How ChatGPT Revolutionizes Data Analytics in Technology
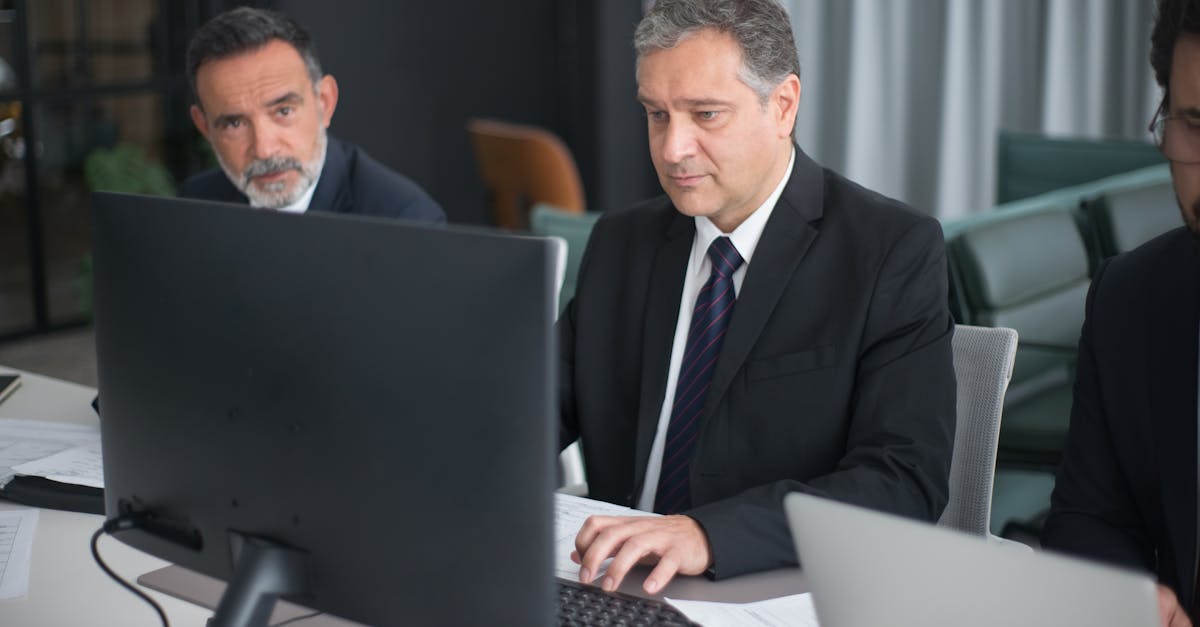
As the digital universe expands, businesses are confronted with vast volumes of data. Data analytics, the heart of digital transformation, has become crucial to drive informed decision making. One critical aspect of this process is 'data preprocessing,' which is about transforming raw data into a comprehensible format for analytical models. In this context, recent advancements in AI - specifically in models like ChatGPT-4 - have revolutionized the way data preprocessing is handled.
Technology: Data Analytics and its Importance
Data analytics refers to the process of analyzing raw data to draw helpful insights and patterns. Businesses use these insights to guide their strategic planning and maximize efficiency. It often involves inspecting, cleansing, transforming, and modeling data. However, dealing with massive amounts of raw, unprocessed data is one of the biggest challenges analytics experts face.
Area: Data Preprocessing and its Challenges
Before the data is ready for analysis, it must undergo initial steps of the data preprocessing. These include data cleaning, data integration, data transformation, and data reduction. Poor quality data, with inconsistencies, missing values, and outliers, can significantly compromise the analysis results and lead to erroneous decision-making. Time expenditure is another substantial constraint. Manual preprocessing of enormous datasets can be time-draining and often impractical.
Usage: ChatGPT-4 in Data Preprocessing
Enter AI models like GPT-4, which holds the capability to transform the way we handle data preprocessing. ChatGPT-4 can function as an automatic data preprocessor, flagging inconsistencies, identifying and filling missing values, and recognizing outliers in datasets.
Automatic Detection and Cleaning of Inconsistencies
Data inconsistencies occur often because of various factors like human error or misconfigured data input systems. Left unchecked, they can corrupt the entire data set and render the subsequent analytics useless. ChatGPT-4 can be brought in to aid with this problem. It can spot inconsistent data points and automatic correct or flag them for further review.
Handling Missing Values
Missing values present another common challenge in the data preprocessing phase. When a data instance lacks a particular attribute value, the missing data can interfere with the modeling process and weaken the reliability of the results. ChatGPT-4 can intelligently estimate and fill those missing values based on the patterns and trends in the rest of the data.
Dealing with Outliers
Outliers are data points that differ drastically from other observations. While some outliers can be critical concept-keepers, many are just errors that distort the model's understanding of the data. ChatGPT-4 is adept at recognizing outliers in the dataset and can automatically determine whether to correct, remove, or include these outliers.
Conclusion: The Future of Data Preprocessing
Streamlining the data preprocessing phase opens the door for faster, higher-quality data analytics. With AI models like ChatGPT-4 at the helm, businesses can navigate smoother seas of their data oceans. Not only will these AI-assisted processes alleviate the time constraints of manual preprocessing, but they'll also ensure the delivery of cleaner, more accurate data for insightful analytics. As we continue to develop and refine these AI models, we can look forward to even more robust and sophisticated data preprocessing capabilities.
Comments:
Thank you all for reading my article on how ChatGPT revolutionizes data analytics in technology. I would love to hear your thoughts and opinions!
Great article, Thuy! I completely agree that ChatGPT has the potential to revolutionize data analytics. It can analyze large datasets in a fraction of the time it would take for humans to do the same task.
Thank you, Michael! You're absolutely right, the speed and efficiency ChatGPT offers can greatly benefit data analysts and improve productivity.
While ChatGPT can be helpful, I think we should be cautious about relying solely on AI for data analytics. A balance between human analysis and automated tools is crucial to avoid potential biases or errors.
I appreciate your perspective, Sarah. You bring up a valid point about the importance of maintaining a human element in data analysis to prevent biases. Human oversight is indeed necessary to ensure accurate results.
This technology sounds promising, but do you think it can handle complex analytical tasks that require in-depth industry knowledge? Human analysts often possess domain expertise that can be vital in certain cases.
That's a great question, David. While ChatGPT can analyze data at scale, it may not possess the same level of industry expertise as human analysts. However, it can still provide valuable insights and assist analysts in making informed decisions.
I'm excited about the potential of ChatGPT in data analytics, but I wonder about the ethics surrounding the use of AI. How can we ensure data privacy and prevent misuse of personal information?
Ethical considerations are crucial, Emily. Protecting data privacy and ensuring responsible AI usage are paramount. It's important for organizations to establish strong security measures, comply with regulations, and prioritize transparency when using AI tools like ChatGPT.
I've used ChatGPT in my data analytics work, and it has been a game-changer for me. The ability to generate insights quickly and handle large volumes of data is incredible. However, there are still occasional inaccuracies that require manual correction.
Thank you for sharing your experience, Daniel! Indeed, while ChatGPT is powerful, it's crucial to review and verify its output to ensure its accuracy. Combining AI capabilities with human judgment can lead to more robust data analysis.
I'm curious about the scalability of ChatGPT. Can it handle real-time data streams and provide immediate insights for time-sensitive decision-making?
Scalability is a key aspect, Sophia. ChatGPT performs well with large datasets, but real-time data streams can pose a challenge. However, there are ongoing advancements in AI technology, and future iterations of ChatGPT may better address real-time analytics requirements.
Overall, I think ChatGPT holds great promise for data analytics. It can assist analysts in handling routine tasks, freeing them up for more complex analyses. However, it's important to continually evaluate its performance and refine its algorithms to maximize its potential.
Absolutely, Oliver! Continuous evaluation and refinement are necessary to optimize ChatGPT's abilities. By leveraging its strengths and addressing its limitations, we can harness the full potential of AI in data analytics.
I'm a bit concerned about the cost implications of relying on ChatGPT for data analytics. Will it be affordable for small businesses or only accessible to large corporations with significant budgets?
Valid point, Ryan. Affordability is an important aspect, and the accessibility and pricing models of AI tools like ChatGPT can impact their adoption. It's essential for developers and providers to consider cost-effective options to ensure broader accessibility across businesses of all sizes.
The potential of ChatGPT in data analytics is exciting, but what about its impact on job roles? Will it replace human analysts in the future, leading to job losses?
A valid concern, Jason. While ChatGPT can automate certain tasks, it's unlikely to replace human analysts entirely. Instead, it can augment their capabilities and enable them to focus on more strategic and creative aspects of data analysis. Adapting job roles to work alongside AI will be crucial.
The potential of ChatGPT to revolutionize data analytics is exciting but also raises questions about accountability and responsibility. Who should be held responsible if an automated analysis based on ChatGPT's output leads to incorrect decisions?
Indeed, Eva. Accountability is a significant consideration when using AI tools like ChatGPT. While developers hold responsibility for creating robust algorithms, it's vital for organizations and analysts to exercise due diligence and verify the accuracy of the output before making critical decisions.
I'm impressed by the potential of ChatGPT in data analytics, but what about its bias? How can we ensure that the AI is not reinforcing existing biases or introducing new ones?
Addressing bias is crucial, Grace. Developers need to implement rigorous testing and validation processes to minimize biases in algorithms. Regular monitoring and ongoing evaluation can help identify and rectify any biases that may arise during the data analytics process.
I believe ChatGPT can enhance data analytics, but what about Explainable AI? Can it provide insights into how it reached a particular conclusion?
Explainability is an important aspect, Liam. While ChatGPT doesn't provide detailed explanations by default, researchers are actively working on developing techniques to enhance explainable AI capabilities. Transparency and interpretability will be crucial as AI tools like ChatGPT are adopted in data analytics workflows.
What about the potential risks of AI-generated analytics being manipulated or tampered with? How can we ensure the integrity and security of the data analytics process?
Great question, Alexandra. Ensuring the integrity and security of AI-generated analytics is of utmost importance. Implementing robust security measures, such as data encryption, secure data handling, and access controls, can help mitigate risks associated with data manipulation or tampering.
I'm interested to know if ChatGPT can handle different data types, such as unstructured text or multimedia. Can it analyze diverse data sources, or is it primarily suited for structured data?
Good question, Emma. While ChatGPT's capabilities with different data types are continually improving, it's currently most proficient with structured data. Analyzing unstructured text and multimedia is a more complex task, but advancements in natural language processing and computer vision may expand its capabilities in the future.
Building on Emma's question, what about non-English language support? Can ChatGPT analyze and provide insights for data in languages other than English?
Language support is an important consideration, Sophia. While ChatGPT's primary strength lies in English language processing, efforts are underway to extend its capabilities to other languages. Multilingual models are being developed to facilitate broader language support in data analytics.
I'm excited about the potential of ChatGPT, but what about data bias? How can we ensure that ChatGPT's training data is diverse and representative to avoid perpetuating biases?
Addressing data bias is essential, Chloe. Efforts should be made to source diverse and representative training data for ChatGPT to avoid perpetuating biases. Ongoing evaluation, feedback loops, and inclusive data collection practices can help improve the fairness and accuracy of AI algorithms.
I'm concerned about the potential loss of human intuition that AI could bring. Will relying heavily on ChatGPT and similar tools diminish the importance of human creativity and critical thinking in data analytics?
You raise an important point, Emily. While AI tools like ChatGPT can assist with data analytics, human creativity, critical thinking, and intuition remain invaluable. AI should enable analysts to focus on higher-order tasks, allowing them to leverage their expertise, insights, and excellent decision-making skills.
I'm curious about the training process for ChatGPT. How do you ensure it understands the nuances of specific industries and domains?
Training ChatGPT to understand the nuances of specific industries and domains requires a combination of large and diverse training datasets. By fine-tuning the model on relevant domain-specific data and incorporating user feedback, the AI can become more familiar with industry-specific language and concepts.
I'm glad to see the potential of ChatGPT in data analytics. However, we must consider potential ethical dilemmas. How can we ensure AI fosters trust, transparency, and fairness in data analysis?
You're right, Isabella. Trust, transparency, and fairness are essential in AI-powered data analysis. Open communication, clear documentation of models and processes, and involving diverse perspectives in the development and evaluation of AI systems can help foster trust and ensure accountability.
The potential of ChatGPT in data analytics is exciting, but what are its limitations? What types of tasks may not be suitable or effectively handled by AI?
Understanding the limitations of AI tools like ChatGPT is crucial, James. While it excels in processing large amounts of data and generating insights, tasks requiring contextual understanding, deep industry knowledge, or human interaction may be less suited for current AI capabilities.
I'm impressed with the potential of ChatGPT, but what about data quality? Can ChatGPT detect and address data quality issues, such as missing values or inconsistent formatting?
Data quality is essential, Hannah. While ChatGPT may not inherently detect data quality issues, it can be trained or combined with data preprocessing techniques to handle missing values, inconsistent formatting, or other data quality challenges. Ensuring clean and reliable data is a crucial step in the data analytics process.
ChatGPT seems promising, but how can we ensure that AI tools align with industry standards and regulations? Are there any regulatory challenges to overcome?
Regulatory challenges are indeed significant, Alex. Aligning AI tools like ChatGPT with industry standards and regulations requires understanding and complying with data protection, privacy, and security laws. Collaboration between developers, regulators, and industry stakeholders can help address these challenges and promote responsible AI usage.
I'm excited about the potential of ChatGPT in data analytics, but what about its reliability? How can we ensure consistent performance and accuracy across various datasets and scenarios?
Ensuring reliability is crucial, Sophia. Ongoing testing, evaluation, and feedback loops are necessary to identify potential biases, errors, or limitations in ChatGPT's performance. Continual improvement and refinement of algorithms based on user input and real-world usage can enhance its reliability in various datasets and scenarios.
I'm impressed by ChatGPT's potential applications in data analytics, but what are the training and computational resource requirements for utilizing it effectively?
Training and computational resource requirements can vary, Andrew. Training large language models like ChatGPT can be computationally intensive and resource-demanding. However, with cloud-based AI services and advancements in hardware, it's becoming more accessible for organizations to leverage these tools effectively.
I'm excited about the potential of ChatGPT in data analytics. Can it be used in real-time decision-making scenarios, or is it primarily suited for retrospective analysis?
While ChatGPT may have limitations in real-time decision-making due to its processing times, it can still be valuable in providing insights and recommendations for data-driven decisions. Its potential to assist in real-time scenarios may improve with future advancements and optimizations.
ChatGPT indeed has the potential to revolutionize data analytics, but what about the energy consumption associated with training and deploying large language models? Could it have significant environmental impacts?
Energy consumption is an important consideration, Mia. Training large language models like ChatGPT can indeed have significant environmental impacts. Exploring efficient computing methods, renewable energy use, and responsible resource management can help mitigate these environmental concerns associated with AI model development and deployment.
I wonder if ChatGPT can help identify data anomalies or outliers during the data exploration process. Can it assist analysts in spotting inconsistencies in large datasets effectively?
Spotting data anomalies and outliers is an area where ChatGPT's capabilities can be valuable, Lucas. While it may not be an out-of-the-box feature, it can be trained and utilized with anomaly detection techniques to assist analysts in identifying inconsistencies in large datasets.
I'm curious about ChatGPT's potential in natural language understanding and sentiment analysis. Can it effectively analyze text data for sentiment and emotional insights?
Analyzing text data for sentiment and emotional insights is within ChatGPT's capabilities, Aiden. Natural language understanding is a strength of this AI tool, and it can assist in sentiment analysis by analyzing textual data and providing insights into emotional nuances.
I'm interested to know how ChatGPT handles data privacy and what measures are in place to protect sensitive information during the data analytics process.
Data privacy is a critical concern, Leah. Protecting sensitive information during the data analytics process requires robust security measures, including proper data anonymization, access controls, and adherence to data protection regulations. Privacy-by-design principles should be incorporated to ensure data privacy throughout the data lifecycle.
ChatGPT's potential in data analytics is impressive, but what about the learning curve for analysts? How easily can they adapt to using AI tools like ChatGPT in their workflows?
The learning curve is an important consideration, Henry. While using AI tools like ChatGPT may require some initial familiarization, developers can focus on creating user-friendly interfaces and providing comprehensive documentation and tutorials. Continuous support and training can help analysts adapt to incorporating AI tools into their existing workflows.
I'm fascinated by the potential of ChatGPT, but what about its data requirements? How much training data is typically needed for it to perform effectively?
Training data requirements for ChatGPT depend on the desired performance and the complexity of the task, Sophie. While models trained on large-scale datasets tend to perform better, smaller models can still provide useful insights. Balancing training data size with computational resources is a crucial consideration when utilizing AI tools like ChatGPT.
While ChatGPT's potential in data analytics is exciting, have there been any notable challenges or limitations faced during its implementation in real-world scenarios?
Several challenges and limitations have been encountered during ChatGPT's implementation, Lily. These include potential biases in output, difficulty in handling ambiguous input, and the need for extensive monitoring and evaluation of results. Continued research and development aim to address these challenges to improve real-world applications.
ChatGPT's potential in data analytics is remarkable. Can it handle multi-modal data, such as analyzing images and text together to draw meaningful insights?
Analyzing multi-modal data, such as images and text together, is an area researchers are actively exploring, Jayden. While ChatGPT's primary strength lies in language processing, advancements in areas like computer vision and multimodal learning could enable it to handle such tasks in the future.
I'm impressed by ChatGPT's potential, but what about the explainability of its decision-making process? Can we understand how it arrives at specific insights or conclusions?
As of now, ChatGPT doesn't provide detailed explanations for its decision-making process by default, Olivia. However, research on explainable AI is progressing, and efforts are underway to develop techniques that enhance AI model interpretability. Ensuring transparency and explainability in AI-powered data analytics is essential for its broader adoption.
I'm interested to know if ChatGPT can assist in exploratory data analysis tasks, such as identifying patterns, correlations, or outliers in data. Can it provide valuable insights during the initial stages of data exploration?
ChatGPT can provide valuable insights during exploratory data analysis, Joshua. It can help identify patterns, correlations, and even suggest potential outliers based on the input data. Leveraging ChatGPT's capabilities in the initial stages of data exploration can aid analysts in gaining a deeper understanding of their datasets.
I'm excited about ChatGPT's potential in data analytics. Can it assist in automated report generation, extracting key insights from data and summarizing them in a coherent manner?
Automated report generation is an area where ChatGPT's potential can be leveraged, Emma. It can assist in extracting key insights from data and summarizing them in a coherent manner. This can save time for analysts and provide them with a foundation for further analysis and decision-making.
I'm curious about the computational resource requirements of utilizing ChatGPT in data analytics. Does it require high-end hardware or can it run effectively on standard computing devices?
The computational resource requirements for ChatGPT can vary, Ava. Training and running large language models may require high-end hardware or cloud-based services. However, smaller variants and model optimizations can enable effective utilization on standard computing devices, making it more accessible for a wider range of analysts.
I wonder about the potential collaboration between AI tools like ChatGPT and human analysts. How can they work together to enhance data analytics workflows?
Collaboration between AI tools like ChatGPT and human analysts is key, Ethan. While ChatGPT can assist with automated tasks, human analysts can provide domain expertise, critical thinking, and contextual understanding. Integrating both AI capabilities and human judgment can enhance the effectiveness and accuracy of data analytics workflows.
I'm excited about the potential of ChatGPT. Can it handle unstructured data, such as social media posts or customer reviews, to derive valuable insights?
Analyzing unstructured data, including social media posts and customer reviews, is within ChatGPT's capabilities, Mason. Its natural language processing abilities allow it to extract insights and sentiments from such data. Leveraging ChatGPT can help derive valuable insights from unstructured sources and inform decision-making processes.
I'm curious about the training process for ChatGPT. How is it trained on data analytics tasks? Does it require specialized training or rely on general language models?
ChatGPT can be trained on data analytics tasks through a combination of supervised fine-tuning and reinforcement learning, Evelyn. It can leverage existing pre-trained models and further refine them on specific data analytics objectives. Custom training approaches can help optimize the model's abilities for specialized tasks.
I'm impressed with the potential of ChatGPT in data analytics. Can it assist in natural language understanding tasks, such as entity recognition or sentiment analysis?
Absolutely, Benjamin. ChatGPT's natural language understanding capabilities make it well suited for tasks like entity recognition and sentiment analysis. By analyzing textual data, it can extract entities and sentiments to provide valuable insights for data analytics.
I'm excited about the potential of ChatGPT in data analytics, but I'm concerned about bias in the training data. How can we ensure it doesn't perpetuate existing biases?
Addressing bias in training data is crucial, Zoe. Data collection practices should focus on diversity and inclusivity to avoid bias perpetuation. Regular evaluation, feedback loops, and continuous improvement can help mitigate biases and ensure fairness in AI models like ChatGPT.
I'm curious about the impact of ChatGPT on the scalability and efficiency of data analytics. Can it handle larger datasets and accelerate the overall analysis process?
ChatGPT's potential in handling larger datasets and accelerating the analysis process is significant, Xavier. Its ability to process and analyze vast amounts of data efficiently can enhance scalability and productivity in data analytics workflows.
I'm glad to see the potential of ChatGPT in data analytics, but are there any notable risks associated with relying heavily on AI for decision-making? How can we mitigate these risks?
Risks associated with relying heavily on AI for decision-making include data biases, inaccuracies, and potential dependency. Mitigating these risks requires continuous human oversight, regular evaluation, and robust quality assurance processes. Striking the right balance between AI assistance and human judgment is crucial to ensure responsible decision-making.
I'm curious about the potential of ChatGPT in predictive analytics. Can it assist in forecasting future trends based on historical data?
ChatGPT's capabilities in analyzing historical data make it useful in predictive analytics, Leo. It can assist in identifying patterns and trends that can inform future forecasts. Combining its abilities with traditional predictive modeling techniques can enhance the accuracy and reliability of predictive analytics.
I'm fascinated by ChatGPT's potential to revolutionize data analytics. What advancements and developments can we expect in the future with this technology?
The future holds exciting possibilities, Nora. Advancements in AI research, natural language processing, and multimodal learning can enhance ChatGPT's capabilities. We can expect improved performance, better handling of complex tasks, and enhanced interoperability with other AI tools. The potential for ChatGPT to transform data analytics is vast and ever-evolving.
ChatGPT's potential in data analytics is impressive, but what are its limitations when it comes to real-time data analysis and decision-making?
Real-time data analysis and decision-making pose challenges for ChatGPT due to its processing times, Hugo. While it may not be well-suited for immediate, time-sensitive scenarios, it can still provide valuable insights for decision-making processes that occur shortly after data collection.
I'm interested to know if ChatGPT can assist in data interpretation tasks, such as explaining complex analytical results to non-technical stakeholders or clients.
Data interpretation is an area where ChatGPT's capabilities can be leveraged, Luis. It can assist in explaining complex analytical results to non-technical stakeholders or clients by providing insights and translating technical jargon into more accessible language.
I'm excited about the potential of ChatGPT in data analytics. Can it assist in anomaly detection to identify irregularities or potential errors in datasets?
Anomaly detection is an area where ChatGPT's capabilities can be utilized, Lucia. By analyzing data patterns and comparing them to normal behavior, it can help identify irregularities or potential errors in datasets, thereby assisting analysts in ensuring data quality and accuracy.
I'm impressed with ChatGPT's potential in data analytics. Can it assist in natural language generation tasks, such as automated report writing?
Natural language generation tasks, including automated report writing, are well within ChatGPT's capabilities, Eva. By leveraging its language processing abilities, it can assist in generating coherent and informative reports based on data analysis, saving time and effort for analysts.
Thank you all for reading my article on how ChatGPT revolutionizes data analytics in technology. I'm excited to hear your thoughts and answer any questions you may have!
Great article, Thuy! I completely agree that ChatGPT has the potential to revolutionize data analytics. It provides a powerful tool for businesses to extract valuable insights from their data in a conversational manner. I'm curious, have you personally used ChatGPT in your data analytics projects?
Thank you, Linda! Yes, I've had the opportunity to work with ChatGPT in some data analytics projects. It's been impressive how it can understand and process complex questions, leading to more efficient analysis and decision-making. I'll be glad to share more details if you're interested!
Interesting article, Thuy! However, I'm a bit skeptical about the accuracy and reliability of ChatGPT in the context of data analytics. How does it handle large datasets and maintain data privacy?
Valid concerns, Michael. ChatGPT does indeed have some limitations when it comes to very large datasets. While it can process substantial amounts of data, it may struggle to scale to extremely big datasets. As for data privacy, it's crucial to use secure methods when implementing ChatGPT to ensure sensitive information is protected. Best practice includes anonymizing data or using encryption techniques, among others.
I enjoyed reading your article, Thuy! ChatGPT has immense potential in data analytics. I can imagine it being a valuable addition to business intelligence tools, allowing users to explore data intuitively. Are there any specific use cases where ChatGPT has shown exceptional results?
Thank you, Emily! One use case where ChatGPT has shown exceptional results is in customer support analytics. By interacting with users and understanding their queries, it can generate real-time insights, identify patterns, and provide personalized recommendations. This helps businesses improve customer satisfaction and optimize support operations.
Interesting point, Thuy! I believe ChatGPT can also excel in financial data analysis. With its ability to understand conversational queries, it can help financial analysts explore complex datasets, identify trends, and make informed investment decisions. This could potentially save a lot of time and effort!
I agree with you, Richard! ChatGPT could be a game-changer in financial analytics. It can assist in tasks like risk analysis, portfolio optimization, and even provide natural language explanations for complex financial models. It empowers analysts to quickly gain insights and communicate findings effectively.
Great article, Thuy! I can see how ChatGPT would facilitate better collaboration between domain experts and data analysts. It bridges the gap by enabling non-technical stakeholders to ask questions in plain language and receive actionable insights. Have you observed any challenges in adoption or user acceptance of ChatGPT in this context?
Thank you, James! Adoption challenges are not uncommon when introducing new technologies. In the case of ChatGPT, some domain experts might initially find it challenging to trust the system's answers without thoroughly understanding the underlying algorithms. Ensuring transparency, providing explanations, and gradually building user confidence are essential to address these challenges.
Great article, Thuy! I'm particularly interested in the integration of ChatGPT with existing data analytics tools. How does it complement existing solutions like SQL queries or business intelligence platforms?
Thank you, Jessica! ChatGPT can be a valuable addition to existing data analytics tools. While SQL queries and business intelligence platforms are powerful, they often require technical expertise. ChatGPT, on the other hand, allows users to interact with data using natural language, reducing the barrier to entry for non-technical stakeholders and enabling more intuitive exploration.
That's a great point, Thuy! ChatGPT can act as a layer of abstraction on top of complex data analytics tools. It bridges the gap between technical and non-technical users, making data analysis more accessible and empowering decision-makers to tap into the insights hidden in their data.
Interesting article, Thuy! I'm curious about the potential impact of ChatGPT on data privacy and security. How can we ensure that sensitive information or intellectual property doesn't get compromised?
Valid concern, Alex. Data privacy and security are paramount. Implementing secure channels such as encrypted communication protocols, access controls, and anonymizing sensitive data can help mitigate risks. It's also essential to regularly update and monitor the system to address emerging vulnerabilities and potential threats.
Great insights, Thuy! In addition to data analytics, do you think ChatGPT could enhance other areas of technology, such as natural language understanding or virtual assistants?
Absolutely, Sarah! ChatGPT's underlying technology has broader applications beyond data analytics. It can indeed enhance natural language understanding, virtual assistants, and even contribute to accessible human-computer interfaces. The ability to generate human-like responses makes it valuable in various contexts.
Thanks for sharing your insights, Thuy! While ChatGPT sounds promising, what are its limitations when it comes to analyzing unstructured or semi-structured data?
You're welcome, Jonathan! ChatGPT's main limitation with unstructured or semi-structured data is that it's primarily trained on text-based data. It may struggle to extract insights from other formats like images, audio, or complex tables. However, text-based data analysis remains its strong suit and it can still provide valuable insights within that domain.
Very informative article, Thuy! How does ChatGPT handle ambiguous or context-dependent queries? Does it seek clarifications or make assumptions?
Thank you, David! ChatGPT tries its best to handle ambiguous queries, but it can sometimes struggle with context-dependent questions. In cases where clarifications are needed, it might either seek additional information or make assumptions based on the available context. However, it's crucial to note that these assumptions might not always be accurate, and that's where human review and moderation come into play.
Fascinating insights, Thuy! How do you envision the future of ChatGPT in the field of data analytics? Are there any upcoming advancements or areas for improvement?
Thank you, Michelle! The future of ChatGPT in data analytics looks promising. Advancements could include better handling of large datasets, improved support for different data formats, and more reliable context awareness. Additionally, addressing biases and ensuring ethical use of the technology will be crucial for widespread adoption. Continuous research and community involvement will drive further improvements.
Great article, Thuy! ChatGPT certainly opens up exciting possibilities. One concern that often arises with AI-driven analytics is interpretability. How can we ensure that the insights generated by ChatGPT are explainable and transparent?
Thank you, Brian! You raise an essential point. Ensuring explainability and transparency of AI-driven analytics is crucial for building trust. Techniques such as generating explanations for model predictions, visualizing intermediate steps, and providing access to relevant data sources can contribute to more interpretable insights. Striking the right balance between accuracy and interpretability is an ongoing challenge.
Interesting article, Thuy! How does ChatGPT handle non-English languages? Can it assist in analyzing multilingual datasets?
Thank you, Karen! ChatGPT has limited support for non-English languages at the moment. While it can handle some other languages, its performance might not be as strong as with English. Analyzing multilingual datasets can still be feasible, but it's important to consider and evaluate the specific language requirements and performance of the model for each particular use case.
Great article, Thuy! How does ChatGPT handle complex statistical analysis and advanced mathematical modeling commonly used in data analytics?
Thank you, Robert! ChatGPT is not specifically designed for complex statistical analysis or advanced mathematical modeling. While it can assist in some related tasks, its primary strength lies in understanding natural language queries and providing insights based on existing data. For complex statistical analysis, traditional statistical tools and software are still essential.
Great insights, Thuy! Considering the continuous advancements in AI, how do you think ChatGPT will evolve to address future challenges and cater to new demands in data analytics?
Thank you, Daniel! The evolution of ChatGPT will be driven by ongoing research and community involvement. This includes addressing current limitations, such as scalability and interpretability, improving performance on various data formats, exploring better context understanding, and actively addressing biases and ethical considerations. Collaborative efforts will shape its future to meet the evolving demands in data analytics.
Thank you for the informative article, Thuy! Apart from data analytics, what other domains or industries can benefit from the capabilities of ChatGPT?
You're welcome, Sophia! ChatGPT can have an impact in various domains and industries. Some examples include legal services, healthcare, customer support, education, and research. It can help professionals in these areas with tasks like answering legal queries, diagnosing diseases, providing personalized recommendations, assisting students, and aiding in research analysis. The potential applications are vast!
Great article, Thuy! How does ChatGPT handle noisy or incomplete datasets? Can it still provide meaningful insights in such scenarios?
Thank you, Olivia! ChatGPT can handle noisy or incomplete datasets to some extent. Its ability to understand natural language queries allows users to ask questions even with imperfect or incomplete data. While it may not always provide accurate insights in such scenarios, it can still help in exploring the available information and providing contextual suggestions.
I enjoyed reading your article, Thuy! How does the training process of ChatGPT influence the accuracy and performance of data analytics queries? Does the training data affect the results significantly?
Thank you, Ryan! The training process of ChatGPT is critical in determining its accuracy and performance. The model is trained on diverse datasets, including internet text, to develop a broad understanding of language. While the training data greatly influences the results, it's important to note that fine-tuning the model on specific data relevant to data analytics queries is necessary to achieve optimal accuracy and performance in that domain.
Very insightful article, Thuy! What are the potential challenges organizations might face while integrating ChatGPT with their existing data analytics infrastructure?
Thank you, Jennifer! Integration challenges can arise when organizations try to incorporate ChatGPT into their existing data analytics infrastructure. Some potential challenges include data compatibility issues, adapting to the conversational nature of ChatGPT, ensuring secure data access and privacy, and addressing any skill gaps for successful adoption. Careful planning, thorough evaluation, and proper implementation strategies can help overcome these challenges.
Great article, Thuy! How does ChatGPT handle domain-specific terminologies and jargon commonly used in data analytics?
Thank you, Patrick! ChatGPT can understand and respond to domain-specific terminologies and jargon to some extent. However, its understanding might be limited by the training data provided. Fine-tuning the model using data specific to the data analytics domain, including relevant terminologies and jargon, can enhance its performance and ensure better alignment with industry-specific requirements.
Thanks for the informative article, Thuy! How does ChatGPT handle questions that require real-time data analysis or access to live data sources?
You're welcome, Laura! ChatGPT can handle real-time data analysis or access to live data sources by integrating with appropriate data retrieval and processing systems. Depending on the specific requirements and infrastructure, the system can be designed to fetch live data during interactions with the users, allowing ChatGPT to provide up-to-date insights and responses using the latest available information.
Very interesting read, Thuy! How can organizations ensure continuous improvement and accuracy of ChatGPT's analytics capabilities over time?
Thank you, Stephen! Continuous improvement and accuracy of ChatGPT's analytics capabilities can be ensured through regular updates and iterations. Incorporating user feedback, monitoring system performance, and leveraging emerging research and advancements in the field of natural language processing are crucial. Organizations should establish mechanisms for ongoing maintenance, evaluation, and retraining of the model to keep pace with evolving data analytics demands.
Great insights, Thuy! How can organizations address potential bias in ChatGPT's data analytics outputs?
Thank you, Natalie! Addressing potential bias in ChatGPT's data analytics outputs is crucial to ensure fairness and accuracy. Organizations can actively evaluate the model's performance across different demographic groups, continuously monitor and mitigate biases, and incorporate fairness techniques during the fine-tuning process. Transparency, diverse training data, and involving multidisciplinary teams can contribute to reducing bias and increasing inclusivity.
Great article, Thuy! How can organizations ensure the responsible and ethical use of ChatGPT in their data analytics practices?
Thank you, George! Ensuring responsible and ethical use of ChatGPT in data analytics practices requires establishing clear guidelines and policies. Organizations should define appropriate boundaries for system usage, address potential biases and fairness concerns, respect privacy and data protection regulations, and prioritize transparency. Regular audits, ongoing monitoring, and involving experts in AI ethics can further contribute to responsible deployment and usage.