Revolutionizing Agriculture with Machine Vision: Harnessing the Power of ChatGPT in Crop Monitoring and Analysis
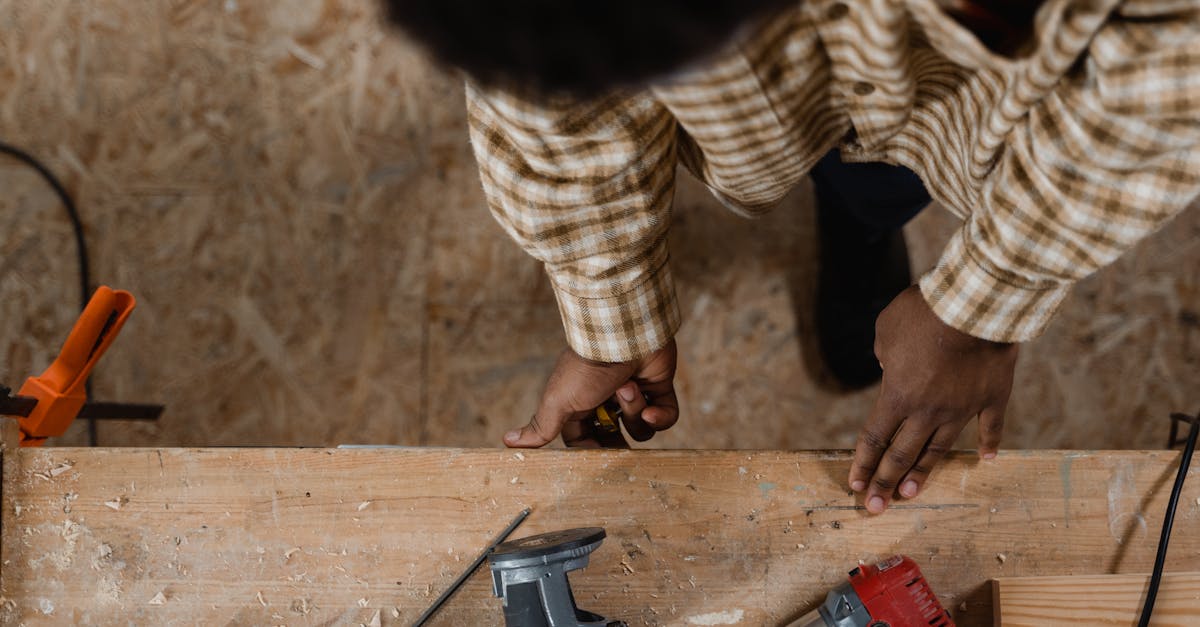
In recent years, advancements in technology have revolutionized various industries, and agriculture is no exception. One such technology that has gained significant traction in the agricultural sector is Machine Vision. Through the use of intelligent algorithms and image processing techniques, Machine Vision has proven to be a valuable tool in detecting and mitigating issues related to pests, diseases, and deficiencies in crops.
The Power of Machine Vision
Machine Vision refers to the ability of a computer system to analyze and interpret images or visual data in a manner similar to human vision. The technology involves the integration of cameras or sensors with sophisticated algorithms that can extract information from images and make informed decisions based on the data obtained.
In the agriculture sector, Machine Vision has been increasingly used to identify and assess various crop-related problems, such as pests, diseases, and nutrient deficiencies. By capturing images of crops with high-resolution cameras or drones equipped with advanced sensors, Machine Vision systems can analyze these images and provide farmers with valuable insights.
Identifying Pests, Diseases, and Deficiencies
One of the main applications of Machine Vision in agriculture is the identification of pests, diseases, and deficiencies in crop images. By analyzing the images captured by cameras or drones, Machine Vision algorithms can detect subtle changes in the appearance of crops that may indicate the presence of pests, diseases, or nutrient deficiencies.
For example, ChatGPT-4, an advanced machine learning model developed by OpenAI, can be trained to analyze crop images and identify specific diseases, pests, or deficiencies. Farmers can capture images of their crops using a smartphone or a dedicated camera and submit them to the ChatGPT-4 system for analysis. The system then analyzes the images and provides a comprehensive report indicating any issues that may require attention.
Early Detection and Mitigation
One of the key advantages of using Machine Vision in agriculture is its ability to detect issues at an early stage. By identifying pests, diseases, or deficiencies in crops before they become severe, farmers can take appropriate measures to mitigate the problems and prevent significant crop yield losses.
With the help of Machine Vision systems like ChatGPT-4, farmers can receive real-time notifications and recommendations based on the analysis of crop images. These recommendations may include specific treatments, interventions, or adjustments to farming practices to address the identified issues. By acting proactively, farmers can optimize crop health and minimize the adverse effects of pests, diseases, or deficiencies.
Conclusion
Machine Vision technology, particularly the use of intelligent algorithms like ChatGPT-4, has opened up new possibilities in the field of agriculture. By harnessing the power of image processing and artificial intelligence, farmers can now identify and mitigate issues such as pests, diseases, and deficiencies more effectively and efficiently. Through early detection and timely intervention, Machine Vision can contribute significantly to the overall health and productivity of crops, ultimately benefiting farmers and ensuring sustainable agricultural practices.
Comments:
Thank you all for joining the discussion! I'm glad to see such enthusiasm for revolutionizing agriculture with machine vision. Please feel free to share your thoughts and ask any questions.
Machine vision in agriculture has huge potential. It can help optimize crop monitoring and analysis, leading to increased efficiency and better yields. Exciting times!
I absolutely agree, Adam. Machine vision can significantly reduce manual labor and provide real-time insights for crop management. Can't wait to see it implemented widely!
But what about the cost? Implementing machine vision technology may require significant investment, especially for small-scale farmers. Do you think it's worth it for them?
That's a valid concern, Roger. While initial costs may be a hurdle, the long-term benefits of enhanced productivity and reduced labor can outweigh the investment. Moreover, as technology advances, costs tend to decrease over time.
I think government support and subsidies could help small-scale farmers adopt machine vision technology. It's essential to ensure equal access and opportunities for all.
Definitely, Hannah. Public-private partnerships and government initiatives can facilitate the adoption of such technologies, making them accessible to farmers of all scales.
Machine vision can also assist in early detection of diseases or pests, allowing timely preventive measures. It has the potential to revolutionize crop protection.
Absolutely, Oliver. Quick identification of issues means better chances of mitigating potential damage before it spreads throughout the crop. Prevention is always better than cure!
I wonder how accurate machine vision systems are in different environmental conditions? Can they adapt to variations in weather, lighting, or other factors?
Great question, Liam! Machine vision systems can be calibrated to account for different environmental conditions. By using advanced algorithms and adaptive techniques, they can adjust to variations in lighting, weather, and other factors, ensuring accurate analysis.
Machine vision could also help reduce the use of chemical inputs by enabling targeted treatments only where necessary. This aligns with sustainable farming practices.
Indeed, Emma. Precision agriculture techniques empowered by machine vision can optimize resource allocation, minimize waste, and promote environmentally friendly practices. It's a win-win situation!
I hope that the data collected through machine vision in agriculture remains secure and private. Privacy concerns are often raised when it comes to new technologies and data management.
You're absolutely right, Sophia. Data privacy and security are crucial considerations. As machine vision becomes more prevalent, industry standards and protocols need to be established to protect farmers' data and ensure compliance with privacy regulations.
I'm curious about the scalability of machine vision in agriculture. Can it be applied to various types of crops and farming methods?
Great question, David. Machine vision technology can be adapted for different types of crops and farming methods. Whether it's row crops, orchards, or greenhouse cultivation, the underlying principles can be customized to suit specific needs.
That's impressive! It opens up possibilities for machine vision to be integrated into diverse agricultural systems, benefiting a wide range of farmers.
Do you foresee any potential limitations or challenges in implementing machine vision widely in agriculture?
Certainly, Ella. While machine vision holds immense potential, challenges could include high initial costs, the need for skilled technicians to set up and maintain the systems, and the requirement for robust connectivity, particularly in rural areas. Overcoming these challenges will be crucial for widespread adoption.
I'm concerned about the potential job displacement caused by increasing automation in agriculture. How can we address this issue?
Valid concern, Sophie. The rise of new technologies often raises questions about jobs. While some tasks may be automated, the implementation of machine vision in agriculture can create new job opportunities in data analysis, system management, and maintenance. Additionally, reskilling and training programs can help people transition into these emerging roles.
I agree with your point, Nell. It's important to focus on upskilling and developing new skill sets that align with the changing agricultural landscape.
Could machine vision technology be used to help small-scale farmers in developing countries, where resources are often limited?
Absolutely, Alexandra. Machine vision can be particularly useful in developing countries where small-scale farmers form a significant portion of the agricultural workforce. By providing cost-effective tools and real-time insights, it can empower these farmers to improve crop productivity and make informed decisions.
What are the current limitations of machine vision technology in agriculture, and how can they be overcome?
Good question, Ethan. Some limitations include data processing speed, the need for high-quality imaging, and the complexity of analyzing diverse crop types. Overcoming these challenges requires advancements in hardware, algorithms, and continuous research collaboration between experts in computer vision and agriculture.
Additionally, building extensive databases for training and fine-tuning machine vision models could further improve accuracy and performance.
I agree, Liam. Access to diverse and comprehensive datasets is crucial for training machine vision models. Collaborative efforts between researchers, industry, and farmers can help establish and maintain such databases.
The collaboration you mentioned is essential. Bringing together different stakeholders can lead to holistic solutions and address the specific challenges faced by farmers in different regions.
Absolutely, Hannah. Collaboration and knowledge-sharing enable us to leverage the collective wisdom of industry experts, researchers, and farmers, fostering innovation and driving sustainable agricultural practices.
I'm excited to see the transformative impact of machine vision in agriculture unfold in the coming years. It has the potential to revolutionize food production and improve global food security.
Indeed, Adam. By harnessing the power of machine vision, we can unlock new possibilities for agriculture, ensuring efficient resource utilization, minimizing waste, and ultimately, enhancing food production to meet the demands of a growing global population.
I have seen some successful use cases of machine vision in precision weed control. It's fascinating how it can distinguish between crops and weeds to target specific areas for treatment.
Indeed, Olivia. Machine vision can accurately identify and classify weeds, enabling targeted treatment. This not only reduces the reliance on herbicides but also minimizes the impact on the environment, making agriculture more sustainable.
That's incredible! Precision weed control can significantly improve the efficiency of crop management and reduce the manual labor required for weed removal.
You're absolutely right, Ella. By automating weed control, farmers can allocate their time and resources more efficiently, focusing on other essential tasks while reducing costs.
I wonder if there are any challenges in integrating machine vision with existing agricultural machinery and equipment?
Good point, David. Integration can be a challenge, as it requires compatibility between machine vision systems and existing agricultural machinery. However, with advancements in technology and engineering, we can expect increased interoperability and seamless integration in the future.
That's reassuring. Compatibility and ease of integration are essential to ensure a smooth transition and widespread adoption of machine vision technology.
Thank you all for your valuable comments and insights. It has been a wonderful discussion. Let's continue to explore the possibilities and work towards a more sustainable future in agriculture!