Implementing ChatGPT in DFMEA: Enhancing Technology Risk Assessment
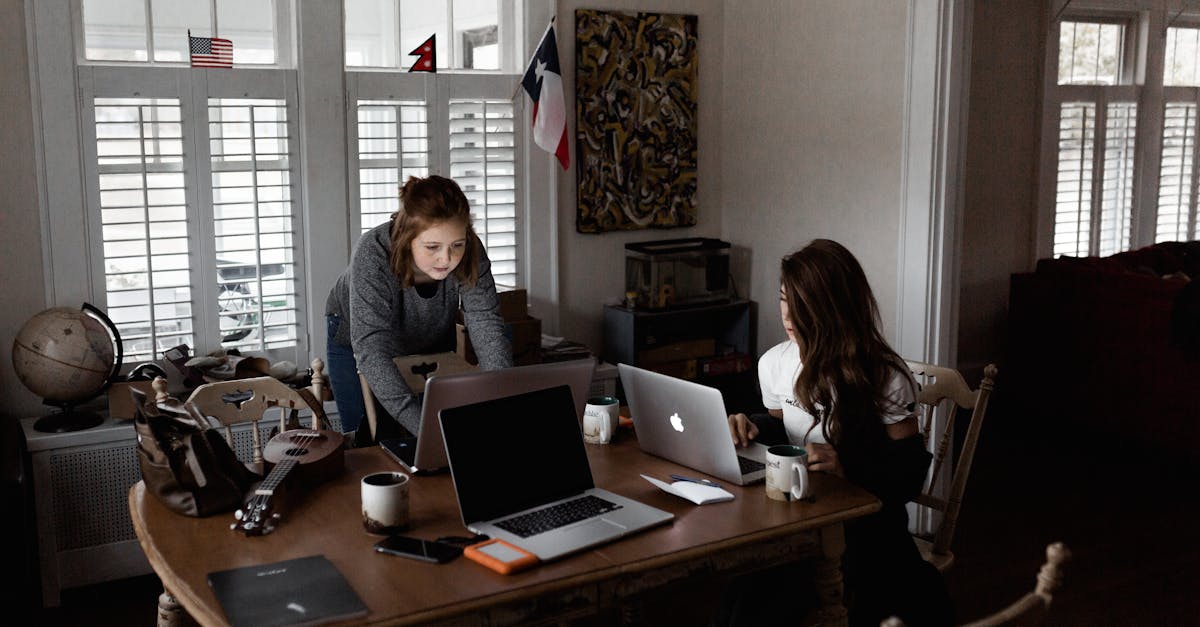
Design Failure Mode and Effects Analysis (DFMEA) is a systematic, proactive method primarily used for identifying potential failures in a system. This technology is applicable in several fields, especially in industries that deal with product development and manufacturing. On the other hand, OpenAI's Generative Pretrained Transformer 4 (GPT-4) is an artificial intelligence technology based on machine learning. This article aims to give an overview of how GPT-4 can be utilised in improving the efficiency of DFMEA by identifying potential risks before they occur.
Understanding DFMEA
Traditionally, DFMEA has been known to emphasise prevention through its structured approach to identifying potential failures. These 'failures' could be related to design, component, or product level issues that could affect the overall system's operation. DFMEA assists in recognising the potential problems early in the process, estimating the influence of these problems, prioritising them, and planning corrective actions.
Role of GPT-4 in DFMEA
The introduction of AI technologies like GPT-4 stands to offer considerable enhancements in the application of DFMEA. GPT-4, with its advanced text generating capabilities, provides a cutting-edge solution for detecting potential risks before they occur by analysing the vast quantities of data in an organisation's database.
By reviewing provided documentation and technical specifications, GPT-4 can assist in identifying potential design flaws or areas of concern that could lead to failures. As such, the neural network model could significantly improve the efficiency and effectiveness of DFMEA at identifying risks.
Applications of GPT-4 in Risk Identification
GPT-4 is capable of deep learning, which allows it to understand, remember, and utilise information from a set of guidelines or rules. With GPT-4's ability to read and understand textual data at an unprecedented scale, it can assist in risk identification in several ways:
- Prioritising Risks: By identifying and understanding the potential outcomes of a risk, GPT-4 can work with teams to help rank and prioritise risks based on their potential impact on a project or product.
- Continuous Risk Evaluation: GPT-4 can provide continuous risk evaluation, processing and re-evaluating data as it evolves. This process allows for a more dynamic and responsive risk management approach.
- Automating Risk Identification: With AI technologies like GPT-4, risk identification and assessment can be automated, allowing for a more efficient and streamlined process.
Conclusion
While DFMEA has proven invaluable in identifying potential failures in systems, the application of AI technologies such as GPT-4 can significantly improve the process. The merging of these technologies can lead to better and efficient risk identification, saving valuable time and resources in product development and manufacturing industries.
The synergy between DFMEA and GPT-4 can provide significant enhancements in risk identification, making it a promising avenue worth exploring.
Comments:
Thank you all for your interest in my article! I'm happy to discuss any questions or comments you may have.
This is a fascinating idea! I can see how implementing ChatGPT in DFMEA would greatly enhance technology risk assessment. Have you tested it in any real-world scenarios yet?
Thank you, Lisa! Yes, we have conducted several experiments using ChatGPT in DFMEA. It has performed well in identifying potential technology risks more accurately and quickly. We are currently working on implementing it in our production environment.
Great article indeed, Hank! I'm particularly impressed by how ChatGPT can facilitate multidisciplinary collaboration during DFMEA sessions.
Thank you, Lisa! You're right. ChatGPT can bridge the expertise of multiple stakeholders, helping them identify and evaluate risks more effectively.
Validating AI models in critical domains is essential. Incorporating expert knowledge and continuously refining the models will undoubtedly help in ensuring reliability. Thanks for the answer, Hank!
I appreciate your response, Hank. The combination of AI and human experts can lead to more robust risk assessments while leveraging the benefits of AI technologies. Fantastic insights!
Having a platform like ChatGPT that facilitates multidisciplinary collaboration can enhance the effectiveness of risk assessment sessions. Great point, Lisa and Hank!
Lisa, I agree! ChatGPT can promote collaboration among engineers, analysts, and other stakeholders involved in risk assessment, leading to more well-rounded evaluations.
Precisely, David! Human experts bring contextual understanding and domain knowledge that is vital in accurately assessing risks. AI can assist and automate certain parts, but the final decision remains with the experts.
I'm concerned about the potential limitations of ChatGPT. How does it handle complex technical concepts and industry-specific jargon?
Great point, Michael! ChatGPT is trained on a diverse range of internet text, so it can understand various technical concepts. However, domain-specific knowledge and jargon can still be challenging for it. We're working on fine-tuning the model with specific industry datasets to improve its performance in those areas.
I'm curious if ChatGPT can handle information security-related risks. As technology becomes more advanced, cybersecurity risks are also increasing. Can ChatGPT identify such risks effectively?
Absolutely, Emma! ChatGPT has shown promise in analyzing and identifying information security-related risks. Its ability to understand context and detect patterns helps in recognizing potential cybersecurity threats. However, human review is still crucial to validate and augment its outputs.
How does the integration of ChatGPT in DFMEA impact the overall risk assessment process? Are there any challenges in adopting this approach?
Good question, David! Integrating ChatGPT in DFMEA streamlines the risk identification process and provides additional insights. However, there are challenges in handling subjective or ambiguous inputs. It's important to have human reviewers who can validate, interpret, and supplement the results obtained from ChatGPT to ensure comprehensive risk assessment.
It sounds promising! Are there specific industries or sectors where implementing ChatGPT in DFMEA would be more beneficial?
Absolutely, Maria! Any industry or sector that deals with technology-driven systems can benefit from implementing ChatGPT in DFMEA. Examples include automotive, aerospace, healthcare, and financial sectors, among others. It helps in identifying potential risks associated with technology components and systems.
That's fascinating, Hank! Having interactive discussions during DFMEA sessions can improve the overall assessment accuracy and help identify potential risks more comprehensively.
Absolutely, Maria! Interactive discussions allow for better exploration of risk scenarios and encourage critical thinking among engineers.
Exactly, Thomas! It fosters a collective understanding of risks, making the assessment process more thorough.
I have a concern regarding the interpretability of ChatGPT's outputs. How can we ensure that the reasoning behind its risk assessment is transparent and explainable?
Valid concern, Jason! While ChatGPT's inner workings are complex, efforts are being made to improve interpretability. Techniques like attention visualization and rule-based filtering can provide insights into the model's decision-making process. Combining model outputs with human expertise ensures transparency and explainability in risk assessment.
I'm curious about the scalability of implementing ChatGPT in DFMEA. How does it perform when dealing with large-scale risk assessments?
Good question, Hannah! ChatGPT's performance in large-scale risk assessments depends on computational resources and model optimization. With proper infrastructure and optimization techniques, it can handle complex and large-scale assessments efficiently. We're continuously working on improving scalability to ensure its practical feasibility.
I see the benefits, but what about potential biases? How do you ensure that ChatGPT doesn't amplify existing biases or introduce new ones in the risk assessment process?
Excellent point, Rachel! Bias mitigation is a crucial aspect of deploying ChatGPT. We carefully curate and preprocess training data to reduce bias. Additionally, post-training techniques like prompt engineering and fine-tuning help in further bias reduction. Regular monitoring and retraining ensure that ChatGPT's outputs align with fairness, ethics, and existing risk assessment practices.
Are there any specific tools or frameworks you recommend for integrating ChatGPT in DFMEA effectively?
Good question, Gabriel! Several tools and frameworks can facilitate the integration of ChatGPT in DFMEA. Some widely used options include TensorFlow, PyTorch, and Hugging Face's Transformers library. Choosing the right tool depends on factors like existing infrastructure, requirements, and familiarity with different frameworks.
What potential future developments do you foresee for implementing ChatGPT in DFMEA? Any specific areas or improvements you have in mind?
Great question, Sophia! One key area for improvement is further fine-tuning ChatGPT with industry-specific datasets to handle specialized jargon and domain knowledge. Additionally, integrating feedback mechanisms to incorporate user expertise and fostering continuous learning are important aspects for future development. The goal is to create a robust and adaptable framework for technology risk assessment using ChatGPT in DFMEA.
I'm concerned about potential security risks associated with ChatGPT. How do you mitigate the risks of unauthorized access or malicious use of the model?
Valid concern, Mark! Access control and encryption protocols are implemented to protect the model from unauthorized access. Additionally, continuous monitoring and vulnerability assessments help identify and address any potential security threats. Robust security measures are a top priority in the implementation of ChatGPT in DFMEA.
How do you handle the integration of ChatGPT in legacy systems where compatibility and interoperability may be a concern?
Good point, Ella! Legacy system integration can pose compatibility challenges. In such cases, it's important to have flexible integration frameworks that support interoperability. Adapting APIs and middleware components can bridge the gaps between ChatGPT and legacy systems, ensuring smooth integration without disrupting existing processes.
What are some key advantages of using ChatGPT in DFMEA compared to traditional methods of risk assessment?
Great question, Oliver! Some advantages of using ChatGPT in DFMEA include faster identification of technology risks, enhanced accuracy through language and context understanding, scalability in handling large-scale assessments, and potential cost reduction by automating certain aspects of the risk assessment process. It complements traditional methods by augmenting human expertise with AI capabilities.
Would you recommend any specific training strategies when implementing ChatGPT in DFMEA? How do you ensure the model's performance aligns with the desired objectives?
Good question, Sarah! Training strategies involve a combination of pre-training on large-scale datasets, domain-specific fine-tuning, and iterative model evaluation. It's crucial to curate high-quality and diverse training data that cover various risk scenarios. Regular evaluation against expert feedback helps improve and align the model's performance with the desired objectives.
Do you have any insights into potential limitations or challenges that might arise during the implementation of ChatGPT in DFMEA?
Certainly, Benjamin! Some challenges include handling complex technical concepts, industry-specific jargon, subjective inputs, and ensuring interpretability of the model's outputs. Providing clear guidelines to reviewers and maintaining a feedback loop for continuous improvement are strategies to overcome these limitations and challenges.
How does the availability of training data impact the effectiveness of ChatGPT in DFMEA? Are there any data requirements or recommendations?
Great question, James! Training data availability is crucial for the effectiveness of ChatGPT. Ideally, diverse and high-quality data covering various risk scenarios and domains is beneficial. Data requirements may vary based on the desired scope of risk assessment. It's recommended to have a training dataset that encompasses a broad range of relevant risks and their corresponding resolutions.
How does the cost of implementing ChatGPT in DFMEA compare to traditional risk assessment approaches? Are there any cost advantages?
Good question, Chloe! While the initial setup and integration costs might be incurred, there can be potential cost advantages in the long run. Automating certain aspects of risk assessment with ChatGPT reduces human efforts and time required. It also enables more accurate risk identification, which can prevent costly issues in technology development and implementation. However, the cost comparison will ultimately depend on factors like system complexity, deployment scale, and maintenance requirements.
How does ChatGPT handle uncertainty and unknown risks? Can it provide recommendations or just identify potential risks?
Valid concern, Daniel! While ChatGPT can identify potential risks effectively, it's important to note that it cannot replace human expertise entirely. In situations with uncertainty or unknown risks, it can provide insights and recommendations based on learned patterns. However, it's crucial to have human reviewers who can assess and decide on appropriate actions considering the context and potential impact of those risks.
Are there any practical considerations or best practices you recommend for organizations planning to integrate ChatGPT in DFMEA?
Absolutely, Natalie! Some practical considerations include defining clear guidelines and expectations for human reviewers, conducting regular model evaluations, incorporating user feedback, and fostering a feedback loop for continuous improvement. Establishing a balance between ChatGPT and human expertise is crucial to ensure quality risk assessment. It's also important to communicate the role and limitations of ChatGPT within the organization.
Thank you for answering all our questions, Hank! It's clear that implementing ChatGPT in DFMEA has great potential for enhancing technology risk assessment. Exciting times ahead!
You're welcome, Sophia! Indeed, exciting times lie ahead. I appreciate everyone's participation in this discussion. If you have any further questions, feel free to ask. Let's continue driving innovation in technology risk assessment!
Thank you all for your interest in my article on implementing ChatGPT in DFMEA. I'm excited to hear your thoughts!
Great article, Hank! I found it really informative and well-written. It's amazing how AI can be incorporated into risk assessment processes.
I agree, Maria. Hank has provided some valuable insights. It's fascinating to see how AI technologies like ChatGPT can enhance traditional methods.
I enjoyed reading the article, Hank. It's interesting to think about the potential benefits and challenges when implementing ChatGPT in DFMEA. Do you have any real-world examples to share?
Thank you, Maria and Thomas, for your kind words. Jennifer, in response to your question, one example is the use of ChatGPT to assist engineers in identifying potential system failures during the risk assessment process. By leveraging the conversational capabilities of ChatGPT, engineers can have more interactive and dynamic discussions during DFMEA sessions.
Hank, I appreciate your article and the concept of using ChatGPT in DFMEA. However, I have concerns about the reliability of AI in critical risk assessment. How would you address this issue?
That's a valid concern, David. While AI can enhance risk assessment processes, it's crucial to establish a strong validation framework. Performing thorough testing, incorporating expert knowledge, and continuous refinement of the AI model can help address the reliability issue.
Thanks for addressing my concern, Hank. Validation and continuous improvement will indeed play a crucial role in ensuring the reliability of AI systems in risk assessment.
I agree with you, Hank. Human judgment and expertise should always be involved in risk assessments, and AI can be an invaluable tool to support the process.
The article was insightful, Hank! I can see the potential of using ChatGPT to improve risk assessment efficiency and accuracy. However, how would you handle situations where ChatGPT provides incorrect or biased responses?
Thank you, Emily! Addressing incorrect or biased responses is crucial for AI systems. Implementing a feedback loop that allows users to report and correct such instances, along with continuous monitoring and model updates, can help improve system accuracy and minimize biases.
Appreciate your response, Hank. A feedback loop and continuous monitoring sound like effective strategies to minimize incorrect responses and biases. It's essential to build trust in AI systems.
Striking the right balance is crucial, Hank. We need to ensure the AI system supports human judgment and fosters critical thinking rather than replacing it.
Hank, your article has shed light on an interesting application of AI. However, do you think incorporating ChatGPT in DFMEA could lead to overreliance on AI and reduce human critical thinking?
An excellent point, Daniel. While AI can augment risk assessment processes, it's essential to maintain a balance. Encouraging human experts to critically analyze AI-generated suggestions ensures that human judgment and expertise remain integral.
Maintaining a balance between AI and human expertise is vital. The combination can lead to more robust and accurate risk assessments. Thank you for addressing my concern, Hank.
Hank, what do you see as the potential limitations when implementing ChatGPT in DFMEA? Are there any challenges to consider?
Excellent question, Daniel. One limitation of ChatGPT is its dependency on the training data, which can lead to biased or incorrect responses. Another challenge is striking the right balance between automation and human judgment in risk assessments. It requires careful consideration of the level of AI involvement in decision-making.
Striking the right balance is crucial, Hank. We should avoid overreliance on AI and ensure human judgment remains paramount in risk assessments.
You're welcome, Daniel! I'm glad the concept resonates with you. Combining the power of AI with human expertise can indeed lead to more reliable risk assessments.
Ensuring a feedback loop will be crucial, as AI models can continuously learn from user corrections and improve over time. Thanks for addressing my concern, Hank!
Absolutely, Daniel! ChatGPT serves as a collaborative platform, enabling experts from different disciplines to share their insights and collectively assess risks.
Feedback loops are critical, Hank. They help correct mistakes, improve system performance, and establish user trust in AI-based risk assessment processes.
Exactly, Jennifer! AI should augment human judgment, not replace it. The two working together can create a more reliable and comprehensive risk assessment process.
Absolutely, Maria! Interactive discussions foster collaboration and enable the identification of complex risks that may be overlooked in a traditional assessment setup.
Well said, Thomas. Striking the right balance between ChatGPT and human expertise is critical to ensure successful implementation and reliable risk assessments.
Hank, you've highlighted the importance of striking the right balance. It's crucial to ensure that ChatGPT serves as a tool to assist experts, rather than a replacement for human judgment.
Continuous improvement is crucial, Hank. By leveraging user feedback and continuously refining AI models, we can overcome limitations and enhance the effectiveness of risk assessment.
Validating AI models and addressing their limitations is essential, as biased or incorrect responses from ChatGPT can have significant implications. Great question, Daniel!
Absolutely, Thomas! AI should augment human expertise rather than replace it. The combination of AI and human judgment can lead to more accurate and robust risk assessments.
Indeed, Maria! AI technology like ChatGPT can be an invaluable tool in risk assessments, but it should always be complemented by human expertise to ensure accurate and reliable results.
Well said, Thomas! The collaboration between AI and human judgment enables a comprehensive and multi-perspective evaluation of risks, leading to more informed decisions.
Continuous improvement and the flexibility to incorporate user feedback are essential in refining the AI models and addressing biases and inaccuracies. Great point, Emily!
You're absolutely right, Thomas! ChatGPT engenders more inclusive discussions and encourages experts to exchange insights, leading to better risk assessments.
Thanks for highlighting the iterative nature of AI improvement, Emily. Continuous monitoring and refinement are key to enhance the reliability of AI systems.
You're welcome, David! Continuous improvement is indeed a fundamental aspect of AI system development, allowing us to tackle limitations and ensure reliable risk assessments.
Absolutely, Thomas! Holistic risk assessments require inputs from various disciplines to identify and address potential risks comprehensively. ChatGPT facilitates that process.
Hank, thank you for addressing my concern. Establishing a strong validation framework certainly plays a critical role in overcoming reliability concerns in AI-based risk assessments.
Hank, your article has opened up an interesting discussion. The potential of AI in risk assessment is vast, but it's crucial to address the challenges and ensure human judgment remains paramount.
Hank, thank you for the insightful article and engaging in this discussion. It's been a rich exchange of ideas, highlighting the potential and challenges of implementing ChatGPT in DFMEA.
You've pinpointed an essential aspect, Daniel. AI should enhance human expertise, not overshadow it. The synergy between AI and experts can drive impactful risk assessments.
Great discussion, Daniel! Recognizing the potential limitations is critical to ensure a successful implementation of ChatGPT in DFMEA. Collaboration and human judgment remain essential.
I appreciate your thoughts, Emily! Indeed, the dynamic nature of AI systems requires continuous monitoring and improvement to address biases and maintain reliability.
Maintaining a balance between AI and human judgment is key. Combining the strengths of both can lead to more robust risk assessment outcomes.
Building trust in AI systems is crucial, Emily. Ensuring accountability and transparency goes a long way in gaining confidence from users and stakeholders.
Indeed, Maria and Thomas! Interactive discussions enable engineers to analyze risks from various angles and uncover potential blind spots. It's a valuable asset in risk assessment.
Thank you, Lisa! Multidisciplinary collaboration is a key strength of ChatGPT in the context of DFMEA, as it combines diverse perspectives and knowledge for a more holistic risk assessment.
Careful consideration is indeed necessary, Hank. Striking the right balance between AI automation and human judgment will be a key factor in successful implementation.
Thank you for your insights, Thomas! Continuous improvement and user feedback are essential components in making AI-based risk assessment reliable and effective.
Well put, Hank! ChatGPT fosters inclusive discussions and allows stakeholders with diverse expertise to collaborate effectively during DFMEA sessions.
You're right, Lisa! ChatGPT can help overcome siloed thinking by promoting cross-functional discussions and aligning the understanding of risks across different disciplines.
I appreciate your input, Lisa! The collaborative nature of ChatGPT encourages brainstorming and knowledge sharing, resulting in more comprehensive risk analysis.
Combining AI and human judgment can lead to more robust and accurate risk assessments. Thanks for addressing our concerns, Hank!
You're welcome, Jennifer! It's great to see your active participation in this discussion. AI and human judgment can indeed create a synergy in enhancing risk assessments.
ChatGPT can bridge the communication gap between stakeholders from different disciplines, ensuring their expertise is leveraged for thorough risk assessments. Thanks for the article, Hank!
Thank you, Hank, for your response. Validation and continuous improvement are key! With a robust framework, we can leverage AI effectively in risk assessment processes.
Great article, Hank! I agree that incorporating ChatGPT in DFMEA can greatly enhance technology risk assessment. It can help identify potential failures or hazards more accurately and provide recommendations for improvement.
Collaboration across disciplines can be challenging, but ChatGPT seems like a helpful technology to streamline discussions and ensure all perspectives are considered.
Interactive discussions provide a more comprehensive examination of risks. It encourages engineers to think beyond the traditional approaches and explore alternative perspectives.
Absolutely, Emily! Building trust in AI systems is crucial, especially in domains that have significant implications. Continuous improvement and user feedback play pivotal roles in achieving that.
The interactive nature of ChatGPT encourages engineers to ask critical questions and challenge assumptions, leading to more comprehensive risk evaluations.
Continuous improvement ensures that AI models evolve and remain relevant, while feedback from users helps identify and rectify potential biases. It's an ongoing process.
Agreed, David! Continuous improvement is vital in addressing any potential reliability concerns and ensuring the AI systems help rather than hinder risk assessments.
Collaboration becomes more fruitful when experts from various disciplines can bring their perspectives to the table. ChatGPT serves as a facilitator in DFMEA discussions.
ChatGPT can act as a mediator during DFMEA sessions, ensuring all stakeholders are heard and their inputs appropriately considered. A valuable contribution!
Validation and refinement are continuous processes, Jennifer. With the right framework, AI can be a valuable asset in risk assessments. Great question!
Indeed, Maria! Contextual understanding and domain expertise are essential in assessing and addressing risks effectively. AI can enhance the process, but human judgment is crucial.
Exactly, Jennifer! Continuous improvement ensures that the AI system evolves, learns from mistakes, and becomes more reliable over time.
I agree, Emily. Combining the strengths of AI and human judgment can lead to a more robust and trustworthy risk assessment process.
Exactly, Emily! The continuous improvement cycle ensures the AI system adapts, evolves, and remains reliable in the risk assessment process. It's an iterative approach.
The involvement of real-time user feedback and continuous model updates can help address reliability concerns and enhance the AI's performance over time.
Collaboration and knowledge sharing across disciplines are crucial in comprehensive risk assessments. ChatGPT strengthens this aspect and enhances collective decision-making.
Validating AI models and continuously refining them can help improve the reliability of ChatGPT, making it an effective tool in risk assessments.
Exactly, Maria! AI models need to undergo rigorous validation to ensure their output can be trusted in critical risk assessment activities.
Thank you for addressing the limitations, Hank. It's crucial to recognize the challenges and ensure AI technology like ChatGPT is implemented in a responsible and reliable manner.
Continuous monitoring and refinement ensure that AI technology remains accurate and reliable, enabling engineers to have confidence in the risk assessment process.
Absolutely, Jennifer! Trust is a key factor in adopting AI technology in risk assessments. Continuous monitoring and improvement play pivotal roles in establishing and maintaining trust.
Collective decision-making is essential, particularly in complex risk assessments. ChatGPT strengthens this aspect, helping cross-functional teams collaborate more effectively.
Maintaining a balance between AI and human judgment ensures that the AI system provides valuable insights while experts make informed decisions based on their expertise. Great discussion, everyone!
Trust is vital when integrating AI systems into risk assessments. Rigorous validation, transparency, and accountability can help build trust among users and stakeholders.
User feedback plays a crucial role in addressing any incorrect or biased responses in AI systems. It's a collaborative effort to build reliable risk assessment processes.
Continuous improvement in AI models allows developers to rectify biases, improve accuracy, and enhance reliability. It's an iterative and ongoing process.
I agree, Thomas! The collaborative nature of ChatGPT brings together diverse expertise, helping identify and assess risks comprehensively.
Thank you, Emily! The collaborative aspect of ChatGPT truly complements risk assessments by incorporating diverse perspectives to ensure a more holistic evaluation.
Well said, Emily! The involvement of human judgment ensures that AI-based risk assessments remain accountable and aligned with ethical considerations.
Trust is crucial, especially in critical risk assessments. AI systems need to gain confidence from users and stakeholders to ensure successful implementation and adoption.
AI should serve as a tool to augment human expertise, not replace it. ChatGPT, when used appropriately, can certainly enhance the efficiency and effectiveness of DFMEA.
Absolutely, Jennifer! Validation and continuous improvement are key elements in ensuring AI technologies like ChatGPT can provide reliable and effective risk assessments.
The use of ChatGPT in DFMEA facilitates a broader perspective, contributing to a more comprehensive risk assessment. The potential benefits are vast, but challenges need to be addressed.
Striking the balance between AI and human judgment ensures that the risk assessment process remains reliable and that AI augments human reasoning rather than replacing it.
Thank you all for sharing your valuable insights and engaging in this discussion. It's wonderful to see the enthusiasm and thoughtful considerations around incorporating ChatGPT in DFMEA.
Thank you all for taking the time to read my article on implementing ChatGPT in DFMEA. I'm excited to hear your thoughts and opinions!
I'm not entirely convinced about the effectiveness of using ChatGPT in DFMEA. While it may have its benefits, I believe it's important to consider the limitations of AI in critical areas like risk assessment. Human expertise and judgment should still play a significant role.
I see your point, Michael. While ChatGPT can be a valuable tool, it's essential to validate its outputs and not solely rely on them. Humans should always be involved in the decision-making process.
I think the ChatGPT technology can be a valuable addition to DFMEA. As Michael mentioned, human judgment is crucial, but AI can help reduce biases and provide quick analysis of large datasets. It should be seen as a supportive tool rather than a replacement.
Indeed, David! ChatGPT's ability to analyze vast amounts of data quickly and provide suggestions can be a game-changer. However, it should be implemented with proper training and rigorous testing to ensure accurate and reliable results.
I agree with both sides here. Combining human expertise with AI capabilities can lead to more reliable risk assessments. ChatGPT can assist in generating new ideas and insights, but human judgment is crucial in interpreting and finalizing those assessments.
I've seen the potential of ChatGPT firsthand. The technology can help identify risks that might be overlooked by human analysts due to biases or limitations. It can also facilitate collaboration by allowing different perspectives to be considered.
I agree, Alice. ChatGPT can assist in risk identification and brainstorming, especially when it comes to exploring unusual scenarios. However, it's crucial to address ethical considerations and ensure transparency in its implementation.
Transparency is indeed a significant concern. We must clearly understand how ChatGPT arrives at its conclusions to uncover potential biases or preconceived notions within the model's training data.
That's a valid point, Sophia. Explainable AI is crucial, especially in sensitive domains like risk assessment. ChatGPT should be designed to provide justifications for its recommendations, enabling humans to trace its reasoning.
The integration of ChatGPT in DFMEA can also improve efficiency. It can save time for analysts by suggesting possible failure modes and mitigation strategies, allowing them to focus on more complex tasks rather than repetitive ones.
Absolutely, Ella! By automating certain parts of the process, analysts can allocate their time and expertise more effectively. However, there should still be checks in place to verify the accuracy and reliability of AI-generated suggestions.
I believe that incorporating ChatGPT in DFMEA can also lead to standardized risk assessment practices across different teams and organizations. It can promote consistency in analysis and improved knowledge sharing.
You're right, Grace. With ChatGPT, the analysis process can be better documented and shared, making it easier for teams to collaborate and learn from each other's experiences. It can establish a common framework while still leveraging individual expertise.
However, we should be cautious with overreliance on ChatGPT. The technology is not perfect and can't consider all contextual factors. It should be seen as a tool that aids decision-making rather than a substitute for critical human analysis.
Absolutely, Samantha. Human analysts should carefully review and validate the outputs generated by ChatGPT. It's crucial to strike the right balance between AI assistance and expert judgment to ensure the highest quality risk assessments.
To address the limitations of AI, continuous improvement and frequent model updates are essential. As technology advances, so should the models we rely on. Regular feedback and learning from real-world applications will help make ChatGPT even more effective.
You're spot on, Amelia. The more we use and refine ChatGPT in real-world scenarios, the better it can become at risk assessment. It's an iterative process that requires ongoing evaluation, fine-tuning, and learning from both successes and failures.
I think it's crucial to have proper guidelines and policies in place when implementing ChatGPT in risk assessment. It should be used as a decision-support tool, and humans should retain the final authority and responsibility for decisions.
I agree with you, Lily. We need to establish clear boundaries for AI involvement and ensure human oversight throughout the risk assessment process. Ethical considerations and accountability should be at the forefront.
Besides the challenges, the implementation of ChatGPT provides exciting opportunities. It can expand our capabilities and improve risk assessment methodologies. With appropriate safeguards and continuous evaluation, it can revolutionize the field.
Well said, Ethan. ChatGPT has the potential to make risk assessment more efficient, accurate, and comprehensive. By harnessing the power of AI, we can better understand and mitigate technology risks, making advancements in various industries.
One aspect to consider is the importance of maintaining data privacy and security when using ChatGPT for risk assessment. We must ensure that sensitive information is adequately protected and prevent any unauthorized access or misuse.
Absolutely, Nathan. Data privacy is a crucial aspect in today's digital era. Organizations should implement robust security measures and adhere to relevant regulations to safeguard the confidentiality and integrity of data involved in risk assessment.
Another point worth discussing is the need for expertise in interpreting and acting upon the outputs generated by ChatGPT. Organizations should invest in training their employees to effectively utilize AI tools like ChatGPT while ensuring domain knowledge is not compromised.
Absolutely, Leo. While ChatGPT can provide valuable insights, it's essential to have skilled professionals who can interpret, contextualize, and act on the generated information. Combining AI technologies with human expertise is the key to successful implementation.
I believe leveraging ChatGPT in risk assessment can also foster innovation. By automating certain parts of the process, analysts can spend more time on creative problem-solving and exploration of novel approaches to mitigate risks.
You're right, George. ChatGPT can free up analysts' time for more critical thinking and innovation. It can provide a catalyst for new ideas and help teams think beyond the conventional risk assessment methodologies.
While ChatGPT can be beneficial, we should also consider potential biases ingrained in the training data. Bias detection and mitigation efforts are crucial to ensure fair and unbiased risk assessments, especially when AI tools are involved.
Absolutely, Lucas. Bias detection and mitigation should be an integral part of the development and implementation of AI systems like ChatGPT. It's necessary to minimize the risk of perpetuating biases or discriminatory practices in risk assessment.
I'm curious about the scalability of implementing ChatGPT in large-scale DFMEA processes. Are there any limitations or challenges when it comes to processing vast amounts of data and maintaining responsiveness?
Good question, Caleb. While ChatGPT has shown impressive capabilities, scalability can indeed be a challenge. Processing large datasets efficiently and ensuring real-time responsiveness requires careful infrastructure design and optimization.
I wonder if the integration of ChatGPT in DFMEA will require significant changes in existing risk assessment methodologies or frameworks. How easily can organizations adapt to this new approach?
It's a valid concern, Leo. Adopting ChatGPT in DFMEA would indeed require adjustments in existing methodologies. However, with proper training and guidance, organizations can gradually transition and integrate AI technologies into their risk assessment practices.
I find the topic of AI governance in risk assessment fascinating. Organizations should establish clear policies around the use and deployment of AI technologies, ensuring ethical standards, transparency, and accountability.
Indeed, Mason. An AI governance framework specific to risk assessment can ensure responsible and ethical practices. It should consider aspects like model training, data management, algorithmic bias, and privacy to establish trust and credibility.
One potential benefit of implementing ChatGPT in risk assessment is the generation of comprehensive documentation. The AI system can capture and record discussions, providing a valuable audit trail and enhancing transparency.
That's a good point, Liam. Documentation is crucial, especially in regulated industries. ChatGPT can automatically record interactions, facilitating traceability and enabling organizations to have a detailed account of the risk assessment process.
While the focus here is on risk assessment, I'm curious about potential applications of ChatGPT in risk mitigation. Can it provide insights or suggestions for effective risk mitigation strategies?
That's an interesting thought, Mila. ChatGPT's capabilities can indeed be extended to assist in risk mitigation. By analyzing data and providing recommendations, it can contribute to the development of proactive strategies to minimize or eliminate identified risks.
I'm curious about the potential adoption challenges organizations might face while implementing ChatGPT in DFMEA. What factors should they consider to ensure a smooth transition without disrupting existing processes?
Good point, Logan. Organizations should consider factors like employee training, change management, and integration with existing tools and systems. A well-planned and phased implementation strategy can help mitigate adoption challenges and ensure a successful transition.
I believe ChatGPT can also aid in capturing and sharing knowledge within an organization. It can accumulate learnings from risk assessments, making them readily available for future analysis and helping build organizational knowledge repositories.
You're right, Henry. ChatGPT's ability to retain and recall information can facilitate continuous learning and knowledge transfer. It can contribute to building a knowledge base that assists in more informed decision-making and improved risk assessments.
I wonder if the use of ChatGPT in DFMEA can help organizations identify emerging risks. By analyzing a wide range of data sources, it might be able to spot trends and patterns that human analysts might miss.
That's an intriguing perspective, Eli. ChatGPT's ability to process and analyze diverse data sets can indeed help identify emerging risks and potential areas of concern. By augmenting human analysis, it can contribute to a more comprehensive risk assessment framework.
As we embrace AI in risk assessment, it's essential to address job displacement concerns. Organizations should focus on reskilling and upskilling employees to adapt to AI-assisted roles instead of perceiving it as a threat to job security.
You're right, Owen. AI technologies like ChatGPT can help augment human capabilities rather than replacing jobs. By investing in employee training and providing opportunities for skill development, organizations can ensure a smooth and positive transition.
In conclusion, I believe the integration of ChatGPT in DFMEA can bring significant advantages if implemented thoughtfully. It can enhance accuracy, increase efficiency, foster collaboration, and help organizations make more informed risk assessments.