Revolutionizing Autonomous Vehicles: Leveraging ChatGPT for Digital Image Processing in Vision Systems
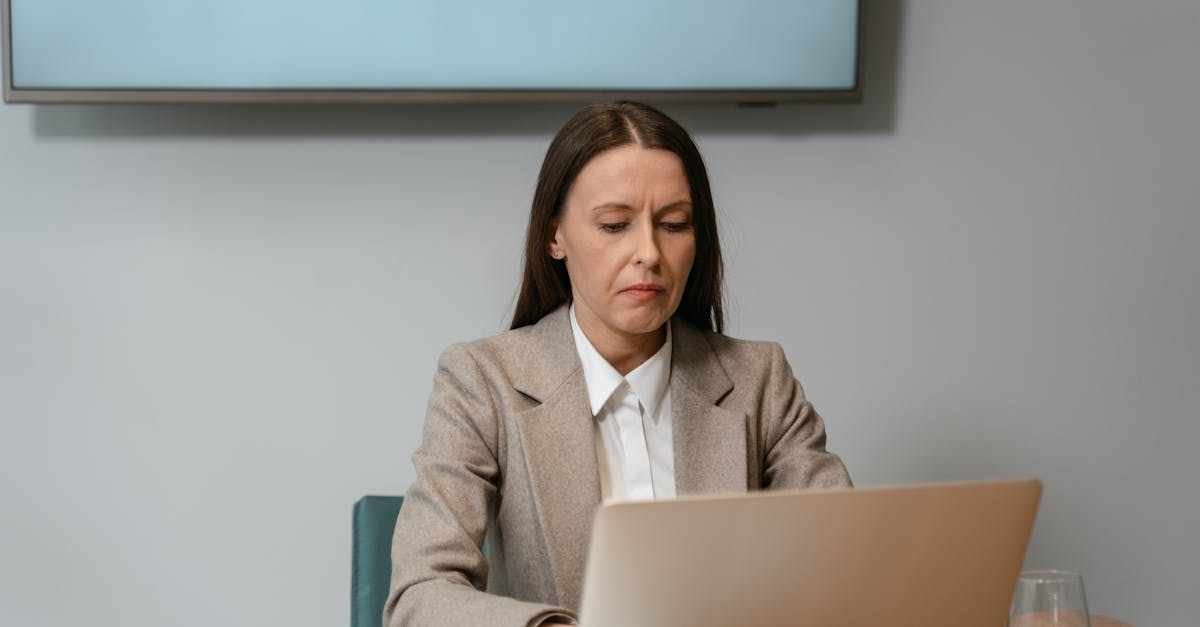
With the advancement of technology, autonomous vehicles have become a reality. These vehicles rely on various sensing mechanisms to perceive and interpret their surroundings. One of the key technologies employed in autonomous vehicles is digital image processing, which plays a vital role in achieving a high level of perception and navigation capabilities.
Digital image processing involves the manipulation and analysis of images using computer algorithms. In the context of autonomous vehicles, this technology is applied to vision systems that enable the vehicle to understand the environment it operates in. This article explores the different areas where digital image processing is utilized and how it contributes to the overall functioning of autonomous vehicles.
Obstacle Detection
One of the fundamental aspects of autonomous driving is the ability to detect and avoid obstacles in real-time. Digital image processing techniques, such as object recognition and segmentation, enable autonomous vehicles to identify and classify different objects in their vicinity. By analyzing the pixels in the captured images, algorithms can determine the presence of obstacles, such as pedestrians, vehicles, or road signs, and take appropriate actions to avoid collisions.
Navigation
An autonomous vehicle needs to navigate through complex road networks and scenarios. Digital image processing plays a crucial role in enabling accurate and reliable navigation. By analyzing the images captured by onboard cameras, the vehicle can extract information about road markings, lane boundaries, and traffic signs. These details help the vehicle make decisions, such as lane changes and turns, while adhering to traffic rules and ensuring safe driving.
Simultaneous Localization and Mapping (SLAM)
SLAM is an essential technology for autonomous vehicles, as it enables the vehicle to map its environment and determine its own location within it. Digital image processing techniques, combined with sensor data from other sources like LIDAR and RADAR, allow the vehicle to create a detailed map of its surroundings. By continuously updating this map through image analysis, the vehicle can estimate its position accurately, even in dynamic environments. SLAM is particularly beneficial in scenarios where GPS signals might be unreliable, such as urban canyons or tunnels.
ChatGPT-4: Vision Techniques Guide
As autonomous vehicles become more prevalent, the need for guidance on vision techniques is critical. OpenAI's ChatGPT-4 provides a valuable resource in this domain. ChatGPT-4 is an advanced language model that can engage in conversational interactions to provide explanations and guidance on various topics, including vision techniques for autonomous vehicles.
Users can interact with ChatGPT-4 through a chat interface, posing questions or discussing specific challenges related to vision in autonomous vehicles. Through its vast knowledge base, ChatGPT-4 can provide insights into digital image processing algorithms used for obstacle detection, navigation, SLAM, and other vision-related tasks. It can explain the underlying principles, suggest best practices, and offer solutions to problems faced during the implementation of vision systems in autonomous vehicles.
By leveraging ChatGPT-4's capabilities, developers, researchers, and enthusiasts can gain a better understanding of vision techniques and enhance their expertise in creating and improving autonomous vehicle systems. This valuable resource can pave the way for more advanced and efficient vision systems, leading to safer and more reliable autonomous vehicles on our roads.
In conclusion, digital image processing is a crucial technology for vision systems employed in autonomous vehicles. It enables obstacle detection, navigation, SLAM, and various other functionalities necessary for safe and efficient autonomous driving. OpenAI's ChatGPT-4 can serve as a helpful guide by providing explanations and guidance on vision techniques, fostering the development of innovative solutions and further advancements in the field of autonomous vehicles.
Comments:
This article is fascinating! The concept of leveraging ChatGPT for digital image processing in vision systems sounds like a game-changer.
I agree, Amy! It's exciting to see how ChatGPT can be applied in different fields. The potential for revolutionizing autonomous vehicles is huge!
I wonder how well ChatGPT performs in image processing compared to traditional methods. Anyone have insights on that?
Sarah, from my understanding, ChatGPT is primarily designed for natural language processing tasks. It might not be as efficient as specialized algorithms for image processing.
Lisa, you're correct. While ChatGPT is impressive in generating text, it may not have the same level of accuracy and efficiency in image processing compared to dedicated techniques.
Thank you all for your comments and insights! It's great to see your engagement. Regarding ChatGPT's performance in image processing, Lisa and Greg are correct. While it can show promising results, specialized algorithms are still more proficient in this area.
I'm curious about the potential drawbacks of relying on ChatGPT for image processing in autonomous vehicles. Can anyone shed light on that?
Eric, one possible drawback is the real-time performance. ChatGPT might introduce more latency compared to optimized image processing techniques, which is critical in autonomous vehicles.
I couldn't agree more, Mark and Brenda. Safety and reliability should be the top priorities when integrating AI models like ChatGPT into vision systems. Adequate evaluation and testing are crucial.
Brenda, Mark, and Amy, your focus on safety and reliability is spot-on. Autonomous vehicles offer incredible potential, but it's crucial to ensure responsible implementation, rigorous testing, and ongoing improvements.
Another concern could be the lack of interpretability. Neural networks like ChatGPT often act as black boxes, making it challenging to understand their decision-making process in image processing tasks.
I hadn't thought about the interpretability aspect, Nathan. That's a valid point. It's essential to understand how AI systems make decisions, especially in safety-critical applications like autonomous vehicles.
Eric, Brenda, and Nathan, you bring up valid concerns. Real-time performance and interpretability are critical factors to consider when integrating AI systems like ChatGPT into autonomous vehicles. Combining the strengths of specialized algorithms and AI models might be the way forward.
I'm curious how well ChatGPT can adapt to different environments and changing conditions in autonomous vehicles. Can it handle complex scenarios with accuracy?
Laura, while ChatGPT can generate impressive responses, its ability to handle complex real-world scenarios is still a challenge. It might struggle with understanding and adapting to rapidly changing conditions in autonomous driving.
Mark, you're right. Autonomous vehicles operate in dynamic environments, and ensuring AI systems can handle complex scenarios accurately is crucial. It would be interesting to explore how ChatGPT can overcome these challenges.
Laura, Mark, and Amy, your observations are on point. Adapting to diverse real-world conditions remains a significant challenge for ChatGPT. Advanced sensor fusion and hybrid approaches might be necessary to tackle these complexities.
I believe that integrating ChatGPT into autonomous vehicles could enhance human-vehicle interaction. ChatGPT's natural language capabilities could make communication and instructions more intuitive.
Daniel, I agree! If ChatGPT can effectively process and respond to natural language inputs, it could revolutionize the way humans interact with autonomous vehicles. It opens up exciting possibilities!
While improved human-vehicle interaction sounds interesting, we must consider safety implications. Dependence on ChatGPT for critical instructions might lead to miscommunications or misunderstandings.
Daniel, Sarah, and Lisa, you raise a crucial point. Safety should always be a priority in autonomous vehicles. Proper validation and fallback systems are necessary to ensure reliable human-vehicle interactions, even with ChatGPT integration.
I'm concerned about potential security risks when using AI models like ChatGPT in autonomous vehicles. AI systems can be vulnerable to adversarial attacks. How can we address this issue?
Jeff, you're right. Adversarial attacks pose a significant threat to AI systems. Robust defense mechanisms, thorough testing, and continuous monitoring are essential to mitigate security risks and protect autonomous vehicles.
Ensuring the security of AI systems from adversarial attacks is crucial, especially in safety-critical domains like autonomous vehicles. It requires a multi-layered approach with ongoing research and development.
Jeff, Nathan, and Amy, your concerns are valid. Security is a significant challenge in AI systems, and the autonomous vehicle industry must invest in robust defense mechanisms to safeguard against potential adversarial attacks.
Even with its limitations and challenges, it's remarkable to see the progress made with AI models like ChatGPT. The potential applications and advancements it brings are truly fascinating.
I agree, Eric! AI models like ChatGPT have opened up new possibilities across various industries. As the technology continues to evolve, we can expect even more exciting advancements in the future.
Eric, Laura, and Sarah, I share your enthusiasm. The advancements and potential of AI models like ChatGPT are truly remarkable. As technology progresses, we'll continue to witness exciting developments.
It's incredible how AI is transforming the world around us. We're witnessing the integration of intelligent systems like ChatGPT into domains we never thought possible before.
While the concept of leveraging ChatGPT for image processing in vision systems is fascinating, we should carefully evaluate the trade-offs and limitations. Safety and reliability should always come first.
Absolutely, Brenda! Considering the inherent challenges and limitations, responsible implementation and thorough testing are essential to ensure the safety and reliability of autonomous vehicles.
Absolutely, Mark and Amy! Collaborative discussions are essential in advancing any technology. It's been great participating in this conversation with all of you.
Mark, Amy, and Sarah, I'm thrilled to hear that you found value in this discussion. Collaborations and knowledge-sharing play a crucial role in driving innovation, and I appreciate your active participation.
The advancements in autonomous vehicles driven by AI models like ChatGPT are undoubtedly impressive. It's exciting to envision a future with safer and more efficient transportation systems.
Indeed, Daniel! Autonomous vehicles have the potential to transform transportation, making it more accessible, efficient, and environmentally friendly. AI models like ChatGPT contribute to this positive shift.
I agree with both Daniel and Lisa. As AI technology matures, we can look forward to a future where autonomous vehicles become the norm, revolutionizing transportation as we know it.
Daniel, Lisa, and Nathan, your optimism is inspiring. The potential impact of AI models in the autonomous vehicle industry is immense, and it's exciting to envision a future with safer, more efficient transportation systems.
I'm curious to know how soon we can expect to see AI models like ChatGPT being widely adopted in autonomous vehicles. Are there any significant challenges to overcome before that happens?
Eric, widespread adoption of AI models in autonomous vehicles might take some time. Overcoming challenges related to real-time performance, safety, interpretability, and security are critical steps towards achieving that goal.
Exactly, Sarah! We need further advancements in AI model capabilities, as well as addressing the challenges you mentioned, before ChatGPT and similar models become widely adopted in autonomous vehicles.
Eric, Sarah, and Laura, you bring up valid points. Achieving widespread adoption of AI models like ChatGPT in autonomous vehicles requires overcoming several challenges. Continuous research, development, and collaboration are key.
I've thoroughly enjoyed reading this article and the insightful comments. It's encouraging to see the discussions and considerations surrounding the application of AI models in autonomous vehicles.
I agree with you, Mark. Engaging in discussions like these helps us gain a better understanding of the opportunities and challenges that lie ahead in the field of autonomous vehicles.