Optimizing Urban Planning with ChatGPT: Leveraging Spatial Databases for Sustainable Development
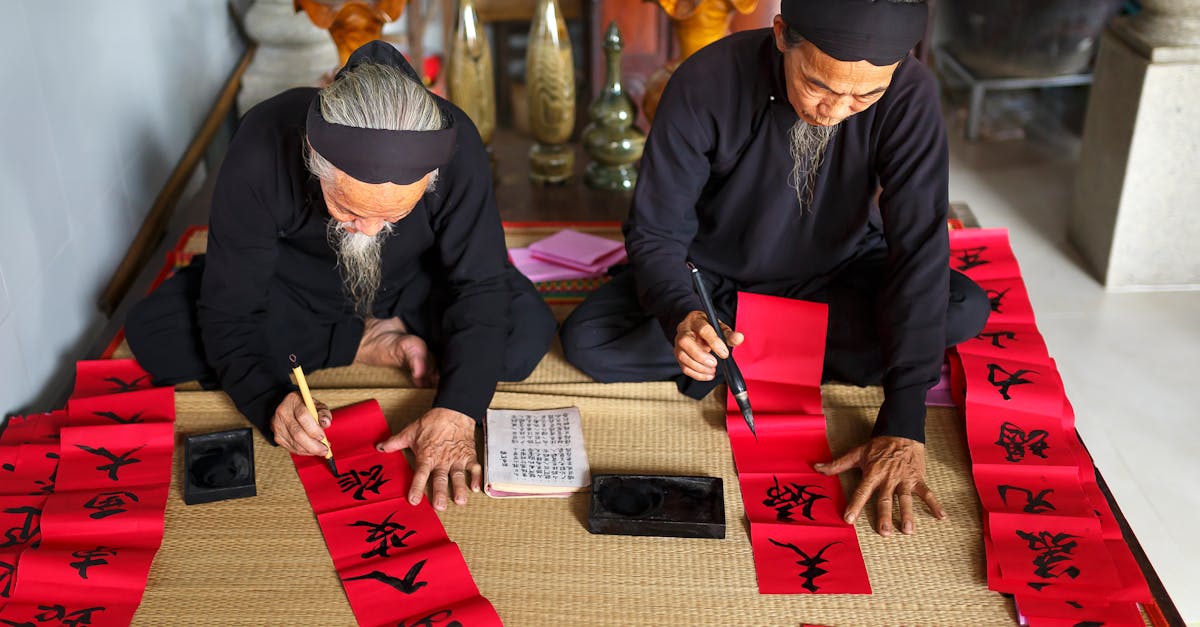
Introduction
In recent years, the field of urban planning has seen tremendous advancements in technology. One technology that has significantly contributed to the process of planning and development of urban areas is spatial databases. These databases, when combined with artificial intelligence and machine learning algorithms such as ChatGPT-4, have the potential to revolutionize urban planning and design.
What are Spatial Databases?
Spatial databases are specialized databases designed to store and manage spatial or geographic data. They allow for the storage and retrieval of data related to geographical locations, such as coordinates, boundaries, distances, and topography. By leveraging spatial databases, urban planners can access and analyze large volumes of spatial data, facilitating informed decision-making and enabling better planning strategies.
How does ChatGPT-4 Benefit from Spatial Databases?
ChatGPT-4, an advanced language model developed by OpenAI, can process spatial data stored in spatial databases to provide accurate predictions and insightful recommendations. By integrating spatial databases with ChatGPT-4, urban planners can leverage the model's ability to understand and generate human-like responses based on the analysis of spatial data.
Application in Urban Planning
The integration of ChatGPT-4 with spatial databases can have several applications in the field of urban planning:
1. Predictive Modeling: By analyzing past urban development patterns and spatial data, ChatGPT-4 can generate predictive models to forecast the future growth and evolution of urban areas. These predictions can help urban planners make informed decisions regarding land use, infrastructure development, and resource allocation.
2. Optimal Route Planning: By using spatial databases, ChatGPT-4 can suggest optimal routes for transportation systems, taking into account various factors such as traffic flow, distance, and accessibility. This can aid in reducing congestion, improving efficiency, and minimizing environmental impact.
3. Identifying Areas for Improvement: Spatial data can be used to identify areas within urban regions that require improvement or redevelopment. By analyzing this data, ChatGPT-4 can provide insights on which areas need attention, allowing urban planners to prioritize their efforts and allocate resources effectively.
Conclusion
With the rapid advancements in spatial databases and the capabilities of ChatGPT-4, the field of urban planning can benefit greatly from the integration of these technologies. By harnessing the power of spatial data analysis and AI-generated insights, urban planners can make more informed decisions, leading to the sustainable and efficient development of urban areas. The future of urban planning is undoubtedly intertwined with the advancements in spatial databases and AI, and it is an exciting time to witness the transformation of our cities.
Comments:
Thank you all for taking the time to read my article on Optimal Urban Planning with ChatGPT. I'm excited to hear your thoughts and engage in this discussion!
Great article, Jeremy! The idea of leveraging spatial databases for sustainable development is intriguing. It can certainly help in making data-driven decisions. Have you been involved in any real-world projects that have implemented this approach?
Thank you, Emily! Yes, I have worked on a project in collaboration with a city's urban planning department. We utilized a spatial database to store various urban data and leveraged ChatGPT to assist decision-makers in generating sustainable development plans. The results were promising, and it provided valuable insights.
Jeremy, it's impressive to hear about your real-world project experience. How did decision-makers find the results generated by ChatGPT in terms of accuracy and usefulness?
Jeremy, I'm curious about the accuracy and reliability of the results generated by ChatGPT. Did you validate the outputs against existing urban planning models or historical data?
Emily, validating the results is crucial. We compared the outputs generated by ChatGPT with existing urban planning models and historical data. While it showed a high degree of accuracy, it's important to note that it serves as an assistive tool rather than a standalone solution. Human expertise and judgment are integral to the decision-making process.
Jeremy, thanks for your response. I'm glad to hear that there are options for smaller cities. Are there any specific resources or learning materials you would recommend for those interested in implementing ChatGPT for urban planning?
Oliver, there are several resources available for learning about ChatGPT and its applications in urban planning. I recommend exploring research papers on Natural Language Processing, attending conferences, and joining relevant online communities for knowledge sharing. Additionally, the OpenAI website provides valuable information and resources to understand and implement ChatGPT effectively.
Jeremy, thank you for the guidance! I'll explore those resources to gain a better understanding of ChatGPT's application in the urban planning domain. Exciting times ahead!
Jeremy, fantastic work! Could you provide more details on the process of training and fine-tuning ChatGPT for urban planning projects? Did it require domain-specific expertise?
Adam, training ChatGPT for urban planning projects required collaboration between domain experts and AI specialists. The domain experts provided valuable insights on urban planning principles, relevant indicators, and data sources. This collaboration enabled us to customize the training process, fine-tune the model, and ensure it aligned with the specific needs of urban planning in different contexts.
Jeremy, thanks for shedding light on the training process. Collaborating with domain experts to fine-tune the model ensures that it is aligned with the specific needs of urban planning. It strikes a balance between human expertise and the capabilities of AI.
Jeremy, it's relieving to hear that the ChatGPT outputs were validated against existing models and historical data. Trust and accuracy are vital when it comes to decision-making in urban planning, and these measures ensure the results are reliable.
Jeremy, optimizing the model architecture and using efficient algorithms for scalability sounds wise. Can you share any specific techniques or approaches that yielded good results in your projects?
Carlos, absolutely. One key technique was the use of graph algorithms to efficiently process spatial data and perform complex operations. This approach allowed us to analyze relationships between various urban elements effectively. Additionally, employing parallel computing techniques and data compression algorithms helped optimize computation time and storage requirements.
Jeremy, I believe community engagement is vital in urban planning. How does integrating ChatGPT with spatial databases facilitate public input and participation?
Samantha, you're right! Community engagement is crucial, and ChatGPT can play a role in facilitating public input. By analyzing community-related data, sentiment analysis, and incorporating user feedback, we ensure that the decision-making process becomes more inclusive and reflective of community needs and aspirations. It's an exciting way to involve citizens in the planning process.
Jeremy, I'm curious about the computational requirements when using ChatGPT for urban planning. Did you encounter any performance or resource limitations while implementing these projects?
David, computational requirements can vary based on the scale of the project and the complexity of the urban data being analyzed. As the datasets grew, we had to adopt parallel computing techniques and leverage distributed systems to ensure the performance remained optimal. Additionally, optimizing the algorithms for computational efficiency helped address resource limitations.
Jeremy, thanks for the detailed insights into computational requirements. It's crucial to balance performance and resources for large-scale projects. Your approach of utilizing parallel computing and optimization techniques offers valuable strategies for others working in this arena.
David, Jeremy mentioned earlier that they employed distributed systems to handle computational requirements effectively. By scaling horizontally and utilizing cloud platforms, they managed to address performance and resource limitations. An optimal configuration of resources can make a significant difference.
Thanks for sharing, Jeremy. Optimizing computation time is crucial in handling large urban datasets. How did you ensure the algorithms used in the project were efficient and performed well in practice?
Maria, understanding the computational complexity of algorithms is crucial. In our projects, we thoroughly analyzed the algorithms used for tasks like spatial analysis, data clustering, and prediction to ensure their efficiency. Additionally, we conducted benchmarking and optimized the implementation code to further enhance performance. Constant evaluation and improvements were key to achieving practical effectiveness.
Jeremy, your article is quite informative. From an environmental perspective, how can ChatGPT and spatial databases contribute to sustainable development by minimizing the adverse ecological impact of urbanization?
Robert, excellent question! ChatGPT and spatial databases can support sustainable development by analyzing ecological factors like green space distribution, air quality data, and energy consumption. By integrating environmental indicators into the decision-making process, urban planners can make informed choices to minimize ecological impact, promote efficient resource usage, and foster a more sustainable urban environment.
Jeremy, that's fantastic! By considering ecological factors in urban planning, we can move towards creating sustainable and environmentally-friendly cities. Thanks for your response!
Samantha, I fully agree on the importance of community engagement. Technology should serve as an enabler rather than a substitute for participatory processes. By incorporating user feedback into ChatGPT models, we can ensure that the planning process aligns with the community's needs.
Jeremy, thanks for sharing your experiences. Employing graph algorithms for spatial data analysis and leveraging parallel computing techniques and data compression algorithms provide valuable insights into the practical optimizations required for successful urban planning projects.
Carlos, you're most welcome! Optimizing performance and computation is indeed crucial for practical implementations. The combination of graph algorithms, parallel computing, and data compression helped us process large urban datasets efficiently and enabled more effective decision-making.
Carlos, Jeremy's approach of employing graph algorithms, parallel computing, and data compression showcases the practical techniques required for effectively handling large-scale urban datasets. These optimizations ensure better performance and enhance the overall urban planning process.
Hey Jeremy, excellent write-up! I completely agree with the potential benefits of integrating ChatGPT and spatial databases. It can greatly enhance the urban planning process. Do you think this approach could also help address issues of traffic congestion and public transportation planning?
Hello, Daniel! Absolutely, tackling traffic congestion and optimizing public transportation planning are among the key areas where ChatGPT and spatial databases can be immensely useful. By analyzing various factors such as population density, transportation infrastructure, and demand patterns, we can make informed decisions to alleviate these challenges.
Jeremy, I assume incorporating real-time data plays a critical role in ensuring the accuracy of the results. Could you share any insights into how you handled and integrated real-time urban data into the spatial database?
Daniel, you're correct. Real-time data is essential for accurate results. In our project, we integrated APIs that provide real-time urban data such as traffic volume, public transportation updates, and demographic changes. These data sources were continuously updated and incorporated into the spatial database, ensuring the model made informed decisions based on the latest information.
Daniel, I can see immense potential in leveraging spatial databases and ChatGPT for optimizing public transportation. It can help identify areas where transportation infrastructure is lacking, estimate demand, and suggest improvements. I'm excited to see how this approach can shape smarter cities!
Sophie, absolutely! Public transportation optimization is a crucial aspect of urban planning. By leveraging ChatGPT and spatial databases, we can design transportation networks that are efficient, sustainable, and cater to the changing needs of the population. It's an exciting frontier!
Daniel, indeed! Efficient and sustainable public transportation networks can significantly enhance urban mobility and reduce both traffic congestion and greenhouse gas emissions. The integration of ChatGPT and spatial databases offers exciting possibilities in this area!
Sophie, precisely! Optimizing public transportation is an integral part of creating sustainable cities. By harnessing the power of AI, we can make better-informed decisions that positively impact urban mobility, accessibility, and environmental sustainability.
Daniel, I agree that addressing traffic congestion is crucial. By leveraging spatial databases and ChatGPT, authorities can consider factors like public transportation options, traffic volume, and road infrastructure to develop comprehensive solutions. It's an exciting prospect!
Alice, I couldn't agree more! It's vital to have a holistic approach to tackle traffic congestion in urban areas. Utilizing advanced technologies like ChatGPT and spatial databases can aid authorities in making more informed decisions that positively impact the community's mobility.
Jennifer, I completely agree. By embracing advanced technologies, urban planning can become more comprehensive and community-centric. It's exciting to witness the positive impact it can have on mobility and overall quality of life!
Alice, definitely! The combination of traditional planning approaches with modern technologies brings us closer to creating sustainable and livable cities. It's inspiring to see the possibilities that emerging technologies like ChatGPT offer in this domain.
Hi Jeremy. Your article is thought-provoking. I'm curious about the scalability of using ChatGPT for urban planning projects. Have you encountered any limitations or challenges when it comes to handling large-scale datasets?
Hi, Linda! Scalability is indeed an important consideration when implementing ChatGPT for urban planning. While handling large-scale datasets can pose challenges, we employed techniques like data partitioning and distributed computing to address them. Additionally, optimizing the model architecture and using efficient algorithms helped mitigate scalability issues.
Jeremy, your article highlights the potential of ChatGPT in urban planning. I'm wondering how accessible this technology is for smaller cities with limited resources. Are there any cost-effective alternatives or simplified versions available?
Jeremy, I'm intrigued by this approach. How does ChatGPT handle the dynamic changes in urban environments? Urban planning requires adapting to evolving needs over time, including demographic shifts and emerging technologies.
Dylan, excellent question! ChatGPT's ability to handle dynamic changes is one of its strengths. By continuously updating the spatial database with new data and training the model regularly, we ensure that it adapts to the evolving environment accurately. It effectively accounts for changing demographics and technological advancements.
Jeremy, that sounds promising! By keeping the model and spatial database up-to-date, the approach can indeed adapt to changing urban environments effectively. Thanks for addressing my concern!
Linda, scalability is indeed a concern when dealing with large-scale urban datasets. However, I believe advances in cloud computing and distributed systems can help overcome these challenges. Jeremy, did you utilize any specific cloud platforms for your projects?
Rachel, you're right. Cloud platforms and distributed systems can definitely help tackle scalability challenges. In our project, we utilized a combination of AWS and Google Cloud Platform for efficient data processing and analysis.