Harnessing the Power of ChatGPT: Enhancing Network Prototyping in Network Design Technology
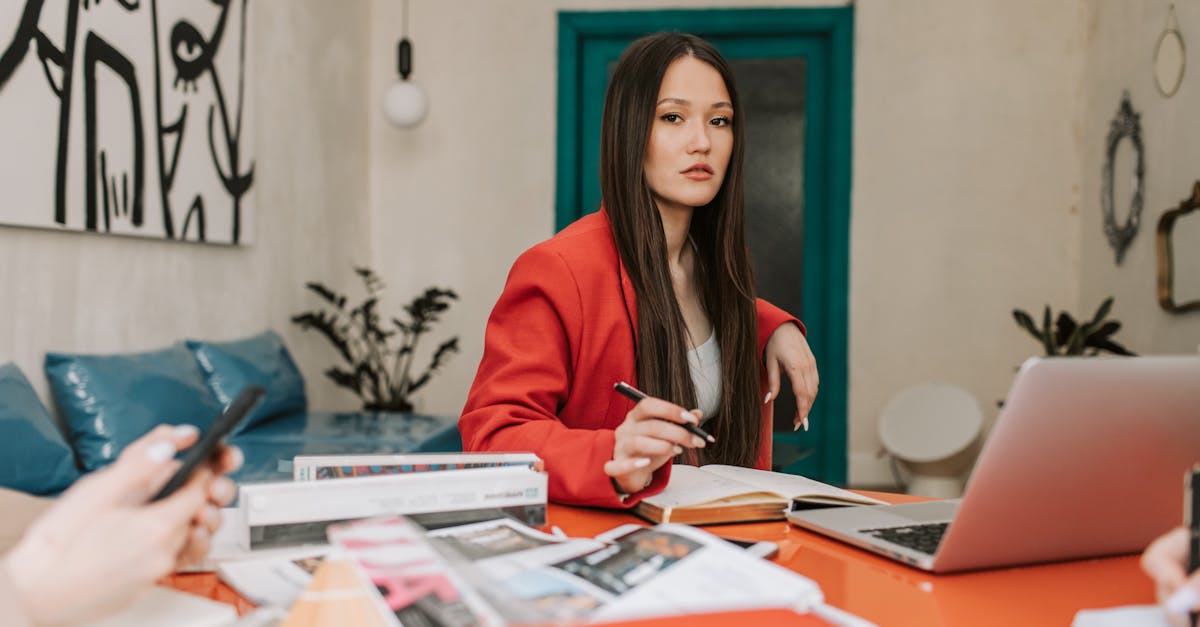
Network design is a critical aspect of building efficient and reliable computer networks. It involves creating a blueprint for the network architecture, including the selection and integration of various hardware and software components. Network prototyping plays a key role in this process, allowing network designers to test and refine their designs before deployment.
With the advancements in artificial intelligence and natural language processing, ChatGPT-4 is a groundbreaking technology that can facilitate the network prototyping process. By leveraging its capabilities, network designers can benefit from the intelligent suggestions and recommendations it offers.
Suggesting Tools
ChatGPT-4 can analyze the requirements and constraints of a network design project and suggest suitable tools for building and testing network prototypes. Whether it's selecting a software-defined networking (SDN) controller, a network simulation tool, or a traffic generator, ChatGPT-4 can provide valuable insights based on its vast knowledge base and understanding of network design principles.
For instance, if a network designer needs to prototype a large-scale data center network, ChatGPT-4 can recommend using tools like Mininet, which is a popular network emulator. Alternatively, it might suggest using GNS3 for simulating complex network topologies. These suggestions can save time and effort by pointing designers in the right direction.
Suggesting Methodologies
Network prototyping involves defining the methodologies and approaches used during the design and testing phases. ChatGPT-4 can provide guidance on selecting appropriate methodologies based on the specific requirements of the network design project.
For example, if the goal is to prototype a wireless mesh network, ChatGPT-4 can suggest using a combination of protocols like IEEE 802.11s and Open Shortest Path First (OSPF) to create a scalable and resilient network structure. By considering the context and objectives of the project, ChatGPT-4 can recommend methodologies that align with industry best practices for network design.
Suggesting Frameworks
Frameworks are essential for organizing and structuring the network prototyping process. They provide a standardized approach and set of tools that can streamline the design and testing phases. ChatGPT-4 can suggest suitable frameworks based on the specific requirements and goals of a network design project.
For instance, if the objective is to prototype a software-defined wide area network (SD-WAN), ChatGPT-4 might recommend using a framework like OpenDaylight or Open vSwitch. These frameworks can simplify the implementation of SDN principles and provide a foundation for building scalable and flexible network prototypes.
Conclusion
ChatGPT-4 offers an innovative solution for network prototyping by providing intelligent suggestions for tools, methodologies, and frameworks. Its ability to analyze project requirements and apply its vast knowledge database can assist network designers in making informed decisions and optimize their prototyping process.
By harnessing the power of artificial intelligence, network designers can leverage ChatGPT-4 to save time, improve efficiency, and ultimately build robust and reliable computer networks.
Comments:
Thank you all for taking the time to read and comment on my article! I'm excited to engage in this discussion and hear your thoughts on harnessing the power of ChatGPT in network design technology.
This article presents an interesting approach to network prototyping. I'm curious to know how ChatGPT can enhance the traditional methods in network design. Can anyone provide more insights on this?
I've had experience with network design, and I can see the potential of using ChatGPT. It could facilitate brainstorming sessions and provide different perspectives that might otherwise be overlooked. However, I wonder if there are any limitations related to its ability to understand complex network configurations?
I agree with Sara Johnson's concern about complex network configurations. AI models might struggle to handle intricate setups where a deep understanding of network protocols is required.
Michael Adams, I agree with you. AI models should be used as supporting tools in network design, not as substitutes for human expertise. Deep knowledge of network protocols will always be valuable in complex configurations.
The concept of using AI to enhance network design is promising. It could potentially speed up the design process and improve efficiency. But what about the security aspect? Should we be concerned about the potential vulnerabilities introduced when relying heavily on AI?
@Emily Ramirez: That's a valid concern. While AI can bring numerous benefits, it's crucial to address security vulnerabilities. Incorporating robust security measures and periodically updating the system can help mitigate potential risks.
@Robyn Barratt: Absolutely, robust security measures and regular updates are essential to mitigate risks. AI should always be used as a tool, while human expertise and domain knowledge remain vital in ensuring network security.
Emily raises a valid concern about the security implications of relying heavily on AI. It's crucial to implement a multi-layered security approach that includes stringent access controls, encryption, and regular vulnerability assessments to protect network designs.
Emily raises an important concern about the security aspects. As AI becomes more prevalent, it's essential to establish measures to safeguard network designs from potential vulnerabilities and unauthorized access.
I'd be interested in learning more about the implementation process. What steps are involved in utilizing ChatGPT for network prototyping? Are there any specific prerequisites or challenges to consider?
While the concept is intriguing, I wonder about the learning curve for users who are not familiar with AI systems. Is ChatGPT user-friendly enough for network designers to leverage effectively, or does it require extensive training?
Linda Parker, user-friendly interfaces should indeed be a priority. Implementing guided prompts and clear instructions within ChatGPT's interface can help network designers seamlessly leverage its capabilities, even with minimal AI training.
@Nathan Hill: Guided prompts and clear instructions within ChatGPT's interface can indeed bridge the gap for network designers who might not have extensive AI training. User-friendly experiences will enhance the adoption of AI technologies in network design.
@Sara Johnson: Excellent question. AI models like ChatGPT have limitations when dealing with complex network configurations. However, by continuously training the model with diverse data, we can improve its understanding and accuracy in these scenarios.
Mark, you mentioned training the model with diverse data to improve its understanding. How would you ensure the training data covers a wide range of network configurations and scenarios?
Good question, Daniel. In the training phase, it's crucial to curate a diverse dataset that covers a wide range of network configurations. This can involve collecting data from various sources, including existing network designs, real-world examples, and simulated scenarios.
@Robyn Barratt: Could you shed some light on the implementation process? What are the main steps involved in leveraging ChatGPT for network prototyping?
@Brian Thompson: Implementing ChatGPT for network prototyping involves data collection, preprocessing, fine-tuning the model on network design data, and creating an interface for designers to interact with the system. It's important to iterate and refine the process to ensure accurate results.
@Robyn Barratt: How does ChatGPT handle scalability? Can it be effectively applied to large-scale network design projects?
@Julia Thompson: ChatGPT's scalability depends on the computational resources available. With sufficient resources, it can be applied effectively to large-scale network design projects. However, it's important to ensure efficient infrastructure to handle the computational demands.
Robyn, during the fine-tuning process, how do you evaluate and measure the accuracy of ChatGPT's suggestions? Are there any metrics in place to assess its performance?
@Samantha Jackson: Evaluating ChatGPT's accuracy involves comparing its suggestions against known correct solutions or expert opinions. Metrics like precision, recall, and F1 score can be used to measure its performance. Additionally, user feedback and real-world validation play an important role in assessing its accuracy.
@Robyn Barratt: User feedback and real-world validation are indeed important in assessing accuracy. Incorporating human oversight and reviewing generated suggestions can help identify any potential biases or errors in the system's outputs.
@Robyn Barratt: In the context of network design, how do you handle cases where ChatGPT generates incorrect or suboptimal suggestions?
@Michael Adams: When ChatGPT generates incorrect or suboptimal suggestions, it highlights the need for human oversight. Designers have the ability to review the suggestions, provide feedback, and make necessary corrections. This collaborative approach ensures the accuracy and quality of the final output.
Robyn, your article showcases the potential of ChatGPT in network prototyping. I'm excited to witness how this technology evolves as it becomes more widely adopted. Great insights!
@Robyn Barratt: The collaborative approach sounds promising to handle incorrect suggestions. It ensures the human expert's influence while leveraging the potential benefits of AI in network design.
@Michael Adams: You're absolutely right. The collaboration between AI and human experts ensures a synergy that combines the best of both worlds. It allows for error detection, knowledge refinement, and enhances the overall quality of network designs.
@Michael Adams: Completely agree with you. AI models can assist in network design, but they should not replace human expertise. The combined strengths of AI and human intelligence can lead to more robust and efficient network solutions.
@Robyn Barratt: Thanks for the clarification. It's reassuring to know that ChatGPT's scalability is dependent on available computational resources. This flexibility can make it suitable for various network design projects.
Robyn, in the interface for designers, how does ChatGPT handle feedback and iteration during the design process? Is there a way to provide clarifications or refine the generated suggestions?
@Liam Wilson: The interface provides designers with the ability to provide feedback on the generated suggestions. This feedback loop is an essential component for refining the system. Designers can ask for clarifications, make modifications, or request new suggestions based on their requirements.
@Robyn Barratt: Glad to hear that the feedback loop is integrated into the interface. It allows designers to have more control and ensures the final output aligns with their specific requirements.
While the potential benefits of ChatGPT in network design are exciting, have any real-world implementations or case studies been conducted? It would be interesting to see some practical examples.
@Sarah Mitchell: Real-world implementations and case studies are still limited in the context of network design, but there have been successful applications of ChatGPT in other domains such as natural language processing and creative writing. Network design can benefit from those experiences and adapt accordingly.
@Robyn Barratt: Thank you for addressing my question. Drawing insights from other domains where ChatGPT has been applied successfully sounds promising. Network design can benefit from lessons learned in those areas.
As an AI enthusiast, I believe that user-friendliness is a crucial aspect to encourage wider adoption. It would be beneficial to prioritize developing intuitive interfaces to make ChatGPT more accessible for network designers.
Alice Collins, I fully support the need for intuitive interfaces. As AI technologies continue to evolve, creating user-friendly experiences will be pivotal in encouraging wider adoption across various industries, including network design.
In network prototyping, quick decision-making is crucial to meet project timelines. Can ChatGPT generate accurate suggestions in real-time?
Lisa, generating accurate suggestions in real-time depends on various factors like system resources, model complexity, and dataset size. With proper setup and optimization, real-time generation can be achieved, but it might require some trade-offs.
@Mark Anderson: Thank you for your insights. I understand that trade-offs may be necessary for real-time generation. It would be interesting to see how well ChatGPT can balance speed and accuracy in network prototyping.
@Lisa Roberts: Balancing speed and accuracy is indeed a crucial aspect. By iteratively refining the model and fine-tuning it based on domain-specific requirements, we can aim for an optimal balance between real-time generation and accurate suggestions.
@Mark Anderson: Thanks for the insights. Curating a diverse dataset seems essential for training. It would be interesting to see how well the model generalizes to real-world, unseen network configurations.
@Mark Anderson: Continuous training with diverse data is indeed crucial to improve ChatGPT's understanding. Incorporating ongoing learning can help the model adapt to different network configurations and enhance its overall performance.
@Mark Anderson: How do you measure the accuracy of ChatGPT's suggestions? Are there any quantitative metrics to determine its effectiveness in network design tasks?
@Emma Wilson: Measuring the accuracy of ChatGPT's suggestions involves both quantitative and qualitative methods. Quantitatively, metrics like BLEU score, ROUGE score, or even perplexity can be used. However, qualitative assessments through human review, feedback, and real-world validation play a crucial role in evaluating its effectiveness in network design tasks.
@Mark Anderson: Thank you for explaining the evaluation process. A comprehensive evaluation methodology that combines quantitative and qualitative aspects ensures a more robust assessment of ChatGPT's performance.
@Mark Anderson: Achieving an optimal balance between speed and accuracy will be critical. It would be fascinating to witness how ChatGPT evolves and adapts to different network design requirements over time.
Considering how rapidly network configurations evolve, it's crucial to have a system like ChatGPT that can adapt and learn from new patterns and industry trends. Keeping the model up to date with the latest developments will be key to its effectiveness.