Interpreting Petroleum Geology: Leveraging ChatGPT for Technological Advancements
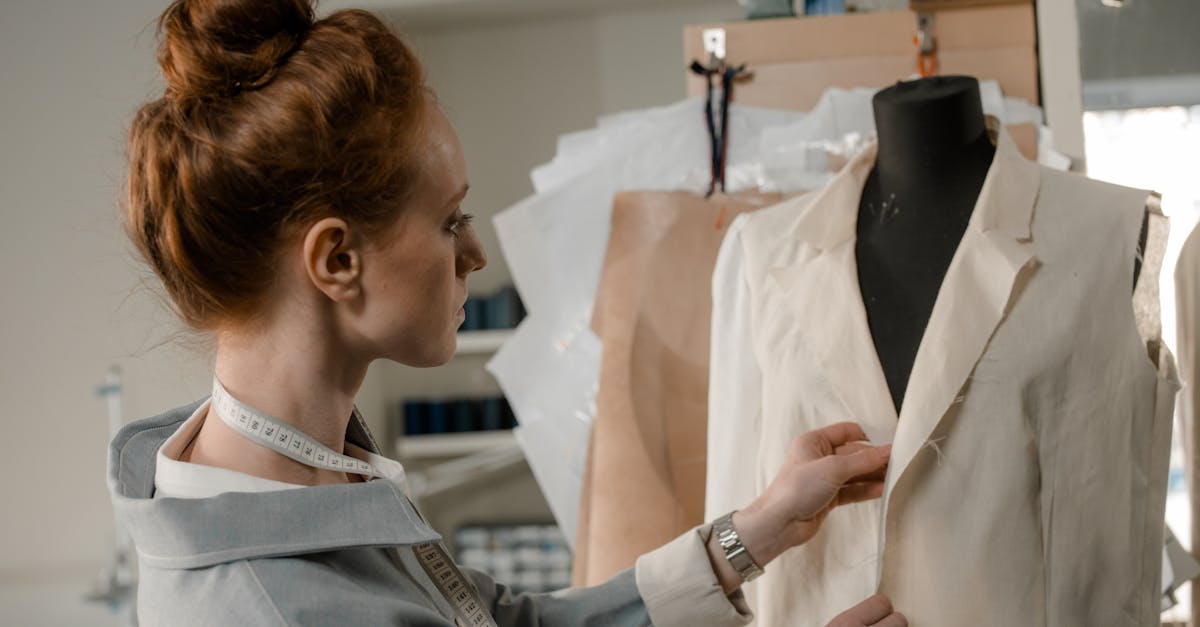
Petroleum geology plays a crucial role in the exploration and production of oil and gas resources. One of the important aspects of this field is reservoir characterization, which involves the assessment and understanding of the properties of subsurface reservoirs that hold hydrocarbons. With advancements in technology, the data interpretation and integration process has been significantly enhanced. One such technology is GPT-4, which can greatly assist in reservoir characterization.
GPT-4: A Game-changer in Reservoir Characterization
GPT-4, short for Generative Pre-trained Transformer-4, is an advanced machine learning model that has revolutionized data interpretation and integration in petroleum geology. It is designed to provide a comprehensive understanding of the reservoir's properties, leading to improved decision-making in exploration and production operations.
The usage of GPT-4 in reservoir characterization can bring several benefits. Firstly, it can handle large volumes of diverse data from multiple sources, such as well logs, seismic data, and production data. This enables geoscientists and engineers to integrate different datasets, allowing for an accurate and holistic view of the reservoir.
Secondly, GPT-4 employs deep learning techniques to identify patterns and relationships within the data. By analyzing the complex interplay between various reservoir properties, it can generate valuable insights into the distribution of hydrocarbons, reservoir connectivity, and rock properties. This information is essential for optimizing production strategies and maximizing recovery rates.
Furthermore, GPT-4 can facilitate the prediction of reservoir properties in areas with limited data availability. By leveraging its pre-trained models, it can extrapolate knowledge and fill in data gaps, enabling efficient decision-making even in challenging scenarios.
Integration of GPT-4 in Reservoir Characterization Workflows
The integration of GPT-4 into reservoir characterization workflows typically involves several steps. Firstly, the relevant data points are collected and pre-processed to ensure consistency and quality. This includes handling missing data, standardizing units, and removing outliers.
Once the data is prepared, it is fed into the GPT-4 model for analysis. The deep learning algorithms in GPT-4 are then applied to identify patterns, correlations, and trends within the dataset. This process helps in generating reliable predictions and interpretations of reservoir properties.
The outputs from GPT-4 are typically visualized using advanced data visualization tools. Geoscientists and engineers can explore the results through interactive maps, cross-sections, and 3D models. This visualization aids in the identification of potential drilling targets, areas of interest for production optimization, and the overall understanding of the reservoir.
Conclusion
The integration of GPT-4 in reservoir characterization workflows has significantly improved the understanding of petroleum reservoirs. By effectively interpreting and integrating diverse datasets, GPT-4 enables geoscientists and engineers to make informed decisions in exploration and production operations. With the ability to handle complex data and generate valuable insights, GPT-4 is indeed a game-changer in the field of petroleum geology.
Comments:
Thank you all for reading my article on 'Interpreting Petroleum Geology: Leveraging ChatGPT for Technological Advancements.' Feel free to share your thoughts and ask any questions!
Great article, Iain! I found the application of ChatGPT in petroleum geology quite fascinating. It seems like an innovative approach to interpreting complex geological data.
Thank you, Michael! ChatGPT indeed opens up new possibilities for analyzing and making sense of vast amounts of geological data. Do you see any specific challenges or limitations in its implementation?
I could envision potential challenges in accurately training the model with domain-specific terminology and geological concepts. Also, the model's interpretation may lack the subtleties and nuances that experienced human geologists bring. But overall, it's an exciting advancement!
As a petroleum geologist myself, I appreciate the exploration of new technologies in our field. The ability to leverage ChatGPT for interpreting petroleum geology has the potential to greatly enhance our understanding and decision-making. Well done, Iain!
Thank you, Emily! I'm glad to hear your positive feedback. In your experience, what geological challenges do you think ChatGPT could effectively address?
One specific challenge is accurately identifying subsurface structures and potential hydrocarbon reservoirs from seismic data. With the ability to leverage ChatGPT's interpretation, this process could be more efficient and accurate.
Absolutely, Emily! The integration of ChatGPT with geophysical data analysis holds great promise in improving our subsurface understanding. Do you think there are any limitations or risks associated with this technology?
While ChatGPT can provide valuable insights, it's important to remember that it's a tool, not a replacement for human expertise. Overreliance on the model without critical human validation could lead to errors. Proper collaboration between geologists and AI is key.
I must say, Iain, your article left me intrigued. As an outsider to the field, could you explain how ChatGPT is trained to interpret petroleum geology? Is it purely based on labeled data?
Good question, James! ChatGPT is trained using a combination of supervised fine-tuning and Reinforcement Learning from Human Feedback (RLHF). Initially, it is pretrained on a large corpus of internet text, and then fine-tuned on specifically generated geological data with the help of human geologists.
That sounds like a robust training approach, Iain. It's fascinating how the model learns from both general internet knowledge and domain-specific experts. Thanks for the clarification!
I find the concept of ChatGPT in petroleum geology intriguing. However, what measures are in place to ensure the model's interpretations align with scientific accuracy? Can it be easily biased?
Valid concern, Sophia. During fine-tuning, the model is supervised by human experts who guide it towards scientifically accurate interpretations. Bias can be a challenge, but efforts are made to reduce it through careful data selection and active moderation. Regular audits and feedback help maintain quality.
Thanks for addressing that, Iain. It's crucial to have scientific accuracy and minimize biases in AI systems. I'm glad to know there are processes in place to ensure that!
I have mixed feelings about this. While ChatGPT seems promising, relying too much on AI for interpreting geological data might diminish the role of human expertise and the art of interpretation. How do you feel about that, Iain?
I understand your concerns, Daniel. It's essential to strike a balance between AI assistance and human expertise. ChatGPT should serve as a tool to augment and streamline our work, empowering geologists rather than replacing them. Human interpretation and judgment remain invaluable.
I couldn't agree more, Iain. Technology should always be seen as a tool to enhance human capabilities rather than a substitute. Thanks for your insights!
This is an exciting advancement! I can see how ChatGPT can revolutionize the industry. It opens up possibilities for faster and more accurate geological interpretations. Well done on your article, Iain!
Thank you, Rebecca! I appreciate your enthusiasm. Indeed, with the power of ChatGPT, we can unlock new efficiencies and insights in petroleum geology. Do you foresee any potential ethical considerations in its application?
Absolutely, Iain. Ethical considerations, such as data privacy, transparency, and unbiased decision-making, need to be carefully addressed to ensure responsible use of technology. Regular audits and accountability measures should be in place.
Well said, Rebecca. Ethical aspects cannot be overlooked. Responsible implementation and continuous evaluation of the technology are vital. Thank you for highlighting these important considerations!
Excellent article, Iain! What impact do you think ChatGPT will have on the industry in terms of cost-effectiveness and exploration success rates?
Thank you, Adam! ChatGPT has the potential to significantly reduce the time and cost involved in geological interpretations. By assisting geologists in analyzing vast amounts of data and providing valuable insights, it can improve exploration success rates and optimize resource allocation.
That's impressive, Iain! Cost reduction and improved success rates are always welcome in our industry. Looking forward to seeing the continued advancements in this technology!
ChatGPT's potential in petroleum geology is remarkable. I wonder if it can also assist in predicting and mitigating geological hazards like earthquakes or subsidence?
Interesting question, Ava. While ChatGPT may not directly assist in hazard prediction, its ability to analyze and interpret geological data can indirectly contribute to enhancing our understanding of geological processes and identifying indicators of potential hazards.
That makes sense, Iain. Leveraging the model for deeper insights into geological processes could indeed have broader implications in hazard prevention. Thank you for sharing your insights!
Kudos on the article, Iain! It's fascinating to see how AI technologies like ChatGPT continue to advance different fields. Exciting times ahead!
Thank you, Oliver! Indeed, it's an exciting time with the continuous advancements in AI technologies. The potential for ChatGPT to transform the way we interpret petroleum geology is full of promise!
I enjoyed reading your article, Iain! From an environmental perspective, do you think ChatGPT can contribute to better decision-making in terms of sustainable practices and minimizing environmental impact?
Thank you, Ella! ChatGPT can certainly play a role in promoting better decision-making when it comes to sustainable practices. By providing more accurate insights, it can help optimize drilling locations, reduce environmental impact, and support responsible resource extraction.
That's great to hear, Iain! Leveraging technology for sustainable practices and environmental stewardship is crucial. Integrating ChatGPT in such decision-making processes can make a positive difference!
Well done, Iain! The potential of ChatGPT in petroleum geology is undeniable. It's amazing how AI continues to shape various industries. Looking forward to witnessing its practical implementation!
Thank you, Lucas! AI, including ChatGPT, holds immense potential in revolutionizing petroleum geology. As advancements continue, we'll see how this technology transforms the industry and enhances our understanding of subsurface systems!
Very informative article, Iain! The combination of AI and geology is fascinating. How do you foresee the role of AI models evolving in the future, specifically in petroleum geology?
Thank you, Freya! In the future, AI models like ChatGPT could become increasingly sophisticated and encompass a broader range of geological tasks, from automated data interpretation to real-time drilling optimization. They will augment our capabilities and drive further discoveries.
That's exciting, Iain! The potential for AI models to automate and optimize various geological tasks is truly promising. I look forward to witnessing their evolution in the industry!
Interesting article, Iain! How do you think ChatGPT can assist in improving the accuracy of resource reserve predictions? Can it account for uncertainties?
Good question, Ivan! ChatGPT can assist in refining resource reserve predictions by considering and analyzing multiple data sources. While it can account for known uncertainties, it's important to note that unforeseen factors or data limitations may still pose challenges in achieving absolute accuracy.
Understood, Iain. ChatGPT's ability to incorporate diverse data sources can certainly improve prediction accuracy. It's important for geologists to understand both the model's capabilities and limitations when making such predictions. Thanks for the insights!
Great article, Iain! The integration of AI in petroleum geology is an exciting development. How do you foresee the adoption of ChatGPT in the industry? Are there any barriers to widespread implementation?
Thank you, Liam! The adoption of ChatGPT and similar AI models will depend on factors like regulatory frameworks, acceptance within the industry, and overcoming any skepticism. Addressing concerns around data privacy, bias, and validation will be crucial in facilitating widespread implementation.
I agree, Iain. Overcoming regulatory challenges and fostering trust within the industry will be essential for the successful implementation of ChatGPT. Exciting times lie ahead in the intersection of AI and petroleum geology!
Fascinating read, Iain! How do you envision the collaboration between geologists and AI models like ChatGPT? What would be the ideal balance to ensure effective utilization?
Great question, William! The ideal collaboration involves geologists leveraging ChatGPT's capabilities as a powerful analytical aid while providing critical human judgment and expertise. Regular communication, validation, and refining the model's output through real-world experience will help strike the right balance.
I completely agree, Iain. Effective collaboration between geologists and AI models like ChatGPT is crucial to maximize their potential. It's all about leveraging the strengths of both humans and machines!
Very well-written article, Iain! ChatGPT's potential in petroleum geology is impressive. How do you see this technology influencing education and training in the field?
Thank you, Charlotte! ChatGPT and similar technologies can play a significant role in education and training. They can assist in providing real-time explanations, answering queries, and offering contextual insights, thereby enhancing the learning experience and supplementing traditional teaching methods.
That's fantastic, Iain! Incorporating AI models in education can undoubtedly enhance the learning process and help prepare the next generation of geologists. It's exciting to think about the possibilities!
Iain, your article on ChatGPT's application in petroleum geology was excellent! How do you see AI technologies evolving alongside traditional geological methods in the coming years?
Thank you, Ethan! Moving forward, I believe AI technologies will become integral to traditional geological methods. They will complement each other, with AI assisting in data analysis, pattern recognition, and optimization, while geologists provide insights, interpret complex scenarios, and make decisions based on their expertise.
That's a great perspective, Iain. The synergy between AI technologies and traditional geological methods will likely lead to powerful advancements and improved efficiencies. Exciting times lie ahead!
Well done, Iain! ChatGPT's potential impact on petroleum geology is enormous. How do you think this technology will shape future research and discoveries in the field?
Thank you, Mia! ChatGPT and similar AI technologies have the potential to accelerate research and facilitate new discoveries in petroleum geology. By analyzing vast amounts of data, identifying patterns, and offering novel insights, they can guide geologists towards untapped reserves and better understand subsurface systems.
That's truly exciting, Iain! The ability of AI technologies to analyze big data and reveal hidden patterns can significantly impact the course of research and lead to groundbreaking discoveries. I look forward to witnessing these advancements!
I enjoyed reading your article, Iain! ChatGPT's potential applications in petroleum geology are vast. What are your thoughts on the ethical considerations surrounding the use of AI models in scientific research?
Thank you, Grace! Ethical considerations are crucial when utilizing AI models in scientific research. Transparency, accountability, unbiased decision-making, and ensuring adherence to proper validation processes are essential factors. Continuous evaluation and feedback loops help address any ethical concerns that may arise.
I completely agree, Iain. Upholding ethical standards in AI research is paramount to maintain the integrity and reliability of results. Proper oversight and a collective responsibility to address ethical challenges will be key in harnessing the power of AI.
Great article, Iain! I'm curious to know if ChatGPT can be applied to other branches of geology, such as environmental or engineering geology?
Thank you, Samuel! Absolutely, ChatGPT can be applied to other branches of geology. Its capabilities can extend to environmental and engineering geology by assisting in data analysis, hazard assessments, and optimization of engineering approaches. Its potential across various geological domains is substantial!
That's fantastic, Iain! The versatility of ChatGPT in different geological domains is impressive. I can see how it would greatly benefit other fields of geology as well. Exciting times ahead for the entire discipline!
I thoroughly enjoyed your article, Iain! How do you see ChatGPT and similar models affecting the traditional workflow of petroleum geologists?
Thank you, Olivia! ChatGPT and similar models have the potential to streamline the traditional workflow of petroleum geologists. They can assist in data analysis, interpretation, and knowledge retrieval, allowing geologists to focus more on higher-level decision-making and leveraging their expertise in critical areas.
That's great, Iain! Automation of routine tasks through AI models can free up geologists' time and expertise for more targeted and strategic work. It's exciting to think about the increased efficiency and impact this could have!
Great article, Iain! How do you envision the future collaboration between AI technologies like ChatGPT and geologists in terms of research?
Thank you, Sebastian! In the future, AI technologies like ChatGPT will likely have a collaborative role in research. Geologists will work in tandem with AI models, leveraging their capabilities for data analysis, pattern recognition, and hypothesis generation, leading to more efficient and impactful research outcomes.
That's an exciting prospect, Iain. The collaborative potential between AI technologies and geologists can make research endeavors more fruitful and open up new avenues of exploration. Looking forward to witnessing the advancements!
Iain, your article provided a captivating glimpse into the potential of AI in petroleum geology. Could ChatGPT aid in optimizing well placement for maximum hydrocarbon recovery?
Thank you, Dylan! ChatGPT's capabilities can indeed contribute to optimizing well placement by considering subsurface data, geological indicators, and reservoir modeling. It has the potential to assist in identifying optimal drilling locations and maximizing hydrocarbon recovery.
That's impressive, Iain! The ability to optimize well placement can have a significant impact on hydrocarbon recovery rates. AI models like ChatGPT truly have the potential to revolutionize the decision-making process!
An insightful article, Iain! How do you think the integration of AI models in petroleum geology will affect the skill sets required for future geologists?
Thank you, Zara! The integration of AI models in petroleum geology will likely shape the skill sets required for future geologists. While domain knowledge and expertise remain essential, familiarity with AI, data analysis, and collaboration with intelligent systems are becoming increasingly valuable skills in our evolving industry.
That's an interesting aspect, Iain. As AI becomes more prevalent, geologists need to adapt and develop new skills to effectively collaborate with intelligent systems. It's important to stay updated with the evolving landscape!
Great article, Iain! How do you see the role of AI models like ChatGPT evolving in unconventional petroleum exploration, such as shale gas or tight oil plays?
Thank you, Muhammad! AI models like ChatGPT can play a valuable role in unconventional petroleum exploration. By analyzing complex geological data, these models can assist in identifying sweet spots, optimizing drilling techniques, and improving recovery rates, unlocking the full potential of shale gas and tight oil plays.
That's impressive, Iain! The unconventional petroleum sector stands to benefit greatly from AI technologies. Optimizing recovery rates in such plays can have a substantial impact on energy production. Exciting times ahead for the industry!
Well-written article, Iain! How do you anticipate ChatGPT and similar models being utilized in real-time drilling and well monitoring?
Thank you, Connor! In real-time drilling and well monitoring, ChatGPT can analyze drilling data, geophysical measurements, and geological responses to provide valuable insights. By continuously interpreting and flagging anomalies, it can assist drilling operations, optimize performance, and aid in decision-making.
That's impressive, Iain! The real-time capabilities of ChatGPT can contribute to safer and more efficient drilling operations. Its assistance in identifying potential issues and optimizing drilling performance can lead to significant improvements!
A thought-provoking article, Iain! Could ChatGPT assist in analyzing and interpreting hydrocarbon shows encountered while drilling to guide decision-making?
Thank you, Isabella! ChatGPT's capabilities can extend to analyzing and interpreting hydrocarbon shows encountered during drilling. By leveraging real-time data and assisting in decision-making, it can help geologists optimize drilling strategies, identify potential hydrocarbon reservoirs, and adjust exploration plans accordingly.
That's impressive, Iain! The ability to analyze hydrocarbon shows in real time can contribute to better decision-making during drilling operations. AI models like ChatGPT open up new possibilities for optimizing the exploration process!
I thoroughly enjoyed reading your article, Iain! Do you foresee collaborations between AI models like ChatGPT and researchers from other scientific disciplines for holistic studies?
Thank you, Lily! Collaborations between AI models like ChatGPT and researchers from diverse scientific disciplines can indeed lead to holistic studies. By integrating knowledge and insights from multiple fields, these collaborations can provide a more comprehensive understanding of complex geological phenomena and their interconnectedness.
That's fascinating, Iain! Collaborations across disciplines can unlock new insights and foster innovation. The potential of AI models in enabling such collaborative research is immense. Thank you for sharing your thoughts!
Excellent article, Iain! What do you think will be the most significant impact of ChatGPT in petroleum geology, both in the short term and long term?
Thank you, Charlie! In the short term, ChatGPT's impact in petroleum geology will likely be seen in improved data interpretation, optimization of routine tasks, and enhanced decision support. In the long term, it has the potential to reshape the way we explore and understand subsurface resources, leading to better resource allocation and increased success rates.
That's a comprehensive perspective, Iain. The short and long-term impacts of ChatGPT in petroleum geology are promising. Exciting times lie ahead as we embrace the potential of AI technologies!
Well done, Iain! How do you see AI models like ChatGPT shaping the decision-making process in risk assessment and exploration investment?
Thank you, Hannah! AI models like ChatGPT can significantly contribute to risk assessment and exploration investments. By analyzing geological data, historical records, and success rates, they can provide insights that aid in more informed decision-making regarding potential risks, resource potential, and investment strategies.
That's impressive, Iain! The ability to leverage AI models for risk assessment and investment decisions can improve resource allocation and optimize exploration strategies. It's exciting to think about the impact such technologies can have!
Great article, Iain! I'm curious to know if ChatGPT-generated interpretations can be utilized in real-world scenarios or if human validation is always required?
Thank you, Finn! ChatGPT-generated interpretations have the potential to be highly valuable in real-world scenarios. However, human validation is crucial, especially in complex or critical situations, to ensure accuracy, account for contextual factors, and address any unforeseen limitations or biases in the model's outputs.
Understood, Iain. The importance of human validation in critical scenarios highlights the need for collaboration and the combined strengths of humans and AI. Thank you for clarifying!
Iain, your article was insightful! What challenges do you anticipate in implementing AI models like ChatGPT in petroleum geology on a larger scale?
Thank you, Luna! Implementing AI models like ChatGPT on a larger scale may face challenges such as data availability, data quality, computational requirements, and integration with existing workflows. Addressing these challenges, along with regulatory aspects and acceptance within the industry, will be crucial for successful widespread implementation.
I can see how those challenges may arise, Iain. Overcoming them will require collective efforts, technological advancements, and a gradual transition towards the integration of such powerful AI models. Thank you for your insights!
You're welcome, Luna! Indeed, addressing these challenges requires a collaborative approach from various stakeholders. The potential benefits make it an endeavor worth pursuing. Thank you for engaging in this discussion!
Thank you all for your valuable comments and questions! It was a pleasure discussing the potential of ChatGPT in petroleum geology with you. I appreciate your engagement and hope you continue to explore the exciting advancements at the intersection of geology and AI.
Thank you all for joining this discussion! I'm excited to hear your thoughts on interpreting petroleum geology and leveraging ChatGPT for technological advancements.
Great article, Iain! I think the use of ChatGPT in petroleum geology can greatly improve data interpretation accuracy. The ability to leverage natural language processing with geological data is a game-changer.
Jennifer, I completely agree! ChatGPT can enhance our understanding of complex geological formations and help us make better decisions in the exploration and extraction of petroleum resources.
I'm impressed by the potential applications of ChatGPT in petroleum geology. It can analyze vast amounts of data and identify important patterns or anomalies that might be missed by human interpretation alone.
Jennifer and Mark, I'm glad you both see the value in applying ChatGPT to petroleum geology. It certainly has the potential to revolutionize the industry. Emma, you're right about its ability to analyze and process large data sets quickly.
I agree that ChatGPT can enhance geological interpretation processes, but how reliable is it compared to human experts? Are there any limitations we should be aware of?
Sophia, you raise a good point. ChatGPT's reliability can be improved by training it on extensive geological datasets and using it as a tool to assist human experts rather than replace them.
Sophia and James, you both have valid concerns. Emma, I completely agree that ChatGPT should be seen as a valuable tool to augment human expertise rather than replace it.
Emma and Jennifer, you raise an important point. ChatGPT's accuracy can be influenced by the complexity and availability of training data. Further research can address these limitations over time.
That's a valid concern, Sophia. While ChatGPT offers great potential, it's important to validate its interpretations against human expertise. It might excel in certain areas but fall short in others.
One potential limitation I see with using ChatGPT in petroleum geology is the need for high-quality input data. Garbage in, garbage out. How do we ensure the accuracy of the input data?
Andrew, that's an essential consideration. It's crucial to have well-curated and accurate geological datasets to train ChatGPT effectively. Data preprocessing and quality control are key.
Andrew and Sarah, the accuracy of input data is indeed vital. Proper preprocessing and validation procedures should be in place to minimize any potential inaccuracies and biases.
I wonder how ChatGPT can handle uncertainties and subjective interpretations often present in petroleum geology. Can it provide probabilistic assessments?
That's an interesting point, John. Incorporating uncertainty estimations and probabilistic assessments into ChatGPT's outputs would be valuable for decision-making processes.
John and Sophia, you've brought up an important aspect. While ChatGPT excels in various tasks, incorporating uncertainties and probabilistic assessments would indeed enhance its applicability in petroleum geology.
I'm curious about the computational resources required to run ChatGPT effectively. Are there any hardware or software limitations we should be aware of?
David, that's a good question. ChatGPT can be computationally intensive, requiring powerful hardware and significant resources. It's essential to optimize its implementation for efficient usage.
David and Jennifer, computational resources are an important consideration. Implementing efficient hardware infrastructure and optimizing software usage will be crucial for practical use.
I'm thrilled about the possibilities ChatGPT brings to petroleum geology research. The ability to analyze textual data, detect trends, and generate insights can significantly accelerate our understanding of geological processes.
Exactly, Eric! ChatGPT can uncover valuable patterns in geological texts, contributing to more efficient research and decision-making. It's exciting to witness the synergy between AI and geology.
Eric and Sarah, I share your excitement. The combination of AI and geology opens up new possibilities and accelerates the pace of discovery in petroleum geology.
While ChatGPT shows promise, I'm concerned about the potential biases it might inherit from the training data. How do we ensure fair and unbiased interpretations?
Jeff, that's a valid concern. To mitigate biases, it's crucial to carefully curate training data, consider diverse data sources, and implement rigorous evaluation procedures.
Jeff and Sophia, mitigating biases is an important aspect. Applying rigorous data curation techniques and evaluation procedures will be essential to ensure fair and unbiased interpretations.
I'm thrilled to see how ChatGPT can aid in knowledge transfer and collaboration among geologists. It has the potential to create a shared platform for sharing insights and expertise.
Absolutely, Nathan! ChatGPT can serve as a collaborative tool, allowing geologists to exchange knowledge and learn from each other's interpretations. It fosters a culture of collaboration.
Nathan and James, collaboration and knowledge sharing are vital aspects of research. ChatGPT can indeed facilitate these processes and create a platform for geologists to collaborate effectively.
I'm curious about ChatGPT's current limitations. Are there any geological scenarios in which it might struggle to provide accurate interpretations?
Emma, that's an interesting question. ChatGPT could potentially struggle with highly complex or poorly understood geological scenarios where limited training data is available.
Privacy and data security are crucial when incorporating AI technologies. How can we ensure the protection of sensitive geological data when using ChatGPT?
Robert, you're right. Robust data security measures, including encryption and access controls, must be implemented to safeguard sensitive geological data from unauthorized access during ChatGPT usage.
Robert and Sophia, ensuring data security and privacy is of utmost importance. Implementing robust data protection measures and adhering to strict access control protocols will help safeguard sensitive information.
I'm excited about the potential of leveraging ChatGPT for real-time decision support in petroleum geology. Its ability to quickly process and analyze data can aid in time-sensitive situations.
Emily, you're absolutely right! ChatGPT's real-time decision support capabilities can prove invaluable in critical scenarios, allowing for prompt analysis and informed decision-making.
Emily and David, real-time decision support is a valuable application of ChatGPT. Its rapid data processing capabilities can assist in time-sensitive situations and enhance decision-making processes.
Could ChatGPT potentially replace the need for certain specialized software commonly used in petroleum geology? I'm curious about its integration as a standalone tool.
Olivia, incorporating ChatGPT as a standalone tool might have limitations. Instead, integrating it with specialized software can enhance the capabilities of existing tools, providing a more comprehensive solution.
Olivia and Andrew, you bring up an important point. ChatGPT's integration with specialized software can enhance its capabilities and offer a more holistic solution in petroleum geology.
I believe ChatGPT can revolutionize the training and education of future geologists. Its ability to provide insights, explanations, and assist in knowledge transfer can greatly benefit the next generation of experts.
Mark, I couldn't agree more. ChatGPT can serve as a valuable educational tool that fosters learning and exploration among aspiring geologists, enabling them to gain insights from AI-powered systems.
Mark and Jennifer, I share your enthusiasm. ChatGPT's educational potential can help shape the future generation of geologists and equip them with valuable AI-driven insights and expertise.
One aspect to consider is the ethical use of ChatGPT in petroleum geology. How do we ensure responsible and unbiased usage?
Eric, that's an important concern. Developing ethical guidelines, promoting transparency, and subjecting the system to rigorous evaluations can help ensure responsible and unbiased usage of ChatGPT.
Eric and Sarah, ensuring ethical and unbiased usage of ChatGPT is a crucial responsibility. Establishing clear guidelines and evaluation frameworks will help maintain responsible practices.
Iain, thank you for sharing this insightful article. It has certainly sparked an engaging discussion. ChatGPT's potential in petroleum geology is undeniably exciting!
Jeff, thank you for your kind words. I'm glad this article has generated such an engaging discussion. The potential of ChatGPT in petroleum geology truly is exciting, and your participation is appreciated!
Thank you, Iain, for initiating this discussion. It's been fascinating to explore the implications of ChatGPT in petroleum geology. The conversations here have been incredibly insightful!
John, thank you for your kind words and active participation. It's been a pleasure to facilitate this discussion. The insights shared here are invaluable, and I appreciate everyone's contributions!
Thank you, Iain, for initiating this informative discussion. It's enlightening to hear diverse perspectives on leveraging ChatGPT in the field of petroleum geology. The future looks promising!