Improving Retrospective Analysis in Software Product Management with ChatGPT
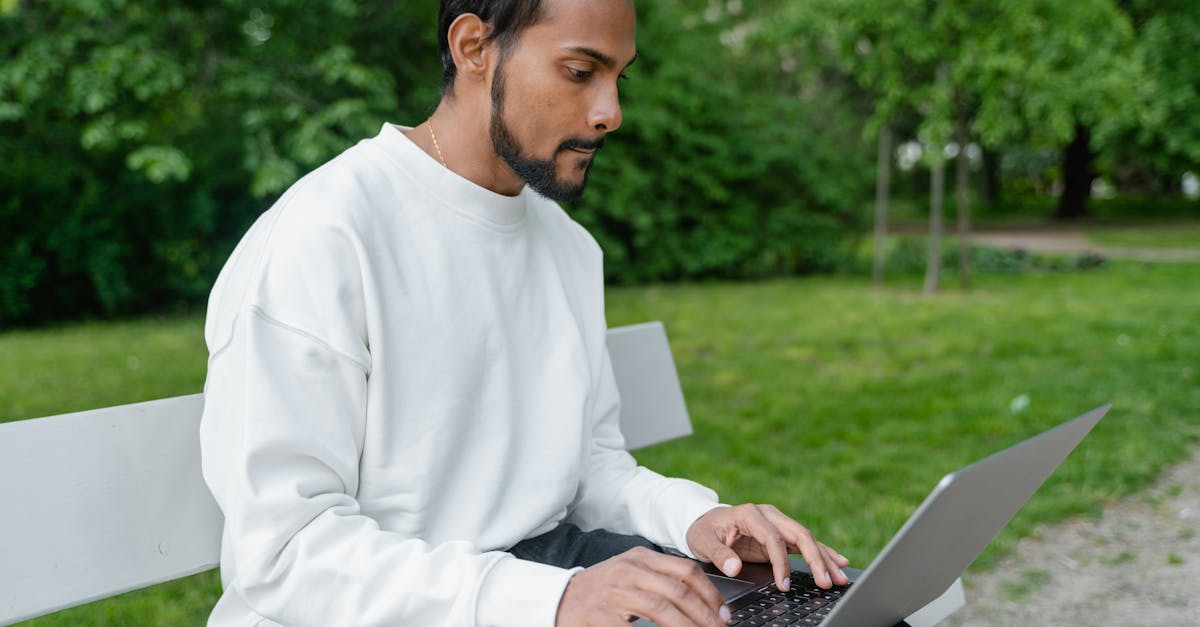
Retrospective analysis is a crucial aspect of the software development process that allows teams to reflect on their performance, identify areas for improvement, and make informed decisions for future sprints. Traditionally, retrospective analysis has been a manual and time-consuming process, requiring team members to manually gather data, analyze it, and generate insights. However, with the advancements in software product management, automation and artificial intelligence (AI) technology, retrospective analysis can now be streamlined and made more efficient.
The Role of Automation in Retrospective Analysis
Automation plays a significant role in simplifying retrospective analysis by eliminating the need for manual data gathering and analysis. With the help of specialized software tools, teams can automatically collect data from various sources, such as project management systems, version control systems, and communication channels. This automated data collection not only saves time but also ensures that all relevant information is captured accurately.
Once the data is collected, software product management tools equipped with retrospective analysis features can analyze the data using AI algorithms. These algorithms can identify patterns, trends, and correlations in the data that may not be apparent to human analysts. By leveraging AI technology, teams can gain deeper insights into their performance and make more informed decisions for future sprints.
AI-Generated Insights for Improved Decision-Making
One of the key advantages of automating retrospective analysis is the ability to generate AI-driven insights. Software product management tools can use AI algorithms to analyze the collected data and generate actionable insights that can help teams identify areas of improvement.
For example, AI algorithms can identify recurring issues or bottlenecks that may have hindered the team's productivity in previous sprints. These insights can help teams address these issues proactively and implement changes to ensure smoother and more efficient future sprints.
Furthermore, AI-driven insights can also help teams identify successful practices or strategies that have led to favorable outcomes in the past. By understanding what worked well, teams can replicate these practices in subsequent sprints and increase the likelihood of success.
Improving Efficiency and Collaboration
Automating retrospective analysis not only improves the accuracy and comprehensiveness of the analysis itself but also enhances overall team efficiency and collaboration. By eliminating the need for manual data gathering and analysis, team members can focus their time and effort on productive tasks, such as implementing improvements and addressing identified issues.
Furthermore, automation enables real-time data collection, analysis, and insights generation. This means that teams can receive immediate feedback on their performance, allowing them to make timely adjustments and improvements.
Moreover, automated retrospective analysis facilitates collaboration among team members. With data readily available and insights generated by software tools, teams can have more informed and productive retrospective discussions. This collaborative approach promotes open communication, fosters a culture of continuous improvement, and empowers teams to work together towards achieving their goals.
Conclusion
Automating retrospective analysis using software product management tools and AI technology offers numerous benefits for software development teams. By streamlining the analysis process, teams can save time, gain deeper insights, and make more informed decisions for future sprints.
Moreover, by automating retrospective analysis, teams can improve overall efficiency, collaboration, and productivity. The ability to generate AI-driven insights allows teams to identify areas for improvement and implement changes that lead to better outcomes.
As software development methodologies continue to evolve, incorporating automation and AI in retrospective analysis will become increasingly important. By embracing these technologies, teams can optimize their development process, enhance team performance, and drive success.
Comments:
Thank you all for taking the time to read and comment on my article. I'm glad to see such an engaged discussion!
I found the idea of using ChatGPT for retrospective analysis really intriguing. It could bring a fresh perspective and help us uncover valuable insights. Great article!
I agree, Lisa. ChatGPT seems like a powerful tool for enhancing retrospective analysis. It could assist in identifying patterns and suggesting improvements based on the conversations. Kudos to the author!
While ChatGPT can be helpful, I have concerns about the potential biases it might introduce. How can we ensure unbiased analysis given that the model's training data could contain biases?
Valid point, Sarah. Bias in the training data could indeed affect the analysis. It's crucial to carefully curate and review the training data to mitigate biases. Additionally, establishing a diverse set of evaluators and having a feedback mechanism can help flag and correct any biases detected.
I see great potential in using ChatGPT for retrospective analysis, but I wonder about the scalability. Does anyone have experience using it with large-scale projects?
Hey Robert, I've tried using ChatGPT for retrospectives on a large-scale project. While it worked well, I'd recommend breaking down the analysis into smaller chunks for better efficiency. Trying to analyze the entire project retrospectively might be overwhelming.
I'm concerned about the potential privacy and security risks associated with using ChatGPT. How can we ensure that sensitive information shared during the retrospective analysis doesn't end up in the wrong hands?
Privacy and security are important considerations, Emily. It's crucial to use a secure platform for implementing ChatGPT, ensuring end-to-end encryption and proper access controls. Anonymizing data and having strict policies in place can further mitigate risks.
I wonder how ChatGPT compares to other AI models when it comes to retrospective analysis. Has anyone conducted a comparative study?
Hi Paul, I haven't seen a direct comparative study, but ChatGPT's ability to engage in dynamic conversations could give it an advantage over other models. It allows for more natural interaction during the analysis.
ChatGPT sounds interesting, but I'm curious if it's easy to implement and integrate into existing retrospective analysis processes.
Hi Nancy, implementing ChatGPT can require some technical expertise, but there are open-source libraries available that can simplify the integration process. It's important to consider the infrastructure and resources needed to support it as well.
Wouldn't using ChatGPT in retrospective analysis lead to biased suggestions if the training data itself contains biases? How can we ensure the suggestions are fair?
That's a valid concern, Robert. One way to tackle this is by incorporating multiple AI models and having a diverse set of evaluators manually review the suggestions. This way, we can ensure fairness and make more informed decisions.
I appreciate the potential benefits ChatGPT brings, but what kind of training data would be required to ensure accurate and relevant analysis?
Good question, Emily. To ensure accurate analysis, the training data should ideally consist of past retrospective discussions, relevant documentation, and user feedback. The more diverse and representative the data, the better the analysis.
ChatGPT could be a valuable addition to the retrospective analysis toolkit. It could help us uncover insights that would otherwise remain hidden. I'm excited to explore its potential!
I understand the benefits, but I worry about the loss of human interaction and intuition that comes with solely relying on AI for retrospective analysis. How do we strike the right balance?
You raise a valid concern, Sarah. While ChatGPT can assist in analysis, human interaction and intuition are indeed crucial. It's important to use AI as a tool to enhance, rather than replace, human involvement in retrospective analysis.
I'm intrigued by the potential of using ChatGPT in retrospective analysis. Has anyone already implemented it in their organization? What were the key learnings?
Hey Paul, we've recently implemented ChatGPT for retrospective analysis. It has helped us uncover new insights and identify improvement opportunities we might have missed. The key learning was to iterate and refine the model based on user feedback continually.
What kind of limitations should we be aware of when using ChatGPT for retrospective analysis?
One limitation is that ChatGPT might generate responses that sound plausible but are factually incorrect. So it's essential to have evaluators who are knowledgeable in the domain to validate the generated suggestions.
ChatGPT can be a powerful tool, but it might be challenging to gain acceptance and trust from the stakeholders. Any advice on how to overcome this?
Building trust is crucial, Emily. It helps to provide stakeholders with insights into the training process and model evaluation. Demonstrating the benefits, starting with smaller pilot projects, and showcasing success stories can also help gain acceptance.
Do you think using ChatGPT for retrospective analysis will require additional training for the team members? How can we ensure everyone can effectively use it?
Training team members on using ChatGPT will be essential, Nancy. Providing workshops, resources, and clear guidelines can ensure effective and consistent usage. It's also crucial to offer ongoing support and address any questions or concerns that arise.
What potential challenges do you foresee when implementing ChatGPT for retrospective analysis, and how can we overcome them?
One challenge could be the need for substantial computational resources, especially when dealing with large-scale projects. Overcoming this would involve careful infrastructure planning and optimizing the system for efficiency.
Another challenge could be effectively handling diverse user inputs while maintaining consistency. Developing a robust dialogue management system and continuously refining it based on user feedback can help address this challenge.
Are there any ethical considerations we should keep in mind when using ChatGPT for retrospective analysis?
Ethical considerations are crucial, Robert. We need to ensure informed consent from all participants and be transparent about the use of AI in retrospective analysis. Additionally, safeguarding user data and addressing any biases are vital aspects to consider.
I'm curious if ChatGPT can handle non-English conversations effectively. Would it be suitable for retrospective analysis in diverse international teams?
Great question, Lisa. While ChatGPT performs well in English conversations, it might face challenges with non-English languages and specific cultural nuances. Adapting ChatGPT or exploring language-specific models could be necessary to make it more suitable for diverse international teams.
How can we evaluate the effectiveness and accuracy of ChatGPT's retrospective analysis?
Validating the effectiveness and accuracy of ChatGPT's retrospect analysis can be done through user feedback and evaluations from domain experts. Comparing its suggestions to those derived from traditional analysis methods can also help establish the model's accuracy.
What kind of computational resources would be required to implement ChatGPT in retrospective analysis?
Robert, implementing ChatGPT would likely require a reasonably powerful hardware setup, including GPUs or TPUs for efficient training and inference. Additionally, having sufficient storage capacity to handle large amounts of data and model checkpoints is crucial.
Does ChatGPT's performance improve with more training data, or is there a saturation point?
Increasing the training data generally improves ChatGPT's performance up to a certain point. However, once the model reaches a saturation point, additional training data might provide diminishing returns. It's important to find the right balance between the training dataset size and performance.
Apart from retrospective analysis, can ChatGPT be used for any other software product management tasks?
Absolutely, Sarah! ChatGPT's conversational abilities can be applied to various software product management tasks, such as requirements gathering, customer support, and even ideation and brainstorming sessions.
What are the potential risks or challenges when using AI models like ChatGPT in software product management, and how can we mitigate them?
Using AI models like ChatGPT in software product management introduces risks such as biased analysis, potential security vulnerabilities, and overreliance on AI suggestions. Mitigating them requires robust bias detection mechanisms, secure implementations, and maintaining a healthy balance between human and AI involvement.
Would using ChatGPT in retrospective analysis make the process more time-consuming, given the back-and-forth conversations?
Jane, while the back-and-forth conversations may extend the analysis process slightly, the benefits gained from the depth of insights and engagement provided by ChatGPT often outweigh the additional time investment.
What kind of model fine-tuning or customization is necessary to ensure ChatGPT aligns well with a specific organization's needs and terminologies?
Fine-tuning ChatGPT on a specific organization's data and providing it with domain-specific terms and examples can align the model better with the organization's needs. Continuous refinement and feedback loops can further ensure the model's effectiveness in understanding the organization's context.
I'm excited about the potential of using ChatGPT in retrospective analysis. It could revolutionize how we gather insights and drive improvements. Looking forward to exploring it further!