Mining Engineering's Next Frontier: Enhancing Equipment Maintenance with ChatGPT
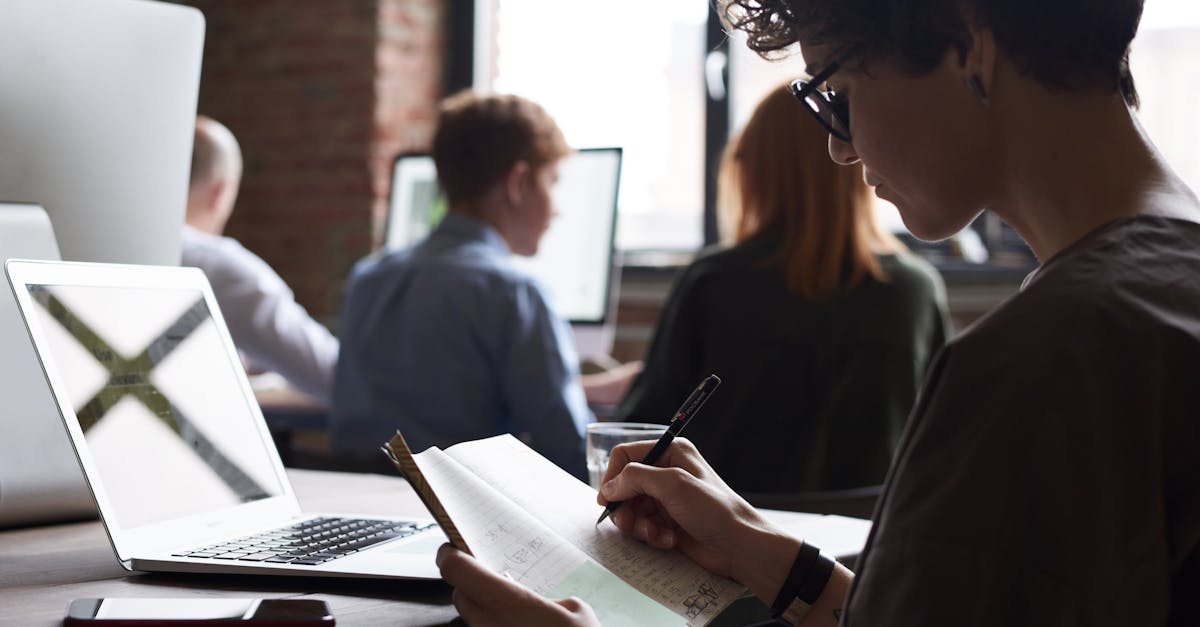
Modern mining operations heavily rely on complex machinery and equipment to extract valuable minerals and resources from the earth. However, these machines are subject to wear and tear, and their maintenance is crucial in order to ensure efficient and uninterrupted mining operations. In recent years, advancements in technology have revolutionized equipment maintenance in the mining industry.
Utilizing Historical Data for Predictive Maintenance
One of the key developments in equipment maintenance in mining engineering is the use of predictive analytics based on historical data. By leveraging the power of data analysis and machine learning algorithms, mining companies can predict when a machine might require maintenance or experience a failure. This proactive approach helps in minimizing downtime, reducing costs, and optimizing operational efficiency.
Benefits of Predictive Maintenance
Predictive maintenance in mining engineering offers several advantages over traditional reactive maintenance practices:
- Cost Reduction: By identifying potential issues in advance, mining companies can plan and schedule maintenance activities more efficiently, minimizing the occurrence of unscheduled downtime and reducing overall maintenance costs.
- Increase in Operational Efficiency: Predictive maintenance allows for better planning of maintenance activities, ensuring that machines are serviced at the right time, resulting in improved operational efficiency and reduced disruptions to production.
- Enhanced Equipment Lifetime: Regular and timely maintenance based on predictive analytics can significantly extend the lifespan of mining equipment, maximizing return on investment and reducing the need for frequent replacements.
- Improved Safety: By proactively addressing potential equipment failures, mining companies can mitigate safety risks associated with unexpected breakdowns, protecting both the workers and the environment.
Implementing Predictive Maintenance in Mining Operations
Implementing predictive maintenance in mining operations involves several key steps:
- Data Collection: Mining companies need to collect and integrate large volumes of historical data related to equipment performance, maintenance history, and operating conditions. This data forms the foundation for predictive analytics.
- Data Analysis: Advanced analytical tools and algorithms are used to analyze the collected data, identifying patterns, trends, and potential failure points. Machine learning techniques are employed for accurate predictive modeling.
- Threshold Setting: Based on the analysis and modeling results, thresholds are set to determine when maintenance actions should be triggered. These thresholds are specific to each equipment type and its failure characteristics.
- Real-time Monitoring: Once the thresholds are set, real-time monitoring systems are put in place to continuously assess the condition of equipment. Any deviation from normal operating conditions triggers alerts, notifying maintenance teams to take preventive action.
- Maintenance Planning: The information obtained from predictive maintenance systems is used to plan and schedule maintenance activities, ensuring minimal disruption to mining operations.
Conclusion
Predictive maintenance leveraging historical data has emerged as a game-changer in the field of equipment maintenance in mining engineering. By using advanced analytics and machine learning, mining companies can proactively identify potential failures and plan maintenance activities accordingly. This approach not only reduces costs and improves operational efficiency but also extends the lifespan of equipment and enhances safety in mining operations. As technology continues to advance, the mining industry can expect further innovations in equipment maintenance, making mining operations more efficient and sustainable in the long run.
Comments:
This article provides a fascinating glimpse into how technology is revolutionizing the field of mining engineering. It's incredible to see how AI-powered chatbots like ChatGPT can enhance equipment maintenance and improve overall efficiency.
I agree, Emily! The integration of AI in maintenance processes is definitely a game-changer. ChatGPT seems to offer an innovative solution by combining engineering expertise with chatbot capabilities. Kudos to the authors for shedding light on this exciting development.
As a mining engineering student, I find this article tremendously interesting. It's inspiring to see how technology is advancing in our field. I wonder how widely ChatGPT is currently used in the industry and what challenges may arise with its implementation.
Thank you, Emily, Michael, and Sophia, for your positive comments! I'm thrilled to see your enthusiasm for this topic. Sophia, ChatGPT is still gaining traction in the industry, but it holds great potential. Implementation challenges may include data security, model bias, and ensuring smooth human-machine cooperation.
I'm also curious about the potential limitations of ChatGPT. While it sounds promising, can it handle complex and unique maintenance situations that may arise in mining engineering?
That's a valid concern, Jonathan. ChatGPT's performance is largely dependent on the data it has been trained on. While it can handle a wide range of maintenance scenarios, addressing complex and unique situations might require further fine-tuning or expert intervention. Continuous improvement is crucial.
I think it's important to remember that ChatGPT shouldn't be seen as a replacement for human expertise, but rather as a valuable tool that complements the skills of mining engineers. It can provide quick suggestions or prompts, but the final decision should always rest with the professionals.
Absolutely, Vanessa! AI-powered tools should augment human capabilities, not replace them. The combination of human expertise and AI assistance could lead to efficient and effective maintenance practices in mining engineering.
I must say, I'm impressed by the potential of ChatGPT in mining engineering. However, I'm concerned about the costs associated with implementing such technology. Would smaller mining companies be able to afford it?
Great point, Grace! Implementing AI technology, including ChatGPT, may involve significant costs, especially for smaller companies. However, as the technology advances and becomes more widespread, we can hope for more accessible and cost-effective solutions for all.
I agree with Grace. Cost is a big factor, especially for small-scale mining operations. It will be essential to consider the return on investment and the long-term benefits that implementing ChatGPT can provide.
Indeed, Daniel. Assessing the cost-benefit ratio is crucial for any technology implementation. While it may have higher upfront costs, the long-term benefits, such as increased efficiency and minimized downtime, can outweigh the initial investment.
The use of AI in equipment maintenance is undeniably promising. However, it's imperative to ensure that the models powering these chatbots are extensively trained on diverse data to avoid any unintentional biases.
I completely agree, Sophie. Bias mitigation should be a crucial consideration in the development and deployment of AI tools like ChatGPT. Diverse and representative training data are crucial for fair and accurate decision-making.
Spot on, Sophie and Hannah! Bias mitigation is an important aspect of AI development. Mining engineering professionals need to actively be involved in data collection and model training to ensure fairness and avoid potential biases.
I'm amazed by how quickly AI technology is advancing in various fields, including mining engineering. The potential for AI-powered maintenance systems like ChatGPT is truly exciting!
You're right, Daniel! AI has the potential to revolutionize the mining industry. I'm particularly looking forward to seeing how it improves equipment maintenance, as it directly impacts operations and productivity.
While the use of AI in mining engineering holds immense promise, we should also consider any potential risks associated with relying too heavily on technology. It's important to have backup plans and alternative approaches in case of any system failures.
Absolutely, Emma! It's crucial to have contingency plans in place and not solely rely on AI technology. Maintaining redundancies and backup strategies help mitigate the risks associated with system failures or technological dependencies.
This article highlights an exciting aspect of mining engineering. Integrating AI assistance in equipment maintenance has the potential to enhance worker safety by predicting and preventing accidents.
I couldn't agree more, Riley. Safety should always be a top priority in mining operations. AI-based systems like ChatGPT can contribute to creating a safer work environment by providing timely suggestions and alerts.
Well said, Riley and Ella! Indeed, AI-powered systems have the potential to prevent accidents and improve overall safety in mining. Timely notifications and proactive maintenance can go a long way in ensuring worker well-being.
As a mining professional, I'm excited about the possibilities offered by these AI-assisted maintenance systems. However, I wonder how the existing workforce will adapt to these technological advancements. Will there be a need for upskilling or retraining?
That's an important point, Lucas. With the integration of AI technologies, upskilling and retraining might become necessary to ensure that the workforce can effectively collaborate with these systems and fully utilize their potential.
Indeed, Lucas and Sophia. As technology progresses, ensuring a smooth transition for the existing workforce becomes vital. Adequate training and upskilling initiatives should be implemented to equip employees with the necessary skills to leverage AI-assisted maintenance systems.
I'm thrilled to see how AI is transforming not only the mining industry but also other engineering fields. ChatGPT seems like an impressive tool that can revolutionize equipment maintenance. Exciting times ahead!
Absolutely, Isabella! AI is leaving its mark on various sectors, and mining engineering is no exception. The advancements in equipment maintenance through ChatGPT have the potential to change the game for the better.
While the benefits of AI in maintenance are evident, I wonder if there are any potential ethical considerations that need to be addressed. How can we ensure that these AI systems make morally sound decisions?
Great question, Jack! Ethical considerations are crucial when deploying AI systems. Ensuring transparency, accountability, and aligning AI decision-making with ethical guidelines are essential steps to foster responsible AI implementation.
I believe AI decision-making ethics in mining engineering should align with environmental sustainability goals as well. It's vital to ensure that the choices made by such systems are environmentally responsible.
Absolutely, Sophie! Environmental sustainability is an important aspect, and integrating it into AI decision-making processes can help drive responsible mining practices. Striking the right balance between technological advancements and sustainability is essential.
I can see how ChatGPT can greatly benefit large mining companies with extensive resources. But what about the small-scale miners or those in remote locations? Will they have access to such technology?
A valid concern, Nathan. Smaller mining companies or those in remote areas might face challenges in accessing and implementing such technologies due to limited resources and infrastructure. However, advancements in cloud computing and the growing popularity of AI services can improve accessibility in the future.
I'm impressed by the possibilities of AI in predictive maintenance. An AI-powered system like ChatGPT can help mining companies move from reactive to proactive maintenance strategies, thereby improving efficiency and cost-effectiveness.
Well said, Emily! With real-time data analysis and predictive capabilities, AI tools have the potential to transform maintenance operations. Being proactive instead of reactive can lead to significant cost savings and minimize disruptions to mining activities.
I wonder if ChatGPT could be enhanced to provide real-time troubleshooting assistance. Having step-by-step instructions and guidance during equipment breakdowns could significantly reduce downtime and the need for on-site maintenance experts.
That's an interesting suggestion, Hannah. Real-time troubleshooting assistance can indeed help reduce downtime and expedite repairs. As AI technology advances, integrating live diagnostics and providing actionable recommendations could be possible additions to ChatGPT or similar systems.
I'm thrilled to see how AI is making its way into the mining industry. It's a testament to the adaptability of engineering fields. Kudos to the authors for exploring the potential of AI in enhancing equipment maintenance.
Indeed, Grace! Embracing emerging technologies like AI enables us to tackle challenges in new and exciting ways. The mining industry is ready for a technological transformation, and ChatGPT can be a significant part of it.
I'm excited to witness the practical implementation of AI in mining engineering. It opens up a world of possibilities for improving efficiency, safety, and sustainability in mining operations.
Couldn't agree more, Liam! AI-powered systems like ChatGPT have the potential to reshape the mining industry and unlock new levels of productivity and sustainability.
Thank you, Grace, Daniel, Liam, and Ella, for your kind words and insightful thoughts! It's been wonderful to engage in this discussion and witness your enthusiasm for the application of AI in mining engineering.
This article has sparked so many interesting conversations about the future of mining engineering. It's great to see professionals and students alike coming together to discuss the potential of AI in improving equipment maintenance.
I couldn't agree more, Sophia. These discussions highlight the need for continuous exploration and collaboration to ensure responsible and effective implementation of AI technologies in mining engineering.
Absolutely, Michael. The future of mining engineering lies in the synergy between human expertise and AI assistance. Together, we can propel the industry forward and overcome the challenges we face.
Thank you, Andrew, for authoring this thought-provoking article and actively participating in the discussion. Your contributions have been valuable in shaping our understanding of the potential of AI in enhancing equipment maintenance.
Indeed, Andrew. Your insights and responses have been enlightening. The article provides a glimpse into an exciting future for mining engineering, and your engagement has added depth to the conversation.
Thank you all for your kind words and thoughtful discussions! It's been a pleasure engaging with each one of you. The potential of AI in mining engineering is immense, and together, we can explore its benefits while addressing the associated challenges.
I really appreciate the open dialogue in this discussion. It's an excellent example of how professionals from different backgrounds can come together to exchange ideas and insights.
Absolutely, Emma! Open discussions promote knowledge sharing and foster innovation. Thank you all once again for contributing to this engaging conversation about AI in mining engineering.
Indeed, Andrew! Let's keep pushing the boundaries of what's possible in the field of mining engineering.
Absolutely, Riley! With the collective effort of passionate professionals like you, the future of mining engineering looks promising.
Thank you for facilitating this insightful discussion, Andrew. It has been a pleasure engaging with everyone.
Thank you, Andrew, for your active participation and for sharing your expertise with us.
We appreciate your engagement, Andrew. Thank you for shedding light on the exciting opportunities presented by ChatGPT in the field of mining engineering.
Thank you, Andrew, for your valuable responses and for hosting this meaningful discussion.
Andrew, your insights as an author and expert in this field have been extremely valuable. Thank you for your time and for addressing our inquiries.
It's been an honor to discuss this topic with you, Andrew. Your contributions have helped broaden our understanding of AI in mining engineering.
Thank you all for your kind words. I'm delighted to have participated in this discussion and shared insights with such an engaging community. Let's continue to explore the exciting possibilities of AI in mining engineering!