GPT-Enabled Molecular Modeling: Revolutionizing Technology in the Lab
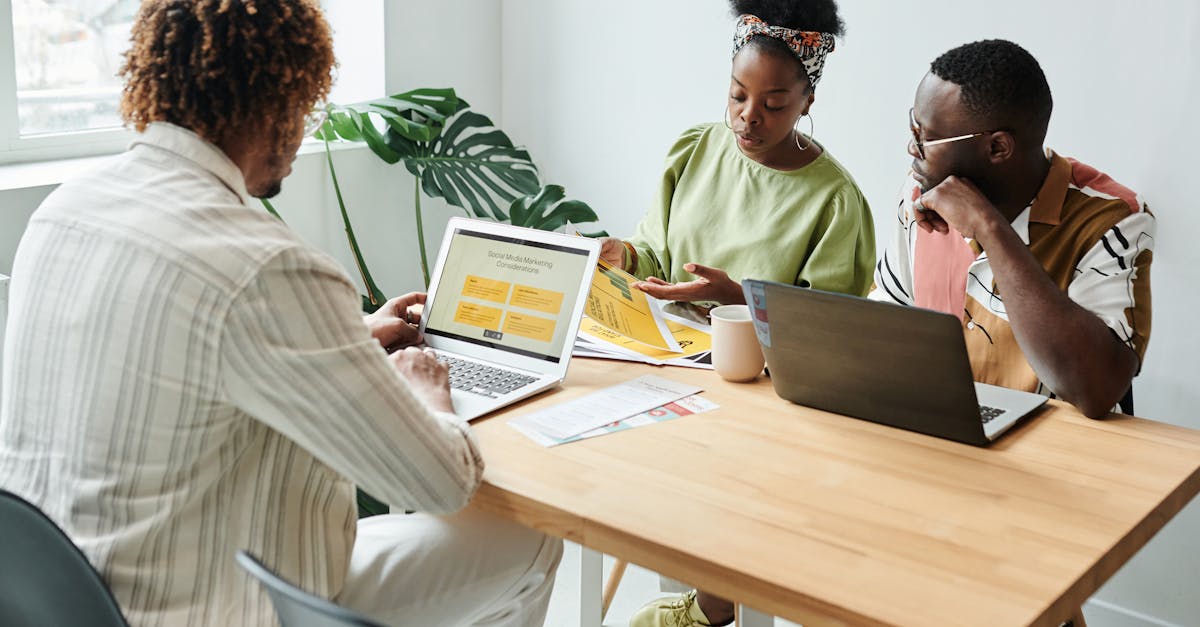
Understanding the structure of proteins is key in many fields of biology, from the designing of drugs to the development of sustainable crops. A myriad of techniques has been devised to predict the structure of proteins, offering approximate, but often useful, information to researchers worldwide. One such technique that has gained considerable traction is molecular modeling. This article focuses on the use of molecular modeling in protein structure prediction and how future developments in AI technology, like ChatGPT-4, are poised to contribute to its progress.
Part I: The Power of Molecular Modeling
Molecular modeling depends on the principles of computational chemistry, mathematics, and physics to analyze and predict the structural, dynamic, and thermodynamic properties of molecules, particularly biomolecules like proteins. These predictions have important implications for understanding the function of these molecules and their potential behavior in biological systems. With the advent of robust computational capabilities, molecular modeling has become an invaluable tool, offering researchers a method to probe the hidden dimensions of biomolecular structure and function.
Part II: Protein Structure Prediction – A Complex Challenge
Predicting protein structures is a problem of extraordinary complexity, primarily due to the astronomically large number of potential configurations that a protein can adopt. Coupled with the fact that the functional state of a protein is intricately tied to its three-dimensional configuration, predicting protein structures is nothing short of decoding the language of life - a complexity that reshapes our understanding of the biological world.
Part III: Molecular Modeling and Protein Structure Prediction
Molecular modeling plays a crucial role in addressing the problem of protein structure prediction. It uses algorithms, fixed rules, and principles of physics and chemistry to accurately predict protein folding. With the help of molecular modeling techniques, researchers can predict the way in which proteins fold by identifying the lowest energy state in a given set of confounding variables. This is fundamental to understanding how they function and interact in the complex theater of biological activity.
Part IV: Harnessing the Power of AI – ChatGPT-4
Modern advancements forthwith ChatGPT-4, a state-of-the-art language-based AI, open up unprecedented opportunities for the field of protein structure prediction through molecular modeling. ChatGPT-4 can be used to predict protein structures by providing possible protein folding structures based on a given amino acid sequence.
At a high level, such accurate predictions will depend on a formidable collection of training data. By training on a variety of protein structures, the AI can 'learn' the complex rules of protein folding, and subsequently make predictions about never-seen-before sequences. This development reflects the core of how machine learning technologies will contribute significantly to protein structure prediction, providing novel insights and guiding researchers towards promising directions.
Conclusion
As we plunge deeper into the era of big data and artificial intelligence, the interplay between computational and experimental methods in protein structure predictions is becoming increasingly important. Molecular modeling, coupled with advanced AI technologies like ChatGPT-4, is armed to make significant strides in the field of protein structure prediction, thereby revolutionizing not just the scientific communities studying these intricate phenomena, but also contributing to major leaps in practical applications.
As we continue to unravel the secrets of the biological world one protein at a time, the aptitude of integrated AI systems such as ChatGPT-4 will inevitably play an instrumental role in decoding the fabric of life, steering us towards a future where new drugs, sustainable crops, and innovative treatments become realities of the day.
Comments:
Thank you all for reading my article on GPT-Enabled Molecular Modeling! I'm excited to hear your thoughts and engage in discussions.
This is a fascinating technology! The possibilities it offers in the lab are immense. Can't wait to see how it develops further.
I agree, Rachel! GPT-Enabled Molecular Modeling has the potential to revolutionize scientific research and accelerate discoveries. It's incredible!
I'm curious about the accuracy of GPT-Enabled Molecular Modeling. While it sounds promising, how reliable is it compared to traditional methods?
That's an excellent question, Amy. GPT-Enabled Molecular Modeling has shown promising results so far, but it's important to validate its accuracy against traditional methods before widespread adoption.
I believe GPT-Enabled Molecular Modeling can revolutionize drug discovery. The potential to generate novel candidate molecules efficiently and cost-effectively is game-changing!
Absolutely, Michael! The ability to generate diverse molecule designs using GPT models can significantly speed up the drug discovery process and lead to breakthroughs in treatments.
This technology also raises ethical concerns. With GPT generating possible designs, what are the risks of unintended consequences or unethical applications?
You raise a valid point, Sara. Like any technology, GPT-Enabled Molecular Modeling requires careful consideration and ethical guidelines to ensure responsible use. As the field progresses, it's essential to address these concerns.
I'm impressed by the potential of GPT-Enabled Molecular Modeling, but I wonder how accessible it is to researchers. Are there any limitations or specific skills needed to utilize this technology?
Great question, Emily! GPT-Enabled Molecular Modeling is still in its early stages, and like any advanced technology, it requires specialized expertise. Collaborations between AI experts and domain scientists can bridge the gap and make it more accessible to researchers.
I'm curious about the computational resources needed for GPT-Enabled Molecular Modeling. Are there any significant infrastructure requirements to leverage its capabilities?
Good point, Daniel. GPT models require substantial computational power and large-scale data processing. Researchers would benefit from access to high-performance computing resources and efficient algorithms for better implementation.
The integration of GPT-Enabled Molecular Modeling with experimental data sounds promising. How can we leverage both approaches effectively to drive scientific progress?
Indeed, Isabella! Integrating GPT-Enabled Molecular Modeling with experimental data can enhance the accuracy of predictions and guide further experiments. This symbiotic relationship between models and real-world data holds tremendous potential for scientific advancement.
I'm concerned about the potential bias in GPT models and how it could impact molecular modeling. How can we ensure fairness and minimize any inherent biases?
Valid concern, John. Addressing biases in AI models, including GPT, is crucial. A rigorous development process, diverse training data, and ongoing evaluation are necessary to minimize biases and ensure fairness and reliability in molecular modeling.
Apart from drug discovery, what other areas could benefit from GPT-Enabled Molecular Modeling? Are there any exciting applications on the horizon?
Great question, Olivia! GPT-Enabled Molecular Modeling has broader applications, including materials science, protein engineering, and renewable energy research. Its versatility opens doors to numerous exciting possibilities!
To what extent can GPT-Enabled Molecular Modeling replace traditional experimentation? Will it completely replace hands-on lab work or only complement it?
An important consideration, Sophia. While GPT-Enabled Molecular Modeling can greatly enhance the efficiency and accuracy of research, it's unlikely to completely replace hands-on lab work. Instead, it will complement experiments and serve as a valuable tool for better understanding and optimization.
I'm fascinated by the potential of GPT-Enabled Molecular Modeling, but what challenges lie ahead in terms of scalability and managing increasingly large datasets?
Good question, Andrew. Scalability and data management are indeed challenges. Developing efficient algorithms, utilizing distributed computing, and leveraging advancements in cloud infrastructure can help overcome these obstacles and ensure smooth operation for large-scale datasets.
As an AI enthusiast, I'm curious about the limitations and potential biases of GPT-Enabled Molecular Modeling. How can we transparently address these concerns?
Transparency is key, Ella. Sharing model architectures, training data sources, and evaluation methodologies can help identify limitations and biases. Collaborative efforts within the scientific community to openly address these concerns are essential for the responsible development and adoption of GPT-Enabled Molecular Modeling.
I'm intrigued by the impact GPT-Enabled Molecular Modeling can have on scientific discovery. However, how can we ensure that researchers remain at the forefront of experimentation while relying on AI-driven models?
A valid concern, Liam. While AI-driven models like GPT-Enabled Molecular Modeling offer valuable insights and accelerate research, it's crucial for researchers to maintain their domain expertise and continuously collaborate with AI experts. This ensures a balanced approach where human creativity and knowledge remain at the forefront of experimentation.
How can we ensure the security and confidentiality of sensitive scientific data when utilizing GPT-Enabled Molecular Modeling, considering potential risks like data breaches?
Excellent question, Emma. The security and confidentiality of data must be a top priority. Implementing robust data protection measures, secure cloud infrastructures, and adhering to established data privacy regulations are key to minimizing risks and ensuring data integrity in GPT-Enabled Molecular Modeling.
I'm excited about GPT-Enabled Molecular Modeling, but how can we foster interdisciplinary collaborations between AI researchers and domain experts to fully harness its potential?
That's a crucial aspect, David. Promoting interdisciplinary collaborations through conferences, workshops, and joint research projects can bridge the gap between AI researchers and domain experts. The exchange of knowledge, insights, and perspectives is invaluable for fully harnessing the potential of GPT-Enabled Molecular Modeling.
I'm curious about the timeline for the adoption of GPT-Enabled Molecular Modeling in various scientific domains. When can we expect to see significant utilization?
It's a dynamic field, Sophie, and adoption timelines can vary based on research domains. Nevertheless, we're already witnessing promising progress. Within the next few years, I believe we'll see significant utilization of GPT-Enabled Molecular Modeling in fields like drug discovery, materials science, and protein engineering.
Considering the complexity of molecular modeling, what are the current limitations of GPT-Enabled Molecular Modeling, and how can we overcome them?
Great question, Ethan. Despite its potential, GPT-Enabled Molecular Modeling is limited by factors such as dataset biases and the interpretability of generated results. Overcoming these challenges requires further research, improved training methodologies, and tool development to enhance transparency and model reliability.
Do you think GPT-Enabled Molecular Modeling will lead to a shift in the skills required for researchers in the future?
Absolutely, Nora. GPT-Enabled Molecular Modeling introduces a paradigm shift in computational approaches in the lab. Researchers will need a blend of traditional scientific expertise and computational skills to effectively utilize and contribute to this evolving field.
How can we ensure that the learning capacity and generalization of GPT models in molecular modeling is reliable across different chemical contexts?
Good question, Alex. Generalization across different chemical contexts is a significant challenge. Diverse training datasets, careful validation, and ongoing fine-tuning of GPT models specific to various chemical domains can help improve reliability and ensure consistent performance.
I'm concerned about the potential biases in training data and how they can affect model predictions. How can we address and mitigate these biases effectively?
Addressing biases is critical, Sophia. To mitigate biases in training data, we need a comprehensive data collection process that ensures representation and diversity. Furthermore, ongoing monitoring and evaluation of model predictions can help identify and mitigate any biases that may arise.
GPT-Enabled Molecular Modeling sounds impressive, but what steps are being taken to ensure that this technology is embraced by the scientific community?
Valid concern, Gabriel. To foster acceptance, the scientific community must actively engage with GPT-Enabled Molecular Modeling through collaborations, shared resources, and open discussions. Peer-reviewed publications, conferences, and expert-led workshops play a crucial role in building trust and promoting wider adoption of this technology.
How can we ensure that GPT-Enabled Molecular Modeling is used responsibly and ethically, given the significant influence AI models can have on critical scientific decisions?
Responsibility and ethics are paramount, Ava. Establishing guidelines, ethical frameworks, and regulatory oversight specific to GPT-Enabled Molecular Modeling is essential. Encouraging transparent reporting, peer review, and ongoing evaluation can help ensure responsible use and prevent misuse of this powerful technology.
Are there any current real-world examples where GPT-Enabled Molecular Modeling has already demonstrated significant advancements or breakthroughs?
Indeed, Benjamin. GPT-Enabled Molecular Modeling has showcased promising advancements in areas like drug discovery, where it has helped in generating novel molecules with desired properties. Researchers have also leveraged the technology in protein structure prediction and optimization of material properties.
I'm concerned about the potential bias in training models based on existing scientific knowledge. How can we prevent reinforcing biases and ensure models explore and learn beyond the known data?
Great point, Grace. To prevent reinforcing biases, we must strike a balance between relying on existing scientific knowledge and encouraging exploration of uncharted territories. Curating diverse datasets, utilizing unsupervised learning methods, and providing appropriate incentives for model exploration can help mitigate the risk of reinforcing biases.
How can GPT-Enabled Molecular Modeling contribute to the development of personalized medicine and tailored treatments?
Personalized medicine is an exciting area where GPT-Enabled Molecular Modeling can make significant contributions, Aaron. By leveraging patient-specific data and combining it with molecular modeling, we can identify personalized treatment options and optimize interventions for better patient outcomes.
I'm impressed by the potential of GPT-Enabled Molecular Modeling, but how can we ensure that AI-driven models do not replace human creativity and intuition in scientific discovery?
You bring up an important point, Aria. AI-driven models like GPT-Enabled Molecular Modeling are tools that augment human capabilities. Encouraging collaborations, interdisciplinary approaches, and maintaining the human element in scientific discovery are vital to ensure a balanced and fruitful exploration of knowledge.
What are the current limitations of GPT-Enabled Molecular Modeling when it comes to complex systems like biological pathways or interactions between molecules?
Excellent question, Ethan. GPT-Enabled Molecular Modeling still faces challenges in accurately representing complex systems like biological pathways. As the technology evolves, integrating domain-specific knowledge, expanding training datasets, and incorporating multi-scale modeling approaches are some of the ways to address these limitations and improve model performance.
Can GPT-Enabled Molecular Modeling help in reducing the cost and time associated with drug discovery and development?
Absolutely, Sophia. GPT-Enabled Molecular Modeling has the potential to significantly shorten the drug discovery and development timeline by enabling rapid screening of vast chemical spaces. This efficiency can help reduce costs associated with traditional trial-and-error approaches and accelerate the availability of life-saving medications.
I'm curious about the application of GPT-Enabled Molecular Modeling in fields like nanotechnology and material science. Can it aid in the design of advanced materials?
Indeed, Jacob! GPT-Enabled Molecular Modeling has immense potential in nanotechnology and material science. By accurately predicting material properties and exploring novel compositions, it can help in designing advanced materials with desired characteristics, leading to breakthroughs in various industries.
How can GPT-Enabled Molecular Modeling assist in tackling grand challenges like climate change or renewable energy?
Great question, Emily! GPT-Enabled Molecular Modeling can contribute to addressing grand challenges like climate change by aiding in the discovery and optimization of renewable energy materials, catalysts, and more. Enhancing energy efficiency and sustainability through molecular-level design is an exciting prospect.
Will GPT-Enabled Molecular Modeling require significant computational resources, and how can researchers access those resources?
Good point, Zoe. GPT-Enabled Molecular Modeling does require substantial computational resources for training and inference. Accessing high-performance computing infrastructure, cloud platforms, and collaborative initiatives can provide researchers with the necessary resources to leverage this technology effectively.
What kind of industry collaborations can help drive the development and adoption of GPT-Enabled Molecular Modeling?
Industry collaborations play a significant role in advancing GPT-Enabled Molecular Modeling, Mason. Partnerships between academia, pharmaceutical companies, and technology firms can facilitate the development of robust models, validate real-world applications, and drive the adoption of this technology in various sectors.
Can GPT-Enabled Molecular Modeling aid in predicting the toxicity of chemical compounds, helping in the early stages of drug development?
Absolutely, Lucy! GPT-Enabled Molecular Modeling can contribute to predicting the toxicity of chemical compounds, enabling early identification of potentially harmful substances in drug development. This capability can significantly streamline the research process and improve patient safety.
How can we foster open-source collaboration and data-sharing in the field of GPT-Enabled Molecular Modeling?
Open-source collaboration and data-sharing are vital, Noah. Establishing platforms, repositories, and standards for sharing models, datasets, and evaluation metrics can promote transparency, reproducibility, and collaboration in GPT-Enabled Molecular Modeling, fostering advancements across the scientific community.
I'm concerned about potential biases or errors in the generated results by GPT models. How can scientists verify the accuracy and reliability of the predictions?
Verification is crucial, Eleanor. Scientists can employ techniques like cross-validation, benchmarking against known experimental data, and carrying out additional empirical tests to validate the accuracy and reliability of GPT models in molecular modeling. Rigorous evaluation ensures confidence in the predictions generated by these models.
How can GPT-Enabled Molecular Modeling be integrated into current research workflows without disrupting existing processes?
Integration is a key consideration, William. GPT-Enabled Molecular Modeling can be introduced gradually, starting with complementary tasks in existing research workflows. By providing seamless interfaces, interoperability, and user-friendly tools, we can ensure a smooth integration that enhances productivity without disrupting established processes.
Is GPT-Enabled Molecular Modeling solely limited to academia and industry, or can citizen scientists also contribute to this field?
Citizen scientists can absolutely contribute, Oscar. Open collaboration, public datasets, and user-friendly interfaces can empower citizen scientists to make meaningful contributions to GPT-Enabled Molecular Modeling. Encouraging and supporting their participation can enhance diversity and broaden the impact of this technology.
How can GPT-Enabled Molecular Modeling aid in understanding complex genetic disorders and facilitate the development of targeted therapies?
GPT-Enabled Molecular Modeling can play a significant role, Evelyn. By analyzing complex genetic data, identifying disease-causing molecular mechanisms, and recommending potential therapeutic interventions, it can contribute to a better understanding of genetic disorders and facilitate the development of targeted and personalized treatments.
I'm interested in the potential ethical dilemmas that can arise from AI-generated molecular designs. How can we navigate the responsible and ethical use of this technology?
Addressing ethical dilemmas is essential, Lucas. Establishing interdisciplinary frameworks, involving ethical experts, and encouraging open dialogue can help navigate the responsible and ethical use of AI-generated molecular designs. Striking a balance between exploration and careful consideration of potential consequences is vital.
What impact can GPT-Enabled Molecular Modeling have on reducing the number of experimental failures and improving research outcomes?
GPT-Enabled Molecular Modeling has the potential to significantly reduce experimental failures and improve research outcomes, Harper. By utilizing predictive models, researchers can prioritize experiments, optimize conditions, and focus on candidates with higher likelihoods of success, thereby making research more efficient and productive.
Can GPT-Enabled Molecular Modeling help in the discovery of new materials with unparalleled properties, opening up possibilities for numerous applications?
Absolutely, Charlotte! GPT-Enabled Molecular Modeling's ability to explore vast chemical spaces can aid in the discovery of new materials with unparalleled properties. This opens up possibilities for applications in fields like electronics, energy storage, and catalysis, revolutionizing various industries.
How can GPT-Enabled Molecular Modeling enhance collaboration among scientists, both within and across disciplines?
GPT-Enabled Molecular Modeling can foster collaboration, Alice. By providing a common computational framework and shared understanding, it enables scientists from different disciplines to work together smoothly. This interdisciplinary collaboration leads to new insights, innovative solutions, and advancements at the intersection of various fields.
I'm intrigued by the potential speed at which GPT-Enabled Molecular Modeling can generate predictions. How can researchers manage the massive amounts of data generated by this technology?
Managing the data is a significant task, Harley. Researchers must develop efficient data storage, retrieval, and analysis pipelines to handle the massive amounts of data generated by GPT-Enabled Molecular Modeling. Adopting scalable computational infrastructures, cloud-based solutions, and robust data management practices can aid in effectively managing this aspect.
What kind of research incentives can encourage scientists to adopt and contribute to the field of GPT-Enabled Molecular Modeling?
Offering research incentives can drive adoption and contributions, Clara. Funding opportunities, establishment of dedicated research centers, recognition through awards and grants, and the availability of high-quality datasets and tools can spark interest, motivate scientists, and encourage their active engagement in the field of GPT-Enabled Molecular Modeling.
What steps can be taken to educate the next generation of scientists and researchers about GPT-Enabled Molecular Modeling and its potential applications?
Education is crucial, Landon. Including relevant content in scientific curriculum, organizing workshops, and developing online resources can equip the next generation of scientists and researchers with knowledge and skills in GPT-Enabled Molecular Modeling. Collaboration between academia and industry can bridge the gap and ensure practical exposure to this technology.
Can GPT-Enabled Molecular Modeling assist in predicting the properties and behavior of complex macromolecules, such as RNA or proteins?
Absolutely, Maxwell! GPT-Enabled Molecular Modeling has the potential to aid in predicting properties and behavior of complex macromolecules like RNA or proteins. By utilizing deep learning models, it can analyze large-scale data and provide valuable insights into macromolecular structures, dynamics, and interactions.
How can GPT-Enabled Molecular Modeling contribute to the design and optimization of enzymes for biotechnological applications?
GPT-Enabled Molecular Modeling can make valuable contributions, Liam. By simulating enzyme structures, predicting catalytic properties, and exploring sequence-space, it can assist in designing and optimizing enzymes for biotechnological applications. This can lead to the development of novel biocatalysts and advancement in enzymatic processes.
How can GPT-Enabled Molecular Modeling aid in understanding and designing protein-protein interactions for drug discovery?
Understanding protein-protein interactions is critical, Grace. GPT-Enabled Molecular Modeling can provide insights into the structures, dynamics, and binding affinities of protein complexes. By predicting and optimizing protein-protein interactions, it can aid in designing more effective drugs and therapeutic interventions.
What role can GPT-Enabled Molecular Modeling play in the field of computational drug repurposing and finding new uses for existing drugs?
GPT-Enabled Molecular Modeling is poised to play a significant role, Henry. By analyzing molecular interactions, similarity patterns, and repurposing databases, it can assist in computational drug repurposing, identifying new uses for existing drugs, and accelerating the discovery of potential treatments for various diseases.
Thank you, Jed Bell, for shedding light on the exciting possibilities of GPT-Enabled Molecular Modeling. Your article provided valuable insights into this emerging technology.
Thank you all for reading my article on GPT-Enabled Molecular Modeling! I'm excited to hear your thoughts and answer any questions you may have.
Great article, Jed! Molecular modeling has come a long way, and it's fascinating to see how GPT enables advancements in this field. Can you elaborate on the specific applications where GPT-Enabled Molecular Modeling has shown promise?
Thanks, Sarah! GPT-Enabled Molecular Modeling has shown promise in various areas such as drug discovery, material design, and protein folding. The ability to generate realistic molecular structures and simulate their behaviors has tremendous potential for accelerating research and development.
I have some concerns regarding the reliability of GPT in molecular modeling. How accurate are the predictions generated by this technology?
Valid concern, Michael. GPT-Enabled Molecular Modeling is still an evolving field, and while it has shown promising results, there are challenges in achieving absolute accuracy. However, continuous improvements are being made, and GPT can be utilized as a valuable tool for hypothesis generation and exploration.
I find GPT-Enabled Molecular Modeling truly revolutionary! Its potential to expedite scientific discoveries is remarkable. Jed, do you see any limitations or potential ethical implications we should be aware of?
Excellent question, Oliver! While the technology is exciting, there are a few limitations and ethical considerations. Trusting AI-generated outputs without proper validation, potential biases in the training data, and ensuring responsible use of the technology are important areas to address as GPT-Enabled Molecular Modeling progresses.
I'm impressed by the possibilities of GPT-Enabled Molecular Modeling, but can it fully replace traditional experimental methods?
Great question, Sophia! While GPT-Enabled Molecular Modeling can augment experimental methods, it is unlikely to fully replace them. The technology serves as a powerful tool for hypothesis generation and simulation, but experimental validation is crucial for real-world applications and to ensure accuracy.
I'm curious about the computational requirements for GPT-Enabled Molecular Modeling. Are there any specific hardware or software prerequisites?
Good question, Emily! GPT-Enabled Molecular Modeling does require substantial computational resources. High-performance computing clusters or cloud-based solutions with GPUs are often used to handle the complex calculations involved. Additionally, software frameworks like TensorFlow or PyTorch are commonly employed for implementing the models.
Molecular modeling has immense potential for personalized medicine. Do you see GPT-Enabled Molecular Modeling playing a significant role in this domain?
Absolutely, Maxwell! GPT-Enabled Molecular Modeling can contribute to personalized medicine by aiding in the prediction of drug responses based on individual genetic profiles. This technology has the potential to revolutionize treatment strategies and improve patient outcomes.
Does the accuracy of GPT-Enabled Molecular Modeling depend on the size and quality of the training dataset?
Good point, Liam. The accuracy of GPT-Enabled Molecular Modeling does depend on the size and quality of the training dataset. Adequate diverse data is crucial to ensure the model's ability to generalize and make reliable predictions across different scenarios.
I'm fascinated by the potential applications of GPT-Enabled Molecular Modeling in tackling environmental challenges. Jed, could you provide some examples in this area?
Certainly, Natalie! GPT-Enabled Molecular Modeling can support environmental challenges by predicting chemical reactions, understanding pollutant behavior, and designing catalysts for sustainable technologies. It can aid in the development of eco-friendly solutions by optimizing material properties and reducing the environmental impact of various processes.
I'm curious about the underlying principles of GPT-Enabled Molecular Modeling. How does it actually work?
Great question, Chloe! GPT-Enabled Molecular Modeling leverages deep learning models, such as transformers, trained on large datasets of molecular structures and properties. These models learn patterns and correlations within the data and can generate new molecules or predict properties based on the learned knowledge. It's a fascinating fusion of AI and chemistry!
As GPT-Enabled Molecular Modeling evolves, what are the key areas of research and development for further improvements?
Good question, Sophie! Key areas of research and development for GPT-Enabled Molecular Modeling include enhancing the accuracy and reliability of generated predictions, addressing potential biases in training data, improving interpretability of the models, and integration with experimental workflows for seamless collaboration between AI and researchers.
Could you shed some light on the computational cost of training GPT-Enabled Molecular Modeling models? Is it an expensive process?
Certainly, Daniel! Training GPT-Enabled Molecular Modeling models can indeed be computationally expensive. The process involves large-scale training on high-performance hardware, which can require significant computational resources, time, and energy. However, with advancements in hardware and optimization techniques, the efficiency of training is improving.
Are there any regulatory or safety considerations associated with the use of GPT-Enabled Molecular Modeling?
Excellent question, Lily! The use of GPT-Enabled Molecular Modeling may introduce regulatory and safety considerations. Proper validation and verification processes, transparent reporting of limitations, and adhering to ethical guidelines are crucial to ensure the responsible and safe deployment of this technology in real-world applications.
How accessible is GPT-Enabled Molecular Modeling to researchers who may not have access to high-performance computing resources?
Valid concern, Emma! GPT-Enabled Molecular Modeling can be resource-intensive; however, cloud computing platforms offer an accessible solution. Researchers can leverage cloud-based services, which provide the necessary computational power and infrastructure on-demand, allowing even those without high-performance computing resources to utilize this technology.
What are some of the key challenges that researchers and developers face when working with GPT-Enabled Molecular Modeling?
Great question, William! Key challenges when working with GPT-Enabled Molecular Modeling include handling data quality and bias, interpretability of the model's decision-making process, optimizing computational performance, addressing domain-specific limitations, and ensuring ethical deployment of the technology. These challenges require collaborative efforts from researchers, developers, and the scientific community.
Are there any ongoing research projects or initiatives focused on advancing GPT-Enabled Molecular Modeling?
Absolutely, Olivia! There are several ongoing research projects and initiatives aiming to advance GPT-Enabled Molecular Modeling. Academic institutions, research organizations, and industry collaborations are actively working on improving model architectures, developing better training datasets, and exploring novel applications of this technology across various scientific domains.
How does GPT-Enabled Molecular Modeling handle uncertainty or variations in experimental data?
Good question, Lucas! GPT-Enabled Molecular Modeling can handle uncertainty and variations in experimental data by incorporating probabilistic frameworks. These frameworks enable the modeling of uncertainties and provide a range of possible predictions with associated probabilities, allowing researchers to assess the reliability of the generated results.
What kind of impact can GPT-Enabled Molecular Modeling have on the field of materials science?
Great question, Ella! GPT-Enabled Molecular Modeling can have a significant impact on materials science. It can facilitate the discovery and design of new materials with desired properties, leading to advancements in energy storage, electronics, catalysis, and more. This technology enables accelerated material screening and optimization, saving time and resources in the development process.
How can researchers ensure the transparency and interpretability of GPT-Enabled Molecular Modeling results?
Good point, Leo! Ensuring transparency and interpretability is crucial. Researchers can employ techniques such as attention mapping, sensitivity analysis, and interpretable model architectures to gain insights into the decision-making process of GPT-Enabled Molecular Modeling. Transparent reporting and documentation of limitations play an important role in supporting the understanding and trustworthiness of the results.
Are there any collaborations between GPT-Enabled Molecular Modeling researchers and experimentalists, combining their expertise?
Absolutely, Harry! Collaborations between GPT-Enabled Molecular Modeling researchers and experimentalists are vital. Combining their expertise allows for a synergistic approach. Researchers can leverage GPT for hypothesis generation, lead optimization, or preliminary screenings, while experimentalists provide real-world validation, feedback, and domain expertise to ensure the reliability and applicability of the generated results.
I'm interested in the potential impact of GPT-Enabled Molecular Modeling in the field of agriculture. Are there any promising applications in this area?
Definitely, Mia! GPT-Enabled Molecular Modeling can have promising applications in agriculture. It can help in developing more efficient pesticides, predicting crop responses to different environmental factors, optimizing enzyme design for crop improvement, and aiding in the discovery of sustainable agricultural solutions. This technology has the potential to make significant contributions to food production and sustainability.
What are the potential implications of GPT-Enabled Molecular Modeling for the pharmaceutical industry?
Great question, David! GPT-Enabled Molecular Modeling can revolutionize the pharmaceutical industry. It can accelerate the drug discovery and development process by assisting in virtual screening, lead optimization, and predicting drug-target interactions. This technology has the potential to enhance efficiency, reduce costs, and lead to the discovery of innovative therapies for various diseases.
Can GPT-Enabled Molecular Modeling simulate complex protein folding accurately?
Valid question, Emma! GPT-Enabled Molecular Modeling shows promise in simulating protein folding. While achieving absolute accuracy is challenging due to the complexity of protein folding processes, this technology can provide valuable insights and predictions. Continuous advancements in GPT architectures and training strategies can further improve the accuracy and reliability of protein folding simulations.
What are the potential impacts of GPT-Enabled Molecular Modeling on academia and scientific research?
Great question, Noah! GPT-Enabled Molecular Modeling can have significant impacts on academia and scientific research. It can accelerate the pace of discoveries, aid in hypothesis generation, enable exploration of vast chemical space, and foster collaborations between AI and human researchers. This technology has the potential to reshape scientific research methodologies and unlock new possibilities.
What kind of data is required to train GPT-Enabled Molecular Modeling models?
Good question, Grace! Training GPT-Enabled Molecular Modeling models requires large-scale datasets of molecular structures, properties, and their relationships. This can include data from experimental measurements, databases, or simulations. Diversity and representativeness of the training data are crucial to ensure the model's ability to generalize and make accurate predictions.
What are the key factors that can affect the performance of GPT-Enabled Molecular Modeling models?
Good question, Harper! Key factors that can affect the performance of GPT-Enabled Molecular Modeling models include the quality and size of the training dataset, model architecture and complexity, regularization techniques employed during training, and the availability of computational resources for training and inference. Fine-tuning and continuous feedback loops also play a role in optimizing model performance.