Optimizing Assembly Processes: Leveraging ChatGPT for Design for Manufacturing in 'Design for Assembly' Technologies
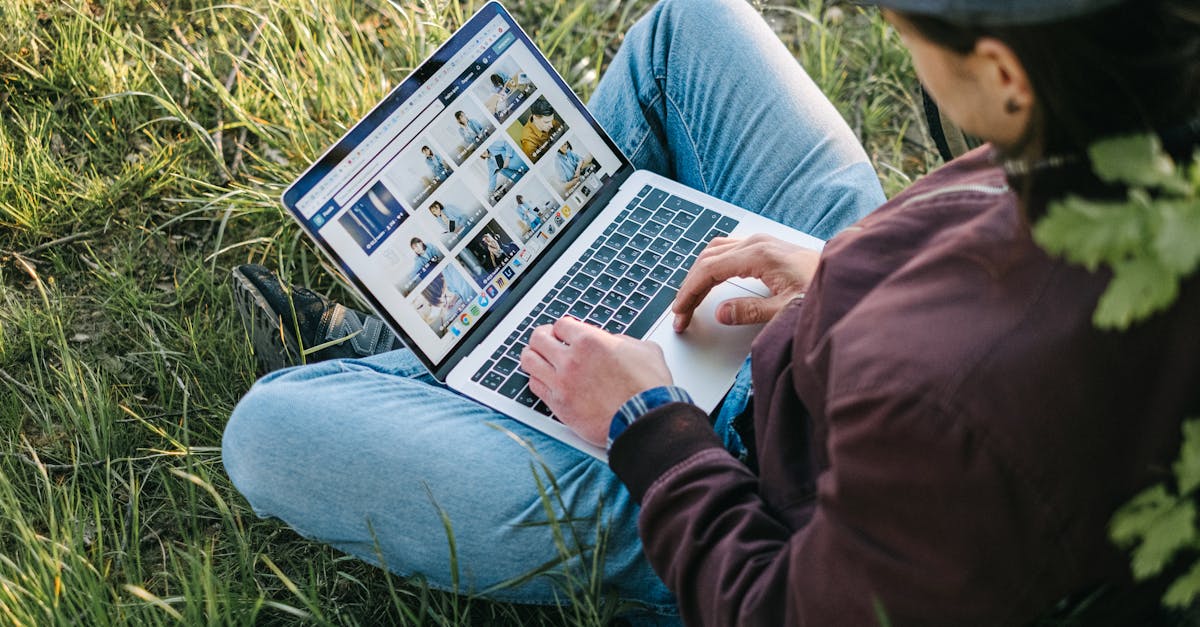
In the realm of manufacturing, optimizing the design for assembly plays a crucial role in ensuring efficient and cost-effective production processes. Design for Assembly (DFA) focuses on creating product designs that can be easily assembled, reducing the time, effort, and cost involved in the assembly process. With advancements in Artificial Intelligence (AI), manufacturers can now leverage AI technologies to suggest techniques that simplify the product structure for an easier and streamlined assembly process.
The Role of AI in Design for Manufacturing
AI-driven DFA solutions analyze product designs, identifying potential assembly challenges, and proposing alternative design choices that simplify the assembly process. By leveraging advanced algorithms and machine learning techniques, AI can quickly analyze and evaluate the product's structural components, identifying areas for improvement.
AI algorithms consider various factors such as part geometries, tolerances, material properties, and assembly sequence to suggest design modifications that enhance ease of assembly. These modifications can include changes to part geometries to allow for easier alignment and insertion, as well as reducing the number of components and their interconnections.
Benefits of AI-driven DFA
The utilization of AI in DFA provides several benefits to manufacturers, including:
- Improved efficiency: By simplifying the product structure, AI-driven DFA techniques reduce the number of assembly steps required, resulting in faster and more efficient assembly processes.
- Reduced costs: Streamlining the assembly process leads to a reduction in labor and assembly time, consequently reducing overall manufacturing costs.
- Enhanced product quality: By optimizing the design for assembly, AI can help minimize potential assembly errors, ensuring higher product quality and reliability.
- Increased profitability: Faster assembly processes and reduced costs contribute to increased profitability for manufacturers.
Implementing AI-driven DFA
To implement AI-driven DFA techniques, manufacturers need to integrate AI platforms or software into their product design process. These AI solutions typically utilize CAD (Computer-Aided Design) software and incorporate DFA analysis tools.
Manufacturers can follow these steps to leverage AI-driven DFA effectively:
- Input the product design into the AI-driven DFA software.
- Configure the desired assembly constraints and manufacturing specifications.
- Run the AI algorithm to analyze the design and suggest modifications.
- Review and evaluate the suggested modifications.
- Implement the approved modifications into the product design.
By following this iterative process, manufacturers can continuously refine their product designs based on AI suggestions, resulting in improved assembly processes and optimized manufacturing efficiency.
Conclusion
The integration of AI technologies in Design for Manufacturing, specifically Design for Assembly, revolutionizes the way manufacturers approach product design and assembly processes. By leveraging AI-driven DFA techniques, manufacturers can simplify the product structure, reduce assembly time, lower costs, and improve overall product quality. As AI algorithms continue to evolve, manufacturers can expect even more advanced and efficient assembly suggestions, further benefiting the manufacturing industry as a whole.
Comments:
Thank you all for taking the time to read my article on optimizing assembly processes using ChatGPT for Design for Manufacturing in 'Design for Assembly' technologies. I'm looking forward to your thoughts and discussions!
Great article, Sam! I found your insights on leveraging ChatGPT for assembly processes quite interesting. It seems like this technology could significantly improve efficiency and reduce errors.
I agree, Lisa. The potential of using AI to optimize assembly processes is huge. It could lead to cost savings and better time management. However, I wonder if there are any limitations to its implementation?
That's a good point, Michael. While AI can greatly enhance efficiency, there might be challenges in terms of training the model to accurately understand complex assembly instructions or adapt to variations in manufacturing setups.
I think another challenge would be ensuring the security and privacy of sensitive assembly data. AI systems like ChatGPT require access to a lot of information, so adequate safeguards need to be in place.
Sam, I enjoyed your article as well. It's fascinating to see how AI can revolutionize the manufacturing industry. Do you think ChatGPT can also assist in product design or just assembly processes?
Thanks, Sarah! Absolutely, ChatGPT can be extended to assist in product design too. It can help generate innovative ideas, provide design recommendations, and even simulate product performance before prototyping.
While the idea of AI assisting in assembly processes is exciting, I'm concerned about potential job losses. Won't this technology replace human workers?
Paul, I can understand your concern. However, I believe AI should be viewed as a tool that complements human workers rather than replaces them. It can handle repetitive and mundane tasks, allowing humans to focus on more creative and complex aspects of assembly.
I agree with David. Automation has always been part of industrial advancements, and it usually leads to the creation of new job roles. AI can augment human capabilities, enabling workers to become more efficient and adaptable.
This article got me thinking about the potential impact of AI on existing assembly line setups. Implementing ChatGPT seems exciting, but it might require significant changes in infrastructure and processes. What are your thoughts?
Rebecca, you raise an important point. Integrating AI technologies like ChatGPT will indeed require adapting existing assembly line setups. There could be initial challenges in integrating the technology but the benefits in terms of improved efficiency make it worth considering.
I see where you're coming from, Rebecca. One possible approach could be to start with pilot projects to evaluate the feasibility and impact of AI integration. This way, any necessary changes can be made gradually and more efficiently.
Sam, great article! I believe AI can also assist in quality control during assembly processes by analyzing real-time data and identifying potential issues. What are your thoughts on this application?
Thanks, Mark! You're right. AI can play a crucial role in quality control as well. By analyzing data from different sensors and cameras, AI systems can quickly detect defects, anomalies, or deviations, improving overall product quality.
I had a question regarding the training process for ChatGPT. How much initial data would be required to train the model for assembly processes? Is it a time-consuming task?
Anna, training ChatGPT can require a large amount of data, including assembly instructions, diagrams, and historical process data. The process can be time-consuming, especially when ensuring the model understands subtle contextual cues required for assembly instructions.
Additionally, the quality and accuracy of the training data are crucial. Incorrect or incomplete data might lead to the model generating inaccurate or unsafe assembly instructions.
One concern I have is the potential bias in the AI model's recommendations. How can we ensure that ChatGPT doesn't reinforce any pre-existing biases in assembly processes?
Emily, you've raised a valid concern. Ensuring AI models like ChatGPT are unbiased requires careful consideration in the training phase. It's important to have diverse and representative data to minimize biases in recommendations and instructions.
I agree with Sam. Regular audits and ongoing monitoring of the AI system's outputs can help identify and rectify any biases that might arise. Openness and transparency in the development process can also address this concern.
Sam, do you think there are any ethical considerations when implementing AI in assembly processes? For example, should there be any regulations or guidelines to govern the usage of AI in this context?
Sarah, ethical considerations are crucial when implementing AI in any domain, including assembly processes. Establishing guidelines and regulations to ensure proper usage, data privacy, and accountability is essential to maintain ethical standards.
I believe collaboration between manufacturers, AI developers, and regulatory bodies is essential to address potential ethical challenges. It's important to strike a balance between innovation and responsible implementation.
In your article, you mentioned leveraging ChatGPT as a tool for Design for Manufacturing (DFM). Could you elaborate more on how this integration can enhance the DFM process?
Daniel, integrating ChatGPT in DFM can offer several benefits. It can assist designers by generating design suggestions that align with assembly constraints, identifying potential manufacturing issues early on, and optimizing the overall product manufacturability.
I can see how leveraging AI in DFM would speed up the iterative design process and help avoid design revisions due to manufacturability limitations. This would save both time and resources.
Sam, the potential benefits of using ChatGPT in assembly processes and DFM are clear. However, what would be the key challenges in implementing AI in the manufacturing industry?
Emma, one key challenge would be addressing the skills gap in the workforce. Manufacturers will need to upskill their employees to work alongside AI systems effectively. Education and training programs would be crucial during this transition.
Another challenge could be the initial investment required to implement AI technologies and infrastructure changes. Manufacturers would need to evaluate the cost-benefit analysis and develop a clear implementation strategy.
I'm curious about the potential limitations of using ChatGPT in assembly processes. Are there any specific scenarios or tasks where it might not be as effective?
Oliver, ChatGPT might face limitations in understanding highly complex or ambiguous assembly instructions, especially when specific contextual cues are required. It might also struggle to adapt to rapidly changing manufacturing setups or deal with unexpected scenarios.
I think ChatGPT's effectiveness might also depend on the quality and accuracy of the training data. Insufficient or biased data could affect its ability to generate reliable and safe assembly instructions.
Sam, I'm curious about the potential scalability of using ChatGPT in a large-scale manufacturing environment. Do you think the system can handle the complexity and volume of instructions?
Emily, scalability is an important consideration. While ChatGPT has shown promise in various domains, including complex environments, scaling it to handle the volume and diversity of assembly instructions across a large-scale manufacturing environment would require continuous improvement and customization.
I agree, Sam. The complexity and diversity of assembly processes in a large-scale environment might require additional fine-tuning and customization of the AI model to ensure optimal performance across different manufacturing setups.
The potential of integrating AI like ChatGPT in assembly processes is exciting, but what steps can manufacturers take to ensure a successful implementation without disrupting existing operations?
Daniel, a gradual approach would be beneficial for successful implementation. Starting with pilot projects, involving key stakeholders, and conducting thorough testing can help identify potential challenges early on and iteratively refine the implementation process.
I think effective change management is essential. Manufacturers should prioritize providing proper training and support to their workforce to ensure a smooth transition to working alongside AI systems.
Thank you all for your valuable insights and questions. It has been a fruitful discussion. The potential of AI in optimizing assembly processes through ChatGPT is substantial, and with proper considerations, its implementation can bring significant improvements to the manufacturing industry.