Improving Data Quality with Gemini: A Game-Changer for Technology
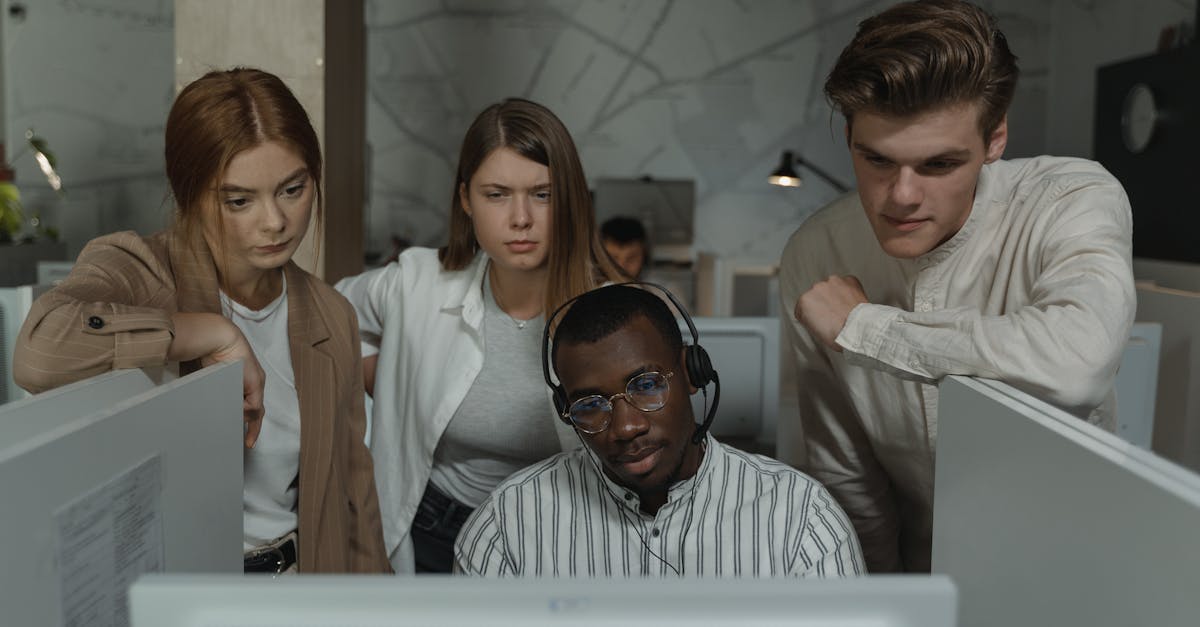
As technology continues to advance, the need for high-quality data is becoming increasingly important. Whether it's for training machine learning models, improving customer interactions, or making data-driven decisions, businesses and individuals rely on accurate and reliable data. However, ensuring data quality can be a challenging task.
Enter Gemini, an Google language model that utilizes cutting-edge natural language processing techniques to generate human-like responses. While Gemini was primarily designed for conversational AI applications, it has also proven to be a game-changer for improving data quality in a variety of technology-related contexts.
Technology
Gemini is built on the foundation of deep learning and neural networks. It utilizes a transformer architecture, which enables it to understand the context and generate contextually relevant responses. This technology has revolutionized how we approach natural language processing tasks, enabling more accurate and sophisticated language modeling.
With its ability to understand and generate human-like responses, Gemini can assist in various technology domains, including customer support systems, chatbots, data analysis, content generation, and more. It can play a crucial role in enhancing user interactions and automating processes in technology-driven industries.
Area
The application of Gemini is not limited to a specific area but extends to a wide range of industries and sectors. From e-commerce to healthcare, finance to education, Gemini can be employed to improve data quality and enhance user experiences.
In e-commerce, for instance, Gemini can aid in providing personalized recommendations, answering customer queries, and improving overall customer satisfaction. In healthcare, it can assist in telemedicine platforms, helping patients get accurate information and guidance. In the finance sector, it can analyze financial data, generate reports, and provide insights for investment decisions.
The practical applications of Gemini are vast and continue to expand as the technology advances. Organizations across various industries are recognizing its potential in improving data quality and driving innovation.
Usage
Integrating Gemini into existing workflows or systems is relatively straightforward. Google provides APIs and SDKs, making it accessible for developers to leverage the power of Gemini. With careful consideration of data privacy and ethical implications, businesses can make use of Gemini to enhance their products and services.
Using Gemini to improve data quality involves incorporating it as an intermediary between data collection and analysis processes. By leveraging Gemini's language generation capabilities, organizations can collect additional data points or validate existing data by asking specific questions or seeking clarifications.
For example, in a data analysis scenario, analysts can interact with Gemini to refine and validate data before further analysis. Gemini can provide insights, correct errors, and identify data inconsistencies, thereby improving the overall quality and reliability of the data.
Furthermore, Gemini's ability to generate language in different styles and tones allows for more diverse and comprehensive data collection. It helps in gathering nuanced responses and understanding varying perspectives, leading to a more accurate representation of the data.
Conclusion
The role of data in today's technology-driven world cannot be understated. As businesses and individuals require reliable and accurate data, technologies like Gemini play a crucial role in improving data quality. By leveraging its advanced language modeling capabilities, organizations can enhance their data analysis processes, drive innovation, and ultimately deliver better products and services to their customers.
As technology continues to evolve, we can expect further advancements in natural language processing and AI models like Gemini. These advancements will undoubtedly contribute to improved data quality and enable organizations to make data-driven decisions with confidence.
Comments:
Thank you all for taking the time to read my article on improving data quality with Gemini! I'd love to hear your thoughts and opinions on the topic.
This article was really insightful, Maicon! I never thought about using Gemini for improving data quality before. It definitely seems like a game-changer for technology.
I completely agree, Anna! The potential of using AI like Gemini to enhance data quality is tremendous. It has the ability to automate tedious tasks and improve the accuracy of data.
Absolutely, Marcos! It's fascinating to see how AI models like Gemini can learn from large datasets and help in identifying inconsistencies or errors in data. This can save a lot of manual effort and time.
I found the article really informative, Maicon. I do wonder, though, if Gemini might introduce biases or errors into the data. How can we address that?
That's a great concern, Michael. While Gemini is typically trained on diverse datasets, biases can still emerge. It's crucial to have monitoring systems in place to identify and mitigate any bias or error. By continuously refining the training data and implementing feedback loops, we can ensure that the system improves over time.
I agree with Maicon. Bias detection and mitigation should be an integral part of using AI models like Gemini. It's essential to have a transparent and accountable process in place to address biases and ensure fair and accurate data.
Interesting article, Maicon! However, how scalable is the use of Gemini for improving data quality? Can it handle large datasets effectively?
Great question, Alex! Gemini can indeed handle large datasets effectively. With its ability to process vast amounts of information quickly, it can assist in analyzing and enhancing data quality at scale. Of course, the infrastructure and resources required should be considered for large-scale deployments.
That's good to know, Maicon! Having a scalable solution for improving data quality is crucial as companies deal with increasingly complex and large datasets. Gemini seems to be a promising tool in that regard.
I'm curious, Maicon, how does Gemini handle unstructured or messy data? Can it still provide value in such cases?
Good question, Daniel! While Gemini primarily operates on text data, it can handle unstructured or messy data to some extent. However, it may require some preprocessing or cleaning depending on the specific use case. In scenarios where the data quality is extremely poor, additional data cleaning steps might be necessary to ensure optimal results.
I agree, Maicon. Preprocessing is often crucial when dealing with unstructured or messy data. It's essential to have a comprehensive understanding of the data and the problem at hand to make the best use of tools like Gemini.
Great article, Maicon! I can see how Gemini can revolutionize data quality improvement. However, what are some potential limitations or challenges when using such AI models?
Thank you, Tom! While AI models like Gemini have tremendous potential, there are indeed challenges. One limitation is that they may have difficulty with out-of-distribution or novel inputs. Also, they might generate output that sounds plausible but is factually incorrect. Continuous monitoring, feedback loops, and human oversight are necessary to mitigate these challenges.
That's a valid point, Maicon. Human involvement and critical thinking are crucial when working with AI models. They should be seen as tools that can assist humans in making better decisions, rather than making decisions on their own.
I enjoyed reading your article, Maicon. It's interesting to see how Gemini can contribute to improving data quality. Do you think it can also be beneficial beyond the technology sector?
Absolutely, Joshua! While the focus of this article is on the technology sector, Gemini has the potential to enhance data quality in various domains. Industries like healthcare, finance, and customer support can greatly benefit from its capabilities.
I agree with Maicon. Improving data quality is a universal challenge, and AI models like Gemini can provide valuable assistance across industries. It's exciting to think of the possibilities!
Great article, Maicon! I'm curious to know if there are any real-world examples where Gemini has been successfully used for improving data quality. Do you have any insights?
Thank you, Nathan! Indeed, there are practical examples where Gemini has shown promise in improving data quality. For instance, it has been used to detect and correct errors in large-scale datasets used in machine learning competitions. It has also assisted in analyzing and validating data collected from various sources, contributing to higher data quality.
That's impressive, Maicon! It's exciting to see real-world applications of Gemini in enhancing data quality. I can see how it can be a valuable addition to data management workflows.
Maicon, I enjoyed your article on improving data quality with Gemini. I'm particularly interested in the challenges that arise when dealing with multilingual or non-English datasets. How does Gemini handle such scenarios?
Thank you, Liam! Gemini can indeed handle multilingual datasets to some extent. However, its performance might vary depending on the language. While it has been trained on diverse data, using domain-specific or higher-quality training data can help improve its effectiveness with non-English datasets.
That's good to know, Maicon. Language diversity is a significant aspect when it comes to improving data quality. It's essential to consider the specific language challenges and adapt the training and deployment accordingly.
Maicon, the article was really informative. I'm wondering if Gemini can help in data cleaning tasks when dealing with unstructured text data?
Thank you, Julia! Yes, Gemini can certainly be utilized in data cleaning tasks involving unstructured text data. For example, it can assist in identifying and removing redundant or irrelevant information, standardizing data formats, or even suggesting improvements for better data consistency.
That sounds really useful, Maicon! Data cleaning can be a time-consuming and tedious process, especially when dealing with unstructured data. Gemini seems like a great tool to make this task more efficient.
Great article, Maicon! I'm curious to know if there are any limitations on the types of data formats that Gemini can handle effectively.
Thank you, Ethan! While Gemini is primarily designed for text-based inputs, it can handle a variety of data formats effectively. However, its performance might vary based on the complexity and structure of the data. Domain-specific preprocessing or adapting the model architecture can help improve its effectiveness with specific formats if needed.
That's interesting, Maicon. It's important to consider the specific data formats when integrating tools like Gemini into data quality improvement processes. Adapting and fine-tuning the model accordingly can yield better results.
Maicon, your article on improving data quality with Gemini was a great read. As technology continues to advance, do you think AI models will become the standard for data quality improvement?
Thank you, David! AI models like Gemini have certainly shown immense potential in enhancing data quality. While they can automate certain aspects and improve efficiency, I believe human oversight and decision-making will always be crucial. Integrating AI models into data quality improvement workflows can become more common, but they are more likely to complement human expertise rather than fully replace it.
I agree with Maicon. AI models can significantly contribute to data quality improvement, but they should be seen as tools that empower human decision-making and assist in complex tasks. The combination of human expertise and AI capabilities can lead to better outcomes.
Great article, Maicon! I'm curious if there are any known security concerns when using AI models like Gemini for data quality improvement?
Thank you, Emma! Security concerns are indeed important to consider. AI models like Gemini should be implemented with proper security measures to protect sensitive data. Additionally, continuous monitoring and auditing should be in place to spot any potential vulnerabilities or risks associated with their usage.
Absolutely, Maicon. Ensuring the confidentiality and integrity of data is crucial. Organizations should follow best practices in data security and regularly assess any potential risks or vulnerabilities that might arise from using AI models.
Maicon, your article provided great insights into using Gemini for data quality improvement. Can you share any resources or references to dive deeper into this topic?
Thank you, Lucas! If you want to explore further, I recommend checking out Google's website, as they provide detailed documentation and research papers on AI models like Gemini. Additionally, there are various articles and academic papers available on the topic of data quality improvement and the application of AI in this domain.
That's helpful, Maicon. Leveraging available resources and staying updated with the latest research and advancements is key to further understanding and applying AI models for data quality improvement.
Thank you all for reading my article on improving data quality with Gemini! I'm excited to discuss this topic with you.
Great article, Maicon! I found your insights on using Gemini to enhance data quality really interesting.
Thanks, Emma! I appreciate your feedback.
Maicon, I find your suggestions regarding resource optimization and cloud infrastructure deployment for Gemini valuable. It allows organizations to scale their data quality improvement efforts efficiently.
Maicon, it's understandable that the guidelines for Gemini might still be evolving. Organizations can leverage existing responsible AI frameworks and adapt them to suit their specific data quality improvement projects.
Emma, that's a great point! Responsible AI frameworks provide a useful foundation for organizations to align their Gemini implementation with ethical and responsible practices.
Maicon, the broad applicability of Gemini for data quality improvement makes it an invaluable tool across industries. Its versatile nature enables organizations to tackle their specific data quality challenges effectively.
Emma, involving diverse perspectives in the refinement process aids in reducing biases, ensuring fairness, and promoting equitable data quality improvement practices.
Maicon, leveraging established responsible AI frameworks provides a strong foundation for organizations to ensure ethical and beneficial use of Gemini when improving data quality.
Emma, indeed! Responsible AI frameworks help organizations embrace the potential of Gemini for data quality improvement while adhering to ethical practices and avoiding any unintended negative impacts.
Maicon, that's precisely the approach organizations should follow. Responsible and ethical practices are crucial when implementing AI technologies like Gemini to ensure positive outcomes in data quality improvement.
Emma, incorporating diverse perspectives helps minimize biases and ensures that Gemini's analysis considers a wide range of viewpoints, contributing to more inclusive and equitable data quality improvement.
Olivia, you're absolutely right. Gemini's contribution to the reliability and trustworthiness of the data paves the way for accurate decisions and meaningful insights.
Oliver, indeed! Reliable and trustworthy data is the backbone of successful data-driven projects, and Gemini's role in improving data quality supports that foundation.
Emma, Gemini's implementation in data quality improvement projects should closely align with existing responsible AI frameworks and ethical guidelines to ensure fairness, transparency, and accountability.
Maicon, the impact of Gemini in improving data quality spans across diverse industries, making it a versatile game-changer for technology regardless of the sector.
Emma, you're absolutely right! Gemini's broad applicability makes it a powerful tool for data quality improvement across various industries, leveraging its versatile capabilities.
Maicon, the versatility of Gemini allows organizations from different industries to leverage its potential to address their unique data quality challenges effectively, enhancing their data-driven initiatives.
Maicon, the versatility of Gemini to address specific data quality challenges in various industries makes it a powerful tool for enhancing data-driven decision-making across domains.
Emma, absolutely! By offering customized solutions for data quality improvement, Gemini holds significant potential in empowering organizations across domains to achieve more reliable and impactful data-driven decision-making.
Maicon, leveraging Gemini's potential for data quality improvement requires organizations to strike a balance between resource allocation, computational requirements, and data relevance, optimizing these elements for maximum effectiveness.
Emma, you summarized it well! Achieving optimal utilization of Gemini for data quality improvement involves finding the right balance between resource allocation, computational requirements, and data relevance. This balance ensures efficient implementation and effective outcomes.
Maicon, striking that balance is key to unlocking the full potential of Gemini, ultimately leading to improved data quality and better decision-making in organizations.
Emma, absolutely! Striking the right balance empowers organizations to harness Gemini effectively, enhancing data quality and enabling informed decision-making throughout their projects.
Maicon, responsible AI implementation ensures that Gemini is used ethically and effectively for data quality improvement, preventing any potential negative impacts on individuals or communities.
Emma, I couldn't agree more. Responsible AI implementation places ethics, accountability, and societal impact at the forefront, ensuring that Gemini's deployment in data quality improvement projects remains beneficial and avoids unintended consequences.
Maicon, responsible AI implementation aligns Gemini's capabilities with ethical guidelines to ensure data quality improvement remains a positive force for organizations, individuals, and communities.
Emma, exactly! Responsible AI implementation empowers organizations to leverage Gemini's capabilities while upholding ethical standards, increasing the positive impact of data quality improvement.
Maicon, responsible AI implementation ensures that the deployment of Gemini follows the best possible practices, considering ethical guidelines, societal impact, and long-term benefits in data quality improvement.
Emma, completely agreed! Responsible AI implementation in Gemini's deployment for data quality improvement optimally combines ethical considerations, stakeholder engagement, and long-term societal benefits for the best possible outcomes.
Maicon, indeed! Responsible AI implementation strengthens data quality improvement practices by ensuring that organizational decision-making remains ethical, accountable, and aligned with societal needs.
Emma, responsible AI implementation creates a pathway for organizations to utilize Gemini for data quality improvement while prioritizing ethics, fairness, and collective well-being, leading to more reliable and impactful results.
Maicon, utilizing cloud infrastructure for deploying Gemini offers scalability and flexibility, which are crucial for organizations in managing varied computational resource demands for optimal data quality improvement.
Emma, absolutely! Cloud infrastructure provides organizations with the scalability and flexibility needed to accommodate the varying computational resource requirements of Gemini, enabling efficient and effective data quality improvement.
Maicon, leveraging cloud infrastructure empowers organizations to dynamically scale their Gemini resources, adapting to the specific demands of data quality improvement with ease.
Emma, you've summarized it perfectly! Cloud infrastructure allows organizations to scale their Gemini resources effortlessly, ensuring a well-managed and resource-efficient data quality improvement process.
Emma, ensuring the balance between resource allocation, computational requirements, and data relevance while employing Gemini for data quality improvement is essential. Optimal utilization of resources maximizes the benefits.
Lauren, I completely agree. Striking the right balance allows organizations to achieve optimal outcomes, ensuring efficient utilization of resources when utilizing Gemini for data quality improvement.
Emma, the effective allocation of resources ensures a sustainable and impactful implementation of Gemini, contributing to enhanced data quality and more informed decision-making.
Lauren, resource optimization drives the effectiveness and efficiency of Gemini's data quality improvement initiatives. Balancing resources enables organizations to achieve tangible improvements in decision-making and overall outcomes.
Emma, responsible AI frameworks provide guiding principles that organizations can follow to deploy Gemini ethically and effectively, ensuring the benefits of data quality improvement while upholding trust and transparency.
Sophia, responsible AI frameworks are invaluable in establishing the necessary guidelines for organizations to adopt and implement Gemini, fostering ethical, trustworthy, and impactful data quality improvement initiatives.
Emma, exactly! Responsible AI frameworks facilitate a structured approach to data quality improvement using Gemini, enabling organizations to harness its potential while meeting ethical, legal, and societal requirements.
Sophia, responsible AI frameworks provide organizations with a solid foundation to ensure that Gemini is deployed ethically and effectively for data quality improvement, promoting positive outcomes and societal benefits.
Emma, resource optimization is key to maximizing the outcomes of Gemini for data quality improvement. Efficient utilization of resources allows organizations to enhance the overall quality of their data-driven projects.
Lauren, exactly! By optimizing resources, organizations can leverage Gemini effectively, improving data quality and elevating the success of their data-driven projects.
Emma, resource optimization enables organizations to unlock Gemini's full potential in data quality improvement while being mindful of computational requirements and data relevance, leading to more impactful insights.
Lauren, resource optimization aligns computational requirements, data relevance, and resource allocation, enabling organizations to harness Gemini effectively and derive maximum value in their data quality improvement journeys.
Emma, involving domain experts at various stages helps in refining Gemini's output, resulting in more accurate and contextually aligned data quality improvement strategies.
Olivia, involving domain experts throughout the refinement process ensures that the outputs of Gemini for data quality improvement are accurate, reliable, and align with specific organizational goals.
Emma, I completely agree. Domain experts possess crucial insights that contribute to refining Gemini's results in ways that improve data quality and foster informed decision-making.
Olivia, you summarized it perfectly! Domain experts play a vital role in refining Gemini's outputs, making data quality improvement more impactful and aligned with the unique requirements of different projects.
Emma, involving diverse perspectives throughout the refinement process helps minimize biases, ensuring fair and inclusive data quality improvement practices.
Oliver, diversity and inclusivity are pivotal in combating biases and ensuring that Gemini's analysis produces equitable and unbiased data quality improvement outcomes.
Emma, absolutely! By embracing diverse perspectives, organizations can effectively address biases in data quality improvement, contributing to more accurate and inclusive results.
Oliver, diversity and inclusivity are crucial for building fair and representative datasets. Engaging diverse perspectives promotes more robust and unbiased data quality improvement practices.
Emma, implementing Gemini for data quality improvement should align with responsible AI frameworks to ensure fairness, transparency, and accountability throughout the process.
Sophia, I completely agree. By adhering to responsible AI frameworks, organizations create an environment that promotes ethical and impactful data quality improvement using Gemini.
Emma, responsible AI frameworks foster inclusive, ethical, and accountable practices when organizations implement Gemini for data quality improvement, ensuring the best outcomes.
Sophia, I couldn't agree more! Responsible AI frameworks provide valuable guidance, enabling organizations to harness the potential of Gemini responsibly while ensuring the highest standards of ethics, transparency, and accountability.
Maicon, your article was very informative. I'm curious to know more about the specific applications of Gemini for improving data quality.
Hi Alex, I'm glad you found the article informative. Gemini can be used in various applications, such as data cleaning and validation, data augmentation, and quality control of large datasets.
Maicon, thanks for elaborating! Gemini seems versatile in its applications. Have you encountered any limitations or challenges in using Gemini for improving data quality?
Alex, I have also wondered about potential limitations. For instance, does Gemini work well with noisy and unstructured datasets where data quality issues are prevalent?
Lauren, Gemini can handle noisy and unstructured datasets to some extent, but it may struggle with extremely messy data or when there's a lack of contextual information. Preprocessing and data cleaning steps are crucial to achieve better results.
I see, Alex. Preprocessing and cleaning the data beforehand becomes even more important when dealing with noisy and unstructured datasets.
Lauren, noise and lack of structure can impact the performance of Gemini. However, fine-tuning the model or limiting the input to relevant quality-related attributes can help cope with such challenges.
Alex, I agree that pre-processing and cleaning are crucial steps to ensure optimal results when using Gemini for data quality improvement.
Alex, limited contextual information can indeed be a challenge in processing messy or unstructured datasets. It highlights the importance of thorough data preparation to enhance Gemini's performance.
That's an excellent point, Alex. Gemini can contribute to data cleaning by flagging missing or incomplete entries, allowing data analysts to rectify and improve the overall data quality.
Indeed, Liam. By using Gemini, we can automate the process of identifying errors, outliers, and inconsistencies, saving valuable time and effort for data professionals.
Alex, I think Gemini can play a crucial role in improving the quality of text-based datasets. It can help identify and correct errors, inconsistencies, and outliers in the data.
Alex, Gemini can also provide an additional layer of quality control by identifying missing data or incomplete entries in large datasets.
Hi Maicon, as a data analyst, I'm excited about the potential of Gemini in enhancing data quality. Can you share any practical examples of its implementation in real-world scenarios?
Hello Lauren! Sure, one practical example is using Gemini to generate synthetic data that follows the patterns and characteristics of real data, which can then be used for testing and validation purposes.
Maicon, do you think Gemini can assist in identifying biases or potential pitfalls in large datasets?
Hi Sophia, absolutely! Gemini can help detect biases in datasets by identifying potentially discriminatory or biased language patterns. It can also assist in flagging potential pitfalls, such as data inconsistencies or erroneous entries.
Maicon, that's an interesting application! Using synthetic data generated by Gemini can indeed serve as a useful approach for data validation. Do you have any tips for implementing this process effectively?
Olivia, when implementing the validation process using synthetic data, it's important to compare the results with real data to ensure accuracy and reliability. It's also crucial to iteratively refine the synthetic data generation process based on feedback from domain experts.
Maicon, using Gemini to generate synthetic data for testing and validation sounds promising. Does it require a large amount of manually labeled data for training the model effectively?
Lauren, good question! While supervised fine-tuning may require labeled data specific to the target application, Gemini can still generate useful synthetic data even without extensive manual labeling. It can leverage pre-training on a large corpus to learn general language patterns while fine-tuning on smaller labeled datasets.
Maicon, thank you for clarifying that. It's good to know that Gemini's ability to generate synthetic data doesn't solely rely on large amounts of manually labeled data.
You're welcome, Lauren! Gemini's pre-training on a large corpus plays a crucial role in its ability to generate high-quality synthetic data, even with limited labeled data available.
Maicon, considering the resource constraints in an enterprise setting, what are the potential trade-offs organizations may need to consider in utilizing Gemini for data quality improvement effectively?
Lauren, one potential trade-off is the availability of computational resources. Deploying and scaling Gemini may require substantial compute power, impacting cost and infrastructure requirements. Additionally, organizations need to balance computational resources with the need for quality training data while ensuring optimal performance.
Maicon, thank you for highlighting the potential trade-offs. It's crucial for organizations to carefully weigh the cost, infrastructure, and training data requirements when leveraging Gemini for data quality improvement.
Lauren, privacy and data protection must be at the core of any AI implementation. Organizations should prioritize establishing robust protection mechanisms to safeguard user data when utilizing Gemini for data quality improvement.
Oliver, I completely agree. Privacy and data security are imperative, and organizations should ensure stringent measures are in place to protect user data when deploying Gemini for data quality improvement.
Lauren, exactly! Upholding the highest standards of privacy and data security is essential for user confidence, enabling organizations to utilize Gemini for data quality improvement effectively.
Oliver, protecting user data not only ensures compliance with regulations but also maintains trust, promoting responsible and ethical use of Gemini for data quality improvement.
Lauren, while Gemini handles unstructured and noisy datasets to some extent, ensuring adequate preprocessing and cleaning steps is crucial for optimal performance and accurate data quality improvement.
Alex, I completely agree. Preprocessing and cleaning the data play a pivotal role in maximizing Gemini's effectiveness in improving the data quality of unstructured and noisy datasets.
Lauren, indeed! To derive reliable insights from unstructured or noisy datasets, it's essential to ensure data preprocessing steps are in place to set the stage for successful data quality improvements with Gemini.
Alex, preprocessing acts as the foundation for accurate and meaningful results. By leveraging Gemini on well-prepared datasets, organizations can achieve significant advancements in data quality improvement.
Alex, Gemini's ability to identify hidden errors, inconsistencies, and missing data significantly contributes to improving the overall data quality, enabling organizations to make informed and accurate decisions.
Liam, absolutely! By flagging errors, inconsistencies, and missing data, Gemini acts as an invaluable assistant in the data cleaning process, elevating data quality and supporting reliable decision-making.
Alex, Gemini's contribution to data quality improvement helps organizations ensure the integrity and reliability of their datasets, fostering trust and facilitating better outcomes in data-driven projects.
Liam, you summarized it well! Gemini empowers organizations to achieve more accurate and reliable data-driven outcomes, bolstering confidence in decision-making and enhancing overall project success.
Alex, Gemini's role in error detection and flagging inconsistencies adds an extra layer of quality control, fostering trustworthy data analysis and decision-making.
Olivia, that's precisely it! By detecting errors and flagging inconsistencies, Gemini augments the quality control process, strengthening the reliability and trustworthiness of data for analysis and decision-making.
That's great! Gemini's ability to detect biases can be invaluable in creating fair and unbiased datasets. Do you have any recommendations for addressing or mitigating biases identified by Gemini?
Sophia, addressing biases identified by Gemini requires a combination of human review and domain expertise. It's essential to involve diverse perspectives and ensure a collaborative approach to mitigate biases effectively.
Maicon, I really enjoyed your article! It was insightful to learn about the potential of Gemini for enhancing data quality. Do you have any specific tips for getting started with implementing Gemini in a data quality improvement project?
Sofia, thank you for your kind words! When implementing Gemini for a data quality improvement project, it's important to start with a clear objective, define evaluation metrics, and gradually fine-tune the model based on specific domain requirements.
Maicon, thank you for the valuable suggestions! I'm excited to explore Gemini's potential for improving data quality in my own projects.
Sofia, you're welcome! I'm glad you found the suggestions valuable. Best of luck with your projects, and feel free to reach out if you have any further questions about Gemini and data quality improvement.
Maicon, your article provided excellent insights into the benefits of using Gemini for data quality improvement. How widely is Gemini being adopted in the industry, and what are the major challenges organizations face when implementing it?
Eric, Gemini's adoption in the industry is growing rapidly. However, organizations often face challenges with data privacy, model interpretability, and fine-tuning the model for specific domain requirements. Overcoming these challenges requires careful planning, collaboration, and addressing ethical considerations.
Maicon, thank you for addressing my question. It's reassuring to see the growing adoption, although the challenges underline the need for cautious implementation and ethical considerations.
Maicon, this article has convinced me of the potential of Gemini for data quality improvement. Are there any challenges in deploying Gemini in an enterprise setting?
Maicon, I'd like to understand the scalability of deploying Gemini in an enterprise setting. Are there any considerations in terms of computational resources or training data volume?
Sophia, incorporating an iterative feedback loop helps to refine the results and minimize biases. It's an ongoing process that requires constant collaboration between data experts and Gemini's output.
Emma, involving domain experts is paramount to refine the results and avoid potential biases, which ultimately leads to better data quality for downstream tasks.
Olivia, indeed! By automating error detection, Gemini empowers data analysts to focus on higher-value tasks that require critical thinking and domain expertise.
Sophia, incorporating diverse perspectives at different stages of the refinement process helps in ensuring fairness and reducing biases in the final outputs.
Emma, absolutely! Diversity and inclusivity play crucial roles in refining Gemini's output, making it more robust and unbiased.
Emma, involving domain experts throughout the iterative process not only addresses biases but also ensures the outputs generated by Gemini are of high quality and aligned with the specific needs of the project.
Emma, I completely agree with you. Including diverse perspectives helps in refining the system to make it fairer and more inclusive.
Emma, diverse perspectives serve as a crucial checkpoint to ensure fairness and avoid potential biases that may arise from using Gemini in data quality improvement.
Maicon, considering the computational resources required for deploying Gemini at scale in an enterprise setting, what would be the optimal approach to managing resource constraints?
Sophia, managing resource constraints can be challenging. One approach is to deploy Gemini on cloud infrastructure, which allows scaling up or down based on varying computational needs. Additionally, optimizing the model architecture can help reduce resource requirements.
Maicon, with the increasing adoption of Gemini, what steps can organizations take to ensure data protection and privacy while leveraging its capabilities?
Sophia, organizations should carefully handle data when using Gemini and adhere to data protection guidelines and privacy regulations. Anonymizing or de-identifying sensitive information and ensuring secure infrastructure play crucial roles in protecting data privacy.
Maicon, I appreciate your response. Adhering to data protection guidelines is crucial to prevent any potential privacy breaches when implementing Gemini for data quality improvement.
Maicon, I agree with Sophia, privacy considerations must be paramount. Organizations should establish robust policies and procedures to protect user data throughout the implementation of Gemini.
Oliver, you're absolutely right. Privacy should be at the forefront of any data-driven initiative, including the adoption of Gemini for data quality improvement in organizations.
Sophia, absolutely! Privacy must be treated as a paramount concern throughout the implementation of any AI systems, including Gemini, to build user trust and maintain ethical practices.
Oliver, Liam, and Alex, you all have highlighted some great points. The combination of automated processes like Gemini and human expertise forms a strong foundation for improving data quality and reaching reliable insights.
Maicon, I completely agree. The collaboration between AI systems like Gemini and human experts is key to achieving comprehensive and accurate data quality improvement.
Maicon, considering the growing adoption of Gemini, are there any guidelines or best practices available for organizations to follow in order to maximize the benefits of using Gemini for data quality improvement?
Sophia, as Gemini is relatively new, comprehensive guidelines might still be evolving. However, organizations can follow established best practices for responsible AI implementation, including transparent documentation, robust evaluation, and involving diverse stakeholders to address potential biases and ensure ethical practices.
Maicon, I appreciate your response. Following responsible AI practices helps ensure that the implementation of Gemini for data quality improvement remains ethical and beneficial for organizations.
Maicon, exploring responsible AI frameworks and adapting them for Gemini implementation is a great way to ensure ethical and beneficial use of the technology in data quality improvement.
Indeed, Sophia! Responsible AI frameworks serve as guiding principles to uphold ethical and beneficial practices when leveraging Gemini for data quality improvement.
Maicon, adhering to data privacy guidelines is crucial to establish trust and protect user data while leveraging Gemini's capabilities for data quality improvement.
Sophia, absolutely! Protecting user data and respecting privacy guidelines are paramount as organizations leverage Gemini for data quality improvement, fostering trust and ethical practices.
Maicon, are there any specific industry domains where Gemini is showing exceptional promise in improving data quality?
Sophia, Gemini shows promise across various industry domains, including healthcare, finance, e-commerce, and customer support. Its versatile applications make it a game-changer for technology in multiple sectors.
Maicon, that's exciting to hear! Gemini's potential impact on data quality improvement spans across diverse industries, which further emphasizes its significance.
Maicon, as organizations leverage Gemini for data quality improvement, it's vital to ensure data privacy, safeguarding user information and maintaining confidentiality.
Sophia, absolutely! Organizations should prioritize strong data governance and security measures when utilizing Gemini for data quality improvement, fostering trust and maintaining privacy.
Maicon, establishing trust and ensuring data privacy are essential. Organizations must handle data carefully and ethically to maintain the trust of their users and stakeholders throughout the data quality improvement process.
Sophia, incorporating diverse perspectives is crucial for recognizing biases and minimizing their impact, resulting in fair and inclusive data quality improvement strategies.
Emma, exactly! By embracing diverse perspectives, organizations can create more equitable and inclusive data quality improvement frameworks, fostering trust and promoting unbiased results.
Sophia, I completely agree. Inclusive data quality improvement practices are paramount to ensuring fairness, trust, and ethicality in the outcomes provided by Gemini.
Sophia, trust and user data protection are significant considerations when deploying Gemini for data quality improvement. Organizations must prioritize building user trust by safeguarding data privacy.
Oliver, absolutely! Trust and data privacy go hand in hand, and organizations must establish robust measures to protect user data throughout the data quality improvement process.
Maicon, incorporating established responsible AI frameworks into Gemini implementation helps organizations ensure higher standards of ethicality, reliability, and accountability in data quality improvement.
Sophia, exactly! Responsible AI frameworks provide organizations with invaluable guidelines to navigate the implementation of Gemini for data quality improvement, promoting ethical practices and ensuring positive outcomes.
Maicon, I appreciate your insights. Responsible AI frameworks are instrumental in guiding organizations towards impactful and responsible use of Gemini for data quality improvement.
Maicon, it's interesting to know that Gemini can generate useful synthetic data even with limited labeled data. This flexibility makes it highly valuable for organizations seeking to achieve data quality improvement.
Sophia, absolutely! Gemini's ability to generate synthetic data with limited labeled data is a valuable asset, especially when organizations face data scarcity or labeling challenges in their data quality improvement endeavors.
Maicon, that's a significant advantage of Gemini in data quality improvement projects. The ability to generate synthetic data despite labeling challenges provides a flexible and cost-effective solution.
Maicon, responsible AI implementation is vital to ensure that organizations deploy Gemini within ethical boundaries for data quality improvement. Ethical practices must always be at the forefront of AI adoption.
Sophia, I couldn't agree more. Responsible AI practices are crucial for organizations adopting Gemini in data quality improvement projects, fostering transparency, accountability, and positive societal impact.
Maicon, responsible AI adoption not only enables effective data quality improvement but also upholds the values of fairness, transparency, and accountability in organizations.
Sophia, exactly! Responsible AI adoption aligns data quality improvement efforts with ethical standards, ensuring organizations positively contribute to society while reaping the benefits of Gemini.
Maicon, an iterative approach that incorporates user feedback allows organizations to refine Gemini's outputs and outputs in data quality improvement. Continuous improvement is crucial to achieve accurate and reliable results.
Sophia, absolutely! An iterative approach that involves user feedback and domain expertise is vital to make data quality improvement efforts with Gemini more effective and precise.
Maicon, refining Gemini's outputs through an iterative process enables organizations to steadily enhance data quality, ensuring that the output aligns with their specific requirements.
Sophia, you're absolutely right! An iterative feedback loop allows organizations to continuously refine and improve Gemini's outputs, ensuring the data quality improvement aligns with their objectives and expectations.
Maicon, iterative refinement of Gemini's outputs is a collaborative process that strengthens data quality improvement, fosters domain knowledge, and ultimately empowers organizations to make informed decisions.
Sophia, you summarized it perfectly! Collaborative iterative refinement enhances data quality efforts by incorporating diverse perspectives, domain knowledge, and valuable insights for better decision-making.
Maicon, responsible AI implementation is paramount to unlock the full potential of Gemini for data quality improvement while maintaining transparency, fairness, and positive societal impact.
Olivia, absolutely! Responsible AI implementation ensures that Gemini's deployment for data quality improvement aligns with ethical principles, respects privacy, and benefits society as a whole.
Maicon, responsible AI implementation paves the way for organizations to adopt Gemini effectively in data quality improvement projects while addressing potential risks and ensuring positive outcomes.
Olivia, responsible AI implementation establishes a strong foundation for organizations to utilize Gemini ethically, responsibly, and in ways that create value while minimizing potential risks in data quality improvement.
Maicon, being able to generate useful synthetic data even without extensive manual labeling is highly beneficial. It widens the possibilities for organizations to leverage Gemini effectively for data quality improvement.
Sophia, exactly! Gemini's ability to generate synthetic data with limited manual labeling requirements offers organizations a flexible and efficient way to improve data quality without being solely dependent on extensive human interventions.
Maicon, by reducing the dependency on manual labeling, Gemini's ability to generate synthetic data enables organizations to overcome resource constraints and enhance data quality more effectively.
Sophia, you've summarized it well! The reduced dependency on manual labeling facilitates a more efficient and cost-effective approach to data quality improvement using Gemini's capabilities.
Maicon, involving users and stakeholders throughout the iterative refinement process establishes a collaborative environment, ensuring that Gemini's outputs align with the desired data quality improvement objectives.
Sophia, absolutely! Involving users and stakeholders creates a collaborative feedback loop, enabling organizations to refine Gemini's outputs iteratively and achieve better alignment with their data quality improvement objectives.
Maicon, collaboration through iterative refinement brings valuable insights and domain-specific expertise into the data quality improvement process, allowing organizations to derive more accurate and contextually aligned results.
Sophia, you're absolutely right! Collaboration and iterative refinement enable organizations to harness the full potential of Gemini and ensure data-driven decision-making based on accurate and precise outputs.
Maicon, what level of interpretability does Gemini offer in the context of data quality improvement? Can organizations understand and explain the decision-making process behind Gemini's outcomes?
Sophia, Gemini's level of interpretability is a topic of ongoing research. While it lacks explicit explanations for its decision-making, organizations can explore techniques like rule-based post-processing or model-agnostic interpretability methods to enhance interpretability in the context of data quality improvement.
Maicon, thank you for clarifying. The ongoing research in interpretability and the use of additional techniques allow organizations to gain more insight into Gemini's decision-making and improve its explainability in data quality improvement projects.
Sophia, you're welcome! Organizations can leverage ongoing research and explore additional techniques to enhance the interpretability of Gemini, enabling better understanding and explainability in the context of data quality improvement.
Maicon, Gemini's ability to generate synthetic data without extensive manual labeling is a significant advantage. This flexibility facilitates data quality improvement, especially when labeled data is scarce or costly.
Sophia, absolutely! The ability of Gemini to generate synthetic data with limited manual labeling requirements empowers organizations to enhance data quality even in scenarios where labeled data is scarce, costly, or time-consuming to acquire.
Maicon, reducing the dependency on extensive manual labeling, especially when labeled data is scarce or challenging to obtain, makes Gemini an efficient and cost-effective solution for data quality improvement.
Sophia, you've captured it well! Gemini's ability to generate synthetic data efficiently with limited labeling requirements presents organizations with a valuable solution for data quality improvement, even in resource-constrained scenarios.
Maicon, incorporating Gemini for data quality improvement projects comes with considerations regarding training data volume. How does the volume and diversity of training data impact Gemini's performance?
Sophia, training data volume and diversity impact Gemini's performance. Models benefit from diverse, high-quality training data that spans different domains, enabling them to learn a wide range of language patterns. Adequate training data volume is crucial in achieving strong performance in data quality improvement tasks.
Maicon, thank you for explaining. Adequate training data volume and diversity play pivotal roles in Gemini's performance, ensuring it learns language patterns effectively and delivers accurate results in data quality improvement.
Sophia, you're most welcome! Adequate training data volume, coupled with diversity, strengthens Gemini's language modeling capacity, making it more reliable and effective in data quality improvement tasks.
Maicon, having a solid training data foundation enables Gemini to perform optimally in data quality improvement, ensuring its language model is robust, accurate, and adaptable to various contexts.
Maicon, responsible AI frameworks ensure organizations make data privacy and protection a priority throughout the Gemini-based data quality improvement process.
Sophia, absolutely! Responsible AI frameworks emphasize the importance of data privacy and protection, guiding organizations to adopt policies and practices that prioritize these aspects when leveraging Gemini for data quality improvement.
Maicon, adhering to responsible AI frameworks helps organizations uphold data privacy and protection, which are fundamental for users' trust and confidence throughout the Gemini-based data quality improvement process.
Sophia, you summarized it perfectly! Responsible AI frameworks provide organizations with invaluable guidance to ensure data privacy and protection remain a key aspect during the application of Gemini in data quality improvement initiatives.
Maicon, thank you for sharing your insights on improving data quality with Gemini. It was a thought-provoking article, and I look forward to exploring the applications of Gemini further.
Sophia, you're welcome! I'm glad you found the article thought-provoking. Feel free to explore the applications of Gemini for data quality improvement, and don't hesitate to reach out if you have any questions. Happy exploring!
Maicon, thank you for your kind words and support. I'll definitely reach out if I have any further questions. Happy exploring indeed!
Sophia, spotting biases related to gender, race, or culture is an affirmative step towards creating fair and unbiased datasets, making Gemini's capabilities highly valuable.
Daniel, I agree completely. Gemini's ability to detect biases is a crucial factor in creating more inclusive and equitable datasets for various applications.
Sophia, identifying biases through Gemini paves the way for organizations to address inequalities and strive towards creating unbiased and representative datasets.
Daniel, indeed! Gemini not only detects biases but also enables organizations to take appropriate actions needed for fostering inclusivity and building trustworthy datasets.
Sophia, absolutely! Identifying and addressing biases with the help of Gemini contributes to the creation of more reliable and unbiased datasets, ensuring fair data quality improvement outcomes.
Sophia, definitely! Gemini's potential in identifying biases can play a crucial role in creating fair and unbiased datasets.
Daniel, I agree. By leveraging Gemini's capabilities to identify biases, organizations can strive towards eliminating unfair biases and ensuring greater inclusivity in their data-driven projects.
Sophia, diversity and inclusivity are crucial in ensuring fair and unbiased datasets. Gemini's identification of biases can be instrumental in achieving such goals.
Daniel, absolutely! Recognizing biases through Gemini's analysis allows data practitioners to address them and work towards creating more equitable and unbiased datasets.
Sophia, involving diverse perspectives during the refinement process is crucial not only for mitigating biases but also for forming resilient and inclusive data quality improvement strategies.
Emma, I completely agree. Diverse perspectives bring valuable insights that ensure robust and inclusive data quality improvement practices.
Sophia, with Gemini's language modeling abilities, I believe it can assist in identifying various types of biases, including gender, racial, or cultural biases, in large datasets.
Daniel, you're right! Identifying biases related to gender, race, or cultural backgrounds is essential, and Gemini can contribute significantly in that aspect.
Sophia, one way to mitigate biases is to incorporate an iterative feedback loop with the domain experts who evaluate the results of Gemini's bias identification. Constant refinement based on their insights helps in reducing potential biases.
Emma, involving domain experts in the validation process ensures that the synthetic data aligns with the real data's characteristics, improving its usefulness in data quality assessments.
Automating the process of spotting errors and inconsistencies through Gemini can definitely enhance data quality and accelerate the overall data analysis pipeline.
Oliver, I completely agree! Gemini's role in data cleaning and quality control is crucial for ensuring reliable and accurate data-driven insights.
Liam, automating error detection using Gemini also reduces the chances of human errors in the data cleaning process, thus enhancing the overall data quality.
Oliver, by automating error detection, Gemini not only improves the data quality but also allows data analysts to focus on more complex aspects of analysis and decision-making.
Exactly, pre-processing and cleaning are the foundation for achieving accurate insights by leveraging Gemini for data quality improvement.
Lauren, prior data preprocessing steps, like data cleaning and handling missing values, contribute significantly to achieving data quality. Gemini can complement these steps by further enhancing data validity and consistency.
Alex, data cleaning and quality control are crucial steps to ensure accurate and reliable insights. Gemini can significantly contribute to achieving higher data quality standards.
Liam, you're right. Gemini's ability to identify missing or incomplete entries aids in enhancing the overall data quality, enabling better decision-making in data-driven projects.
Alex, Gemini's ability to identify errors, outliers, and inconsistencies can significantly streamline the data cleaning process and enhance the overall data quality.
That's true, Oliver. By automating parts of the data cleaning process, Gemini can free up time for data analysts to focus on more complex data tasks and analysis.
Alex, Gemini's ability to flag missing or incomplete data entries can expedite the data cleaning process and improve the overall quality of the dataset.
Absolutely, Liam! Gemini can act as an effective assistant in identifying and handling missing or incomplete data entries, contributing to higher data quality standards.
Alex, preprocessing and cleaning are indeed critical steps to ensure data quality. Gemini's additional quality control abilities can help improve the overall reliability of the data.
Lauren, I couldn't agree more! Gemini can act as a valuable asset in the data cleaning and quality control process, elevating the reliability and trustworthiness of the data.
Alex, I definitely agree. Gemini's contribution to data cleaning and quality control is vital for uncovering hidden errors or inconsistencies, ensuring accurate data analysis.
Liam, that's a great way to put it! Gemini provides an additional layer of scrutiny in the data cleaning process, enabling data professionals to achieve more accurate data-driven insights.
Alex, Gemini's ability to identify errors, outliers, and inconsistencies can indeed expedite the data cleaning process, ultimately enhancing the accuracy and reliability of the data.
Olivia, absolutely! Involving domain experts for refining Gemini's output is crucial, as it ensures high-quality results aligned with the given data context and specific project requirements.
Emma, incorporating diverse perspectives helps address biases and ensures that Gemini's identification is more accurate, leading to fair and inclusive data quality improvement.
Olivia, involving domain experts in refining Gemini's results brings invaluable contextual insights, which are integral to achieving high-quality outputs and making data-driven decisions.
Emma, you're absolutely right! Integrating expert insights throughout the iterative process helps produce refined outputs that enhance data quality for better decision-making.
Olivia, I couldn't agree more! Gemini's automated error detection capabilities contribute significantly to the overall data cleaning process, ultimately improving the data quality for downstream analysis.
Alex, Gemini's contribution to the data cleaning process significantly improves the reliability and trustworthiness of the data, ultimately enabling organizations to make more accurate and informed decisions.
Alex, privacy considerations are a crucial aspect in implementing technologies like Gemini for data quality improvement. Organizations must prioritize secure infrastructure and protect user data throughout the process.