Maximizing Returns: Leveraging ChatGPT for Public Sentiment Analysis in ROI Technology
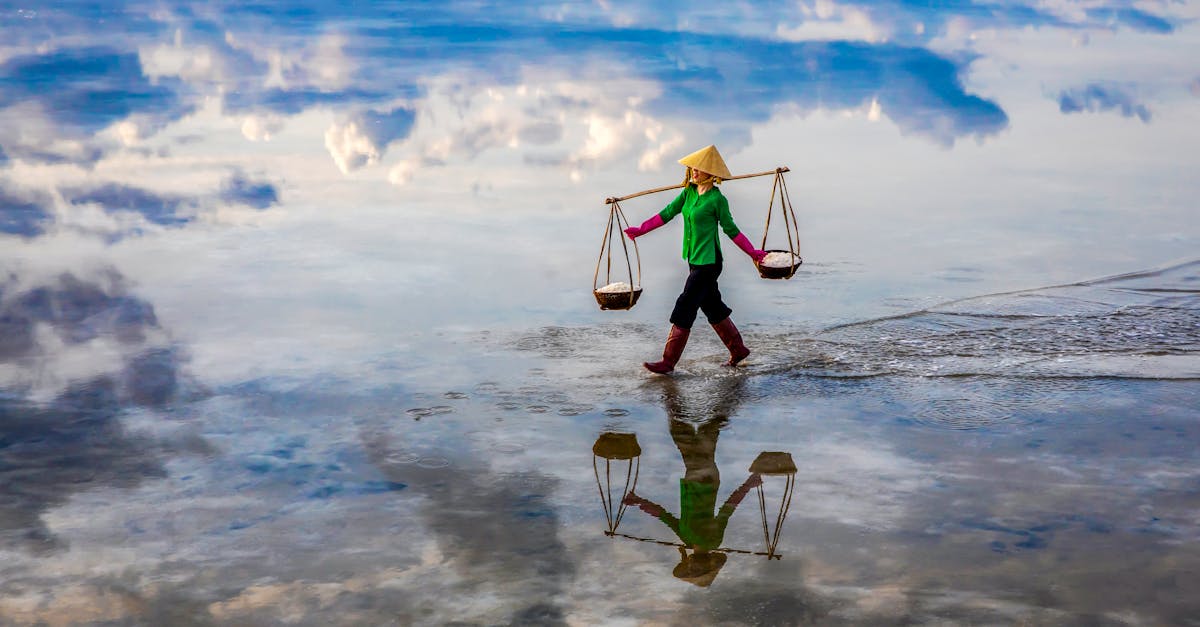
Introduction
Advances in technology have brought about numerous benefits across various industries. One such area is public sentiment analysis, which plays a crucial role in understanding public opinion about products, services, events, or ideas. With the advent of GPT-4, a powerful language model, the analysis process has become increasingly accurate and efficient, ultimately leading to a higher return on investment (ROI).
GPT-4: The Next Generation Language Model
GPT-4, the fourth iteration of OpenAI's Generative Pre-trained Transformer (GPT) series, takes natural language processing to new heights. It has been trained on a vast corpus of data, including social media posts, reviews, blogs, and various other sources of text from the internet. This extensive training allows GPT-4 to understand and generate human-like text with exceptional accuracy and contextual understanding.
The Importance of Public Sentiment Analysis
Understanding public sentiment has always been vital for businesses and organizations. It helps them gauge how their offerings are received by the public and identify areas for improvement. Traditionally, such analysis was time-consuming and often subject to biases. However, with the implementation of GPT-4, sentiment analysis has become significantly more efficient and reliable.
Application in Product and Service Development
By utilizing GPT-4's language processing capabilities, companies can analyze social media posts, reviews, and blogs to gain valuable insights into public opinion. This analysis aids in the development of products and services that align with the preferences and needs of the target audience. By understanding sentiment trends, businesses can make informed decisions and optimize their ROI.
Enhancing Event Planning and Marketing Campaigns
GPT-4's ability to analyze public sentiment extends beyond analyzing products and services. Event planners and marketers can harness its power to gain insights into public opinion about upcoming events or ongoing marketing campaigns. By understanding sentiment, event planners can tailor their programs to meet the expectations of attendees, leading to enhanced participant satisfaction and increased ROI.
The Impact on Public Sentiment Tracking
Previously, tracking public sentiment was a complex task, requiring extensive manual efforts or the use of simpler sentiment analysis tools. However, GPT-4's advanced language processing capabilities streamline the sentiment tracking process. Its ability to comprehend context, infer opinions, and identify important aspects of public sentiment makes it invaluable for businesses aiming to stay ahead in highly competitive markets.
Conclusion
GPT-4's revolutionary language processing abilities have revolutionized public sentiment analysis, allowing businesses and organizations to effectively gauge public opinion. By leveraging GPT-4 to analyze social media posts, reviews, and blogs, companies can make informed decisions when it comes to product development, event planning, and marketing campaigns. With improved insight into public sentiment, businesses can optimize their return on investment and achieve greater success in their respective industries.
Comments:
Thank you all for taking the time to read my article on maximizing returns with ChatGPT for public sentiment analysis in ROI technology. I appreciate your feedback and comments!
Great article, Alan! I found your insights on using ChatGPT for sentiment analysis very informative. It seems like this technology has a lot of potential.
I agree, Linda. Sentiment analysis plays an important role in understanding customer behavior and maximizing returns. Do you think ChatGPT is more effective than other sentiment analysis tools?
Hi Tom, it's hard to say definitively without proper comparisons, but based on my initial research, ChatGPT seems to offer great accuracy and flexibility in sentiment analysis. Perhaps Alan can provide more insights on this?
Alan, I enjoyed reading your article. Sentiment analysis is crucial in understanding customer sentiment and making informed business decisions. How do you see ChatGPT evolving in the future? Are there any limitations?
Thanks for sharing your knowledge, Alan! ChatGPT has undoubtedly revolutionized various aspects of natural language processing. What industries do you think would benefit the most from leveraging ChatGPT for sentiment analysis?
Interesting read, Alan. Sentiment analysis can significantly impact ROI. Are there any key challenges or potential biases that need to be considered when using ChatGPT for sentiment analysis?
Thanks, Linda, Tom, Robert, Emily, and Richard for your engaging comments! I'm glad you found the article valuable. Let me address your questions one by one.
Alan, in your opinion, what are the areas where ChatGPT can provide the most value in sentiment analysis? Are there specific sectors where it outperforms other tools?
Robert, in terms of value, ChatGPT can be highly beneficial in industries such as customer support, brand reputation management, market research, and social media sentiment analysis. While it may not outperform other tools in every scenario, its versatility and language understanding capabilities make it a valuable resource.
Can you share some examples or success stories, Alan, where companies have benefited from leveraging ChatGPT for sentiment analysis? It would provide concrete evidence of its value.
Robert, there are several success stories demonstrating the value of ChatGPT in sentiment analysis. For example, a large e-commerce company saw a significant increase in customer satisfaction by leveraging ChatGPT to analyze feedback sentiment and improve their products and services accordingly.
That's an impressive example, Alan. It demonstrates the tangible benefits of sentiment analysis using ChatGPT. Real-world success stories like these encourage more organizations to explore the potential of this technology.
Alan, what are your thoughts on real-time sentiment analysis using ChatGPT? Can it be used effectively for monitoring and responding to customer sentiment in real-time?
Robert and Linda, real-time sentiment analysis is indeed a valuable application. While ChatGPT can provide near real-time sentiment analysis, it's important to consider response time and computational resources for handling high volumes of streaming data.
Alan, what are the key privacy considerations when implementing ChatGPT for sentiment analysis? How can organizations protect user data while leveraging this technology?
Robert, privacy is a critical aspect. When using ChatGPT for sentiment analysis, organizations should follow best practices like anonymizing user data, implementing data encryption, and ensuring compliance with relevant data protection regulations such as GDPR or CCPA. Respecting user privacy should be a top priority.
Alan, while sentiment analysis is essential, do you think incorporating other NLP techniques along with ChatGPT could enhance the overall accuracy and relevance of sentiment analysis?
I've been using various sentiment analysis tools, including ChatGPT, for a while now. While ChatGPT is quite powerful, it still faces challenges with sarcasm and context understanding. I'm curious to know Alan's thoughts on this.
That's a good point, Sandra. The contextual understanding of natural language by GPT models can sometimes be limited. Alan, how do you address these challenges when using ChatGPT for sentiment analysis?
I agree with Sandra and Tom. The limitations should be considered. Alan, any methods or techniques you recommend to overcome the challenges of sarcasm and contextual understanding by ChatGPT?
Thank you for your questions, Sandra, Tom, Linda, Robert, and Richard. I'll try to address each of your points.
Alan, given the potential of sentiment analysis using ChatGPT, which industry sectors do you think would benefit the most in terms of strategic decision-making and improved customer satisfaction?
Linda, Sandra, and Tom—regarding the effectiveness of ChatGPT in sentiment analysis compared to other tools, it's important to evaluate performance on specific tasks and datasets. In general, ChatGPT has demonstrated promising results, but more extensive research and benchmarks are needed for thorough comparisons.
I see, thanks for your answer, Alan. It makes sense to consider specific tasks and datasets for accurate comparisons. I appreciate your perspective.
That's true, Tom. Sarcasm, humor, and context can be challenging for AI models. Alan, do you think fine-tuning ChatGPT on domain-specific data could help improve its contextual understanding in sentiment analysis?
Great point, Sandra. Fine-tuning the models with domain-specific data might enhance the contextual understanding. Alan, any insights on the best practices for fine-tuning ChatGPT for sentiment analysis?
Combining ChatGPT with other NLP techniques seems like a good idea, Alan. It would provide a more comprehensive analysis by leveraging the strengths of different tools. I'd be interested to know your thoughts on this.
I'm also curious about that, Alan. It would be great to understand how blending ChatGPT with other NLP techniques can improve sentiment analysis, especially in sectors like eCommerce or social media.
Emily, strategic decision-making can be significantly improved with accurate sentiment analysis. Sectors like retail, hospitality, healthcare, and finance could benefit by leveraging ChatGPT's insights to enhance customer satisfaction, brand perception, and market understanding.
Fine-tuning ChatGPT on domain-specific data is indeed a practical approach, Alan. It can help the model better grasp the nuances and context of the given domain, leading to more accurate sentiment analysis results.
Thanks for your response, Alan. Fine-tuning with domain-specific data seems like the way to go. It would ensure better performance and relevance. I appreciate your insights on this.
Alan, blending ChatGPT with other NLP techniques like Named Entity Recognition or Topic Modeling could offer a more comprehensive sentiment analysis. It would provide deeper insights and reduce potential biases. Do you agree?
I completely agree, Richard. By combining various NLP techniques, we can create a more robust sentiment analysis system, especially in industries where accurate understanding of entities and key topics greatly influences decision-making.
Alan, are there any specific domain-specific datasets or techniques you recommend for fine-tuning ChatGPT? It would be helpful for those interested in implementing sentiment analysis using this technology.
Sandra, the choice of domain-specific datasets for fine-tuning depends on the particular industry. It's essential to select datasets with a diverse range of sentiment expressions and topics related to the target domain. Techniques like transfer learning, self-supervised learning, and domain adaptation can also be applied.
Thank you for the insights, Alan. It's helpful to have guidelines on dataset selection and techniques for fine-tuning. Transfer learning and domain adaptation seem like crucial steps in achieving domain-specific accuracy.
Alan, I'm curious about the scalability of ChatGPT for sentiment analysis. Can it handle large volumes of data effectively, or are there any limitations to consider?
That's a good question, Emily. Understanding the scalability of ChatGPT in sentiment analysis is vital, especially for industries dealing with massive amounts of customer feedback and online interactions.
Emily, ChatGPT can handle large volumes of data but may have limitations in terms of response time and cost efficiency. Efficient data preprocessing, parallelization techniques, and prioritizing relevant data subsets can help mitigate these challenges.
Thank you, Alan. That clarifies the scalability aspect. Prioritizing relevant data subsets and using parallelization techniques seems like a practical approach to ensure efficient sentiment analysis.
Exactly, Emily. Prioritization and parallelization are key factors for efficient sentiment analysis. It ensures faster processing and avoids unnecessary overhead, especially when dealing with large-scale data.
Real-time sentiment analysis is crucial, Alan. Especially in sectors like social media, where immediate action or response is often required. I'm interested in knowing if ChatGPT can handle such scenarios effectively.
Linda, ChatGPT's effectiveness for real-time sentiment analysis varies based on the specific use case and data volume. Implementing efficient streaming processing and employing technologies like Apache Kafka or RabbitMQ for data ingestion can help ensure real-time capabilities.
Alan, given the increasing concerns around bias in AI models, how can we ensure that ChatGPT-based sentiment analysis remains unbiased and fair?
Linda, mitigating bias in sentiment analysis is crucial. Regularly evaluating and retraining ChatGPT on diverse and balanced datasets can help in reducing biases. Additionally, having a strong feedback loop and involving humans in the decision-making process can contribute to fairness and accountability.
Thank you for addressing my concern, Alan. Regular evaluation, diverse datasets, and human involvement indeed seem essential in ensuring the fairness and unbiased nature of sentiment analysis using ChatGPT.
Alan, considering the ever-evolving nature of language and online interactions, how does ChatGPT handle or adapt to changes in sentiment expression and user behavior over time?
That's an interesting question, Emily. Sentiment expression can vary greatly, and user behavior evolves over time. Alan, any insights on how ChatGPT can adapt to these changes?
Emily and Richard, ChatGPT's ability to adapt to changes in sentiment expression and user behavior relies on continuous training and fine-tuning with up-to-date datasets. Regularly updating training data and leveraging transfer learning can help address the evolving nature of language and user interactions.