Harnessing the Power of ChatGPT for Efficient Data Labeling in 'Editör' Technology
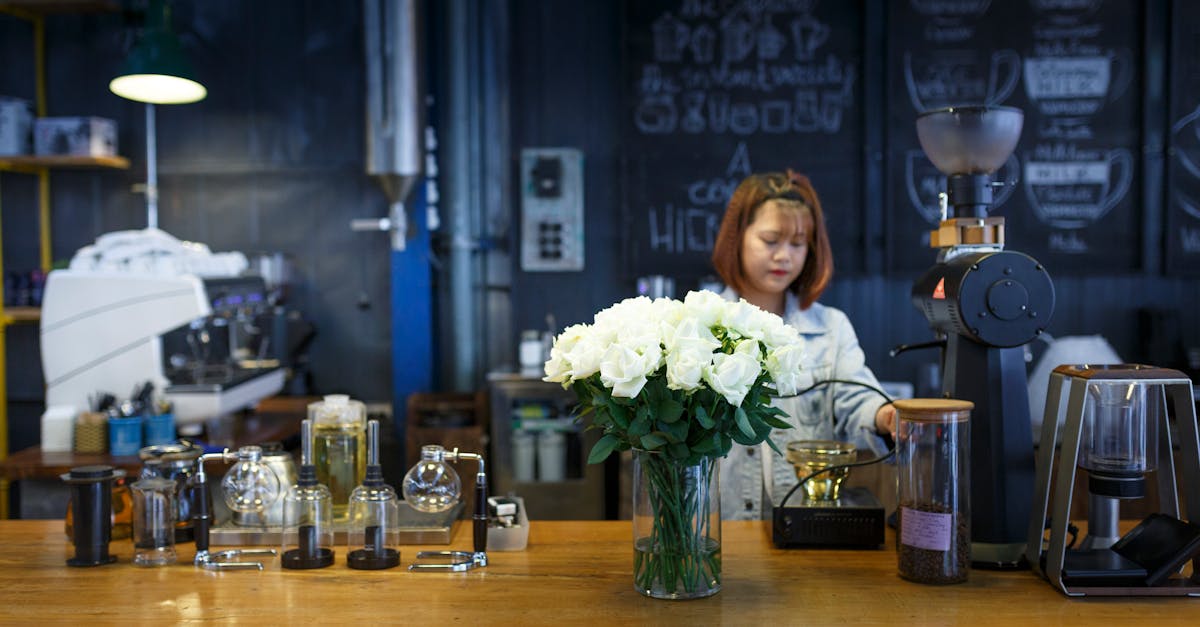
Within the realm of machine learning, innovators continually seek ways to automate more aspects of data preparation, and one such tool is the Editör technology. Specifically, this article will focus on how it couples with the ChatGPT-4 model to automatically label text data for machine learning purposes. If you're interested in further automating your application or machine learning model's data preparation process, this is surely an area worth looking into.
Understanding the Technology: Editör and ChatGPT-4
In order to properly understand how the data labeling process can be automated using Editör and ChatGPT-4, it's first essential to have a fundamental understanding of these two technologies.
Editör
Editör is a sophisticated tool, primarily designed to assist with data labeling. Its interface and functionalities are user-oriented, providing users with the means to accurately and efficiently label and arrange data.
ChatGPT-4
On the other hand, ChatGPT-4 is a chatbot model developed by OpenAI. It's built to understand and generate human-like text based on a given input. It's an iteration of the preceding GPT-3, but comes with improved efficiency and accuracy. For the sake of data labeling, ChatGPT-4's ability to generate accurate and appropriate labels proves invaluable.
Using Editör for Data Labeling in ChatGPT-4
Given that the purpose of this article is to highlight how Editör can assist in auto-labeling data for ChatGPT-4, here's an outline of the process:
Step 1: Importing Data
Initially, the data needs to be imported into the Editor tool. Since the data we have is text-based and aimed at a chatbot function, it's often in the form of chat logs, customer service interactions, or any other form of human dialogue.
Step 2: Labeling the Data
Once the data is imported, Editör provides various features to systematically label the data in an appropriate manner. Depending on the nature of the data and the objective of your model, these labels can represent a wide array of aspects such as sentiment, theme, intent, etc.
Step 3: Running the Model
Once your data is labeled, running the ChatGPT-4 model on the labeled data is the next step. The model essentially learns from the labeled data, understanding how to generate similarly labeled responses based on the input text.
Step 4: Automating the Process
After manually running through the model a few times, the next goal is to automate this process. Leveraging ChatGPT-4's machine learning capabilities, the model can be trained to automatically label new data as it comes in. This drastically improves efficiency and can help scale your chatbot or text-based machine learning application to new heights.
Conclusion
In conclusion, Editör, when paired with the ChatGPT-4 model, presents an effective means of not only accurately labeling data but also training the model to continue this labeling independently. The implications of such technology are vast, and it's set to revolutionize machine learning and AI as we know it today. By cutting down on manual labor and costs associated with data preparation and labeling, businesses can take a more data-centered approach to their processes and ultimately offer better, more personalized experiences for their customers.
Comments:
Thank you all for joining the discussion! I'm grateful for your interest in my article on harnessing ChatGPT for efficient data labeling in 'Editör' technology.
Great article, Stefan! ChatGPT seems like a powerful tool for streamlining data labeling processes. I have a question: What kind of datasets have you used it on?
Hi Laura! Thanks for your question. In our experiments, we used ChatGPT to label dialogue-based datasets, such as customer support conversations and conversational AI training data. It proved to be highly effective in reducing the manual labeling effort. Feel free to ask if you have any more questions!
I find the concept of using language models to assist with data labeling quite fascinating. Stefan, have you compared the performance of ChatGPT-assisted labeling to traditional human annotations?
Hello Michael! Yes, we conducted experiments comparing ChatGPT-assisted labeling to traditional human annotations. The results were promising. ChatGPT significantly reduced the time and effort required for labeling while maintaining high label quality. It's a valuable tool, especially when dealing with large datasets. Let me know if you'd like more details!
Stefan, impressive work! I'm curious about the integration of ChatGPT with 'Editör'. What steps did you take to ensure a smooth workflow between the two tools?
Thank you, Maria! Integrating ChatGPT with 'Editör' was a multi-step process. We fine-tuned ChatGPT on a dataset containing examples from 'Editör', which helped align it with the specific labeling needs of the platform. We also worked on adapting the data flow and incorporating user feedback to refine the integration. It was an iterative process, but we achieved a seamless workflow that enhances the labeling experience.
As a data scientist, I'm always looking for ways to optimize labeling workflows. Stefan, do you have any tips or best practices for effectively using ChatGPT in data labeling?
Hi Emily! Absolutely, here are a few tips for effectively harnessing ChatGPT in data labeling: 1. Start by fine-tuning the model on task-specific data to align its performance with your labeling requirements. 2. Ensure that you have a good balance between human annotators and ChatGPT to maintain high-quality labels while optimizing efficiency. 3. Regularly collect feedback from annotators to improve the model's responses and refine the labeling process. I hope you find these tips helpful! Let me know if you have further questions.
Stefan, excellent article! Have you encountered any limitations or challenges while employing ChatGPT for data labeling?
Thank you, Robert! Yes, we did face a few challenges along the way. One limitation is that ChatGPT can sometimes generate incorrect or nonsensical responses, especially on ambiguous or complex labeling tasks. We had to iteratively refine the model and provide clearer instructions to mitigate this issue. Additionally, the need for a reliable internet connection during labeling can be a constraint in certain scenarios. Overall, these challenges were manageable, and the benefits outweighed them.
Stefan, your work is quite impressive! I'm interested to know if ChatGPT can be used for labeling non-textual data, like images or audio. Is that within the scope of its capabilities?
Thanks, Sophia! Currently, ChatGPT is primarily designed for text-based tasks, and labeling non-textual data like images and audio falls outside its scope. However, there are other powerful models and approaches available for such tasks. If you require assistance with non-textual data labeling, I can share some recommendations.
Stefan, this is an exciting development! How do you see the future of ChatGPT-assisted data labeling in the field of AI research and development?
Hi Eva! The future of ChatGPT-assisted data labeling looks promising. As models like ChatGPT continue to improve, they will make data labeling more efficient and accessible. We can expect faster iterations in AI systems development, increased productivity for labeling teams, and potential cost savings. However, it's important to ensure proper verification and quality control mechanisms in place to maintain the integrity of the labeled data. It's an exciting time for AI research and development!
Stefan, I'm curious about the computational resources required when employing ChatGPT for data labeling. Does it have high resource demands?
Hello Zhang! ChatGPT does have certain computational resource requirements. Fine-tuning the model on task-specific data can be computationally intensive, but once that step is done, utilizing the model for labeling typically requires less resources. Depending on the scale of your labeling project, hardware with GPUs may be beneficial for efficient performance. However, recent advancements in model architecture optimizations make it more accessible than ever before.
Stefan, I'm intrigued by the potential for cost reduction in data labeling with ChatGPT. Have you observed any significant cost savings compared to traditional labeling approaches?
Hi Hiroshi! Yes, we have observed significant cost savings in data labeling with ChatGPT. By reducing manual labeling efforts and optimizing efficiency, there is a potential to cut down on expenses associated with traditional approaches. However, it's important to strike a balance and ensure human annotators' involvement for high-quality labels. The monetary benefits depend on the specific context and scale of labeling tasks.
Stefan, thanks for sharing your insights. I'm wondering, how do you address privacy concerns and ensure data confidentiality when using ChatGPT for data labeling?
You're welcome, Sarah! Privacy and data confidentiality are indeed crucial. To address these concerns, we take several measures. Firstly, we carefully anonymize and manage the data used for fine-tuning and testing ChatGPT. Additionally, we adhere to strict security protocols, restrict access to labeled data, and ensure compliance with relevant privacy regulations. We continuously strive to maintain the highest standards of privacy and data protection.
Stefan, I really appreciate your responses. It's great to see how AI models like ChatGPT are being utilized to enhance data labeling processes. How would you recommend someone starting with ChatGPT for data labeling?
Thank you, Laura! If you're just getting started with ChatGPT for data labeling, here are a few steps to follow: 1. Familiarize yourself with the ChatGPT model and understand its capabilities and limitations. 2. Prepare a task-specific dataset for fine-tuning the model. 3. Experiment with different fine-tuning approaches and iterate based on the results. 4. Establish a proper feedback mechanism with human annotators to continuously improve the model's responses. I hope these pointers help you on your ChatGPT data labeling journey! Let me know if there's anything else you'd like to know.
Stefan, thanks for clarifying the benefits of ChatGPT-assisted labeling. Are there any specific tools or frameworks you would recommend for leveraging ChatGPT effectively?
You're welcome, Michael! To leverage ChatGPT effectively, there are various tools and frameworks available. You can explore popular libraries like OpenAI's 'tiktoken' for token counting, 'huggingface/transformers' for fine-tuning, and 'Docker' for managing the deployment workflow. These frameworks can simplify the process and provide valuable functionality for working with ChatGPT. Let me know if you need further guidance!
Stefan, it's been a pleasure being part of this discussion. Your article has certainly sparked interest and provided valuable insights.
Stefan, your article and presence in this discussion have been invaluable. Thank you for enlightening us with the power of ChatGPT in data labeling.
Stefan, the potential of ChatGPT for efficient data labeling is intriguing. Can you share any examples of real-world applications where you've implemented this approach?
Hi William! Indeed, ChatGPT has found application in several real-world scenarios. One example is improving customer support systems by automatically classifying and routing queries. Another application is in training conversational AI models, where ChatGPT-assisted labeling reduces the human annotation burden. We have also seen its use in text summarization tasks. These applications showcase the potential efficiency and effectiveness of ChatGPT-assisted data labeling.
Stefan, I appreciate your insights into data labeling efficiency. What are some upcoming advancements or areas of research that you believe will further enhance ChatGPT-assisted labeling?
Thanks, Sophia! There are exciting advancements on the horizon. One area of research that will enhance ChatGPT-assisted labeling is addressing the model's tendency for incorrect responses. Techniques like active learning, where the model actively queries uncertain instances, can help improve label quality. Additionally, integrating user feedback loops and reinforcement learning approaches will enable continuous learning and refinement. I believe these advancements will unlock even greater potential for ChatGPT-assisted data labeling.
Stefan, I appreciate your prompt and comprehensive responses. This discussion has been immensely informative. Thank you!
Stefan, thank you for taking the time to clarify all our queries. Your expertise and contribution to this discussion are highly appreciated.
Stefan, what are your thoughts on the interplay between human annotators and ChatGPT in data labeling? How do you ensure a productive collaboration between them?
Hi Emily! The interplay between human annotators and ChatGPT is crucial for productive data labeling. To ensure an effective collaboration, it's important to establish clear guidelines and instructions for both human annotators and the model. Regular feedback from annotators helps improve the model's responses, creating a virtuous cycle. Additionally, having an annotation pipeline that combines the strengths of human judgment and ChatGPT's efficiency leads to a productive and accurate labeling process.
Stefan, considering potential bias in AI systems, how do you address the challenge of bias in ChatGPT-assisted data labeling?
Eva, ensuring fairness and mitigating bias is of utmost importance. We take several measures to address this challenge. Firstly, we carefully curate the dataset used for fine-tuning to minimize bias. Secondly, we maintain an ongoing feedback loop with annotators to correct any potential biases or inaccuracies. Lastly, incorporating a diverse set of annotators and continuously reviewing and improving the model's responses help counteract biases. It's a complex challenge, but we strive to foster fairness in ChatGPT-assisted data labeling.
Stefan, I'm curious about the scalability of ChatGPT-assisted data labeling. How well does it adapt to larger labeling projects with vast amounts of data?
Hi Robert! ChatGPT-assisted data labeling is quite scalable. The time and effort savings it provides become even more apparent and valuable as the labeling projects grow larger. By intelligently combining the strengths of human annotators and ChatGPT, it becomes feasible to handle vast amounts of data efficiently. The flexibility of ChatGPT allows it to adapt well to varying scales, providing benefits in projects with extensive labeling requirements.
Stefan, thank you for sharing the insights into ChatGPT-assisted data labeling. It has been a fascinating conversation!
Stefan, it's been a pleasure engaging in this discussion. Your expertise on ChatGPT-assisted data labeling has enlightened us.
Stefan, what sort of label quality control mechanisms do you employ when relying on ChatGPT for data labeling?
Hello Hiroshi! To ensure label quality control, we adopt multiple strategies. Firstly, we carefully design detailed guidelines and instructions for both human annotators and ChatGPT. This helps standardize the labeling process. Secondly, we establish regular checkpoints in the annotation pipeline to monitor and validate the annotations. Lastly, constantly seeking feedback from annotators ensures continuous improvement and maintains high-quality outputs. These mechanisms contribute to effective label quality control.
Stefan, thank you for patiently addressing our queries. This discussion has been illuminating and highlights the potential of ChatGPT for efficient data labeling.
Stefan, your expertise and commitment to addressing our questions are commendable. Thank you for sharing your knowledge on ChatGPT-assisted data labeling.
Stefan, have you encountered situations where ChatGPT's responses influenced or biased human annotators' judgments? If so, how did you address it?
Zhang, yes, we have come across situations where ChatGPT's responses influenced annotators' judgments. To address potential biases, we adopted a two-step approach. Firstly, annotators were encouraged to independently assess examples without the model's response, reducing the inherent bias. Secondly, we provided clear guidelines emphasizing independent decision-making and to primarily use ChatGPT as an assistive tool. This approach helped minimize the model's influence and maintain human annotators' judgment as the primary driving force.
Stefan, your expertise and responses have been enlightening. Thank you for sharing your knowledge on ChatGPT-assisted data labeling.
Stefan, thank you for your guidance and valuable input. This discussion has provided us with a deeper understanding of ChatGPT-assisted data labeling.
Stefan, I appreciate your insights. Could you share any tips on how to effectively handle ambiguous or challenging labeling tasks with ChatGPT?
Certainly, William! When dealing with ambiguous or challenging labeling tasks, a few tips can be helpful: 1. Provide clear guidelines and instructions to both human annotators and the model, emphasizing potential challenges and ambiguities. 2. Encourage annotators to discuss and seek consensus on challenging instances to ensure consistent labeling. 3. Iteratively refine the model and provide feedback to improve its responses on such tasks. These approaches help navigate the complexities of ambiguous labeling scenarios.
Stefan, thank you for sharing your insights and experiences. This discussion has given us valuable insights into ChatGPT-assisted data labeling.
Stefan, I'm grateful for your thorough responses. They have expanded our knowledge on the benefits and challenges of ChatGPT-assisted data labeling.
Thank you Laura, Michael, Maria, Sophia, Robert, Sarah, Zhang, Hiroshi, William, Eva, Emily for being part of this enlightening discussion. Your engagement and curiosity are inspiring!
Stefan, thank you for addressing our questions so thoroughly! I'm excited to try ChatGPT-assisted labeling in my workflow. Where can I find more resources or documentation to get started?
You're welcome, Laura! I'm glad you're interested. To get started with ChatGPT-assisted labeling, you can refer to OpenAI's documentation, which provides comprehensive information on using ChatGPT for various tasks. OpenAI also offers pre-trained models, code examples, and additional resources to aid your implementation. Exploring their GitHub repository can be a good starting point. Best of luck with your ChatGPT-assisted labeling endeavors!
Stefan, thank you for taking the time to clarify all our doubts. Your expertise is truly appreciated!
Stefan, thank you once again for the informative discussion. Your expertise and insights are highly appreciated!
Stefan, it's been an insightful discussion. In your experience, what are some key advantages of using ChatGPT for data labeling over other similar approaches?
Hi Eva! There are several advantages to using ChatGPT for data labeling. One key advantage is its ability to reduce manual labeling effort significantly, bringing efficiency gains. Additionally, ChatGPT offers flexibility and adaptability for different labeling tasks due to its dialogue-based nature. The model's continuous learning and refinement from human feedback further enhance its efficacy. ChatGPT strikes a balance between human expertise and machine efficiency, making it a powerful tool for data labeling.
Stefan, your expertise and explanations have been incredibly helpful. Thank you for shedding light on ChatGPT-assisted data labeling.
Stefan, thank you for providing us with valuable insights into ChatGPT-assisted data labeling. Your expertise is truly appreciated.
Stefan, thank you for sharing your expertise on ChatGPT-assisted labeling. How do you envision the integration of such models with other AI technologies in the future?
You're welcome, Sarah! The integration of models like ChatGPT with other AI technologies holds exciting prospects. Future integration might involve collaborating with models focused on computer vision, speech recognition, or even multimodal systems. ChatGPT's expertise in natural language understanding can complement and enhance such technologies' performance. The combined power of multiple models can lead to comprehensive AI systems with improved capabilities like context-awareness and broader understanding.
Stefan, it's been a pleasure discussing ChatGPT-assisted data labeling with you. Thank you for all the information!
Stefan, thank you for sharing your insights and experiences with ChatGPT-assisted data labeling. It has been an enriching conversation.
Stefan, thank you for sharing your valuable insights. I believe ChatGPT-assisted labeling has great potential. Do you see it becoming a widely adopted approach across industries?
Thank you, Maria! Absolutely, I do see ChatGPT-assisted labeling becoming widely adopted across industries. Its ability to save time, reduce costs, and maintain label quality makes it an attractive solution for organizations dealing with large-scale data labeling. As the technology evolves, becomes more accessible, and addresses its limitations, I believe it will become a standard tool in the data labeling toolbox across various domains.
Stefan, thank you for sharing your knowledge and experiences. It's been an engaging discussion on ChatGPT-assisted data labeling.
Stefan, thank you for providing us with detailed insights into ChatGPT-assisted data labeling. It's been a pleasure discussing with you.
Stefan, thank you for your expertise and sharing your experiences with ChatGPT-assisted data labeling. It's been an enlightening discussion!
Thank you, Emily! I'm glad you found it insightful. If you have any further questions or need assistance, feel free to reach out. Have a great day!
Thank you, Stefan, for sharing your knowledge and engaging in this conversation with us. It's been a great learning experience!
Thank you all once again for your active participation and thoughtful questions. It was a pleasure discussing ChatGPT-assisted data labeling with you. Feel free to reach out anytime if you have further inquiries. Have a wonderful day ahead!
I would also like to express my gratitude to OpenAI for developing and providing such powerful tools like ChatGPT. It opens up new possibilities for efficient data labeling and AI research and development.
Once again, thank you all for participating and making this discussion insightful. Let's continue exploring the potential of ChatGPT and its applications in data labeling and beyond!