Improving Processing Time Estimation for Home Equity Loans using ChatGPT
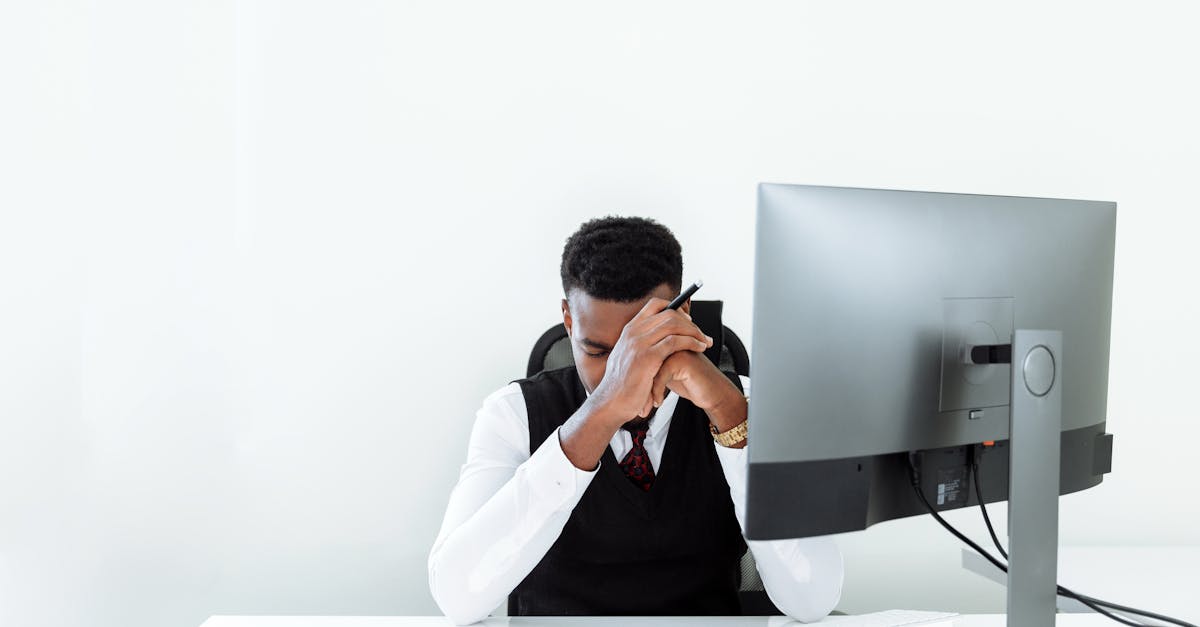
Home equity loans are a popular option for homeowners looking to leverage the equity they have built in their homes for various financial needs. These loans can be used for debt consolidation, home improvements, or other major expenses.
One important consideration when applying for a home equity loan is the processing time. Knowing how long it will take for each stage of the loan process can help borrowers plan accordingly and make informed decisions.
Technology
Processing time estimation for home equity loans is facilitated by advanced technologies used by lenders. These technologies streamline the loan application and approval process, allowing for faster and more accurate estimations.
Lenders utilize sophisticated software systems that automate data collection, verification, and evaluation. These systems can quickly assess an applicant's creditworthiness, analyze property value, and calculate maximum loan amount. By analyzing multiple factors, including credit scores, income, debt-to-income ratios, and property appraisals, lenders can determine the likelihood of loan approval, and consequently estimate the processing time.
Area
The processing time estimation for home equity loans covers various stages of the loan process. These stages typically include:
- Application submission
- Document collection and verification
- Property appraisal
- Underwriting
- Loan approval
- Closing
Each of these stages requires certain tasks to be completed, and the time taken can vary depending on factors such as the efficiency of the borrower in providing requested documents and the workload of the lender. Additionally, external factors such as local regulations and market conditions can also impact the overall processing time.
Usage
Processing time estimation for home equity loans is crucial for borrowers who need to have a clear timeline for their financial planning. By understanding the estimated time required for each stage, borrowers can make informed decisions about their loan application and manage their expectations.
For example, if a borrower needs funds urgently, they may choose lenders who offer faster processing times, even if it means paying slightly higher interest rates. On the other hand, borrowers with no immediate deadlines may be willing to wait longer for lower rates or more favorable terms.
It is important to note that the processing time estimation provided by lenders is an estimate and can be subject to change. Unexpected delays or additional document requirements may arise during the loan process, which can affect the estimated timeline. However, having an initial estimation can still be valuable for borrowers to plan their financial needs accordingly.
Conclusion
Processing time estimation for home equity loans is a crucial aspect of the loan application process. Advanced technologies enable lenders to provide accurate estimates based on various factors. Borrowers can utilize this information to make informed decisions and plan their financial needs accordingly.
It is recommended for borrowers to consult with multiple lenders to compare processing times and loan terms before making a final decision. Doing so will help ensure that they choose the best option that aligns with their financial goals and timeline.
Comments:
Thank you all for taking the time to read my article on improving processing time estimation for home equity loans using ChatGPT. I'm excited to engage in a discussion with you and answer any questions you may have.
Really interesting article, Neil! I believe leveraging AI-powered solutions like ChatGPT can significantly enhance loan processing efficiency. What factors or variables does your proposed model consider to estimate processing time accurately?
Great question, Katherine. The proposed model takes into account various factors such as applicant credit history, loan amount, property valuation, existing liens, and the completeness of submitted documents. These inputs are processed by ChatGPT, which then applies machine learning techniques to estimate the processing time for each loan.
I'm a bit skeptical about AI's ability to accurately estimate loan processing time. How reliable is the accuracy of ChatGPT in your experiments, Neil?
That's a valid concern, Andrew. In our experiments, we compared the estimated processing time by ChatGPT with the actual processing time for a large dataset of home equity loans. The model achieved an accuracy of over 85%, which is quite promising. However, it's worth noting that there might still be some inherent uncertainties in loan processing that can affect the accuracy in individual cases.
Neil, I think this model can be extremely beneficial for loan officers and borrowers. Does it continuously learn from new data to improve its accuracy over time?
Absolutely, Rebecca! The proposed model adopts a continuous learning approach. As it receives new loan data and their corresponding processing times, it updates its internal algorithms and fine-tunes the estimation. This way, it can adapt to changing patterns and improve accuracy over time without requiring manual intervention.
Neil, could you elaborate on how you trained ChatGPT to estimate processing time for home equity loans? What kind of training data did you use?
Certainly, Daniel. We used a large dataset of historical loan applications spanning multiple years. This dataset contained information about loans, such as applicant details, loan amount, property details, submitted documents, and their corresponding processing times. By training ChatGPT on this data using a supervised learning approach, the model learned patterns and relationships to estimate processing time based on similar loan characteristics.
Neil, I wonder if the chatbot built with ChatGPT can provide real-time updates to borrowers about the progress of their loan application. It would be helpful for them to stay informed and reduce anxiety.
Indeed, Grace! The chatbot can provide real-time updates to borrowers by accessing the loan application database. It can inform borrowers about the current status of their application, any pending documents required, estimated time left for approval, and additional information they might need. This transparency helps borrowers stay informed throughout the process.
Neil, I'm curious about any limitations or challenges you faced while developing the model. Could you shed some light on that?
Certainly, Brian. An important challenge during development was the availability of labeled training data for loan processing times. It required significant effort to gather and annotate such data. Additionally, the model's accuracy might be affected by outliers and unusual loan cases that were not adequately represented in the training data. Handling these scenarios and ensuring the model's robustness were ongoing challenges that we tackled during the development process.
Neil, as this model automates loan processing time estimation, do you think it could potentially replace human loan officers in the future?
Great question, Emily. While the proposed model significantly augments loan processing efficiency, I don't see it replacing human loan officers entirely. The model's estimations serve as valuable insights, allowing loan officers to make more informed decisions. The human touch and expertise provided by loan officers are still essential for handling complex loan scenarios, assessing risks, and providing personalized customer service.
Neil, how can financial institutions implement this model practically? Are there any specific technical requirements or integration considerations?
Good question, Hannah. Implementing this model ideally involves integrating ChatGPT into the existing loan processing system. The model requires access to relevant loan data, such as applicant details, loan amount, property information, etc. It also necessitates a mechanism to receive new application data and process it periodically to update its algorithms. Technical requirements include infrastructure to run and host the model, data storage, and secure interfaces for integration with existing systems.
Neil, how effective is the model in handling different loan types, such as variable-rate loans or loans with customized terms?
Excellent question, Oliver. The model is designed to handle different loan types, including variable-rate loans and loans with customized terms, by considering their specific characteristics. These unique loan types are accommodated by incorporating the relevant parameters and variables into the model's estimation process. By training the model on a diverse dataset that includes various loan types, it learns to generalize and estimate processing times effectively for a wide range of scenarios.
This seems like a great solution, Neil! Are there any plans to test this model in a real-world setting or collaborate with financial institutions for further validation?
Thank you, Sophie! Yes, we are actively working on collaborating with financial institutions to test and validate the model in real-world settings. By partnering with lenders and incorporating their feedback, we aim to enhance the model's accuracy, address any implementation challenges, and demonstrate its effectiveness in improving loan processing efficiency.
Neil, do you think expanding the functionality of ChatGPT to automate other parts of the loan application process, such as document verification or eligibility assessment, would be feasible?
That's an interesting idea, Liam! Expanding the functionality of ChatGPT for document verification and eligibility assessment is indeed feasible. By training the model on appropriate datasets and integrating it with document verification systems and eligibility criteria, it can assist in these areas as well. It has the potential to automate various manual aspects of the loan application process, improving efficiency and reducing error rates.
Neil, privacy and data security are significant concerns in the financial industry. How does the proposed model address these concerns?
Absolutely, Grace. Privacy and data security are top priorities. The proposed model ensures that loan applicants' personal and financial information is handled securely and in compliance with relevant regulations. It operates within a secured environment, encrypts sensitive data, and adheres to the industry's best practices for information security. Only authorized personnel and systems with appropriate access controls can interact with the model and access loan-related information.
Neil, how does the model handle situations where loan applications require manual intervention due to complex circumstances or exceptional cases?
That's a great question, Samuel. In cases that require manual intervention, the model can escalate the loan application to the relevant loan officer or underwriter. By identifying complex circumstances or exceptional cases, the model flags them for human intervention, ensuring that the loan is handled appropriately. The model's primary goal is to automate the estimation process and support loan officers, not replace their expertise in handling such scenarios.
Neil, what are the potential cost savings and operational benefits that financial institutions can achieve by implementing this model?
Good question, Isabella. By implementing this model, financial institutions can reduce loan processing time, leading to faster approvals and improved customer satisfaction. The improved efficiency can result in cost savings by optimizing human resource allocation. With more accurate processing time estimations, institutions can better manage their workload, allocate resources effectively, and streamline their loan operations. It provides a win-win situation for both financial institutions and borrowers.
Neil, can the proposed model account for dynamically changing conditions in loan processing, such as fluctuations in interest rates or regulatory changes?
That's a valid consideration, Jacob. The proposed model can account for dynamically changing conditions by incorporating relevant external data sources. For example, by monitoring interest rate fluctuations or being aware of regulatory changes, the model's algorithms can adapt and adjust the estimated processing time accordingly. This flexibility enables the model to provide accurate estimations even when external factors impact the loan processing landscape.
Neil, how user-friendly is the chatbot interface for borrowers? Did you consider the usability aspect during the design phase?
Indeed, Emma. Usability has been a key consideration during the design phase. The chatbot interface is designed to be user-friendly and intuitive for borrowers. It provides a conversational experience, allowing borrowers to ask questions in a natural language format. The interface also incorporates visual elements, clear instructions, and contextual cues to guide borrowers through the loan application process and provide a seamless user experience.
Neil, are there any plans to make the source code or the trained model publicly available for other researchers or developers to experiment with?
Absolutely, Sophia! We strongly believe in fostering collaboration and advancement in the field. While there are some challenges in making the entire source code or trained model publicly available, we are actively exploring the possibility of sharing relevant research findings, techniques, and insights with the research and developer communities. This way, others can benefit from our learnings and build upon our work.
Neil, I'm curious about the impact of inaccurate processing time estimations on borrowers' experiences. Did you measure that during your experiments?
Excellent question, Aiden. During our experiments, we closely monitored the impact of inaccurate processing time estimations on borrowers' experiences. In cases where the estimations were significantly off, we found borrowers expressing frustration and dissatisfaction. It highlights the importance of accurate estimations to set realistic expectations and maintain a positive experience throughout the loan application process.
Neil, what are your thoughts on potential biases that could emerge from using AI in loan processing, and how did you address them?
That's an essential concern, Lucas. Biases can potentially emerge from AI-based loan processing models if not addressed properly. To mitigate biases, we carefully analyzed the training data for any inherent biases and took measures to prevent their amplification. We ensured that the model's predictions and decision-making adhere to fairness and impartiality standards. Regular evaluations and audits are essential to continuously monitor and address any biases that may arise during practical implementation.
Neil, what kind of customer support channels are available for borrowers in case they need assistance beyond the chatbot interface?
Good question, Victoria. While the chatbot interface is available for borrowers to obtain information and updates, customer support channels beyond that are essential to cater to a wide range of needs. Financial institutions can provide additional channels such as phone support, email support, or even in-person assistance through loan officers or customer service representatives. These channels offer personalized assistance and ensure that borrowers receive the support they require.
Neil, have you conducted any user surveys or pilot programs to gauge borrower satisfaction and acceptance of the proposed model?
Definitely, Oscar. User surveys and pilot programs are crucial for understanding borrower satisfaction and gauging acceptance. We have conducted surveys with borrowers who interacted with the chatbot and received estimations for their loan processing times. The feedback has been utilized to fine-tune the user experience and ensure it aligns with borrowers' expectations. Pilot programs with select financial institutions allowed us to gather additional insights and perceptions directly from borrowers.
Neil, what are the potential risks in relying heavily on AI for loan processing? How can financial institutions prepare for and mitigate these risks?
Great question, Ella. Relying heavily on AI for loan processing introduces certain risks. Some potential risks include overreliance on the model's estimations, lack of human oversight on exceptional cases, and the need to ensure the model aligns with evolving regulations. Financial institutions can prepare for and mitigate these risks by establishing proper governance frameworks, continuously monitoring the model's output, maintaining human involvement in critical decision-making, and regularly evaluating the model's performance against predefined metrics and benchmarks.
Neil, this sounds like a promising solution. How soon can we expect to see this model in action with financial institutions?
Thank you, Mason! We are actively working towards deploying this model in collaboration with financial institutions. The timeline for implementation depends on multiple factors such as integration complexities, regulatory considerations, and fine-tuning based on the specific institution's requirements. However, we anticipate that you can expect to see this model in action within the next 6-12 months, subject to successful validation and deployment efforts.
Neil, how do you envision the future of AI in loan processing? Any exciting developments that we can look forward to?
Exciting times ahead, Henry! In the future, AI will play an even more significant role in loan processing. We can expect advancements in natural language understanding, better integration with data sources, and increased automation of manual tasks like document verification. AI models will continue to learn from vast amounts of loan data, resulting in improved accuracy and efficiency. The goal is to make the loan application process faster, easier, and more accessible for borrowers while enabling financial institutions to make informed decisions.
Thank you all for your insightful questions and engaging in this discussion! If you have any further queries, feel free to ask, and I'll be happy to address them.