Optimizing Material Selection in PCB Design with ChatGPT: Revolutionizing the Way We Engineer Circuit Boards
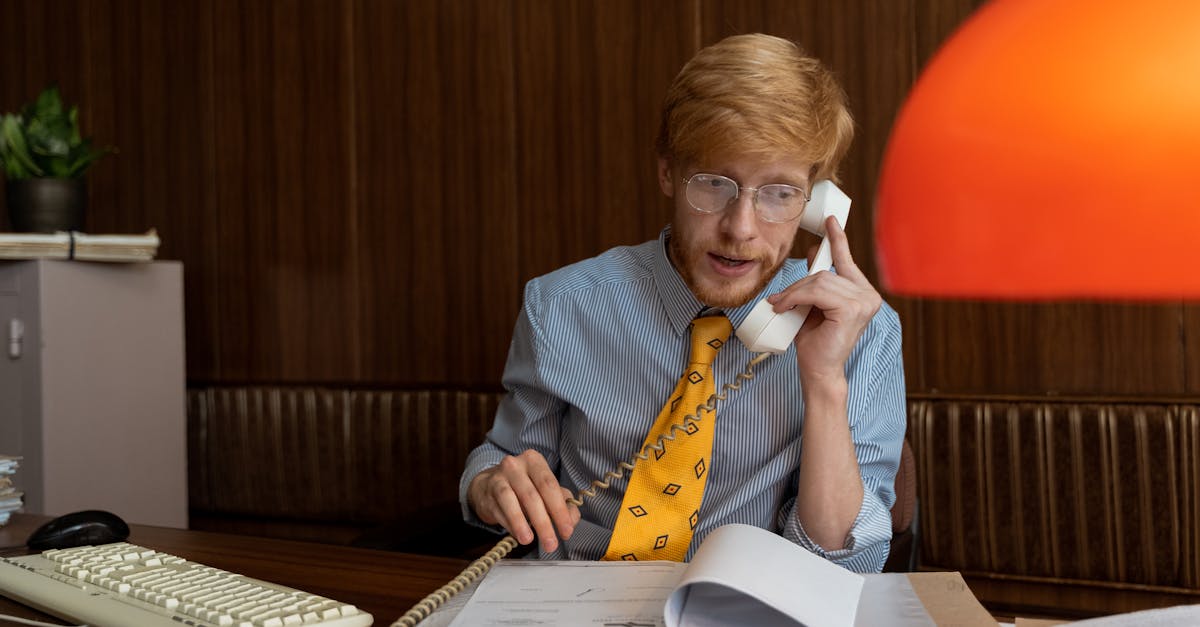
Printed Circuit Board (PCB) design is a crucial aspect of electronic product development. Material selection plays a vital role in determining the overall performance, reliability, and cost-effectiveness of PCBs. With the advancements in artificial intelligence, specifically with the highly anticipated release of ChatGPT-4, designers can now leverage its capabilities to suggest suitable materials for specific board applications.
Traditionally, material selection for PCBs involved extensive research, consultation, and testing. Designers had to consider various factors such as electrical properties, thermal conductivity, mechanical strength, environmental resistance, and cost before making an informed material choice. However, with ChatGPT-4's natural language processing and machine learning capabilities, designers can interact with the AI system to obtain tailored recommendations for their specific design requirements.
ChatGPT-4 can analyze vast amounts of data on different PCB material properties, such as FR-4, metal core substrates, and polyimide, to name a few. By understanding the design specifications, power requirements, operating conditions, and desired performance goals, ChatGPT-4 can suggest the most suitable material options for the application at hand. This greatly accelerates the material selection process, saving designers valuable time and effort.
Another notable advantage of incorporating ChatGPT-4 into the material selection process is its ability to consider emerging trends and new material developments. The AI system can access and analyze the latest research papers, industry reports, and material databases to provide up-to-date suggestions. This ensures that designers have access to cutting-edge materials that may offer improved performance or cost-efficiency compared to traditional options.
ChatGPT-4's ease of use makes it accessible to both experienced PCB designers and those new to the field. Its intuitive interface allows designers to input their design requirements in natural language, making it effortless to communicate with the AI system. Designers can ask specific questions about material properties, compare different options, or seek recommendations based on specific constraints.
While ChatGPT-4 can provide valuable material suggestions, it is important to note that designers should still validate the recommendations through prototyping, testing, and verification. Additionally, designers must consider other aspects such as manufacturing capabilities, supplier availability, and cost limitations.
In conclusion, ChatGPT-4 presents a promising opportunity for PCB designers to streamline the material selection process. By leveraging its natural language processing and machine learning capabilities, designers can receive tailored material recommendations for their specific board applications. The AI system's ability to analyze vast amounts of data, consider emerging trends, and offer intuitive interaction makes it a valuable tool in the PCB design workflow. However, designers should always validate the suggestions through practical experimentation and consider additional factors before finalizing material choices.
Comments:
Thank you all for reading my article! I hope you find it insightful. Feel free to ask any questions or share your thoughts on the topic.
Great article, Zachary! I've been working with PCB designs for years, and incorporating AI like ChatGPT sounds really promising. Can you provide some examples of how it can optimize material selection?
Hi Lisa! Absolutely, ChatGPT can help optimize material selection in PCB design by analyzing various factors such as electrical properties, thermal conductivity, and cost-effectiveness. It can suggest suitable materials based on the specific requirements of the circuit, leading to better overall performance.
Thanks, Zachary! It's really exciting to see how AI can revolutionize the way we engineer circuit boards. Looking forward to implementing ChatGPT in our future projects.
I'm intrigued by the idea of using AI to optimize material selection in PCB design. It could potentially save a lot of time and cost. Zachary, have you seen any significant improvements in circuit board performance with this approach?
Nathan, AI has shown great potential in improving circuit board performance. By analyzing vast amounts of data and simulating different material options, AI algorithms like ChatGPT can identify optimal material choices that can enhance signal integrity, reduce power loss, and increase efficiency in electronic circuits.
That's impressive, Zachary! The ability to optimize material selection using AI would definitely be a game-changer in the field of PCB design.
Thank you, Nathan! The combination of AI and PCB design indeed has the potential to elevate the field and open up new possibilities.
Zachary, I'm curious, what kind of AI models are used in ChatGPT? Are they based on machine learning algorithms?
Nathan, ChatGPT is based on cutting-edge deep learning algorithms. It uses a variant of the Transformer architecture, which has shown remarkable performance in natural language processing tasks. The AI models are trained on a massive corpus of text data to generate relevant and coherent responses.
Zachary, I appreciate the detailed explanation. It's fascinating how AI can leverage the power of modern deep learning algorithms to assist in material selection decisions.
Nathan, it's indeed an exciting intersection of advanced algorithms and PCB design. Machine learning techniques combined with the abundance of PCB data enable AI models to gain valuable insights, helping us make more optimal material choices.
Zachary, the ability to leverage large datasets to enhance material selection decisions is definitely one of the most exciting aspects of using AI. It opens up new possibilities and improves the design process tremendously.
Indeed, Nathan. The availability of vast amounts of data enables AI algorithms to learn from and adapt to various scenarios, leading to better decision-making in selecting optimized materials.
Zachary, it's impressive to see how AI algorithms can handle conflicting requirements and provide recommendations that strike the best balance. This capability has the potential to save designers a significant amount of time and effort.
Indeed, Nathan. The ability of AI to assist in complex decision-making by offering valuable material recommendations is one of its most valuable contributions to PCB design.
Well said, Zachary. AI has the potential to be a valuable ally in the design process, streamlining workflows and enhancing the overall quality of electronic systems.
Thank you, Nathan! It's exciting to witness how AI is transforming traditional processes, and I'm glad to see the interest and positive response from professionals like you in the field of PCB design.
This is fascinating! I'm wondering, Zachary, what are the limitations of using AI in PCB material selection? Are there any scenarios where it might not be as effective or accurate?
Karen, AI's effectiveness in PCB material selection relies on the accuracy of the data fed into the system. In scenarios where the available data is limited or not representative, the AI algorithm may not produce optimal results. It's crucial to ensure the dataset used for training AI models encompasses a wide range of scenarios and covers relevant material properties.
I see, Zachary. So, human expertise is still critical to ensure the AI doesn't overlook critical regulatory factors or fail to consider real-world limitations. That makes sense!
I have some concerns about relying too heavily on AI for material selection. What if the AI algorithm makes errors and suggests inappropriate materials? How can we ensure the reliability of ChatGPT's recommendations?
Absolutely, Michael! While AI can provide valuable insights, it's important to validate the recommendations by cross-referencing them with industry standards, conducting physical tests, and involving human expertise in the final decision-making process.
Indeed, Lisa! Leveraging AI should augment human expertise and not replace it entirely. The synergy between human intelligence and AI-powered tools is the key to successful implementation.
Well said, Michael! AI should be seen as a tool that complements our knowledge and expertise, enabling us to make better-informed decisions and optimize our design processes.
Indeed, Lisa. Combining our expertise with AI algorithms like ChatGPT allows us to tap into the power of data-driven insights and make more informed decisions in PCB design.
Zachary, I'm wondering how AI factors in any specific industry regulations or compliance requirements when suggesting materials for PCB design.
Emily, that's an excellent question. When suggesting materials, AI algorithms can consider specific industry regulations and compliance requirements by cross-referencing the dataset with relevant standards. It helps ensure the recommended materials meet necessary safety, environmental, and reliability standards.
That's good to know, Zachary. It's crucial to consider compliance factors, and it's reassuring that AI can assist in that process.
Has ChatGPT been widely adopted in the industry? I haven't come across it in my circuit board design work yet.
Robert, AI technologies often take time to become widely adopted, but as more success stories and case studies emerge, companies tend to embrace them. ChatGPT is still relatively new, but its potential in optimizing material selection is gaining attention, so keep an eye out for its integration in the industry!
Laura, I agree. It's exciting to see AI technologies gain traction in PCB design. I'll definitely keep an eye out for the integration of ChatGPT or similar AI-powered tools in the industry.
Robert, the integration of AI in PCB design is a promising direction. As AI technologies mature and more success stories arise, we can expect their wider adoption and the subsequent transformation of the design process.
Absolutely, Laura! The future of PCB design looks fascinating, with AI-driven tools paving the way for innovative and optimized solutions.
Robert, AI in PCB design is still relatively new, but there is growing interest across the industry. While ChatGPT may not be widely adopted yet, its potential benefits are starting to gain recognition, and I expect it to become more prevalent in the future as the technology continues to mature.
As an electrical engineering student, this article has sparked my interest in exploring the integration of AI in PCB design. Are there any resources or tools you recommend to get started with AI-powered PCB design?
Ethan, I'm glad to hear you're interested in exploring AI in PCB design! To get started, you can look into open-source machine learning frameworks like TensorFlow and PyTorch. Additionally, there are online courses and tutorials available that specifically focus on AI integration in electronics design. Feel free to reach out if you need more specific recommendations!
Thank you, Zachary! I'll definitely look into those resources and frameworks to kickstart my exploration. If I need more recommendations along the way, I'll be sure to seek your guidance.
Fantastic article, Zachary! The integration of AI in PCB design brings tremendous potential for innovation. Can you share any personal experiences where ChatGPT has helped you achieve remarkable results?
Thank you, Oliver! One notable experience was when ChatGPT suggested a specific material for a high-speed signal path in a design. The material had excellent signal integrity properties, resulting in significantly improved performance and reduced signal distortion. It was truly remarkable to witness the positive impact of AI-powered material selection.
I'm curious about the training process for ChatGPT. Zachary, could you explain how the AI model is trained to suggest optimal materials?
Sophia, the training process of ChatGPT involves exposing the model to a vast amount of data consisting of PCB designs, simulations, material properties, and their corresponding performances. This data is used to teach the model how to associate specific materials with desired circuit behavior, enabling it to generate valuable material recommendations based on the input requirements.
That sounds like a comprehensive training process, Zachary! It's impressive how the model can learn from such immense datasets to provide valuable material recommendations.
Exactly, Zachary! AI-powered tools like ChatGPT can significantly improve efficiency in the design process, allowing us to focus more on creativity and innovative solutions.
This article has piqued my interest in exploring AI for PCB material selection. I'm excited about the potential of ChatGPT to streamline the design process!
Zachary, how does ChatGPT handle conflicting requirements when suggesting materials? For example, if there's a trade-off between signal integrity and cost-effectiveness?
Sara, when facing conflicting requirements, ChatGPT considers multiple factors and uses a weighted approach based on predefined priorities. By assigning importance to different criteria such as signal integrity, cost, and performance, the algorithm ranks and suggests materials that strike the best balance within the given constraints.
I'm curious, Zachary, how long does it usually take for the AI algorithm to suggest optimized materials? Is it a time-consuming process?
Emma, the time required for the AI algorithm to suggest optimized materials depends on the complexity of the design requirements, the scale of the dataset, and the computational resources available. While it may take longer for more intricate designs, advancements in AI algorithms and hardware acceleration techniques make the process increasingly efficient.
Thank you for explaining, Zachary. It's good to know that the time required for the AI algorithm to suggest optimized materials is reasonable and improving.