Optimizing Recommendation System for Revenue Analysis: The Power of ChatGPT
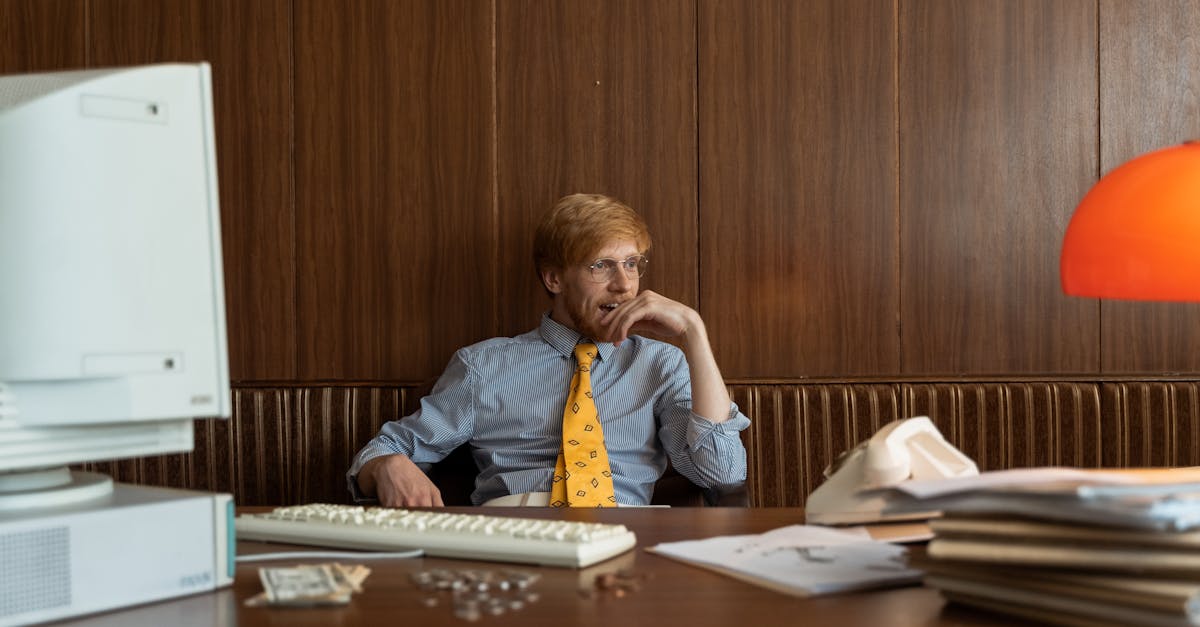
Recommendation systems play a crucial role in the success of e-commerce platforms or any business that relies on providing personalized suggestions to its users. These systems utilize algorithms to analyze user data and deliver relevant product recommendations, ultimately aiming to increase conversion rates and generate higher revenue. With the advent of advanced AI technologies like ChatGPT-4, optimizing recommendation systems for revenue analysis has become even more effective.
Understanding ChatGPT-4
ChatGPT-4 is a powerful language model developed by OpenAI that can assist businesses in various tasks, including revenue analysis. It excels in understanding and generating human-like text, making it an ideal tool for optimizing recommendation systems. By utilizing ChatGPT-4's capabilities, businesses can undertake a comprehensive analysis of customer behavior, preferences, purchase history, and other relevant data to enhance their recommendation algorithms.
Enhancing Recommendation Algorithms
By harnessing the power of ChatGPT-4, businesses can improve their recommendation algorithms by incorporating advanced data analysis techniques. ChatGPT-4 can process large volumes of data and generate insights that might not be readily apparent to human analysts. Its ability to recognize patterns and trends allows for more accurate predictions regarding customer preferences, leading to personalized recommendations that are more likely to convert.
Analyzing Customer Behavior
One key area where ChatGPT-4 excels is in analyzing customer behavior. By understanding how customers interact with a platform and the products offered, businesses can attain valuable insights. ChatGPT-4 can identify and interpret various data points, including browsing history, dwell time, search queries, and even sentiments expressed in customer reviews. With this information, it becomes easier to map customer journeys and tailor recommendations accordingly.
Optimizing Product Relevance
Another crucial aspect of optimizing recommendation systems is ensuring the relevance of product suggestions. ChatGPT-4 can assist in this regard by evaluating different product attributes and customer preferences. It can recognize patterns in customer preferences and suggest related products or alternatives that customers are more likely to purchase. By fine-tuning algorithms using ChatGPT-4's insights, businesses can minimize irrelevant recommendations and improve customer satisfaction.
Increasing Conversion Rates and Revenue
By leveraging the capabilities of ChatGPT-4 to optimize recommendation systems, businesses can significantly increase conversion rates and generate higher revenue. The enhanced relevancy of recommendations drives customer engagement and encourages more frequent purchases. Additionally, personalized suggestions based on customer preferences can foster customer loyalty and result in repeat business, further boosting revenue.
Conclusion
In today's competitive digital landscape, optimizing recommendation systems is crucial for businesses to succeed. By utilizing advanced AI technologies like ChatGPT-4, revenue analysis can be significantly improved. ChatGPT-4's ability to analyze customer behavior, preferences, purchase history, and other data allows for the optimization of recommendation algorithms, resulting in more relevant and personalized product recommendations. Ultimately, this leads to higher conversion rates and increased revenue, providing businesses with a strong competitive edge.
Comments:
Thank you all for your interest in my article on optimizing recommendation systems for revenue analysis using ChatGPT. I'm excited to discuss this topic with you. Please feel free to ask questions or share your thoughts!
Great article, Hitesh! I found it very informative and well-written. The use of ChatGPT for revenue analysis sounds promising. Have you implemented this in any real-world applications yet?
Thank you, Samantha! Yes, we have implemented ChatGPT in a few e-commerce platforms to optimize their recommendation systems. The initial results have been promising in terms of improving revenue generation. However, more testing and refinement are still needed.
Interesting approach, Hitesh! How does ChatGPT handle ethical considerations and biases in recommendations?
A valid concern, Oliver. When using ChatGPT, we actively work on ensuring ethical use and minimizing biases. We have implemented an ongoing monitoring system to identify and rectify any biases that might arise. It's essential to ensure fair and unbiased recommendations to all users.
I love the idea of harnessing AI for revenue analysis! Hitesh, have you observed any major challenges while implementing ChatGPT in recommendation systems?
Absolutely, Sophia! One of the major challenges we faced while implementing ChatGPT was the need for a large and high-quality dataset for training the model. The accuracy and effectiveness of the recommendations heavily rely on the quality and diversity of the data provided during the training phase.
Hi Hitesh! Great article. I'm curious, how does ChatGPT handle privacy concerns when analyzing user behavior to optimize recommendations?
Hi Maxwell! Privacy is of utmost importance to us. When analyzing user behavior, we prioritize anonymizing and aggregating data to protect individual privacy. We also strictly adhere to privacy regulations and ensure that no personally identifiable information is used for recommendation optimization.
Great insight, Hitesh! How scalable is the use of ChatGPT in recommendation systems? Can it handle large-scale e-commerce platforms with millions of users?
Thank you, Liam! ChatGPT is designed to be scalable and can handle large-scale e-commerce platforms with millions of users. However, there are certain infrastructure requirements for optimal performance, such as powerful hardware and efficient parallel processing. Careful system design and architecture are crucial for achieving scalability.
Hello Hitesh! Your article was fascinating. How do you handle situations where user preferences or behaviors change over time?
Hi Emily! User behavior and preferences can indeed change over time. In our recommendation systems, we continuously update and retrain the ChatGPT models based on real-time data. This helps in adapting to changing user preferences and ensuring up-to-date recommendations for users.
Interesting read, Hitesh. Have you compared ChatGPT with other recommendation algorithms, like collaborative filtering or content-based filtering?
Thanks, Ethan! Yes, we have compared ChatGPT with traditional recommendation algorithms like collaborative filtering and content-based filtering. In our experiments, ChatGPT showed significant improvements in terms of revenue generation and recommendation quality. However, it's essential to note that the optimal approach may vary depending on the specific use case and dataset.
Fascinating topic and a well-written article, Hitesh! How do you handle the cold start problem when recommending items to new users?
Thank you, Mia! The cold start problem is a common challenge in recommendation systems. To address it, we utilize a combination of item popularity, similar user behavior, and domain expertise during the initial recommendations for new users. As users engage with the system, the recommendations become personalized based on their interactions and feedback.
Hi Hitesh! I'm curious about the resource requirements of implementing ChatGPT for revenue analysis. Can you shed some light on the computational resources needed?
Hi Nathan! The computational resources required for implementing ChatGPT depend on factors like the size of the dataset, model complexity, and desired response times. For large-scale systems, powerful hardware like high-performance GPUs or distributed computing frameworks might be necessary. It's important to strike a balance between resource allocation and expected performance to optimize revenue analysis effectively.
Great article, Hitesh! How do you handle diversity in recommendations to ensure a user isn't always shown similar items?
Thank you, Chloe! Ensuring diversity in recommendations is crucial to provide a better user experience. We employ techniques like incorporating diversity metrics in our recommendation models, exploring different recommendation strategies, and incorporating serendipity, novelty, and personalization trade-offs. Striking the right balance is essential to offer a mix of familiar and new items to users.
Hi Hitesh! I'm interested in the training process for ChatGPT. How often do you retrain the models to keep them up-to-date?
Hi Aiden! The frequency of retraining the ChatGPT models depends on various factors like the rate of data collection, the pace of changing user preferences, and system requirements. In our implementations, we retrain the models periodically, usually ranging from weekly to monthly, to ensure the recommendations stay relevant and up-to-date.
Impressive work, Hitesh! How do you handle cases where users have diverse interests or belong to different cultures?
Thank you, Luna! Handling diverse user interests and cultural differences is a priority for us. Our recommendation models are designed to take user preferences, feedback, and cultural backgrounds into account. We continuously strive to enhance our understanding of users' individual needs, ensuring a personalized and relevant experience for all.
Hi Hitesh! How does ChatGPT handle sparsity in user-item interactions, especially when dealing with long-tail items?
Hi Sarah! Dealing with sparsity when it comes to long-tail items is indeed a challenge. To address this, we employ various techniques like matrix factorization, item embeddings, and content-based filtering approaches. These techniques can help in capturing user preferences even when interactions with long-tail items are limited.
Hi Hitesh! Loved your article. Are there any specific domains or industries where ChatGPT has shown exceptional results for revenue analysis?
Hi Emma! ChatGPT has shown promising results across various domains and industries. Specifically, we have observed exceptional results in e-commerce, media streaming, and online marketplaces. However, the potential for revenue analysis using ChatGPT extends to many other industries as well, depending on the specific use case and dataset.
Great article, Hitesh! How do you approach the evaluation of recommendation systems powered by ChatGPT?
Thank you, Nora! Evaluating recommendation systems is a multi-faceted process. We employ a combination of offline evaluation metrics like precision, recall, and novelty, as well as user-centric evaluations such as A/B testing, user feedback, and conversion rates. The evaluation process helps us assess the effectiveness, relevancy, and revenue impact of the recommendation system.
Hello Hitesh! I really enjoyed your article. How do you handle situations where users want to discover new items but also expect recommendations based on their previous purchasing history?
Hi Isabella! Balancing user expectations regarding new item discovery and recommendations based on past history is crucial. We employ a hybrid approach that combines collaborative filtering techniques, content-based recommendations, and techniques like item popularity and trending items to strike a balance. It's important to provide a mix of personalized recommendations and opportunities for exploration.
Impressive article, Hitesh! How do you handle online user feedback to continuously improve the recommendation systems?
Thank you, Adam! Online user feedback is invaluable for continuous improvement. We have built a feedback loop where users can rate recommendations, provide explicit feedback, or report any issues. This feedback is then utilized to refine the models, identify areas of improvement, and provide more relevant and accurate recommendations.
Hi Hitesh! Very interesting read. How do you handle situations where users have very limited historical data?
Hi Lucas! When users have limited historical data, we leverage various techniques like content-based filtering, item similarity, and popularity to provide initial recommendations. As users engage with the system and provide feedback, the recommendations become more personalized and adaptive.
Hello Hitesh! I found your article informative. Do you plan to explore any other AI models for revenue analysis in the future?
Hi Sofia! Absolutely, we are always exploring new AI models and techniques for revenue analysis. The field is rapidly evolving, and we are keen to stay at the forefront of innovation. Our focus remains on continuously improving the accuracy and effectiveness of recommendation systems to drive revenue growth for businesses.
Great work, Hitesh! How do you handle real-time recommendation updates to ensure up-to-date and timely recommendations?
Thank you, Ava! Real-time recommendation updates are crucial to provide up-to-date recommendations. We leverage caching mechanisms, batch processing, and real-time user interactions to ensure timely updates. By continuously monitoring user behavior and transaction data, we strive to deliver recommendations that reflect the most recent preferences and trends.
Hi Hitesh! Your article was very insightful. How do you handle situations where users have multiple accounts or profiles?
Hi Alexis! Handling situations where users have multiple accounts or profiles requires careful identification and consolidation of user data. We employ techniques like user merging, cross-account analysis, and identity resolution to ensure recommendations are personalized and relevant, while avoiding duplicate or conflicting recommendations.
Great article, Hitesh! How do you handle dynamic pricing when optimizing revenue analysis?
Thank you, Benjamin! Dynamic pricing plays a crucial role in revenue optimization efforts. We integrate real-time pricing data, demand forecasting models, and pricing strategies into our recommendation systems. By dynamically adjusting prices based on factors like market demand, user preferences, and competition, we aim to maximize both revenue and user satisfaction.
Hello Hitesh! I enjoyed reading your article. How do you handle outlier user behaviors when optimizing recommendations for revenue?
Hi Mila! Handling outlier user behaviors is important to avoid skewing recommendations. We employ outlier detection techniques like statistical analysis, anomaly detection, and clustering to identify and filter out extreme or inconsistent data points. This helps prevent outliers from unduly influencing the recommendation algorithms and ensures a more accurate revenue analysis.
Impressive work, Hitesh! How do you handle recommendations for new or niche items with limited historical data?
Thank you, Henry! Recommendations for new or niche items with limited historical data can be challenging. In such cases, we leverage techniques like content-based recommendations, item characteristics, and similarity measures to provide initial recommendations. As user interactions and feedback accumulate, the system becomes better at making personalized recommendations for those items.
Thank you all for the engaging discussion and valuable questions! I appreciate your interest in the topic and hope this article has shed some light on the power of ChatGPT for revenue analysis. Feel free to reach out to me if you have any further questions or would like to explore this topic in more detail!