Improving Toxicity Prediction in Cell Based Assays: The Power of ChatGPT
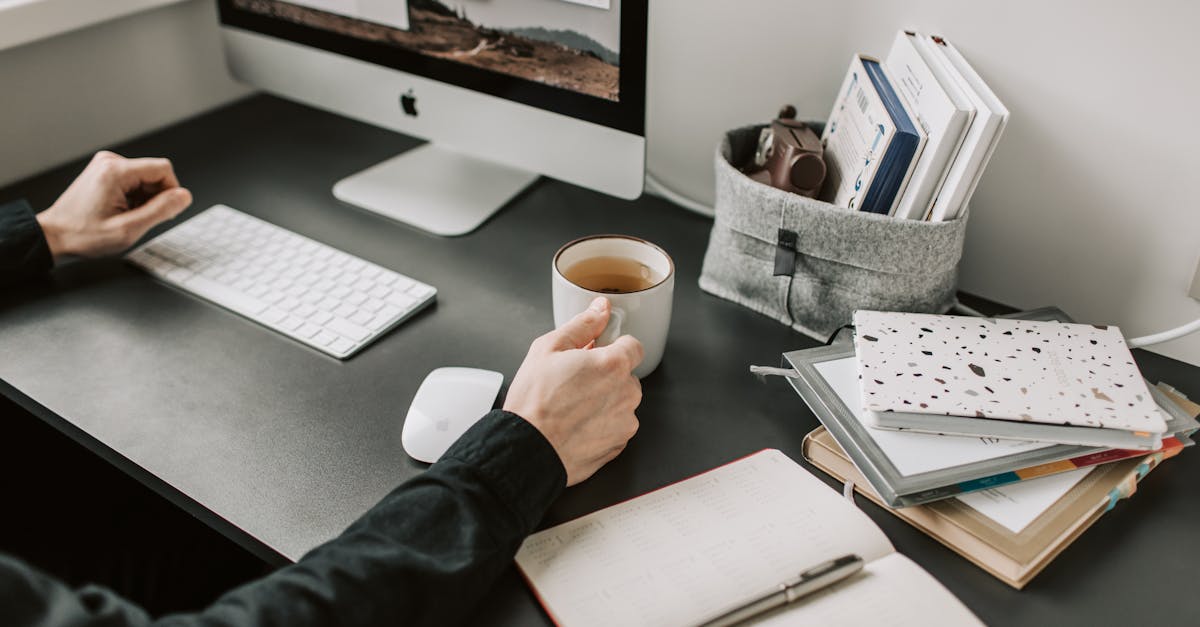
Cell-based assays have revolutionized the field of toxicity prediction by providing a reliable and efficient method to assess the potential adverse effects of chemical compounds. One such assay, Chargpt-4, has emerged as a powerful tool for predicting toxicity based on available data derived from various cell-based experiments.
The field of toxicity prediction plays a critical role in drug discovery, environmental risk assessment, and chemical safety. Traditional methods of toxicity testing, such as animal studies, are time-consuming, expensive, and often associated with ethical concerns. In contrast, cell-based assays offer a faster, cost-effective, and more ethical alternative for toxicity screening.
What are Cell-Based Assays?
Cell-based assays involve exposing cultured cells to different chemical compounds and observing their response. These assays are designed to mimic the complex interactions between the compound and living cells, providing valuable insights into the compound's toxicity profile. They can evaluate a wide range of cellular endpoints, including cell viability, apoptosis, genotoxicity, and oxidative stress, to name a few.
Chargpt-4 is an advanced cell-based assay that utilizes predictive modeling techniques to estimate the toxicity of chemical compounds. By analyzing a wide range of cellular data gathered from previous experiments, it can generate toxicity predictions for new compounds. This allows researchers to prioritize their resources, focus on compounds with potentially lower toxicity, and reduce the need for extensive in vivo testing.
How Does Chargpt-4 Work?
Chargpt-4 uses machine learning algorithms and predictive modeling to analyze the large dataset derived from cell-based assays. The dataset consists of information on the cellular response to thousands of chemical compounds, including their concentration, exposure time, and resulting toxicity levels.
By identifying patterns and correlations within the dataset, Chargpt-4 can create a predictive model capable of estimating the toxicity of new compounds. The model takes into account various cellular endpoints and their relationship to toxicity. Given the characteristics of a new compound, such as its structural features and physicochemical properties, Chargpt-4 can generate a toxicity score or classification.
Benefits of Using Chargpt-4
Chargpt-4 offers numerous benefits in the field of toxicity prediction:
- Efficiency: By utilizing existing data, Chargpt-4 provides a rapid and efficient method for toxicity prediction, saving time and resources.
- Cost-effectiveness: Cell-based assays are generally less expensive than traditional animal studies, and Chargpt-4 further reduces the need for extensive in vivo testing.
- Ethical considerations: The use of cell-based assays eliminates the need for animal testing, addressing ethical concerns and promoting the principles of 3Rs (Replace, Reduce, Refine).
- Improved compound prioritization: Chargpt-4 allows researchers to focus their efforts on compounds with potentially lower toxicity, reducing the likelihood of late-stage drug failures and improving overall success rates in drug discovery.
- Accessible technology: Chargpt-4 is designed to be user-friendly and can be easily integrated into existing toxicity prediction workflows.
Limitations and Future Scope
While Chargpt-4 offers promising results in toxicity prediction, it does have some limitations. The accuracy of its predictions heavily depends on the quality and diversity of the dataset used to train the model. Additionally, it may not account for potential interactions between different compounds, which are crucial in complex biological systems.
In the future, it is crucial to expand the dataset used by Chargpt-4 and incorporate more diverse cellular endpoints. This will enhance the accuracy and reliability of the toxicity predictions. Additionally, integrating Chargpt-4 with other computational models and experimental methods can provide a comprehensive approach to toxicity prediction.
Conclusion
Cell-based assays, such as Chargpt-4, have revolutionized toxicity prediction by providing a faster, cost-effective, and ethical alternative to traditional methods. By leveraging available data from cell-based experiments, Chargpt-4 can generate toxicity predictions for new compounds, aiding in compound prioritization and reducing the need for extensive in vivo testing. While there are limitations to this technology, ongoing advancements hold great promise for improving the accuracy and reliability of toxicity predictions.
Comments:
This article on improving toxicity prediction using ChatGPT is fascinating! It's amazing to see how artificial intelligence can enhance research and make predictions more accurate.
I agree with you, Sophia. The potential of ChatGPT in improving toxicity prediction in cell-based assays is really impressive. It could pave the way for more efficient drug discovery and development processes.
Absolutely, Sophia and Michael! The power of AI in assisting scientific research is undeniable. I'm excited to see how ChatGPT can contribute to further advancements in toxicology studies.
While the use of AI like ChatGPT offers exciting possibilities, it's essential to ensure that these predictive models are validated thoroughly to avoid any potential drawbacks or false positives. Rigorous testing should be a priority.
Thank you all for your comments and insights. I completely agree that validation and rigorous testing are fundamental when utilizing AI models like ChatGPT. We must ensure their reliability in toxicity prediction.
I find it fascinating how ChatGPT can potentially improve the accuracy of toxicity predictions. It could lead to a significant reduction in the need for extensive lab-based experiments. This can save time, money, and minimize animal testing.
Absolutely, Emily! AI-powered models like ChatGPT could revolutionize toxicology studies by reducing the reliance on traditional experiments, ultimately reducing ethical concerns related to animal testing.
Exactly, Emily and Sophia. The potential to reduce animal testing while improving the accuracy of toxicity predictions is a huge step forward. It's crucial to continue exploring AI's role in advancing scientific research.
I couldn't agree more, Michael. The ethical implications of reducing animal testing while achieving better predictions make the integration of AI models like ChatGPT incredibly promising.
I appreciate the potential benefits discussed here, but we must also remain cautious. AI models, though powerful, can still produce false positives or overlook important factors in toxicity prediction. Proper validation and human supervision are vital.
You raise an essential point, Oliver. The interpretability and transparency of AI models like ChatGPT should be prioritized. Human supervision and validation must go hand in hand to ensure the accuracy and reliability of toxicity predictions.
Absolutely, Thomas! Incorporating human expertise and judgment is crucial to make AI models like ChatGPT reliable and accountable. It's a collaborative effort to harness the full potential of this technology.
AI-powered models can indeed enhance efficiency and reduce the need for extensive lab-based experimentation. However, we should also consider the potential limitations of these models in capturing all the complexities of biological systems. They are tools to assist, not replace, scientific expertise.
Absolutely, Chloe. AI models are valuable tools to augment scientific capabilities, but they should never replace domain expertise, especially when dealing with complex biological systems. The collaboration between AI and human experts is the way to go.
Well said, Michael. The synergy between AI and human expertise is the key to unlock the full potential of these models in improving toxicity prediction and having practical applications in drug discovery.
True, Michael. The knowledge and experience human experts bring to the table are invaluable. By combining AI and human expertise effectively, we can advance both the accuracy and efficiency of toxicity prediction.
It's interesting to see the potential benefits AI offers in toxicity prediction. However, we must also acknowledge the challenges of training AI models on large and diverse datasets. Ensuring data quality is vital to avoid biases in predictions.
Absolutely, Daniel. High-quality and diverse datasets are essential to train AI models effectively. Careful consideration should be given to data collection, curation, and addressing any inherent biases that might arise.
You make a valid point, Daniel. AI's effectiveness in toxicity prediction heavily depends on the quality and representativeness of the data used for training. Bias mitigation and diversity in datasets should be a priority for reliable predictions.
I'm excited about how AI models like ChatGPT can improve the efficiency of toxicity prediction, especially in the early stages of drug discovery. It can help scientists prioritize resources and focus on the most promising compounds.
Absolutely, Jennifer. Streamlining the drug discovery process is crucial to accelerate advancements in medicine. AI models like ChatGPT can certainly play a significant role in achieving that goal.
Indeed, Jennifer! The ability to prioritize compounds based on toxicity prediction can save valuable time and resources, enabling faster identification of potentially safe and effective drugs.
It's exciting to think about the impact that AI tools like ChatGPT can have on drug discovery processes. The potential for faster and more accurate predictions is a game-changer.
Indeed, Sophia. The efficiency enhancements offered by AI models like ChatGPT can lead to significant time and cost savings in drug discovery, allowing us to focus resources on the most promising candidates.
AI models like ChatGPT can also play a critical role in knowledge discovery and hypothesis generation. By analyzing vast amounts of data, they can identify patterns and relationships that may not be apparent to human researchers alone.
That's a great point, Chloe. AI models have the potential to augment human creativity by uncovering hidden insights and suggesting new research directions. It's an exciting time for scientific discovery!
Absolutely, Sophia. The combination of AI's data analysis capabilities and human ingenuity can lead to breakthrough discoveries and advancements in the field of toxicology.
I completely agree, Daniel. AI models like ChatGPT can complement human expertise by processing and analyzing vast amounts of data, accelerating the pace of scientific progress.
It's incredible how AI models can assist scientists in exploring new research directions and making significant discoveries. The potential to uncover novel insights is truly promising!
I'm curious, how can we ensure the transparency of AI models like ChatGPT? Understanding their decision-making process is crucial, especially in an area as critical as toxicity prediction.
Transparency is indeed a key concern, Daniel. Techniques such as interpretability algorithms can help shed light on the decision-making process of AI models, enabling scientists to trust their predictions.
I agree, Sophia. Interpretable AI models can ensure that scientists understand how and why predictions are made, making the models more trustworthy and facilitating their integration into practical applications.
Transparency and interpretability are crucial if AI models like ChatGPT are to gain widespread acceptance and adoption in the scientific community. We need to build trust in their capabilities.
I couldn't agree more, Sophia. Trust is essential, and transparency is a significant factor in establishing that trust. It allows researchers to understand and verify the predictions made by AI models.
Transparency also helps in identifying potential biases or limitations in AI models. It allows for continuous improvement and safeguards against unwarranted risks posed by biased predictions.
Well said, Jennifer. Identifying and addressing biases is essential to ensure that the predictions made by AI models like ChatGPT are fair, reliable, and applicable across diverse populations and experimental conditions.
I'm excited to see how the conversation around AI's transparency and interpretability progresses. It's a crucial aspect of the ethical and responsible deployment of AI in scientific research and decision-making processes.
Absolutely, Chloe. The ethical considerations surrounding AI's transparency will significantly shape how these models are adopted and trusted within the scientific community.
As AI models like ChatGPT become more integrated into scientific research, discussions around ethics and transparency need to be ongoing, ensuring responsible and accountable use of these technologies.
Thank you all for sharing your valuable insights and engaging in this discussion. Ensuring the ethical use of AI models like ChatGPT is crucial for the progress of scientific research.