Leveraging ChatGPT for Seamless Data Integration in Big Data Technology
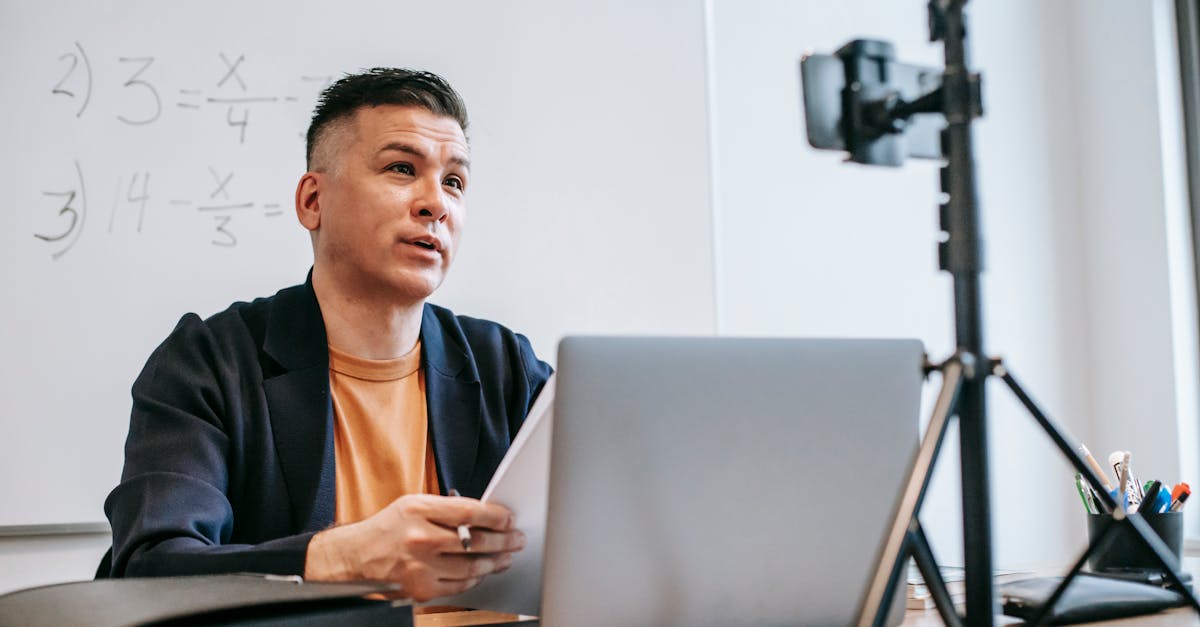
With the exponential growth of data in recent years, organizations are faced with the challenge of managing and integrating vast amounts of information from various sources. This is where Big Data technology comes into play, enabling businesses to extract valuable insights from disparate datasets.
Data integration is a crucial aspect of Big Data analytics as it involves combining data from different sources to create a unified view for analysis. This process can be complex and time-consuming, requiring expertise in data mappings, schema matching, and data normalization techniques.
Enter ChatGPT-4, the latest innovation in natural language processing and artificial intelligence. Powered by cutting-edge technology, ChatGPT-4 can assist in the data integration process by providing valuable insights and guidance.
Data Mappings
One of the challenges in data integration is mapping data elements between different sources that may use different terminologies or formats. ChatGPT-4 can help by understanding and suggesting data mappings based on its vast knowledge base. By discussing the specific data elements and their meanings with ChatGPT-4, users can receive recommendations on how to map them efficiently.
Schema Matching
Data integration often involves combining datasets with different schemas or structures. Schema matching is the process of identifying the common attributes and relationships between these schemas. ChatGPT-4 can assist in schema matching by analyzing the structure and content of the datasets and providing recommendations on how to match and align them effectively.
Data Normalization Techniques
Data normalization is crucial to ensure consistency and accuracy when combining datasets from disparate sources. It involves transforming data into a standardized format to eliminate redundancies and inconsistencies. ChatGPT-4 can provide insights into various data normalization techniques, such as removing duplicate records, handling missing values, and standardizing data formats, to ensure high-quality integrated datasets.
Using ChatGPT-4 for data integration offers several advantages. Firstly, it reduces the complexity and time required for data integration tasks, allowing organizations to streamline their analytical processes. Secondly, it leverages the vast knowledge and capabilities of artificial intelligence to provide accurate and effective insights. Lastly, it empowers users with a user-friendly and interactive interface, allowing them to engage in natural language conversations to address their integration needs.
In conclusion, Big Data technology has revolutionized the way organizations handle and analyze data. With the help of ChatGPT-4, data integration becomes more accessible and efficient, enabling businesses to harness the power of diverse datasets. By leveraging its capabilities in data mappings, schema matching, and data normalization techniques, ChatGPT-4 proves to be an invaluable tool in the ever-evolving field of Big Data analytics.
Comments:
Thank you all for reading my blog article on leveraging ChatGPT for seamless data integration in Big Data Technology! I hope you found it informative and useful. I'm here to answer any questions or discuss any points you'd like to explore further.
Great article, Tony! I've been exploring the potential of ChatGPT in big data projects, and your insights were very valuable. One question: have you encountered any specific challenges when integrating ChatGPT with large datasets?
Thanks, Maria! Integrating ChatGPT with large datasets can indeed pose some challenges. One of the main issues is the processing power required to handle large volumes of data in real-time. Additionally, ensuring data quality and accuracy is crucial for reliable results. It's essential to carefully preprocess and validate the input data before leveraging ChatGPT's capabilities.
Tony, I really enjoyed your article! You highlighted some important use cases for ChatGPT in the context of big data. I particularly appreciate the section on real-time processing. Do you have any recommendations on tools or frameworks that work well for integrating ChatGPT in real-time data pipelines?
Thank you, David! When it comes to integrating ChatGPT in real-time data pipelines, there are several options worth considering. Some popular choices are Apache Kafka and Apache Flink, as they offer scalable and reliable messaging and stream processing capabilities. Of course, the specific tools and frameworks to use depend on the requirements and existing technology stack of each project.
Interesting read, Tony! I can see how leveraging ChatGPT can enhance data integration processes. Do you have any advice on ensuring the security and privacy of sensitive data when using ChatGPT in big data technology?
Thanks, Anna! Security and privacy are indeed paramount when dealing with sensitive data. It's crucial to implement robust access controls, encryption mechanisms, and data anonymization techniques. Additionally, considering data residency and compliance requirements is necessary to ensure regulatory compliance. Collaborating with experts in cybersecurity can help design and implement a secure ChatGPT integration within big data technology.
Tony, your article shed light on the benefits of using ChatGPT in big data technology. I'm curious, though - have you observed any limitations or potential pitfalls when using ChatGPT for data integration?
Thank you, Sara! While ChatGPT is a powerful tool, there are a couple of limitations to be aware of. One limitation is the model's tendency to generate responses that may sound plausible but are factually incorrect. Careful validation and verification are necessary to prevent misleading results. Additionally, ChatGPT might struggle with domain-specific terminology or require fine-tuning to specialized use cases. Regular model updates and fine-tuning can mitigate these challenges.
Thanks for sharing your insights, Tony! I appreciated your examples of how ChatGPT can improve data integration. In your experience, how much time and effort does it typically take to train ChatGPT models for big data applications?
You're welcome, Alex! The time and effort required to train ChatGPT models for big data applications can vary depending on the scale and complexity of the data. Training large-scale language models like ChatGPT often involves substantial computational resources and time. However, with cloud-based infrastructure and tools like OpenAI's GPT-3, the process has become more accessible and efficient compared to training models from scratch.
Tony, your article provided an excellent overview of using ChatGPT for seamless data integration. I'm curious about the potential biases in the generated responses. Have you encountered any challenges related to bias, and how do you mitigate them?
Thank you, Lisa! Addressing biases is crucial when using AI models like ChatGPT. Biases can emerge due to the data used for training, which may reflect existing societal biases. OpenAI puts effort into reducing both glaring and subtle biases, but it's an ongoing challenge. By carefully curating the training data and implementing bias detection and mitigation techniques, we can strive for fair and unbiased results.
Tony, your insights are enlightening! I'm curious about the scalability of ChatGPT in the context of big data technology. Can it handle large volumes of data efficiently?
Thanks, Daniel! ChatGPT's scalability depends on several factors, including the hardware infrastructure available for model inference and the specific use case requirements. While it can handle large volumes of data, scaling up to handle big data efficiently may require optimizations like parallelization, distributed computing, or using specialized hardware like GPUs or TPUs. The scalability aspect should be carefully considered during system design and implementation.
Great article, Tony! I'm curious to know if there are any specific industries or sectors where ChatGPT's data integration capabilities can have a significant impact?
Thank you, Olivia! ChatGPT's data integration capabilities can find applications in various industries and sectors. Some notable areas include customer service, e-commerce, healthcare, finance, and research. Its ability to process and generate human-like responses makes it valuable for tasks like natural language understanding, recommendation systems, and knowledge bases in these domains.
Tony, I enjoyed reading your article! How do you see the future of ChatGPT evolving in the big data technology landscape? Are there any emerging trends or developments we should watch out for?
Thanks, Sophia! The future of ChatGPT in the big data technology landscape is promising. We can expect further advancements in natural language processing models, enabling even more accurate and context-aware responses. Fine-tuning models for industry-specific use cases will likely become more prevalent, leading to better integration with different domains. Collaboration between AI researchers, data engineers, and domain experts will be key to harnessing ChatGPT's potential fully.
Great article, Tony! I found your insights on leveraging ChatGPT for data integration quite valuable. I'm curious, though - have you observed any instances where ChatGPT's responses were too verbose or lacked conciseness?
Thank you, Adam! Yes, ChatGPT tends to generate lengthy and verbose responses by default. While this can sometimes be desirable for thorough explanations, it might not always be ideal for concise data integration. In such cases, post-processing techniques like summarization or sentiment analysis can help extract relevant information or ensure more concise responses based on specific requirements.
Tony, congratulations on a well-written article! I'm curious about the computational resources required to deploy ChatGPT for real-time data integration. Are there any recommendations on optimizing resource usage?
Thanks, Grace! Deploying ChatGPT for real-time data integration does require sufficient computational resources. To optimize resource usage, techniques like batching multiple requests together can help minimize overhead. Additionally, leveraging efficient hardware accelerators and optimizing the model's inference code can significantly improve performance. It's essential to profile and benchmark the system to identify and address any bottlenecks.
Great article, Tony! I found it intriguing to see the potential of ChatGPT in the context of big data integration. Are there any ongoing research efforts or open challenges in making ChatGPT more adaptable to different data integration scenarios?
Thank you, Liam! Research and development efforts are continually being made to enhance ChatGPT's adaptability to diverse data integration scenarios. Some open challenges include improving domain-specific knowledge representation, reducing biases, and enabling better control over generating responses. Additionally, making the model more explainable and interpretable is another important area of exploration. It's an exciting time to be involved in the field!
Tony, thank you for sharing your knowledge on leveraging ChatGPT! I'm curious - have you come across any noteworthy use cases or success stories where ChatGPT significantly improved data integration processes?
You're welcome, Julia! ChatGPT has shown promising results in various use cases. One notable example is customer support automation, where it can provide quick and accurate responses to user queries, minimizing manual intervention. Another use case is the integration of unstructured data from multiple sources, where ChatGPT can help make sense of complex information and enable better decision-making. Further exploration and case studies are continuously highlighting ChatGPT's potential.
Tony, your article provided a comprehensive overview of leveraging ChatGPT in big data technology. I'm curious about the potential ethical considerations when using AI models for data integration. Are there any particular guidelines or best practices you'd recommend following?
Thanks, Isabella! Ethical considerations are paramount in AI applications, including data integration. Some best practices include ensuring transparency about the use of AI models, obtaining necessary consent for data usage, and addressing potential biases and fairness issues. It's crucial to adhere to relevant legal and regulatory frameworks, as well as establishing proper governance frameworks to ensure ethical data practices throughout the integration process.
Excellent article, Tony! Your insights on using ChatGPT for seamless data integration are very informative. I'd like to know if you've encountered any limitations in terms of integrating ChatGPT with real-time streaming data sources?
Thank you, Sophie! Integrating ChatGPT with real-time streaming data sources can indeed present challenges. The speed at which streaming data arrives and the need for immediate processing can strain the system's responsiveness. Efficient ingestion techniques, stream processing frameworks, and optimization strategies become critical to handle the continuous flow of data effectively. Balancing real-time requirements and data integration capabilities is key for successful deployments.
Great article, Tony! Your explanations on leveraging ChatGPT for big data integration were concise and clear. I'm curious, though - are there any specific considerations one should keep in mind for integrating ChatGPT into existing big data ecosystems?
Thanks, Emma! When integrating ChatGPT into existing big data ecosystems, a few considerations are important. Ensuring interoperability with existing tools and frameworks, considering data formats and APIs, and evaluating resource requirements are crucial steps. Additionally, integrating appropriate monitoring and logging mechanisms ensures visibility into the system's performance and aids in troubleshooting. Collaboration with data engineers and solution architects can help navigate these considerations smoothly.
Tony, I thoroughly enjoyed your article! It's fascinating to see how ChatGPT can revolutionize data integration. Have you encountered any challenges in deploying ChatGPT at scale?
Thank you, Lucas! Deploying ChatGPT at scale can indeed present challenges. Besides the computational resources required, ensuring reliable and efficient communication between components within a distributed system becomes crucial. Handling high volumes of concurrent requests, optimizing latency, and monitoring performance are some of the key challenges to address. Robust deployment strategies, load testing, and continuous monitoring are essential for successful large-scale deployments.
Interesting read, Tony! Your article shed light on the potential benefits of integrating ChatGPT in big data technology. I'm curious about the maintenance and updating aspect. How often should models be retrained or fine-tuned for optimal performance?
Thanks, Jason! The frequency of retraining or fine-tuning ChatGPT models depends on various factors such as the dynamic nature of the data, rate of concept drift, and evolving requirements. For some use cases, models might need regular retraining to adapt to changing trends or industry-specific terminology. Continuous monitoring of performance and user feedback helps determine the optimal schedule for updates. Striking a balance between model freshness and resource requirements is crucial.
Great article, Tony! Your insights on ChatGPT for seamless data integration were quite enlightening. I'm curious, though - have you encountered any challenges with data compatibility or data types when integrating ChatGPT?
Thank you, Sophia! Data compatibility and handling different data types can present challenges during ChatGPT integration. Preprocessing pipelines to handle varied formats and ensuring data cleanliness are crucial to mitigate compatibility issues. Additionally, aligning data types with the model's input requirements and understanding how each data type influences the model's behavior is important. Robust data validation, transformation techniques, and maintaining data dictionaries can aid in addressing data compatibility challenges.
Tony, your article provided excellent insights into ChatGPT's role in big data technology. I'm interested in knowing if there are any cognitive biases associated with ChatGPT's responses?
Thanks, Andrew! Like any AI system, ChatGPT can exhibit cognitive biases, especially if the training data carries biases from human-generated sources. OpenAI is actively working on reducing both obvious and subtle biases in model responses. However, users must be cautious and apply critical thinking while interpreting the outputs. Regular audits, diverse training data, and involving a diverse group of reviewers help in addressing biases and improving the system's fairness.
Tony, your article provided a great overview of leveraging ChatGPT for data integration! I'm curious - are there any considerations one should pay attention to when using pre-trained models versus training custom models from scratch?
Thank you, Ethan! When choosing between pre-trained models and training custom models, several considerations come into play. Pre-trained models like GPT-3 offer the advantage of leveraging a vast knowledge base. However, they might lack fine-tuning for specific use cases or niche domains. Training custom models from scratch provides more control over the learning process but requires significant labeled data and computational resources. The decision ultimately depends on factors like available data, time, and resource constraints.
Impressive article, Tony! I found your insights on ChatGPT for seamless data integration in big data quite insightful. Can you share any tips on handling data inconsistencies or noisy data during the integration process?
Thanks, Nathan! Handling data inconsistencies and noisy data during integration is crucial to maintain data quality. Implementing robust data cleaning and preprocessing techniques helps address inconsistencies and eliminate noise to a certain extent. Using statistical methods, outlier detection, or leveraging domain knowledge for data validation can aid in filtering noisy data. Striking a balance between data cleansing and preserving valuable information is essential for successful integration.
Tony, your article on ChatGPT's role in big data integration was well-articulated and insightful. I'm curious about the computational cost associated with deploying ChatGPT in real-time data processing. Can you provide any insights?
Thank you, Emily! Deploying ChatGPT in real-time data processing can indeed have computational costs. The cost depends on factors like the model size, the complexity of the inference pipeline, and the scale of the data being processed. Efficient resource allocation, parallelization techniques, and hardware acceleration can help mitigate the computational cost and improve performance. Optimizing the system architecture and infrastructure design based on specific requirements is vital.
Great article, Tony! You provided a clear and concise overview of leveraging ChatGPT for big data integration. I'm curious, though - how can we measure the accuracy or success of ChatGPT's responses in the context of data integration?
Thanks, Jacob! Measuring the accuracy or success of ChatGPT's responses in data integration involves a multi-faceted evaluation approach. Quantitative metrics like precision, recall, and F1 scores can be employed where feasible. Additionally, user feedback, satisfaction surveys, and domain-specific evaluation criteria help gauge the relevance and usefulness of responses. Piloting, A/B testing, and involving domain experts are valuable strategies to measure the accuracy and success of the integrated system within specific use cases.
Tony, your article provided a great introduction to leveraging ChatGPT for data integration in big data technology. I'm curious if there are any specific pre-processing steps one should take to prepare data for ChatGPT integration?
Thank you, Grace! Preparing data for ChatGPT integration typically involves some essential pre-processing steps. These steps can include cleaning and normalizing the data, removing noise or irrelevant information, handling missing values, and converting the data into appropriate formats compatible with ChatGPT's input requirements. Depending on the specific use case, additional steps like tokenization, lemmatization, or entity recognition may be necessary to enhance the quality and relevance of data for integration.