Harnessing the Power of ChatGPT for Advanced Commodity Risk Management in Investment Analysis
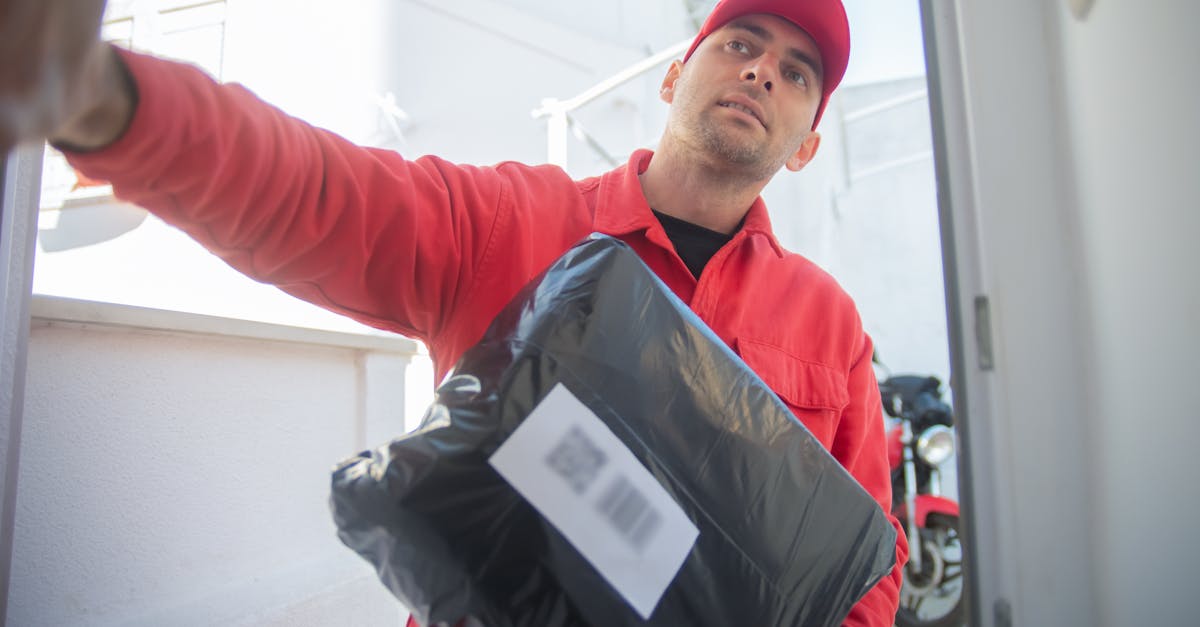
Commodity risk management in the field of investment analysis plays a crucial role in evaluating the potential return of investment in specific commodities and assessing associated risks. With the advancements in artificial intelligence (AI), the process of analyzing commodity markets has become more efficient and accurate.
AI technology utilizes machine learning algorithms and statistical models to analyze vast amounts of data from various sources, such as market prices, weather patterns, geopolitical events, and supply and demand trends. By applying these algorithms, AI can generate meaningful insights and predictions regarding future price movements and associated risks.
One of the key advantages of AI in commodity risk management is its ability to evaluate historical data and identify patterns, assisting in the identification of potential investment opportunities and risks. By analyzing past market behavior, AI models can highlight correlations, seasonal trends, and anomalies that might impact the future performance of commodities or markets.
Furthermore, AI can process unstructured data from diverse sources, including news articles, social media, and online forums, to capture market sentiment and public opinions. This sentiment analysis helps investors in understanding the broader market dynamics and the impact of external factors on commodity prices.
The real-time monitoring capabilities of AI technology are also beneficial for commodity risk management. AI algorithms can continuously analyze market conditions and react to changing circumstances promptly. As commodity prices are influenced by several variables, including economic indicators, political events, and natural disasters, AI can quickly identify potential risks and assist in implementing appropriate risk mitigation strategies.
Another crucial aspect of AI technology in commodity risk management is the ability to quantify and manage risk exposure. AI models enable investors to simulate different scenarios and assess the potential impact of various risk factors on their investment portfolios. By understanding the potential gains and losses associated with different investment decisions, investors can make informed choices and implement risk management strategies accordingly.
The use of AI in commodity risk management also allows for improved decision-making processes. AI models can analyze large datasets with speed and precision, reducing the impact of human biases and emotional decision-making. By relying on data-driven insights, investors can make more objective and informed decisions, resulting in potentially better investment outcomes.
However, it is important to note that while AI can provide valuable insights and predictions, it is not a guarantee of success in investment analysis. Commodity markets can be volatile and unpredictable, and unforeseen events can disrupt even the most advanced AI models. Therefore, it is crucial for investors to combine AI technology with comprehensive market knowledge and effective risk management strategies.
In conclusion, AI technology has revolutionized commodity risk management in investment analysis. By leveraging machine learning algorithms and statistical models, AI can analyze vast amounts of data, identify patterns, and make predictions regarding the potential return of investment and associated risks in specific commodities. With real-time monitoring capabilities and the ability to process unstructured data, AI provides investors with valuable tools to make informed decisions and mitigate risk exposure. However, it is important to remember that AI should be used as a tool alongside human judgment and market knowledge to achieve optimal investment outcomes.
Comments:
Thank you all for joining the discussion on my article 'Harnessing the Power of ChatGPT for Advanced Commodity Risk Management in Investment Analysis'. I'm excited to hear your thoughts and answer any questions you may have.
Great article, Ely! I found your approach to using ChatGPT in investment analysis very insightful. It seems like a powerful tool for improving commodity risk management.
Thanks, Brian! I appreciate your feedback. Yes, ChatGPT can indeed enhance risk management by providing a more comprehensive analysis of commodity markets. Have you had any experience using ChatGPT in your investment analysis?
I enjoyed reading the article, Ely. It's impressive how AI is revolutionizing various industries, including investment analysis. Do you think ChatGPT can accurately predict commodity price movements?
Thank you, Karen! AI is indeed transforming the way we approach investment analysis. While ChatGPT can provide valuable insights, it's important to note that predicting commodity prices accurately is still a challenging task. However, ChatGPT's analysis can help identify trends and patterns that contribute to a more informed decision-making process.
Ely, I found your article to be a fascinating read. Can you explain how ChatGPT tackles the issue of fluctuating market conditions when analyzing commodity risks?
Thank you, Samuel! ChatGPT adapts to market conditions by continuously learning from updated data. It takes into account historical trends, current news, and market indicators to provide risk assessments that align with the latest market conditions. This adaptability allows for a more dynamic analysis of commodity risks.
Ely, I appreciate your article and insights. However, do you think there are any limitations to relying solely on ChatGPT for commodity risk management? Human judgment is vital in investment analysis, after all.
Hi Isabel! You raise an excellent point. While ChatGPT can enhance risk management, human judgment remains crucial. Commodity markets are complex, and a combination of AI-powered analysis and human expertise provides the most comprehensive approach.
Ely, I'm intrigued by the potential of ChatGPT in commodity risk management. Are there any specific prerequisites or data requirements for implementing this technology effectively?
Thanks for your interest, Robert! Effective implementation of ChatGPT requires a vast amount of historical market data, relevant news and articles, and suitable training datasets. Additionally, continuous updates and fine-tuning are needed to ensure the model stays up to date with the evolving commodity markets.
Ely, your article opened my eyes to the potential of AI in investment analysis. How accessible is this technology for small and medium-sized investment firms?
Thank you, Linda! AI technology, including ChatGPT, is becoming increasingly accessible for small and medium-sized investment firms. With cloud-based solutions and platform-as-a-service offerings, such technologies are more affordable and scalable. However, specific implementation details may vary, so it's essential to consult with AI solution providers for tailored solutions.
Ely, fantastic article! I can see the potential of ChatGPT in improving investment analysis. Do you think this technology will eventually replace traditional methods?
Thanks, Adam! While ChatGPT and AI technologies offer powerful analysis capabilities, I believe they will augment traditional methods rather than replace them entirely. Human expertise, critical thinking, and domain knowledge are indispensable in investment analysis. The synergy between AI and human judgment is where we can truly maximize the potential of these technologies.
Ely, your article shed light on the fascinating application of ChatGPT in commodity risk management. How does this technology handle rare events or unforeseen market disruptions that can significantly impact investments?
Thank you, Sophia! Handling rare events and unforeseen market disruptions is a challenge for any analysis tool, including ChatGPT. Training the model on historical data that encompasses such events helps to a certain extent. However, staying updated with real-time news and monitoring market indicators remain crucial to spot early signs of significant disruptions and make informed decisions.
Ely, your article presents an exciting use case for ChatGPT. What are your thoughts on potential ethical concerns or biases that may arise from using AI models in investment analysis?
Thanks, Martin! Ethical concerns and biases are indeed important considerations. It's crucial to ensure the training data for ChatGPT is diverse, representative, and carefully evaluated to mitigate bias issues. Regular evaluation and monitoring of the model's outputs are necessary to maintain ethical standards and avoid unintended consequences.
Ely, your article provides valuable insights into the potential of ChatGPT. Is there any specific feedback mechanism to improve the AI model's performance over time?
Thank you, Emily! Continuous feedback is essential to improve the AI model's performance. Gathering feedback from users, investment professionals, and domain experts helps identify model limitations, address any biases, and refine the analysis over time. This iterative process contributes to the ongoing development and enhancement of the technology.
Ely, I thoroughly enjoyed your article. How do you envision the future of AI in commodity risk management? Any exciting developments we can expect?
Thanks, Daniel! The future of AI in commodity risk management is promising. We can anticipate more sophisticated AI models that integrate various data sources, including unstructured data like social media and satellite imagery. As technology advances, AI will continue to evolve, providing enhanced risk management capabilities and unlocking new insights for investment professionals.
Ely, your article was a fascinating read. How does ChatGPT handle complex and interconnected commodity markets, where factors in one market can impact others?
Thank you, Sarah! ChatGPT leverages its ability to analyze multiple sources of information to handle complex and interconnected commodity markets. By considering a broad range of factors, it can identify interdependencies and correlations between markets, enabling a more comprehensive understanding of the overall commodity landscape.
Ely, I found your article incredibly informative. Are there any regulatory considerations or frameworks in place for using ChatGPT in investment analysis?
Thanks, Grace! Regulatory considerations are crucial in using AI in investment analysis. Depending on the jurisdiction, there might be specific frameworks or guidelines to follow. Adhering to data privacy regulations, ensuring transparency, and regularly assessing the model's compliance with regulatory requirements are essential aspects when implementing ChatGPT or any AI technology in investment analysis.
Ely, your article provided valuable insights into the application of ChatGPT in commodity risk management. Can you discuss any challenges in implementing AI technology within investment firms?
Thank you, Oliver! Implementing AI technology within investment firms presents certain challenges. Acquiring and managing the necessary data, ensuring data quality, and addressing potential biases are crucial steps. Additionally, integrating AI solutions seamlessly into existing workflows, addressing any resistance to technology adoption, and continuously updating and improving the models are challenges that need to be addressed for successful implementation.
Ely, I enjoyed your article. Can ChatGPT be used beyond commodity risk management? Are there other areas in investment analysis where this technology can be beneficial?
Thanks, Thomas! Absolutely, ChatGPT's capabilities extend beyond commodity risk management. It can be leveraged for sentiment analysis, macroeconomic forecasts, portfolio optimization, and even driving real-time investment decisions based on market news and trends. The diverse applications in investment analysis make it a versatile tool for professionals in this field.
Ely, your article was a great read! I'm curious about the computational resources required to implement ChatGPT for commodity risk management. Can you provide some insights?
Thank you, Sophie! Implementing ChatGPT for commodity risk management requires significant computational resources. Training large-scale models like ChatGPT can be computationally intensive and requires specialized hardware configurations or access to cloud-based AI services. However, once trained, deploying the model for risk analysis can be done on more moderate hardware.
Ely, your article was excellent! How would you address concerns about potential biases in ChatGPT's analysis, particularly if the training data itself contains biases?
Thanks, Michael! Addressing biases is a crucial aspect of deploying AI models like ChatGPT. Careful evaluation and preprocessing of the training data can help mitigate biases to a certain extent. Additionally, monitoring the model's outputs and including diverse perspectives during the training stage helps mitigate and rectify any biased or skewed analysis.
Ely, your article provided an interesting perspective on ChatGPT in investment analysis. Can you provide any insights into the implementation timeline of such AI systems for risk management?
Thank you, Emma! The timeline for implementing AI systems like ChatGPT for risk management can vary depending on numerous factors. Acquiring and processing the necessary data, developing and training the model, fine-tuning and validating the results, and deploying the system within the investment firm's infrastructure all contribute to the implementation timeline. It's essential to plan and allocate resources accordingly to ensure a successful deployment.
Ely, your article was thought-provoking. Considering the dynamic nature of commodity markets, what steps can be taken to keep ChatGPT's analysis up to date?
Thanks, Lucas! Keeping ChatGPT's analysis up to date is crucial in commodity risk management. Regularly updating the model with new data, continuously monitoring market indicators, and incorporating real-time news and events into the analysis are essential steps. Additionally, fine-tuning the model periodically based on newly available information helps in adapting to evolving market conditions and ensuring the analysis remains accurate.
Ely, your article shed light on the potential of using ChatGPT for advanced commodity risk management. Do you think this technology will be widely adopted in investment firms in the near future?
Thank you, Laura! I believe the adoption of technology like ChatGPT in investment firms will continue to grow. As AI systems become more mature, affordable, and accessible, investment professionals are discovering the benefits of incorporating these technologies into their risk management strategies. While there may be challenges in implementation, the potential for improved insights and decision-making makes it a compelling choice for investment firms.
Ely, your article provided great insights into the application of AI in investment analysis. What considerations should investment firms keep in mind when vetting AI solutions like ChatGPT?
Thanks, Matthew! When evaluating AI solutions like ChatGPT, investment firms should consider factors such as the model's accuracy, transparency, interpretability, and scalability. Assessing the vendor's track record, understanding the model's limitations and potential biases, and ensuring data privacy and regulatory compliance are also essential aspects. Collaborating with experienced AI solution providers and conducting thorough evaluations will help investment firms make informed decisions.
Ely, your article was an eye-opener to the potential of ChatGPT in commodity risk management. What are the key advantages of using AI technologies compared to traditional approaches?
Thank you, Julia! AI technologies like ChatGPT offer several advantages compared to traditional approaches. These include the ability to process large volumes of data quickly, uncovering hidden patterns, identifying complex interdependencies, and providing more thorough risk assessments. AI can also augment human expertise by analyzing diverse sources of information simultaneously, freeing up time for investment professionals to focus on strategic decision-making.
Ely, great article! Do you think there are any potential risks associated with overreliance on AI models like ChatGPT in investment analysis?
Thanks, Jason! Overreliance on AI models has its risks. AI models are only as good as the data they are trained on, and unexpected events or rare market conditions can challenge the model's accuracy. Additionally, biases in the training data, interpretability issues, and potential algorithmic vulnerabilities are other risks to consider. It's important to strike a balance between AI-powered analysis and human judgment to ensure comprehensive risk management.
Ely, your article provided a fresh perspective on using AI in investment analysis. Can ChatGPT's analysis be customized based on specific investment strategies or preferences?
Thank you, Natalie! Yes, ChatGPT's analysis can be customized based on specific investment strategies and preferences. By training the model on tailored datasets and incorporating relevant indicators, investment firms can fine-tune the analysis to align with their unique approaches. This customization enhances the model's relevance and ensures that the insights generated are aligned with the investment firm's strategies and objectives.
Thank you all for your valuable contributions to the discussion. Your questions and insights have been insightful, and I hope this conversation has provided a deeper understanding of leveraging ChatGPT for advanced commodity risk management. Feel free to reach out if you have any further questions or if I can be of assistance in any way. Have a great day!