Improving Hypothesis Testing in Statistics with ChatGPT: A Powerful Tool for Accurate Analysis
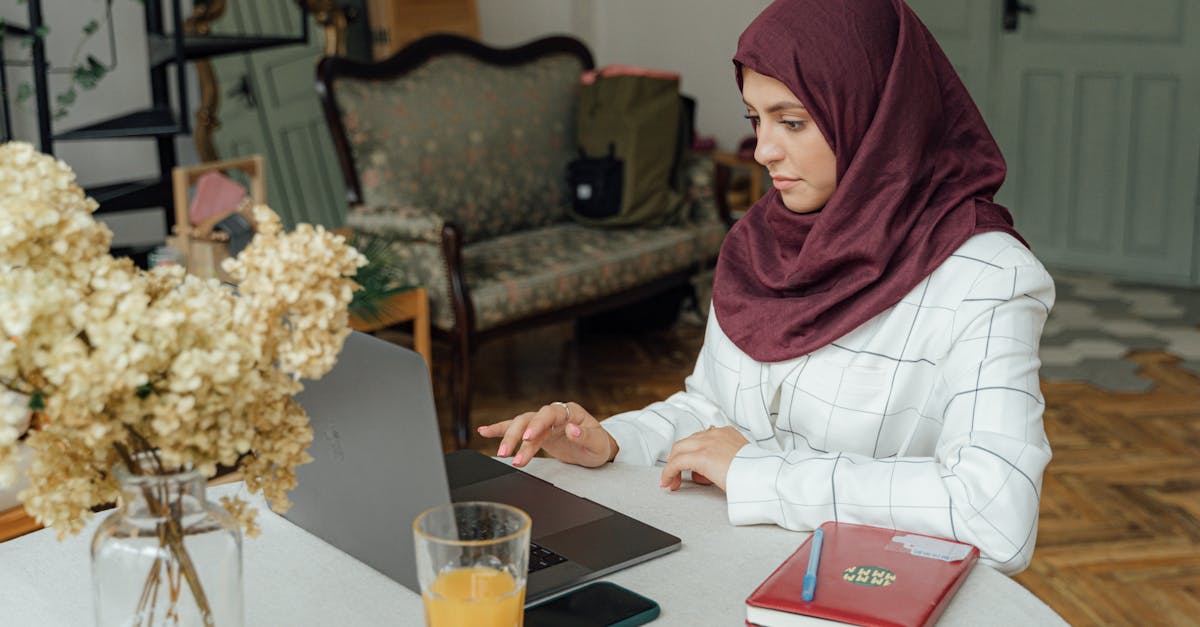
Hypothesis testing plays a crucial role in statistics, allowing researchers to make informed decisions about population parameters based on sample data. The advent of natural language processing and AI has led to the development of ChatGPT-4, an advanced AI chatbot that can provide explanations and guidance on various hypothesis tests.
Hypothesis Testing Overview
Hypothesis testing is used to make inferences about the population based on sample data. It involves formulating a null hypothesis (H0) and an alternative hypothesis (H1). The null hypothesis represents the assumption of no effect or no difference, while the alternative hypothesis suggests the presence of an effect or difference.
The process of hypothesis testing involves several steps:
- Formulate the null and alternative hypotheses
- Select a significance level, denoted as α (e.g., 0.05)
- Collect sample data
- Analyze the data
- Make a decision based on the test statistic and the significance level
- Interpret the results
Hypothesis Tests and their Usage
1. T-Tests
T-tests are used to test the means of two populations or to compare the mean of a single population against a known value. The assumptions include the data being normally distributed and having equal variances for independent samples. ChatGPT-4 can guide users on conducting t-tests, interpreting the results, and identifying appropriate test variants, such as independent samples t-tests or paired samples t-tests.
2. Analysis of Variance (ANOVA)
ANOVA is used to compare the means of more than two groups simultaneously. It is useful when examining the effects of one or more categorical independent variables on a continuous dependent variable. Assumptions for ANOVA include normality of data and homogeneity of variances. ChatGPT-4 can provide explanations on conducting ANOVA, interpreting the results, and addressing post-hoc analysis if required.
3. Chi-Square Tests
Chi-square tests, including the chi-square goodness-of-fit test and the chi-square test of independence, are used to analyze categorical data. The goodness-of-fit test compares observed frequencies with expected frequencies, while the test of independence assesses the relationship between two categorical variables. ChatGPT-4 can assist users in performing chi-square tests, understanding the assumptions, and interpreting the results.
Interpreting Hypothesis Testing Results
Once the hypothesis test is conducted, it is crucial to interpret the results accurately. ChatGPT-4 can provide guidance on understanding p-values, effect sizes, and confidence intervals. It can help users determine whether the results are statistically significant and make informed conclusions about the hypotheses being tested.
In conclusion
With the assistance of ChatGPT-4, researchers and students can gain a better understanding of various hypothesis tests, their assumptions, and the interpretation of their results. Whether it's t-tests, ANOVA, chi-square tests, or other statistical tests, ChatGPT-4 can offer explanations, guidance, and support in conducting statistical analyses accurately and efficiently.
Comments:
Great article, Virginia! I found the idea of using ChatGPT in hypothesis testing quite intriguing. It seems like this tool could really enhance accuracy in statistical analysis.
@Ethan I agree! The potential of using AI to improve hypothesis testing is remarkable. It's exciting to see how technologies like ChatGPT can revolutionize statistical analysis.
As a statistician, I have mixed views on this. While AI tools like ChatGPT might improve accuracy, it's crucial to maintain transparency and interpretability of statistical analysis. How can we address this concern?
@Jack That's a valid concern. Transparency and interpretability are essential in statistical analysis. Perhaps integrating an explanation feature with AI tools like ChatGPT could help address this issue?
@Sophia Integrating an explanation feature sounds promising. It would allow statisticians to understand the reasoning behind ChatGPT's conclusions, ensuring transparency. That would alleviate my concerns to an extent.
@Jack Indeed, the interpretability of AI-driven hypothesis testing is an area that requires further exploration. The explanation feature could address this, but it needs rigorous development and evaluation.
@Sophia Agreed. Explanation features can help control for transparency, but it's also important to understand how ChatGPT processes and weighs the available information to avoid creating additional biases.
@Sophia Completely agree! The key lies in finding a balance between AI-driven analysis and the expertise of statisticians. Integration should aim to enhance accuracy, while human judgment verifies it.
I'm not entirely convinced that AI is the answer to improving hypothesis testing. AI models are trained on data, but data can still be biased. How can we ensure unbiased analysis using ChatGPT?
I see great potential in using ChatGPT for hypothesis testing. It could reduce human error and provide a more standardized approach. However, we must still validate its accuracy against traditional methods.
While ChatGPT seems helpful, we should remember that it's a tool that supports statistical analysis. Proper understanding of statistical concepts and careful analysis is still essential. It shouldn't replace expertise.
AI tools like ChatGPT can definitely enhance hypothesis testing. However, we need to be cautious about over-reliance and ensure that statisticians continue to guide the analysis process.
The idea of using ChatGPT in hypothesis testing is fascinating! It could potentially speed up the analysis process while maintaining accuracy. Virginia, do you have any thoughts on potential limitations?
@Emily Regarding limitations, one important aspect to consider is the generalizability of ChatGPT. It's crucial to train the model on a diverse set of data to avoid bias and improve performance across various domains.
@Virginia Can you provide some insights into the training regimen for ChatGPT in the context of hypothesis testing? How can we ensure it's robust and accurate?
@Ethan To ensure the robustness of ChatGPT, rigorous pre-training and fine-tuning processes are employed. Extensive testing against known statistical problems and benchmark datasets helps ensure accuracy in hypothesis testing.
@Virginia Thank you for the clarification! It's reassuring to know that ChatGPT undergoes rigorous testing and evaluation. The importance of benchmark datasets cannot be overstated.
@Virginia Continuous improvement based on user feedback is a brilliant approach. It ensures that ChatGPT evolves to address real-world challenges and pushes the boundaries of hypothesis testing.
@Emily Another limitation is the potential for ChatGPT to generate responses that appear plausible but lack statistical rigor. Proper evaluation and validation should be conducted to assess its robustness.
@Virginia Thank you for sharing those insights! I agree, a diverse training dataset and robust evaluation are essential to harness the full potential of ChatGPT in hypothesis testing.
ChatGPT can be valuable in hypothesis testing, but we must be cautious about ethical implications. The responsibility lies with us to ensure that AI tools are used ethically and fairly.
Incorporating AI like ChatGPT into hypothesis testing may streamline analyses, but it's important to remember that statistical expertise shouldn't be overlooked. AI is a tool, not a substitute for human judgment.
ChatGPT could have a significant impact on hypothesis testing, but we shouldn't disregard traditional statistical methods. A combined approach, utilizing both AI and human expertise, might yield the best results.
This article is fascinating! The impact that ChatGPT could have on hypothesis testing is immense. I can't wait to see how it develops in the future.
ChatGPT can accelerate hypothesis testing and offer new insights. However, we should be cautious about potential algorithmic biases that could affect statistical analysis. Addressing this issue is crucial for fair and equitable results.