Revolutionizing Analysis of Protein-Ligand Interactions: Unlocking the Potential of ChatGPT in Biochemistry
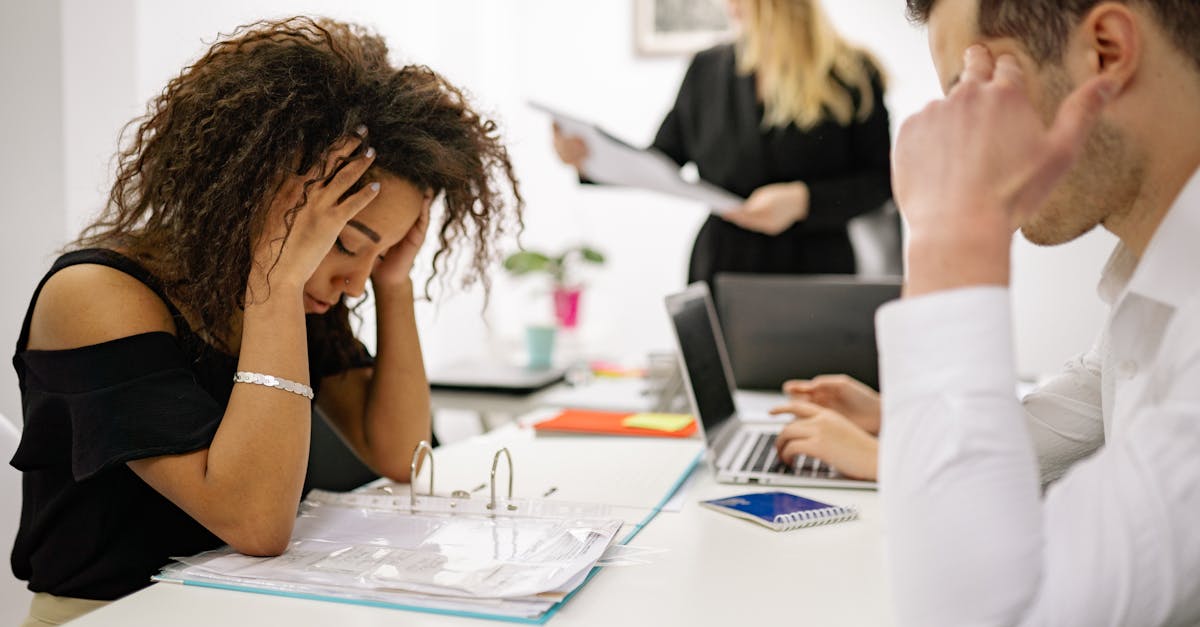
Introduction
In the field of biochemistry, the study of protein-ligand interactions plays a crucial role in understanding various biological processes and developing new drugs. These interactions refer to the binding of small molecules, called ligands, to proteins, which can influence the functional properties of both entities. With advancements in technology, predictive models have emerged to assist researchers in assessing protein-ligand binding affinities. One such technological breakthrough is ChatGPT-4, a language model that leverages artificial intelligence to predict and guide the design of small molecule inhibitors and approaches for lead optimization.
Understanding Protein-Ligand Interactions
Proteins are macromolecules responsible for performing numerous essential functions in biological systems. Ligands, on the other hand, are typically small molecules that bind to specific protein targets. The binding occurs through various non-covalent interactions, such as hydrogen bonding, hydrophobic interactions, and electrostatic forces. Protein-ligand interactions are fundamental to many biological processes, including enzymatic reactions, signal transduction, and gene expression regulation.
Predicting Protein-Ligand Binding Affinities
Accurately predicting protein-ligand binding affinities is crucial for drug discovery and development. Traditionally, experimental methods like X-ray crystallography and nuclear magnetic resonance (NMR) spectroscopy have been employed to evaluate these affinities. However, these methods can be time-consuming and expensive. With the advent of computational techniques, the field of predictive modeling has gained significant momentum.
ChatGPT-4, equipped with a deep understanding of biochemistry and molecular interactions, is a cutting-edge technology capable of predicting protein-ligand binding affinities. By analyzing the structural and physicochemical properties of both proteins and ligands, ChatGPT-4 can provide researchers with valuable insights into the strength and stability of interactions. It utilizes deep learning algorithms and a vast arsenal of training data to generate predictions based on learned patterns and relationships.
Designing Small Molecule Inhibitors
Small molecule inhibitors play a crucial role in drug discovery, particularly in designing therapeutics against target proteins involved in diseases. ChatGPT-4 can assist in the design of small molecule inhibitors by suggesting suitable ligand structures that interact favorably with specific protein targets. By predicting binding affinities and understanding the intricacies of protein-ligand interactions, researchers can optimize the structure and properties of potential inhibitors to enhance their effectiveness and selectivity.
Optimizing Lead Compounds
Lead optimization is a crucial step in the drug discovery process. It involves refining and improving the properties of initial lead compounds to enhance their binding affinity, selectivity, and pharmacokinetic properties. ChatGPT-4 can provide valuable guidance in lead optimization by suggesting modifications to lead compounds, identifying potential off-target effects, and exploring alternative binding modes. Such insights greatly expedite the drug discovery process and increase the chances of developing successful therapeutic agents.
Conclusion
With the rapid advancements in artificial intelligence and bioinformatics, ChatGPT-4 has emerged as a powerful tool in predicting protein-ligand interactions. Its ability to accurately predict binding affinities, guide small molecule inhibitor design, and assist in lead optimization opens up endless possibilities for researchers in the field of biochemistry. By leveraging the capabilities of ChatGPT-4, scientists can streamline their drug discovery efforts and pave the way for the development of innovative treatments for various diseases.
Comments:
This article on revolutionizing analysis of protein-ligand interactions with ChatGPT is fascinating! As a biochemist, I'm excited to see how this technology can enhance our understanding of these complex interactions.
Thank you, Amy! I'm thrilled to hear your enthusiasm. ChatGPT has indeed shown great potential in biochemistry. It can assist in discovering new drug targets and designing more effective drugs.
I'm not a biochemist, but this article captured my interest. The ability of ChatGPT to analyze protein-ligand interactions could have significant implications in the development of new medicines.
I agree, Peter. It's exciting to think about the impact this technology could have on drug discovery. The ChatGPT model seems to excel at analyzing complex data and providing valuable insights.
Absolutely, Sara. ChatGPT's ability to analyze data and provide insights is quite impressive. The potential for accelerating drug discovery is immense.
I have some concerns about relying solely on AI for complex analysis in biochemistry. Human judgment and intuition are essential in this field. What are your thoughts?
Good point, Mark. While AI can be a powerful tool, it should always be complemented with human expertise. ChatGPT can augment our capabilities, but human analysis and interpretation remain crucial.
I agree, Mark and Sarah. AI is not meant to replace human analysis but rather to enhance it. The combination of AI and human expertise can lead to better and more efficient research outcomes.
I'm curious about the limitations of ChatGPT when applied to the analysis of protein-ligand interactions. Could it encounter challenges in interpreting complex biochemical data?
Great question, Julia. While ChatGPT has shown promise, it has its limitations. Interpreting highly complex biochemical data accurately can be challenging, and there's always a need for human validation.
I appreciate your response, Mitchell. Human validation remains crucial, especially with intricate biochemical data that may present challenges for AI systems like ChatGPT.
You're welcome, Julia. Absolutely, human validation ensures accuracy when dealing with complex biochemical data. ChatGPT's role is to assist and augment human analysis, not replace it.
I'm impressed with the potential of ChatGPT in biochemistry. It could greatly speed up the analysis process and help researchers focus on areas that require further investigation.
Thank you, Emily! You're right, ChatGPT can save time and help researchers sift through vast amounts of data more effectively. It allows for a more targeted approach in further investigation.
I wonder if ChatGPT has been tested on a diverse range of protein-ligand interactions. It's important to ensure its utility in various scenarios.
Valid point, Michael. ChatGPT has been trained on a diverse range of protein-ligand interactions, but testing it on a wider variety of scenarios will undoubtedly further validate its utility.
This article highlights the potential of AI in accelerating drug discovery. While it's exciting, we must also address ethical considerations surrounding AI implementation in biochemistry.
Absolutely, Elena. Ethical considerations are crucial when implementing AI in any field, including biochemistry. We must ensure responsible and transparent use of AI technology.
I'm intrigued by the possibilities of using ChatGPT to analyze protein-ligand interactions. It could open doors to new discoveries and more targeted drug design.
You're right, David. ChatGPT's ability to analyze and model protein-ligand interactions has tremendous potential for discovering new insights and advancing targeted drug design.
I'm concerned about the potential bias in AI models like ChatGPT. How can we ensure impartiality in the analysis, especially when it comes to diverse populations?
Valid concern, Rebecca. Addressing bias is of utmost importance. To ensure impartiality, training data should be diverse and inclusive, and continuous evaluation and mitigation of biases must be practiced.
ChatGPT's potential in analyzing protein-ligand interactions sounds promising. It could be a valuable tool in combination with experimental methods for understanding complex interactions.
Indeed, Karen. The combination of ChatGPT's analytical capabilities and experimental methods can provide a comprehensive approach to understanding protein-ligand interactions at a deeper level.
I'm curious about the computational resources required for running ChatGPT in biochemistry analysis. Are there any challenges in terms of computing power?
Great question, Gregory. Running ChatGPT in biochemistry analysis can be computationally intensive. However, with advancements in hardware and distributed computing, these challenges can be mitigated.
This article demonstrates the potential of AI to revolutionize biochemistry research. It's exciting to witness how technology is transforming the field.
Thank you, Melissa. AI, like ChatGPT, is indeed transforming the landscape of biochemistry. It empowers researchers with advanced capabilities and accelerates scientific discovery.
I'm glad to see the integration of AI in biochemistry research. The ability of ChatGPT to analyze protein-ligand interactions can lead to breakthroughs in drug development.
Absolutely, Adam. By leveraging ChatGPT's analysis potential, we can gain new insights into protein-ligand interactions, opening doors to more effective drug development strategies.
I'm excited about the possibilities ChatGPT presents in biochemistry. It could facilitate the discovery of novel protein-ligand interactions that were previously overlooked.
You're right, Olivia. ChatGPT can uncover previously overlooked interactions, thus expanding our understanding of protein-ligand binding and potentially leading to novel therapeutic avenues.
While ChatGPT seems promising, we have to be cautious about potential errors or false interpretations. Thorough validation and cross-checking are essential.
Absolutely, Daniel. Validation and cross-checking are crucial steps to ensure accuracy and avoid false interpretations. ChatGPT's results should always be critically evaluated.
As a budding biochemist, I find the integration of AI with biochemistry fascinating. It allows us to unravel complex interactions and delve deeper into structure-activity relationships.
That's wonderful to hear, Sophie. AI integration opens up exciting possibilities in biochemistry research. It empowers us to explore complex interactions and identify key aspects in structure-activity relationships.
I'm curious about the practical implementation of ChatGPT in a laboratory setting. How user-friendly is the interface for researchers who may not have strong coding skills?
Good question, Jacob. The usability of ChatGPT's interface for researchers is an important aspect. Efforts are being made to develop user-friendly interfaces that require minimal coding skills, ensuring broader accessibility.
This article provides a glimpse into the future of biochemistry research. The synergy between AI and human expertise can unlock innovative solutions to complex problems.
Well said, Sam. The combination of AI like ChatGPT and human expertise is a powerful force in advancing biochemistry research. Together, we can tackle complex problems and achieve groundbreaking solutions.
ChatGPT's potential seems immense in unraveling intricate protein-ligand interactions. The prospect of accelerating drug discovery through AI-driven analysis is thrilling!
Indeed, Vanessa! ChatGPT holds immense potential in unraveling the intricacies of protein-ligand interactions. By accelerating drug discovery, it takes us closer to more effective treatments and cures.
As a non-biochemist, I appreciate how this article simplifies the importance of protein-ligand interactions. It's incredible how technology can enhance our understanding.
I agree, Lucas! Simplifying complex concepts is crucial to make scientific research accessible. Technology like ChatGPT plays a vital role in enhancing our understanding across disciplines.
AI should be a complement, not a replacement. It's comforting to see the agreement among experts regarding the importance of human analysis in biochemistry.
Indeed, Peter. Human analysis will always be indispensable in biochemistry. Leveraging AI tools like ChatGPT can aid us, but human expertise and critical thinking are irreplaceable.
The combination of analytical and experimental approaches can provide a more comprehensive understanding of protein-ligand interactions. Exciting times ahead for biochemistry research!
Absolutely, Karen! When we combine analytical approaches like ChatGPT with experimental methods, we unlock new avenues for research and gain deeper insights in biochemistry.
Having accessible AI tools ensures that more researchers can leverage advanced analysis techniques in biochemistry. It democratizes the field and encourages innovation.
Well said, Sophie. Accessibility and democratization of AI tools are crucial in encouraging innovation and driving advancements in biochemistry. It allows a wider pool of researchers to contribute to the field.