Improving Credit Risk Assessment: Leveraging ChatGPT in Savings Technology
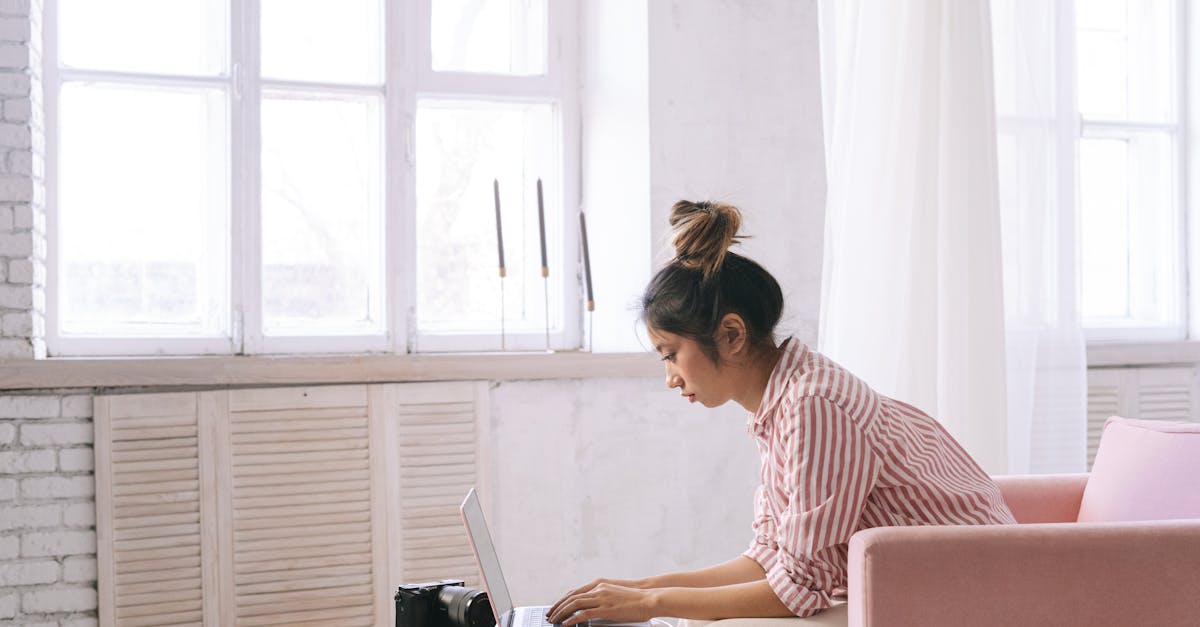
Credit risk assessment plays a vital role in the financial industry. Companies rely on accurate credit risk predictions to make informed decisions when extending credit to customers. With advancements in savings technology, a new model has emerged that allows companies to analyze customer financial histories and predict their credit risks effectively.
Savings technology, which combines financial savings data with advanced algorithms, offers immense potential for credit risk assessment. By leveraging the vast dataset of customer financial histories, this technology can identify patterns, trends, and risk factors that might act as indicators of future creditworthiness or default.
The savings technology model employs machine learning algorithms to analyze historical financial data collected from customers. It identifies various financial parameters that are highly correlated with credit risks, such as income stability, debt-to-income ratio, payment history, and outstanding debts. By considering these factors in aggregate, the model generates a credit risk score for each customer.
The fine-tuning process of the model involves training it on a comprehensive dataset, which includes both positive and negative credit outcomes. It learns to detect subtle patterns and relationships among different financial variables to make accurate predictions. Through iterative refinements, the model becomes more reliable and capable of delivering robust credit risk assessments.
The predictive power of savings technology in credit risk assessment can bring several benefits to companies across various industries:
- Improved decision-making: By utilizing savings technology, companies are empowered to make better-informed decisions when it comes to extending credit. The model provides an objective evaluation of credit risks, reducing the likelihood of default and potential financial losses.
- Efficiency and automation: Traditional credit risk assessment methods often involve manual analysis of financial statements and credit scoring, which can be time-consuming and prone to human error. The use of savings technology automates the process, significantly increasing efficiency and reducing costs.
- Enhanced customer experiences: With accurate credit risk predictions, companies can tailor their offers and terms based on individual customer profiles. This personalized approach enhances customer experiences and fosters long-term relationships.
Furthermore, this technology can be scaled and implemented across different business sectors, including banking, lending institutions, e-commerce, and even insurance companies. The comprehensive credit risk assessments produced by the model provide valuable insights into customer creditworthiness, enabling companies to mitigate risks and optimize their financial operations.
Savings technology has revolutionized credit risk assessment by leveraging customer financial histories and advanced machine learning algorithms. With the ability to predict credit risks accurately, companies can make informed decisions and minimize potential financial losses. The benefits of this technology extend beyond the financial industry, bringing efficiency, automation, and enhanced customer experiences. As society becomes increasingly data-driven, savings technology offers a new paradigm for credit risk assessment, ensuring companies stay competitive and secure in their financial transactions.
Comments:
Thank you all for taking the time to read my article on improving credit risk assessment with the use of ChatGPT in savings technology. I'm excited to hear your thoughts and engage in a fruitful discussion!
Great article, Add Email! The advancements in AI technology, such as leveraging ChatGPT, have definitely revolutionized various industries. I believe incorporating it into credit risk assessment could lead to more accurate predictions and better decision-making.
I agree, Michael. ChatGPT can assist in analyzing vast amounts of data efficiently, providing deeper insights into credit risk. However, do you think there could be any ethical concerns regarding AI-driven assessment and potential biases?
That's a valid point, Sarah. Biases can inadvertently be present in AI models if not properly trained or monitored. It's crucial to ensure the data used to train these models is representative and diverse to avoid unfair outcomes. Regular audits and transparency can help address any potential bias issues.
I found the article fascinating, Add Email! Integrating ChatGPT in credit risk assessment not only streamlines the process but also allows for more personalized customer experiences. It enables financial institutions to offer tailored solutions to their customers based on accurate risk evaluations.
Add Email, kudos on the article! The use of ChatGPT can also greatly benefit smaller financial institutions that may not have the resources for extensive risk assessment teams. It levels the playing field and provides access to sophisticated analysis tools, ultimately reducing costs and improving competitiveness.
I have mixed feelings about the adoption of AI in credit risk assessment. While it can be extremely efficient and accurate, I worry about the potential job displacement for human underwriters. Will they become obsolete?
Rachel, I share your concern. While AI may streamline processes, human oversight is still crucial. I believe AI should be seen as a supportive tool rather than a complete replacement. Human underwriters bring their expertise, judgment, and ability to assess more nuanced factors that AI might miss.
Adding AI capabilities to credit risk assessment can indeed enhance decision-making and accuracy. However, we must ensure there is a balance between automation and the human touch. Some customers may still prefer interacting with a person when discussing financial matters. Keeping a hybrid approach might be beneficial.
Great insights, everyone! The adoption of AI in credit risk assessment is indeed a complex matter that requires careful consideration. The points raised regarding ethics, potential biases, job displacement, and maintaining a hybrid approach are all crucial aspects to address. Thank you for your valuable input.
Add Email, I really enjoyed your article. As an AI enthusiast, I believe the possibilities for leveraging ChatGPT in various sectors are limitless. If properly implemented and monitored, AI can truly revolutionize credit risk assessment and yield tremendous benefits.
Thank you, Chris! I share your enthusiasm for AI's potential in revolutionizing different domains. It's important to keep exploring ways to optimize its use while being mindful of its limitations and ethical considerations.
Add Email, your article highlights an exciting application of AI. I believe leveraging ChatGPT in credit risk assessment will not only speed up the process but also improve accuracy, reducing losses for financial institutions. Are there any specific challenges in implementing this technology?
Linda, great question! One of the challenges in implementing ChatGPT for credit risk assessment is ensuring the accuracy and reliability of the underlying data used for training. It's crucial to have high-quality and diverse data to avoid biases and provide reliable risk evaluations.
Add Email, your article got me thinking about the potential use of ChatGPT in other financial areas, such as fraud detection. Do you believe it could be as effective in those fields as well?
Adam, absolutely! ChatGPT can have significant applications in fraud detection too. By analyzing patterns and deviations from normal behavior, it can help identify suspicious activities and enable financial institutions to respond promptly. It has the potential to enhance existing fraud detection systems.
I'm curious to know if there have been any real-life implementations of ChatGPT in credit risk assessment. Are there any success stories you'd like to share, Add Email?
Laura, there are indeed some real-life implementations of ChatGPT in credit risk assessment. Several financial institutions have started experimenting with AI-driven risk evaluation models, and initial feedback has been positive. However, it's still an evolving field, and more extensive research and testing are needed for broader adoption.
I appreciate your article, Add Email. While the benefits of ChatGPT in credit risk assessment are evident, what potential risks or downsides should financial institutions consider before implementing this technology?
Sophia, it's essential for financial institutions to consider potential risks before implementing ChatGPT. Some challenges include mitigating algorithmic biases, ensuring data privacy and security, and addressing system vulnerabilities. Regulatory compliance is another aspect that needs careful attention. Thorough risk assessments and robust frameworks should be in place to address these concerns.
Add Email, your article is insightful! With the adoption of ChatGPT in credit risk assessment, do you anticipate any significant changes in the overall credit industry landscape?
Jennifer, the integration of AI technologies like ChatGPT in credit risk assessment has the potential to reshape the industry landscape. It can lead to more efficient credit evaluations, improved risk management, increased accuracy, and faster decision-making processes. It might also encourage better access to credit for individuals who were previously underserved.
Great article, Add Email! I believe the use of ChatGPT in credit risk assessment could also play a vital role in promoting financial inclusion. By analyzing non-traditional data and evaluating risks more accurately, access to credit might extend to individuals who lack established credit histories.
Elizabeth, you raise an essential point. AI-powered credit risk assessment has the potential to break existing barriers, allowing financial institutions to evaluate creditworthiness beyond traditional credit scores. This can indeed contribute to inclusive financing and increased economic opportunities for more individuals.
Add Email, thank you for shedding light on this exciting topic. I'm curious to know if regulatory bodies are proactive in addressing the implications of AI-based credit risk assessment. Are there any guidelines or frameworks in place?
Mark, regulatory bodies are actively working to keep pace with technological advancements in credit risk assessment. Many jurisdictions have started acknowledging the need for specific guidelines and frameworks regarding the use of AI in evaluating credit risks. However, the field is still evolving, and ongoing collaboration is required to ensure effective and comprehensive regulations.
Add Email, excellent article! I wonder if there are any potential limitations to ChatGPT in credit risk assessment. Are there specific scenarios where it might not be as effective?
Jonathan, ChatGPT is indeed a powerful tool, but it has its limitations. It performs better when dealing with structured and well-labeled data. In scenarios where the data quality is poor, unstructured, or lacks clear patterns, its effectiveness might be reduced. It's crucial to choose the right tools for the specific requirements, considering the strengths and limitations of AI models.
I enjoyed your article, Add Email. The use of ChatGPT in credit risk assessment seems very promising. However, should financial institutions be concerned about potential AI-related risks, such as adversarial attacks or model exploitation?
Michelle, it's essential for financial institutions to be mindful of AI-related risks. Adversarial attacks and model exploitation are legitimate concerns. Robust security measures, continuous model verification, and readiness to adapt to emerging threats are necessary to minimize such risks. It's an ongoing process that requires vigilance and collaboration between data scientists, cybersecurity experts, and industry professionals.
Add Email, I found your article very insightful. Do you envision any future developments or enhancements of ChatGPT that could further benefit credit risk assessment?
David, future developments in ChatGPT and broader AI research hold significant potential for further enhancing credit risk assessment. Advancements in natural language understanding, improved models, and increased interpretability can lead to more accurate risk evaluations, reduced bias, and enhanced customer experiences. Continuous research and innovation are essential to leverage AI's full capabilities.
Great article, Add Email! I'm wondering if there are any notable cost implications associated with implementing ChatGPT in credit risk assessment, especially for smaller financial institutions.
Sophie, cost implications can be a concern for smaller institutions, but ChatGPT also offers potential cost-saving benefits. While there may be initial implementation and integration costs, the long-term benefits outweigh them. It reduces the need for extensive manual analysis, minimizes human error, and improves efficiency. It can enable smaller institutions to compete with larger ones in terms of risk assessment capabilities.
Add Email, your article got me thinking about the accessibility of ChatGPT. Are there any prerequisites or technical expertise required for financial institutions interested in leveraging this technology for credit risk assessment?
Jason, integrating ChatGPT or similar technologies does require certain prerequisites. Financial institutions need access to quality data, infrastructure to deploy and maintain AI models, and talent with technical expertise in AI. While it may seem challenging, collaborations with AI solution providers and investing in upskilling can bridge the gap for institutions interested in adopting these technologies.
Add Email, I enjoyed reading your article. Is there a specific timeframe financial institutions should consider for implementing ChatGPT in credit risk assessment, or is it more of an ongoing process?
Lisa, the timeframe for implementing ChatGPT in credit risk assessment can vary depending on each institution's readiness and priorities. It's crucial to start with small pilot projects to assess effectiveness, identify challenges, and adapt accordingly. From there, a gradual scaling-up approach can be adopted, prioritizing areas where the technology can bring the most value.
Add Email, you've prompted stimulating discussions with your article. The potential benefits of ChatGPT in credit risk assessment are intriguing. It's exciting to think about how AI can continue transforming various industries.
Jonathan, I'm glad to have sparked these discussions and ideas. AI's transformative potential is indeed remarkable, and continuous exploration of its applications can lead to further advancements across industries. Thank you all for your engagement and valuable insights!