Improving User Experience Testing with ChatGPT: Exploring its Potential in A/B Testing
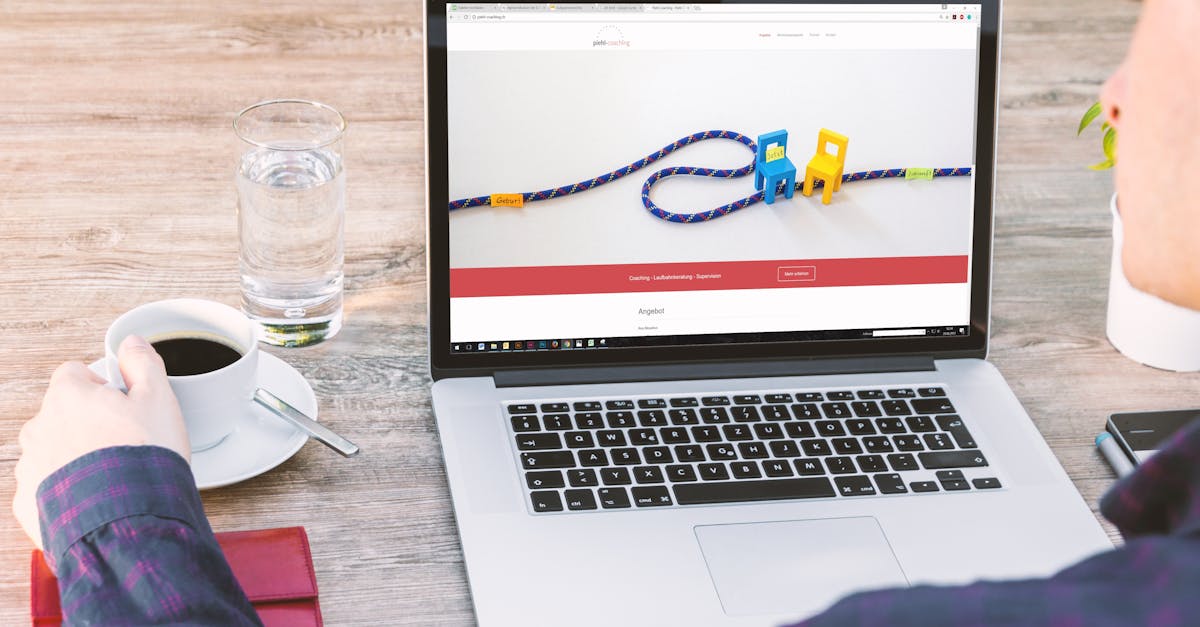
In the world of digital product development, user experience testing plays an integral role in understanding user behavior and preferences. One popular technique for evaluating the effectiveness of different design options is A/B testing.
The Significance of A/B Testing
A/B testing is a statistical method used to compare two or more versions of a website or application. It involves presenting different variations (designs, features, or content) to different users to determine which version performs better in terms of predefined goals such as click-through rates, conversions, or user satisfaction.
This method allows developers to make data-driven decisions based on real user behavior and preferences. By comparing user responses to different design elements, developers gain insights that can inform future iterations and improvements.
Introducing ChatGPT-4
ChatGPT-4, developed by OpenAI, is an advanced language model that utilizes artificial intelligence to generate human-like conversational responses. Its capabilities span a wide range of applications, including content creation, customer service, and now, user experience testing.
How ChatGPT-4 Enhances A/B Testing
Traditionally, conducting A/B tests required real users to interact with different versions of a product. This process can be time-consuming, expensive, and prone to bias, as users may have inherent preferences or exhibit behavior influenced by factors outside the testing environment.
ChatGPT-4 offers an innovative solution by simulating user behavior and generating unbiased feedback. By leveraging its natural language processing capabilities, developers can utilize ChatGPT-4 to simulate the actions and preferences of thousands of potential users, allowing for comprehensive A/B tests without the need for extensive user recruitment.
Implementation Example
Let's consider a hypothetical scenario where a development team wants to test two different designs for a chat feature on their website. They can use ChatGPT-4 to generate simulated interactions between users and the chat feature for both designs.
The team can set predefined metrics like user satisfaction, response time, or completion rate, and compare the results between the two designs. This data-driven approach provides valuable insights into which design performs better, enabling developers to make informed decisions for optimizing the chat feature in future iterations.
Conclusion
With the advent of AI-powered language models like ChatGPT-4, A/B testing becomes more efficient, cost-effective, and unbiased. Leveraging the power of machine learning, developers can generate simulated user behavior and preferences, offering valuable insights for optimizing digital products.
As the field of user experience testing continues to evolve, ChatGPT-4 presents an exciting opportunity to streamline the A/B testing process and enhance decision-making in digital product development.
Comments:
Great article! I've always been curious about ChatGPT's potential in A/B testing.
I agree with Emma, this article explores an intriguing topic.
Mark, what specific part of the article intrigued you the most?
I've used ChatGPT in user experience testing before, and it has shown promising results.
Thank you for your positive feedback, Emma, Mark, and Sophia! I'm glad you find the topic interesting.
User experience testing is crucial in product development. Excited to learn more about ChatGPT's potential.
I've never used ChatGPT for A/B testing, but I'm curious to see how it performs compared to traditional methods.
Interesting article! The advancement of AI in testing methodologies is fascinating.
Thank you, Robert, Nicole, and Chris, for your comments! I appreciate your interest in the topic.
As a UX designer, I'm looking forward to incorporating ChatGPT into our testing processes.
How does ChatGPT handle non-textual elements in user experience testing?
Hi Jason! ChatGPT primarily focuses on generating and understanding text-based responses, but it can still provide valuable insights in testing user experiences that involve textual interactions.
This article is well-researched and presents a fresh perspective. Kudos to the author!
I enjoyed reading this article. It's thought-provoking and well-written.
I'm curious to know if ChatGPT can adapt to different user demographics in A/B testing.
Hello Emily, Maria, and Michael! Thank you for your kind words. To address Michael's question, ChatGPT can be fine-tuned to understand and respond to different user demographics, which can enhance the A/B testing process.
ChatGPT seems like a useful tool to gather user feedback and improve UX design.
I wonder if ChatGPT can effectively evaluate the emotional response of users in A/B testing.
As an AI enthusiast, I find the potential of ChatGPT in A/B testing extremely intriguing.
Thanks, Rebecca, Sarah, and Daniel, for sharing your thoughts! ChatGPT can indeed analyze the emotional response of users through text, enabling valuable insights for A/B testing.
I'm excited to see how ChatGPT could enhance our usability testing process.
This article provides an interesting use case for ChatGPT. I'm intrigued by its potential.
Has ChatGPT been extensively tested in real-world A/B experiments?
Hi Melissa, Andrew, and Olivia! I appreciate your enthusiasm. ChatGPT has been used in various real-world A/B experiments, proving its efficacy in improving user experience.
This article highlights the importance of integrating AI in user experience testing.
Do you think ChatGPT has the potential to replace human testers in the future?
Hello Jennifer and Samuel! AI like ChatGPT can enhance user experience testing, but it's unlikely to completely replace human testers. Human judgment and qualitative insights are still valuable in the testing process.
I've used ChatGPT in our A/B testing, and it significantly improved our testing efficiency.
Can ChatGPT be generalized across different industries or is it more specialized for certain sectors?
The potential of AI in A/B testing is exciting. ChatGPT could revolutionize the way we gather user insights.
Thanks, James, Mia, and George, for your comments! ChatGPT can be applied across various industries, although fine-tuning it to specific sectors can yield better results.
Would ChatGPT only be used for A/B testing, or could it provide insights in other testing methodologies as well?
I wonder if ChatGPT can also provide automated suggestions for A/B testing variables.
Hello David and Emily! ChatGPT can certainly be used in other testing methodologies apart from A/B testing. It can also assist in generating automated suggestions for testing variables, saving time and effort.
I'm excited about the possibilities ChatGPT offers in improving user experiences.
Has ChatGPT been benchmarked against other user testing tools to assess its performance?
Using ChatGPT in user experience testing sounds intriguing. I'd like to explore its potential further.
Thanks, Matthew, Sophie, and Emma, for your input! ChatGPT has indeed been benchmarked against other user testing tools, demonstrating its competitive performance.
I'm excited about the era of AI in user experience testing. ChatGPT can be a game-changer.
I wonder if ChatGPT has any limitations or challenges in A/B testing.
Hello Thomas and Lily! ChatGPT has its limitations, such as potential biases and difficulties in handling non-textual elements. However, these challenges can be mitigated with careful fine-tuning and human oversight.
Duncan, could you share any examples where ChatGPT's adaptability to different user demographics has been proven?
Duncan, do you think AI like ChatGPT will become a standard tool for user testing in the future?
Duncan, could you elaborate on how ChatGPT analyzes emotional responses through text-based interactions?
I'm particularly intrigued by the potential of ChatGPT to improve personalization in user experiences.
Can ChatGPT assist in generating hypotheses for testing, or is it primarily focused on analyzing the outcomes?
David, I believe ChatGPT is primarily focused on analyzing outcomes rather than generating initial hypotheses.
Emily, I see. It would be interesting if ChatGPT could assist in generating hypotheses as well, but its analysis capabilities are still valuable.
Could you provide insights into the fine-tuning process for ChatGPT for different user demographics?
If ChatGPT can be fine-tuned for specific sectors, how much additional effort does that require?