Harnessing the Power of ChatGPT: Revolutionizing Content Recommendation for Broadcast Engineering
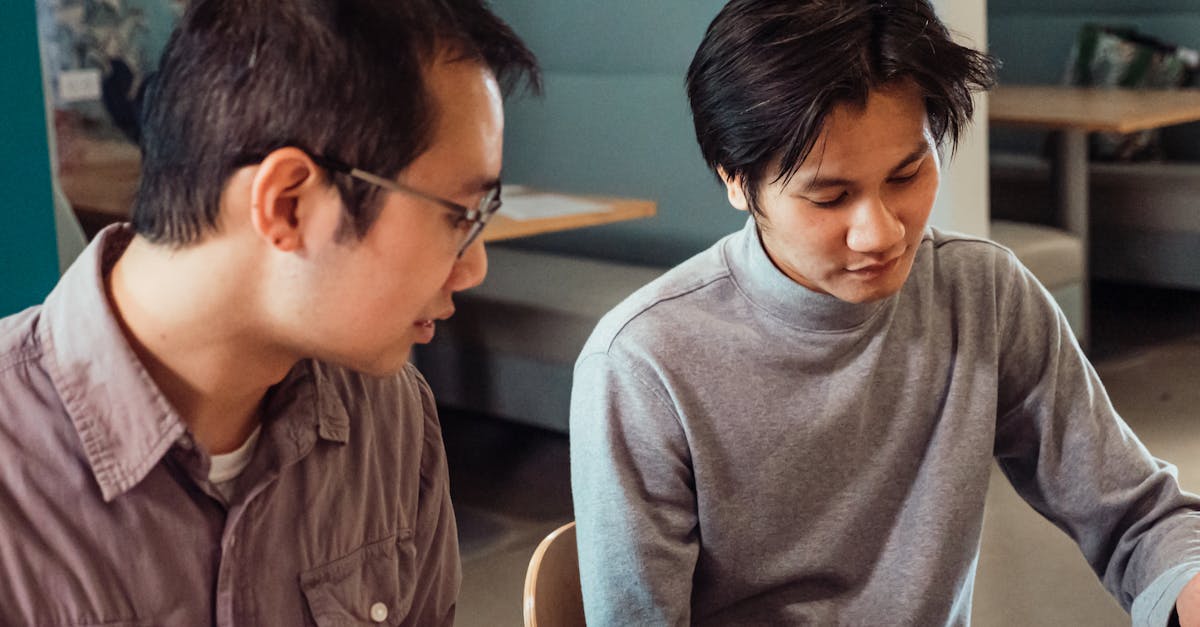
In today's digital age, the role of technology in the field of broadcast engineering has become increasingly important. One such technological innovation that has transformed the way viewers consume content is content recommendation. Content recommendation systems have revolutionized the broadcasting industry by providing personalized recommendations to viewers based on their past interactions and viewing habits.
Understanding Content Recommendation
Content recommendation is a technology-driven approach that leverages machine learning algorithms to analyze user behavior, preferences, and viewing patterns. By collecting and analyzing vast amounts of data, content recommendation systems can generate relevant recommendations that cater to the individual preferences of viewers.
The Role of Broadcast Engineering
Broadcast engineering plays a crucial role in the implementation and maintenance of content recommendation systems. These systems require advanced infrastructure, including high-performance servers, storage solutions, and network capabilities, to handle the enormous amounts of data processed in real-time.
Broadcast engineers are responsible for designing and implementing the technical architecture of content recommendation systems. This involves integrating various components, such as data collection mechanisms, machine learning algorithms, and user interface elements, into a cohesive system that can efficiently analyze and generate recommendations.
Benefits of Content Recommendation
Content recommendation systems offer numerous benefits to both broadcasters and viewers. For broadcasters, these systems enable better audience engagement and retention, leading to increased viewership and revenue. By understanding viewer preferences, broadcasters can optimize their content lineup and tailor their advertising strategies to target specific demographics.
Viewers also benefit from content recommendation systems as they are provided with personalized content suggestions that align with their interests. This enhances the overall viewing experience by reducing the time spent searching for relevant content and discovering new and exciting options that they may have otherwise missed.
How Does Content Recommendation Work?
The functioning of content recommendation systems can be broadly divided into two main stages: data collection and recommendation generation.
Data Collection
Content recommendation systems gather data from various sources, including viewer interactions with content, browsing history, demographics, and social media activity. This data is then consolidated and processed to create user profiles that capture individual preferences and viewing habits.
Recommendation Generation
Once user profiles are created, machine learning algorithms analyze the data to identify patterns, similarities, and relationships between viewers and content. These algorithms utilize techniques such as collaborative filtering, content-based filtering, and hybrid approaches to generate personalized content recommendations.
Challenges and Future Developments
While content recommendation systems have revolutionized the broadcasting industry, there are challenges that need to be addressed. Privacy concerns, data security, and the ethical use of user data are areas that require continuous attention and regulation.
Looking to the future, advancements in artificial intelligence and machine learning will further enhance content recommendation systems. Deep learning techniques, natural language processing, and real-time data analytics will lead to more accurate recommendations and a more personalized viewing experience.
Conclusion
Content recommendation systems have significantly transformed the way viewers discover and consume content in the broadcasting industry. With the help of broadcast engineering, personalized recommendations based on past interactions and viewing habits have become a key factor in creating a satisfying and engaging viewing experience. As technology continues to advance, content recommendation systems will only become more sophisticated, providing viewers with even better content suggestions tailored to their individual preferences.
Comments:
This article is really interesting! I never realized the potential of ChatGPT in revolutionizing content recommendation for broadcast engineering. It seems like it has the ability to greatly enhance user experience.
Emily, I'm curious about how ChatGPT can differentiate between different users within the same household. It would be challenging to personalize recommendations effectively in such cases.
I agree, Emily! The advancements in AI and natural language processing are truly remarkable. I can imagine how ChatGPT can personalize content recommendations for viewers, making their experience more enjoyable and engaging.
Samuel, I think ChatGPT can also help broadcasters better understand viewers' preferences, allowing them to create tailored content that matches their interests. It's a win-win situation!
Sarah, personalized content recommendations can also assist in reducing viewer churn. By suggesting relevant shows that align with each viewer's taste, broadcasters can increase viewer loyalty.
As a broadcast engineer, I'm excited to learn more about ChatGPT's potential applications in our industry. How can it be integrated effectively with existing systems?
Eliza, I believe that integrating ChatGPT into broadcast systems requires careful consideration of data security, ensuring that confidential information doesn't become accessible. It's crucial to have robust safeguards in place.
Eliza, ChatGPT can also be used to automate customer support, creating interactive chatbots that can handle user queries and provide relevant information, further enhancing user experience.
Thank you, Emily, Samuel, and Eliza, for your comments! ChatGPT can indeed revolutionize content recommendation. Eliza, integrating ChatGPT with existing systems can be done by leveraging APIs and customizing the recommendation algorithms to align with your broadcast requirements. It's a fascinating challenge!
This article is insightful! ChatGPT's potential in revolutionizing content recommendation can deliver highly personalized experiences to viewers. I can foresee this technology shaping the future of broadcast engineering.
Nathan, I completely agree! ChatGPT's capability to deliver highly personalized content recommendations can significantly improve viewer satisfaction and engagement, ultimately benefiting broadcasters too.
The possibilities with ChatGPT are fascinating! It can help broadcasters in discovering niche content, recommending related shows or documentaries to enhance viewers' engagement with the platform.
Olivia, I believe ChatGPT can also help to identify content gaps and optimize the overall catalog to cater better to viewers' preferences. It can be an invaluable tool for broadcasters in staying competitive.
I wonder if ChatGPT can be trained to understand the impact of cultural diversity on content preferences? It would be interesting to see how it handles personalized recommendations for viewers from diverse backgrounds.
Great observation, Brandon! ChatGPT's ability to learn from diverse datasets can certainly help in understanding the impact of cultural diversity on content preferences. It can adapt its recommendations according to viewers' specific interests, including those from diverse backgrounds.
I'm curious about the ethical considerations when using ChatGPT for content recommendations. How do we ensure that biased or inappropriate recommendations are avoided?
That's a valid concern, Grace. By meticulously curating and diversifying training data, as well as continually monitoring and evaluating the recommendation outputs, broadcasters can mitigate bias risks and ensure ethical practices.
I agree, Brandon. It could also involve allowing users to provide feedback on suggested content, helping to improve the accuracy and fairness of recommendations over time.
Brandon, I think implementing transparent explainability models alongside ChatGPT can also aid in identifying any potential bias in content recommendations.
Absolutely, Rachel! Transparency and explainability are essential to address concerns related to recommendation bias and ensure fair and inclusive content suggestions.
ChatGPT's potential to revolutionize content recommendation for broadcast engineering is exciting. However, I'm interested in knowing how it handles real-time data and dynamic content changes.
Adam, I believe ChatGPT would need a robust feedback loop and continuous learning mechanisms to handle real-time data updates effectively. It should be able to adapt to changing content and user preferences.
That's a good point, Sophie. Continuous retraining and updating of ChatGPT based on user feedback and evolving data can ensure its recommendations stay relevant and accurate.
Sophia, user feedback is indeed crucial for improving the accuracy of recommendations and fine-tuning the system to handle personalized suggestions within a household.
Absolutely, Brandon. Incorporating user feedback will help ChatGPT adapt and differentiate content recommendations effectively even among users in the same household.
I appreciate your insights, Brandon and Sophia. It's essential to ensure that content recommendations avoid perpetuating stereotypes and are representative of diverse perspectives.
Grace, I completely agree. Building fairness and diversity into recommendation models is critical to avoid any biased outcomes and provide content that caters to a wide range of viewers.
Brandon, it's worth mentioning that while ChatGPT can handle diverse backgrounds, continually monitoring and addressing bias concerns is crucial. Regular audits and adjustments to training data and recommendation algorithms help improve fairness over time.
I completely agree, Dan. Including personalization capabilities with careful consideration of ethical and fair practices ensures a positive user experience in content consumption.
That's a great point, Dan. Leveraging ChatGPT for automated customer support can improve response times and address common user queries efficiently.
I wonder if the integration of ChatGPT in broadcast engineering could potentially lead to reduced reliance on manual content curation and recommendation algorithms?
Jacob, that's an interesting thought! While ChatGPT can optimize and personalize content recommendations, it's crucial to have a balance between manual curation and the power of AI-driven algorithms. There will always be a need for human expertise in content selection.
Thank you, Dan! I agree that a human touch is important, ensuring a curated mix of content that aligns with the broadcaster's goals and values.
This article highlights the immense potential of ChatGPT in elevating content recommendation. It's fascinating to see how AI continues to reshape the broadcast industry.
Emma, the advancements in AI are truly remarkable. ChatGPT has the potential to be a game-changer in enhancing user experiences and driving engagement.
Samuel, I completely agree. With AI's ability to analyze vast amounts of data and personalize recommendations, viewers can discover new content that aligns with their interests.
Sophie, feedback loops can be valuable not only in personalizing content recommendations but also in identifying areas for improvement and enhancing the overall user experience.
Sophia, you raised a great point. Regular feedback loops enable broadcasters to refine and optimize the recommendations, keeping them aligned with viewers' evolving preferences.
I'm curious about the computational requirements for deploying ChatGPT in broadcast engineering. Does it demand significant computational power?
David, deploying ChatGPT does require computational resources, especially during training and inference phases. However, with efficient hardware and software optimizations, it's becoming more feasible to harness its power in real-world applications.
The potential benefits of ChatGPT in revolutionizing content recommendation for broadcast engineering are impressive. I'm excited to see how it evolves in the coming years.
I agree, Benjamin. The advancements in AI-driven recommendation systems like ChatGPT open up new possibilities for content personalization and viewer engagement.
This article provides valuable insights into the transformative potential of ChatGPT in the broadcast industry. The ability to deliver personalized recommendations can significantly enhance the viewing experience.
I'm impressed by the applications of ChatGPT in broadcast engineering. It has the potential to reshape how viewers discover and consume content, promoting a more interactive and tailored experience.
It's inspiring to see how AI technologies like ChatGPT are advancing the content recommendation landscape. Broadcast engineering is undoubtedly at the forefront of this revolution.
ChatGPT's potential in revolutionizing content recommendation could significantly impact viewer engagement and content monetization. I'm excited to see its implementation!
The future of broadcast engineering seems exciting with advancements like ChatGPT. I'm looking forward to enjoying more personalized and relevant content recommendations.
I can see ChatGPT playing a crucial role in content discovery, helping broadcasters widen their viewership by suggesting exciting and lesser-known shows to users.
ChatGPT could help broadcasters tackle information overload by curating personalized content recommendations that align with viewers' tastes, making it easier to discover relevant shows.
Thank you all for your insightful comments! It's encouraging to see the excitement for ChatGPT's potential in revolutionizing content recommendation for broadcast engineering. The future looks promising as we harness the power of AI to provide personalized experiences for viewers.