemini can effectively handle?
Good question, Christopher. Gemini has some limitations on the length and complexity of queries it can handle effectively. Very long or highly complex queries may not receive optimal responses, as it works best within certain bounds.
I'm impressed by Gemini's capabilities, but can it understand and categorize technical diagrams or charts?
While Gemini primarily focuses on understanding and categorizing text-based inputs, Lily, it may face limitations when analyzing technical diagrams or charts. It's more suited for textual data processing tasks.
How can developers provide feedback to Google about Gemini's performance and suggest improvements?
Developers can provide feedback directly to Google, Jacob. Google encourages user feedback, especially regarding problematic model outputs or potential biases, to iteratively improve the system.
Jeremy, what are the factors that make Gemini a reliable technology categorization tool?
Great question, Emma. Gemini's reliability is built upon training it on diverse data sources and continuously fine-tuning it with human reviewers. It also integrates user feedback to address any shortcomings or errors.
Are there any privacy concerns associated with using Gemini in technology categorization tasks?
Google takes privacy seriously, Andrew. They retain user API data for 30 days but do not use it to improve their models. Google keeps privacy and data security in mind to ensure user confidentiality.
Jeremy, what are some potential applications of Gemini in technology categorization beyond software and websites?
Great question, Ella. Gemini can be useful in diverse technology categorization domains such as hardware documentation, technology-related research, content moderation, and more. Its applications can extend beyond traditional software and websites.
After reading your article, Jeremy, I'm excited to explore how Gemini can enhance technology categorization in my organization. Thanks for sharing!
Thank you all for reading my article! I'm excited to discuss Gemini and its potential in technology categorization. Feel free to share your thoughts and ask questions!
Great article, Jeremy! Gemini seems like a game-changer in technology categorization. I can imagine it being a valuable tool for companies dealing with large amounts of data. Can you provide more insights on how Gemini specifically handles categorization?
Thanks, Michael! Gemini uses conversational AI to improve technology categorization. It can engage in a dialogue with users, ask clarifying questions, and provide real-time categorization suggestions based on user-provided information. Its ability to understand context and ask follow-up questions sets it apart from traditional categorization methods.
I'm impressed with the potential of Gemini! In my experience, categorization can be time-consuming, especially with rapidly changing technology. Have there been any limitations or challenges identified in using Gemini for technology categorization?
Great question, Emma! While Gemini offers promising results, there are some limitations. It may occasionally provide incorrect categorization suggestions, especially when dealing with complex or ambiguous topics. The model may also require additional training to perform optimally in specific domains. Continuous improvement and feedback are critical to refine and enhance its categorization capabilities.
I can see how Gemini would be useful, but how can we ensure its suggestions align with the desired categorization? Can it be trained specifically for different industries or domains?
Excellent point, Sophia! Gemini's suggestions can be influenced through fine-tuning and domain-specific training. By training the model with data relevant to specific industries or domains, we can align its categorization suggestions accordingly. This adaptability makes Gemini a versatile tool that can cater to different needs.
I believe Gemini has immense potential in technology categorization, but what about privacy concerns? How does the model handle user data and ensure the protection of sensitive information?
Valid concern, Robert! Google takes user privacy seriously. The data used to train the model is processed keeping strict privacy and security protocols in place. However, it's important to remain cautious and be mindful when using any AI-powered service. Google is committed to addressing privacy concerns and adhering to best practices.
I'm curious about the accuracy and reliability of Gemini. Are there any metrics or benchmarks that demonstrate its performance in technology categorization?
Good question, Amy! Gemini's performance can be evaluated through metrics such as precision, recall, and F1 score. However, due to the nature of categorization being subjective, it's challenging to establish a single benchmark. The effectiveness of the model can vary based on the application and individual requirements, so it's important to assess its performance within specific contexts.
I wonder if Gemini has any practical implementations currently? Are there any companies or organizations already utilizing this technology for technology categorization?
Great question, Daniel! Gemini is a relatively new technology, but there are companies already exploring its applications. Google is working with various partners to develop real-world use cases, and some organizations have started incorporating Gemini for technology categorization tasks. It's an exciting time with potential for further advancements!
Jeremy, I appreciate your insights on Gemini. As an AI enthusiast, I'm thrilled to see advancements in conversational AI. How do you envision Gemini evolving in the future?
Thank you, Michelle! In the future, I believe Gemini will continue to evolve and become more accurate, reliable, and versatile. Enhanced training techniques, increased domain-specific fine-tuning, and valuable user feedback will be vital in improving its capabilities. We can anticipate its integration into a broader range of applications, ultimately benefiting various industries.
This article sparked my interest! I see potential for Gemini not only in technology categorization but also in customer support automation. Imagine having a capable AI agent engaging with customers in real-time. What are your thoughts on this, Jeremy?
You've hit the nail on the head, David! Gemini's conversational abilities make it ideal for customer support automation. By understanding and responding to customer queries, it can enhance the support experience. While it might not replace human agents entirely, it can assist them in handling common inquiries and delivering prompt assistance.
I'm curious about the pre-training process of Gemini. How is the model trained to understand and generate human-like responses?
Good question, Olivia! Gemini undergoes a two-step training process. Initially, it is pre-trained using a vast amount of publicly available text from the internet, learning grammar, facts, and some level of reasoning. Then, it goes through fine-tuning using custom datasets created by Google. This iterative process helps the model develop a more human-like conversational ability while being informative.
What steps does Google take to prevent biases in Gemini's responses? Bias in AI models has been a concern lately.
Indeed, Liam! Google is actively working to reduce biases in Gemini's responses. During the fine-tuning process, the model is trained with guidelines that emphasize not favoring any political group, and efforts are made to mitigate biased behavior. Google encourages user feedback to identify and rectify any biases, ensuring fairness and inclusivity in the system's responses.
I'm amazed by the potential of Gemini. Are there any plans to release an API to enable developers to integrate Gemini into their own applications?
Absolutely, Ethan! Google has plans to release a Gemini API, allowing developers to build applications and services with it. This will provide more accessibility and unleash the creative potential of developers in utilizing Gemini's capabilities. Stay tuned for updates on Google's roadmap!
Gemini's conversational abilities are impressive, but have there been any instances where the generated responses were inappropriate or misleading?
Good question, Sophie! Gemini's responses can sometimes be inaccurate or inappropriate, although Google has made efforts to reduce such occurrences. User feedback is critical in identifying and rectifying any issues. Google has implemented a Moderation API to warn or block certain types of unsafe content. Responsible usage and continuous improvement are key elements in making Gemini safer and more reliable.
I'm curious about the scalability aspect. Can Gemini handle large volumes of concurrent conversations effectively, especially in scenarios like customer support?
Scalability is an important consideration, Jason! Gemini can handle multiple conversations at once, but there are practical limits to the number it can handle effectively. When deployed, adequate resource allocation and management are crucial to ensure optimal performance. Google is actively working on enhancing scalability and exploring ways to make Gemini more efficient in handling concurrent conversations.
Jeremy, what are some potential challenges users might face when adopting Gemini for technology categorization, and how can they overcome them?
Great question, Lucy! One challenge is that Gemini's suggestions might not always align perfectly with users' expectations due to the subjective nature of categorization. Users can overcome this by providing clear guidelines and indicating their desired criteria for categorization. Collaboration with Gemini and iteratively refining its suggestions based on user feedback will help improve its categorization effectiveness.
Does Gemini take into account the historical context of a conversation when providing categorization suggestions? For example, if a user suggests different categories for two similar queries, will the model remember the previous conversation and adjust its suggestions accordingly?
Yes, Sophia! Gemini maintains a sense of context within conversations. It can remember and refer to prior queries and replies within a conversation, which allows it to adjust its suggestions accordingly. This contextual understanding strengthens its ability to provide more coherent and accurate categorization suggestions based on the ongoing conversation.
Are there any plans to make Gemini more accessible for non-technical users who might also benefit from its technology categorization capabilities?
Absolutely, Oliver! Google aims to make Gemini more user-friendly and accessible beyond technical experts. Usability improvements, intuitive interfaces, and simplifying the interaction process are part of the roadmap. By lowering the barriers to entry, non-technical users will be able to leverage Gemini's technology categorization features and apply them effectively in their respective domains.
I find Gemini's conversational AI fascinating! Can the model understand queries with typos or poorly structured sentences? Does it offer suggestions to improve the clarity of user input?
Good question, Chloe! While Gemini can sometimes handle queries with typos or poorly structured sentences, its performance may vary depending on the input quality. It can offer suggestions to improve clarity in some cases, but it's important to note that Gemini is not a grammar or spell-checking tool. Providing clear and well-structured input helps enhance the accuracy of its responses.
How does Gemini maintain coherence and consistency within a conversation, especially when users can change topics or introduce unrelated queries?
Maintaining coherence is a challenge, Noah, when conversations shift or introduce unrelated queries. Gemini may not handle topic changes gracefully in all cases, and its responses can sometimes veer off-topic. By providing clear context and guiding the conversation, users can help mitigate this issue. Google is actively researching and investing in techniques to improve the model's ability to stay coherent even in such situations.
I'm curious about the computational requirements to deploy Gemini. Does it place significant demands on hardware resources, or can it run on relatively modest setups?
Ava, deploying Gemini requires sufficient hardware resources, particularly during high-demand situations like multiple concurrent conversations or large-scale deployments. While it can run on relatively modest setups, optimal performance might require more substantial computing resources. Google is actively researching ways to improve efficiency and resource utilization, aiming to make deployment more accessible and cost-effective.
Jeremy, how does Gemini handle user queries that include confidential or sensitive information to ensure data privacy?
Data privacy is crucial, Emily! Gemini's developers at Google have taken measures to minimize the risk of exposing confidential or sensitive information. Since Google aims to respect user privacy, it's essential for users to be cautious and avoid sharing highly sensitive data during interactions. By following best practices and being mindful of the information exchanged, users can maintain a high level of data privacy.
Would it be possible to make Gemini's underlying code open-source, enabling independent audits, and ensuring transparency?
Open-sourcing Gemini's code is an interesting suggestion, Sophia! While currently, the underlying code is not open-source, Google is actively considering different paths to increase transparency and enable audits. Striking a balance between transparency, user privacy, and mitigating potential misuse is a complex challenge, but Google values the input and concerns of the wider community.
Do you think Gemini has the potential to surpass human performance in technology categorization?
William, while Gemini shows promising capabilities, surpassing human performance in technology categorization is a challenging goal. Human expertise and intuition still play a crucial role in subjective decision-making and context-specific categorization. However, with ongoing advancements and wider adoption, Gemini can complement human efforts, augment productivity, and provide valuable insights in technology categorization.
Great article, Jeremy! I wonder how Gemini's training process addresses potential biases in the data it learns from?
Thank you, Alexa! During the pre-training phase, Gemini learns from a wide range of publicly available text on the internet. This diverse data helps mitigate specific biases. However, biases can still arise from the data's collective nature. Google is investing in research and engineering to address biases and improve the fairness of Gemini's responses, ensuring a more balanced and inclusive system.
Do you think Gemini's conversational AI capabilities have the potential to significantly impact other fields apart from technology categorization?
Absolutely, Isabella! Gemini's conversational AI capabilities hold promise beyond technology categorization. Applications like customer support, content generation, personal assistants, and more can benefit from its abilities. As the technology evolves and more use cases are explored, the impact of Gemini's conversational AI can extend to various domains, transforming the way we interact with AI-driven systems.
Thank you all for engaging in this discussion! Your questions and insights have been valuable. I hope this insight into Gemini's potential in technology categorization sparks further exploration and excitement. Feel free to continue the conversation or reach out with any additional queries. Have a great day!