Optimizing Oilfield Operations: Harnessing the Power of ChatGPT for Predictive Analytics
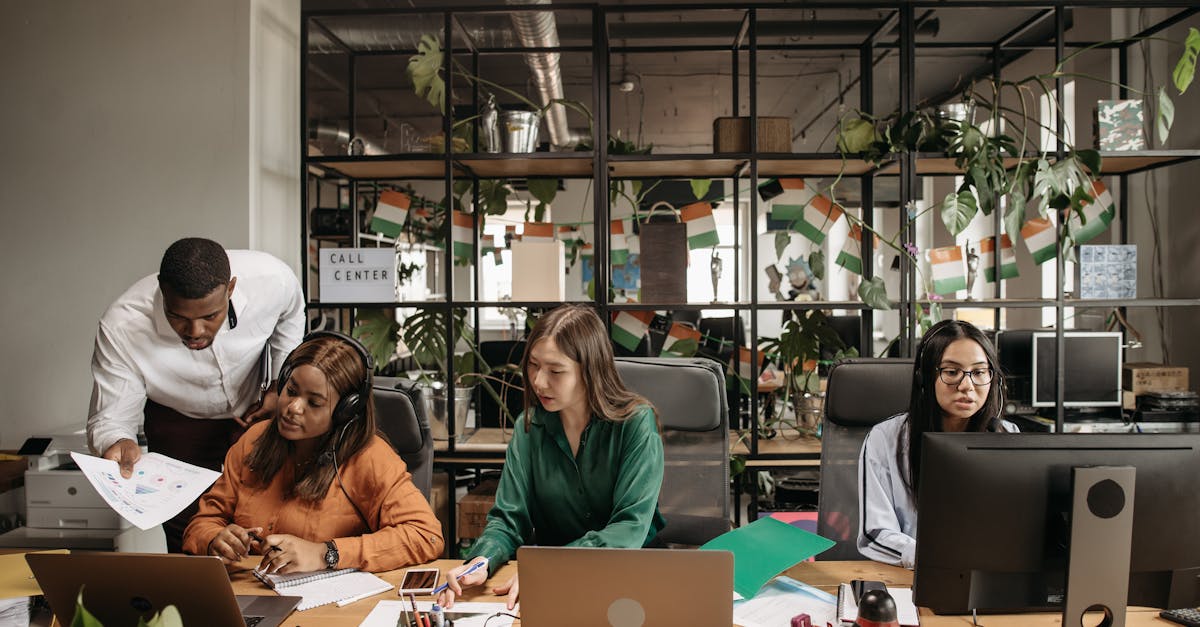
Predictive analytics has become an integral part of the oilfield industry, allowing companies to make informed decisions based on historical and real-time data. With the recent advancements in natural language processing and machine learning, technologies like ChatGPT-4 have emerged as powerful tools in predicting possible future scenarios.
ChatGPT-4 is an AI-powered language model developed by OpenAI. It is designed to understand and generate human-like text responses to various prompts. It uses a combination of structured and unstructured data to provide accurate predictions in real-time.
In the context of the oilfield, ChatGPT-4 can be utilized to forecast production levels, equipment failure, and maintenance schedules. By analyzing historical data, such as well performance, production rates, and maintenance records, the model can identify patterns and correlations that can help predict future outcomes.
Real-time data plays a crucial role in predictive analytics. With the integration of sensors, monitoring systems, and IoT devices, oilfield operators can collect vast amounts of data in real-time. This data includes information such as temperature, pressure, flow rates, and equipment status. ChatGPT-4 can analyze this data and provide insights into potential issues, allowing operators to take preventive measures and minimize downtime.
One of the significant advantages of ChatGPT-4 is its ability to generate text-based reports and alerts. By processing and summarizing complex data, the model can communicate predictions and recommendations in a concise and actionable manner. This enables decision-makers to react quickly to potential risks or opportunities in the oilfield.
Furthermore, ChatGPT-4 can be used to optimize resource allocation. By studying historical data on well performance, production, and maintenance, the model can identify areas where resources can be effectively allocated to maximize efficiency and profitability. This includes managing drilling schedules, equipment deployment, and workforce planning.
However, it is important to note that predictive analytics is not infallible. While ChatGPT-4 can provide accurate predictions based on available data, unforeseen variables and external factors can impact the accuracy of the forecasts. Ongoing monitoring and fine-tuning of the predictive models are necessary to improve their performance over time.
The oilfield industry can hugely benefit from the application of predictive analytics using technologies like ChatGPT-4. By leveraging historical and real-time data, companies can make more informed decisions, prevent costly equipment failures, enhance production efficiency, and optimize resource allocation. As technology continues to evolve, the potential for predictive analytics in the oilfield will only expand, leading to increased operational excellence and profitability.
Comments:
Thank you all for taking the time to read my article on optimizing oilfield operations using ChatGPT for predictive analytics! I'm excited to hear your thoughts and answer any questions you may have.
Great article, Ujjwal! I really enjoyed reading about how ChatGPT can be applied to oilfield operations. The potential for predictive analytics to optimize efficiency is fascinating. Do you have any examples of how ChatGPT has been successfully implemented in the industry?
Thank you, David! I'm glad you found the article interesting. ChatGPT has been successfully utilized in various oilfield applications. One example is its ability to analyze real-time sensor data to predict equipment failures and prevent costly downtime. By detecting patterns and anomalies, it helps in proactive maintenance scheduling.
I appreciate your article, Ujjwal. It's evident that predictive analytics can benefit oilfield operations. However, what challenges are faced while implementing ChatGPT in such a complex and dynamic environment?
Thank you, Maria. Implementing ChatGPT in oilfield operations does come with challenges. The dynamic nature of the industry means that data patterns and conditions constantly change, making it essential to continuously train and update the model. Additionally, ensuring the security and privacy of sensitive data is crucial.
Ujjwal, your article provides valuable insights into the potential of predictive analytics in oilfield operations. How do you think ChatGPT compares to other machine learning models in terms of accuracy and reliability?
Thanks, Daniel! ChatGPT has shown great accuracy and reliability in various domains. While it performs well, it's important to note that it may have limitations when faced with uncommon or unseen scenarios. Continuous model evaluation and monitoring are essential to ensure accurate results.
Ujjwal, your article highlights the benefits of proactive maintenance through predictive analytics. Have you encountered any specific use cases where ChatGPT has significantly reduced equipment failures and maintenance costs?
Thank you, Laura. Yes, there are several use cases where ChatGPT has demonstrated significant improvements. For example, an oil company implemented predictive analytics using ChatGPT with their equipment sensor data, resulting in a 30% reduction in equipment failures and substantial cost savings in maintenance and repairs.
Ujjwal, I find the integration of ChatGPT into oilfield operations intriguing. However, are there any potential risks associated with relying on a machine learning model for critical decision-making?
That's an excellent question, Michael. While ChatGPT offers valuable insights, it's important to acknowledge its limitations. Incorrect predictions or lack of understanding of complex scenarios could result in undesired outcomes. A hybrid approach that combines human expertise and machine learning can help mitigate risks and ensure better decision-making.
Ujjwal, your article opens up a new perspective on leveraging AI in the oil industry. How scalable is implementing ChatGPT, considering the vast amount of data generated in oilfield operations?
Thank you, Sophia. ChatGPT's scalability depends on factors like computational resources and the size of the dataset. With a distributed computing setup and efficient data pipelines, it's feasible to handle large volumes of data. However, organizations must optimize their infrastructure to ensure smooth operations.
Ujjwal, your article emphasizes the role of predictive analytics in optimizing operations. Are there any specific oilfield operations where implementing ChatGPT has proven to be particularly effective?
Thank you, Alex. ChatGPT has been effective in various oilfield operations. Some notable areas include predictive maintenance, real-time anomaly detection, well optimization, production forecasting, and supply chain management. Its versatility makes it applicable to different aspects of the industry.
Ujjwal, your article sheds light on the potential of ChatGPT in the oil industry. How challenging is it to interpret and analyze the insights provided by a machine learning model like ChatGPT?
Thanks, Mark. Interpretability is indeed a challenge with complex machine learning models like ChatGPT. Techniques like sensitivity analysis, model visualization, and feature importance can aid in understanding the model's decision-making process. Interpreting the outputs with domain experts helps in transforming the insights into actionable strategies.
Ujjwal, your article highlights the benefits of using ChatGPT for predictive analytics. Are there any limitations or risks that organizations need to be aware of while implementing such systems?
Thank you, Sarah. While deploying ChatGPT, organizations should consider limitations like data quality, potential biases, interpretability challenges, and robust validation. Ensuring a thorough understanding of the model's strengths and weaknesses, and continuous evaluation, helps mitigate risks and ensures reliable results.
Ujjwal, your article provides valuable insights into optimizing oilfield operations. Do you foresee any advancements or future developments in ChatGPT that could further enhance its applications in the industry?
Thanks, Emily! ChatGPT research is a rapidly evolving field, and we can expect advancements going forward. Enhanced interpretability, better handling of rare events, and improved calibration are some areas where future developments can enhance ChatGPT's reliability and effectiveness in diverse oil industry applications.
Ujjwal, thank you for explaining the implementation of ChatGPT in oilfield operations. I'm curious to know if the model's accuracy and performance deteriorate over time. Is retraining necessary periodically?
You're welcome, David. The model's accuracy and performance can indeed degrade over time due to data drift and changing conditions. Periodic retraining is necessary to ensure the model adapts to new patterns and remains reliable. However, organizations must strike a balance and avoid overfitting by carefully choosing the right retraining frequency.
Ujjwal, thanks for sharing your knowledge about ChatGPT in the oil industry. How do you see the future of AI-driven predictive analytics shaping the oilfield operations landscape?
You're welcome, Daniel. AI-driven predictive analytics will play an increasingly significant role in the oilfield operations landscape. It will enable more informed decision-making, optimized resource allocation, proactive maintenance, reduced downtime, and improved safety. As technology advances, we can expect even more sophisticated applications that revolutionize the industry.
Ujjwal, your article showcases the potential of ChatGPT for predictive analytics. Are there any specific data requirements or challenges that organizations should be aware of before adopting this approach?
Thank you, Laura. Preparing the data for ChatGPT requires a clean and labeled dataset that aligns with the specific objectives. Identifying relevant features, dealing with missing data, and removing biases are crucial steps. Additionally, organizations should ensure data privacy and comply with relevant regulations to protect sensitive information.
Ujjwal, your article paints a compelling picture of the potential impact of ChatGPT in oilfield operations. What are the computational requirements to deploy such a model at scale?
Thanks, Thomas. Deploying ChatGPT at scale requires significant computational resources. High-performance GPUs or specialized hardware accelerators are commonly used to handle the model's large size and complex computations. Additionally, organizations must carefully manage memory usage, storage, and parallel processing to ensure efficient and scalable deployments.
Ujjwal, your article highlights the potential benefits of predictive analytics in the oil industry. What kind of data sources are typically used to train ChatGPT models for oilfield operations?
Thank you, Sophia. ChatGPT models for oilfield operations are typically trained on a combination of historical sensor data, maintenance records, drilling and production reports, equipment logs, and other relevant structured and unstructured data sources. By leveraging diverse data streams, the model can learn patterns and make accurate predictions.
Ujjwal, your article provides valuable insights into the applications of ChatGPT in the oil industry. How long does it typically take to train a model like ChatGPT for oilfield operations?
Thanks, Mark. The time required to train a ChatGPT model for oilfield operations depends on factors like the size of the dataset, complexity of the task, and available computational resources. Training can take several days to weeks, and it often involves an iterative process of fine-tuning and experimentation to achieve the desired performance.
Ujjwal, your article highlights the potential of optimal decision-making through predictive analytics. How important is the quality and granularity of data for accurate predictions?
Thank you, Sarah. The quality and granularity of data play a crucial role in accurate predictions. High-quality, labeled data that captures fine-grained details and represents the underlying dynamics of oilfield operations leads to more reliable insights. Organizations should invest in data collection and preprocessing to ensure the desired level of prediction accuracy.
Ujjwal, your article discusses the potential of applying ChatGPT in oilfield operations. Can you shed some light on the ethical considerations and challenges involved in implementing AI-driven predictive analytics?
Thanks, Alex. Implementing AI-driven predictive analytics comes with ethical considerations. Ensuring fairness, transparency, and accountability in the decision-making process is crucial. Addressing potential biases in data and models, monitoring for unintended consequences, and protecting privacy are key challenges. Organizations must adopt ethical AI principles and continually assess and mitigate ethical risks.
Ujjwal, your article explores the potential of ChatGPT for predictive analytics in oilfield operations. What types of oilfield-specific insights can organizations gain using this approach?
Thank you, Michael. ChatGPT can provide valuable oilfield-specific insights such as failure prediction, anomaly detection, asset performance optimization, production forecasting, preventive maintenance scheduling, and supply chain optimization. These insights enable organizations to make data-driven decisions, reduce costs, enhance productivity, and improve overall operational efficiency.
Ujjwal, your article enlightens us about the potential of ChatGPT in oilfield operations. How do you envision the collaboration between AI models like ChatGPT and human experts in the industry?
Thanks, Emily! Collaboration between AI models like ChatGPT and human experts is vital. Human expertise helps in interpreting and validating the model's outputs, addressing domain-specific nuances, and making complex decisions. The combination of AI-driven insights and human judgment leads to more effective and reliable outcomes in oilfield operations.
Ujjwal, your article discusses the use of ChatGPT in proactive maintenance. Can you elaborate on the benefits of using predictive analytics for maintenance scheduling?
Certainly, Daniel. Using predictive analytics for maintenance scheduling helps organizations transition from reactive to proactive maintenance. By analyzing historical data and patterns, ChatGPT can predict potential equipment failures in advance. This enables scheduled maintenance during planned downtime, avoiding unexpected breakdowns and costly production loss, ultimately improving asset reliability and reducing maintenance costs.
Ujjwal, your article demonstrates the potential of ChatGPT in the oil industry. Are you aware of any challenges related to data integration and interoperability while implementing predictive analytics?
Thank you, Laura. Data integration and interoperability can indeed pose challenges in implementing predictive analytics. Different data sources and formats need to be harmonized and appropriately combined. Dealing with data inconsistencies, missing values, and establishing scalable data pipelines that can handle large volumes are some of the key considerations for successful implementation.
Ujjwal, your article presents intriguing applications of ChatGPT in oilfield operations. How do you see the adoption of AI-driven predictive analytics expanding in the coming years?
Thanks, Thomas. The adoption of AI-driven predictive analytics is expected to expand significantly in the coming years. With advancements in AI research, increased availability of big data, and growing recognition of its benefits, more organizations will leverage predictive analytics to optimize operations, reduce costs, and gain a competitive edge in the oilfield industry.
Ujjwal, your article showcases the potential of ChatGPT in oilfield operations. What steps can organizations take to overcome the initial barriers and implement predictive analytics successfully?
Thank you, Sophia. Overcoming initial barriers involves a systematic approach. Organizations should start by clearly defining objectives, identifying relevant data sources, and investing in data quality and collection. Collaborating with experts in machine learning is crucial for model development and validation. Additionally, aligning the implementation with organizational goals and ensuring commitment from stakeholders are important factors for successful adoption.
Ujjwal, your article provides valuable insights into the impact of predictive analytics in oilfield operations. How can organizations ensure the transparency and explainability of ChatGPT's predictions?
Thanks, John. Ensuring transparency and explainability of ChatGPT's predictions is essential. Techniques like attention visualization, model interpretation, and generating post-hoc explanations can shed light on how the model arrives at its predictions. By providing reasoning and insights into the decision-making process, organizations can gain trust and facilitate better understanding and acceptance of the model's outputs.