Revolutionizing Biomarker Discovery: Harnessing ChatGPT for Cutting-Edge Technology
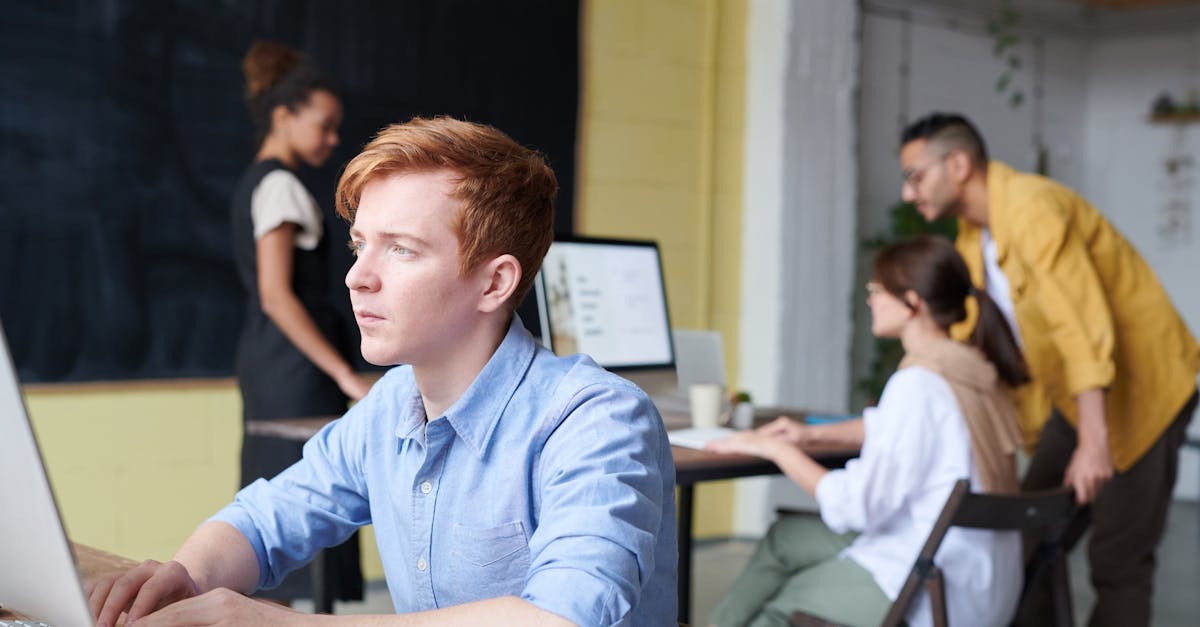
In the field of biomarker discovery, one of the most critical steps is analyzing and interpreting large datasets generated from genome sequencing. Genome sequencing allows scientists to obtain a comprehensive understanding of an individual's genetic makeup, providing insights into potential biomarkers that can be used for various purposes, such as disease diagnosis, drug development, and personalized medicine.
With the rapid advancement of technology, the generation of large genomic datasets has become more commonplace. However, the sheer volume and complexity of the data pose challenges for researchers, making it necessary to employ advanced tools and technologies to aid in the analysis process. One such revolutionary tool is ChatGPT-4.
Introducing ChatGPT-4
ChatGPT-4 is an AI language model developed by OpenAI. It represents the next generation of natural language processing technology, capable of understanding and generating human-like text. Building upon the success of its predecessors, ChatGPT-4 offers enhanced capabilities, making it an invaluable tool for scientists and researchers involved in biomarker discovery.
Analyzing and Interpreting Large Genomic Datasets
The power of ChatGPT-4 lies in its ability to effectively analyze, interpret, and discuss large datasets arising from genome sequencing. By leveraging its advanced natural language processing algorithms, ChatGPT-4 can sift through immense amounts of genomic data, identifying patterns, correlations, and potential biomarkers.
Using ChatGPT-4, researchers can interact with the model by posing questions, providing data, or seeking clarifications. The model can help scientists navigate through the complexities of genomic datasets, uncovering hidden relationships, and surfacing novel insights.
The Role of ChatGPT-4 in Biomarker Discovery
ChatGPT-4 presents researchers with several key advantages in the realm of biomarker discovery:
1. Efficient Data Analysis:
Through conversational interaction, researchers can swiftly analyze and interpret large genomic datasets without the need for complex data manipulation procedures. ChatGPT-4 can assist in pinpointing potential biomarkers and provide valuable insights into their significance.
2. Enhanced Collaboration:
ChatGPT-4 serves as a virtual collaborator for researchers, fostering discussions and brainstorming sessions. It can offer alternative viewpoints, propose hypotheses, and engage in insightful conversations to enhance the overall research process.
3. Rapid Iteration:
Researchers can quickly iterate and refine their hypotheses and research questions by engaging in conversation with ChatGPT-4. This allows for accelerated biomarker discovery and reduces the time required for analysis and interpretation of large genomic datasets.
4. Knowledge Expansion:
ChatGPT-4 has been trained on vast amounts of data across numerous scientific domains. It possesses extensive knowledge regarding genome sequencing, biomarker discovery, and related fields. Interacting with ChatGPT-4 can assist researchers in expanding their own understanding and domain expertise.
Conclusion
Biomarker discovery is a complex and data-intensive process, and genome sequencing plays a vital role in generating the necessary datasets. With ChatGPT-4, scientists and researchers benefit from an advanced AI tool that can effectively analyze, interpret, and discuss large genomic datasets. By leveraging the power of natural language processing, ChatGPT-4 enhances the biomarker discovery process, leading to novel insights and advancements in personalized medicine, disease diagnosis, and drug development.
Comments:
Thank you all for taking the time to read my article on Revolutionizing Biomarker Discovery using ChatGPT! I hope you found it informative and thought-provoking. I'm looking forward to hearing your thoughts and engaging in meaningful discussions.
Great article, Bridgett! The potential of using AI, like ChatGPT, in biomarker discovery is immense. It can accelerate the research process and open new doors for advancements in medical technology.
Michael, you mentioned the potential of AI in accelerating research. Can you elaborate on how ChatGPT specifically assists in biomarker discovery? I'm curious about the practical implementation.
Sara, ChatGPT can assist in several ways. For example, it can help researchers analyze complex data sets, generate hypotheses, and aid in decision-making. Its ability to understand and generate human-like text enables valuable interactions, making it a valuable tool in biomarker discovery.
I agree, Michael! The ability of AI to quickly analyze vast amounts of data can help identify patterns and correlations that humans may overlook. It's exciting to see how this technology can contribute to medical research.
Michael and Emma, thank you for your kind words! AI, indeed, has the potential to revolutionize biomarker discovery. It can complement human analysis and enhance our understanding of complex biological systems.
While the idea is interesting, there are significant ethical concerns that need to be addressed. How can we ensure the use of AI doesn't lead to biases or misinterpretations in biomarker discovery?
That's a valid concern, David. Bias in AI is a critical issue, especially in sensitive domains like healthcare. It's crucial to develop robust ethical guidelines, thoroughly test AI models, and ensure transparency in the decision-making process.
Bridgett, I appreciate your response. Would an independent oversight committee be necessary to monitor AI systems' ethical use in biomarker discovery?
David, an independent oversight committee can indeed provide an additional layer of accountability. Their role would involve assessing and monitoring the ethical use of AI in biomarker discovery, considering potential biases, and ensuring adherence to established guidelines.
I think this technology has great potential, but we should remember that AI is only as good as the data it's trained on. High-quality, diverse datasets are essential to minimize biases and ensure accurate results.
Absolutely, Sophia! Data quality and diversity play a significant role in the reliability of AI models. Collaborative efforts among researchers, medical professionals, and data scientists can help address these challenges.
Bridgett, collaboration is crucial for addressing data challenges. How can organizations encourage data sharing without compromising patient privacy?
Sophia, organizations can establish comprehensive data sharing frameworks that prioritize patient privacy and comply with regulations. Anonymizing data, implementing strict access controls, and obtaining informed consent are some strategies that can enable responsible data sharing while protecting patient privacy.
Bridgett, implementing data sharing frameworks can be challenging due to legal and organizational barriers. How can we encourage stakeholders to overcome these obstacles?
Sophia, transparency and open communication among stakeholders are crucial in overcoming legal and organizational barriers. Highlighting the benefits of responsible data sharing, addressing concerns, and establishing clear guidelines can foster trust and encourage stakeholders to work together towards effective data sharing frameworks.
Bridgett, what collaborative models or partnerships can help establish effective data sharing frameworks in the context of biomarker discovery?
Sophia, establishing collaborations between different stakeholders such as academic institutions, healthcare organizations, and technology companies can facilitate the development of effective data sharing frameworks. These partnerships can leverage each stakeholder's expertise to address legal and organizational barriers, ensure privacy, and promote responsible data sharing practices.
Bridgett, what strategies can help ensure that data sharing frameworks prioritize patient privacy appropriately?
Sophia, to prioritize patient privacy, frameworks can implement stringent data anonymization techniques to remove personally identifiable information. Applying access controls to limit data access to authorized personnel, obtaining informed consent, and adopting secure data storage practices are essential strategies to safeguard patient privacy while facilitating data sharing for biomarker discovery.
Bridgett, what are the challenges in implementing secure data storage practices when sharing datasets for biomarker discovery?
Sophia, implementing secure data storage practices involves addressing technical challenges such as data encryption, access controls, and vulnerability management. Additionally, ensuring compliance with data protection regulations, establishing secure data transfer mechanisms, and maintaining data integrity are vital challenges to consider. Collaborative efforts among data security experts and researchers can help address these challenges effectively.
Bridgett, addressing the challenges in secure data storage practices is vital for establishing trust among researchers when sharing datasets. Continuous vigilance and the adoption of best practices can help mitigate potential risks.
Absolutely, Sophia! Establishing trust through secure data storage practices is crucial for encouraging data sharing and collaboration. By staying vigilant, adopting best practices, and continuously improving security measures, we can create a framework that safeguards both data privacy and the potential benefits of AI in biomarker discovery.
Bridgett, continuous improvement and strict adherence to best practices in secure data storage are vital in building trust among researchers. Responsible data sharing can pave the way for unprecedented advancements in biomarker discovery.
Absolutely, Sophia! Continuous improvement, staying vigilant, and adhering to best practices in secure data storage are fundamental in gaining and maintaining researchers' trust. Responsible data sharing is a powerful catalyst for transformative advancements in biomarker discovery, and we must ensure privacy and security throughout the process.
The potential benefits of AI in biomarker discovery are immense, but we should also be cautious about overreliance. Human expertise and critical thinking should still be at the forefront of decision-making.
Justin, that's an excellent point! AI should be seen as a powerful tool that complements human intelligence, rather than replacing it. Empowering researchers with AI technology can enhance their capabilities in biomarker discovery.
Bridgett, in your opinion, what are the key skills researchers would need to develop to effectively leverage AI in biomarker discovery?
Justin, researchers would benefit from developing skills in data analysis, familiarity with AI tools and techniques, and understanding AI's limitations. Collaboration with AI experts and continuous learning are essential to fully leverage AI's potential in biomarker discovery.
Bridgett, continuous learning is essential, as you mentioned. Are there any specific resources or training programs you would recommend for researchers interested in integrating AI into biomarker discovery?
Justin, there are several online courses and resources available for researchers to develop their AI skills. Platforms like Coursera and edX offer courses on machine learning, data science, and AI. Additionally, attending conferences and workshops focused on AI in healthcare can provide valuable insights and networking opportunities.
Bridgett, thank you for the suggestions. Attending conferences sounds beneficial. Are there any specific conferences related to AI in healthcare that you recommend?
Justin, some notable conferences in the field include the International Conference on Machine Learning (ICML), Conference on Neural Information Processing Systems (NeurIPS), and the International Conference on Artificial Intelligence in Medicine (AIME). These conferences often feature AI in healthcare topics and provide a platform for knowledge sharing and networking.
Bridgett, thank you for sharing the conference names. I will definitely look into them. Could you suggest any notable online courses specifically focused on AI in healthcare?
Justin, certainly! Some notable online courses focused on AI in healthcare include 'AI in Healthcare Specialization' on Coursera offered by Stanford University, 'Machine Learning for Healthcare' on Coursera by the University of California, and 'Artificial Intelligence in Healthcare' on edX by Harvard University. These courses provide valuable insights into applying AI in the healthcare domain.
Bridgett, thank you for recommending the courses. They seem perfect for gaining a deeper understanding of AI in healthcare. Looking forward to exploring them!
You're welcome, Justin! I'm glad you find the course recommendations helpful. Enjoy exploring the fascinating intersection of AI and healthcare!
Bridgett, thank you once again for your guidance and insights. I'm excited to dive deeper into AI in healthcare. Your article has sparked my interest!
You're welcome, Justin! I'm thrilled that the article ignited your interest in AI in healthcare. I wish you all the best in your explorations, and don't hesitate to reach out if you have any further questions!
Bridgett, thank you once again for your willingness to help and share your expertise. I'm really grateful for your valuable insights!
You're very welcome, Justin! I'm always here to help and support your journey. Feel free to reach out anytime you have questions or need further guidance. Best of luck in your explorations!
What are the main challenges you foresee in implementing AI-based approaches like ChatGPT in biomarker discovery? Are there any limitations to consider?
Amanda, great question! One challenge is the interpretability of AI models. Understanding how and why AI algorithms reach certain conclusions is crucial in the context of biomarker discovery. Additionally, ensuring scalability and handling large datasets are areas we need to address.
I'm concerned about potential privacy issues. When using AI models in biomarker discovery, sensitive patient data needs to be handled securely and in compliance with privacy regulations.
Robert, you raise a valid concern. Privacy and data security are of utmost importance. Adhering to robust privacy protocols and obtaining proper consent from patients are necessary steps to protect sensitive information.
Bridgett, I appreciate your response. Compliance with privacy protocols is vital, but how can we ensure AI models themselves don't inadvertently reveal private information?
Robert, that's an important concern. Properly examining and auditing AI models can help identify potential vulnerabilities and privacy risks. Employing techniques like differential privacy and rigorous testing can mitigate the risk of inadvertently revealing private information.
Bridgett, thank you for your response. Do you think implementing AI safeguards is solely the responsibility of researchers, or should regulatory bodies play a role as well?
Robert, ensuring AI safeguards is a shared responsibility between researchers, regulatory bodies, and other stakeholders. Researchers should implement best practices, but regulatory bodies play a crucial role in setting guidelines and ensuring adherence to ethical and safety standards, fostering trust in the wider community.
Bridgett, you mentioned regulatory bodies' role. What steps can they take to strike a balance between AI regulation and facilitating innovation?
Robert, regulatory bodies can strike a balance by engaging in ongoing conversations with researchers, industry experts, and policymakers. By understanding the unique challenges and opportunities of AI in biomarker discovery, they can develop adaptive frameworks that encourage innovation while ensuring safety, ethics, and protection of patient rights.
Bridgett, striking a balance between AI regulation and innovation seems challenging. Are there examples of countries that have successfully implemented AI regulations in healthcare?
Robert, several countries have taken steps in implementing AI regulations in healthcare. For instance, the European Union has developed guidelines for trustworthy AI and is working on an AI Act. Countries like Canada, Singapore, and the United States have also made significant progress in establishing regulatory frameworks to govern AI implementation in healthcare.
Bridgett, thank you for sharing examples. It's reassuring to know that various countries are actively working on AI regulations in healthcare to ensure responsible implementation.
Absolutely, Robert! Regulatory efforts are crucial to foster trust, ensure ethical use of AI, and protect patient rights. By working collaboratively on establishing these regulations, countries can set a strong foundation for responsible AI implementation in healthcare.
Bridgett, I completely agree. Collaboration in establishing AI regulations will lead to responsible AI implementation, enabling the healthcare sector to harness the full potential of this transformative technology.
Indeed, Robert! Collaboration is key to ensuring responsible AI implementation and maximizing the benefits it can bring to the healthcare sector. By working together, we can cultivate an environment where AI facilitates breakthroughs in biomarker discovery while maintaining ethical standards and patient well-being.
Incorporating AI in biomarker discovery can lead to faster, more accurate diagnoses. This can significantly improve patient outcomes and provide better treatment options.
Absolutely, Olivia! The potential impact on patient outcomes is one of the most promising aspects of AI in healthcare. By harnessing the power of technology, we can advance medical research and improve treatment strategies.
Bridgett, do you think AI technology like ChatGPT can be integrated with existing medical research frameworks smoothly?
Olivia, integrating AI technology with existing frameworks can present some challenges, but it's certainly achievable. Collaboration between AI developers and medical researchers can help identify the best ways to integrate AI tools like ChatGPT into existing research workflows while ensuring compatibility with established frameworks.
Bridgett, how can we address the potential resistance from researchers who might be skeptical about integrating AI technology like ChatGPT into their workflow?
Olivia, addressing skepticism requires demonstrating the value and potential impact of AI in biomarker discovery through successful use cases and sharing experiences. Researchers' involvement in the development and validation of AI models can help build trust and alleviate concerns by highlighting the benefits in their specific field.
Bridgett, how can interdisciplinary collaboration between AI developers and medical researchers facilitate the integration of AI into biomarker discovery?
Olivia, interdisciplinary collaboration enables AI developers and medical researchers to work together, leverage their respective expertise, and bridge any gaps that may exist. This collaboration ensures AI tools like ChatGPT are developed considering the specific needs of biomarker discovery, fostering successful integration and enabling optimal utilization of AI technology in healthcare research.
Bridgett, what are the potential challenges researchers might face when integrating AI tools like ChatGPT into their existing biomarker discovery workflows?
Olivia, integrating AI tools into existing workflows can present challenges, such as the need for researchers to acquire AI knowledge and skills. Adapting established processes to accommodate the AI integration, data compatibility issues, and ensuring trust in AI technology are some challenges researchers might face. However, with proper training, support, and collaborative partnerships, these challenges can be overcome.
Bridgett, the challenges you mentioned seem manageable with the right support and collaboration. It's essential to focus on empowering researchers and facilitating their transition to AI-enabled biomarker discovery.
Absolutely, Olivia! Empowering researchers with the necessary resources, training, and collaborations is key to smooth integration of AI into existing biomarker discovery workflows. By addressing challenges and providing adequate support, we can pave the way for transformative advancements in healthcare research.
Bridgett, empowering researchers and facilitating their transition to AI-enabled biomarker discovery is an important objective. Through collaboration and continuous support, we can create a positive impact and drive innovation in healthcare research.
Absolutely, Olivia! Empowering researchers to embrace AI technology and providing the necessary resources and support is crucial. By doing so, we can foster a culture of innovation and collaboration, driving advancements in healthcare research and ultimately benefiting patients.
Is ChatGPT specifically designed for biomarker discovery, or can it be applied to other areas of medical research as well?
Emily, ChatGPT is a versatile language model that can be applied to a wide range of tasks. While it's not specifically designed for biomarker discovery, it can certainly contribute to the field by assisting researchers in analyzing data, generating hypotheses, and facilitating scientific discussions.
Bridgett, how can researchers ensure the reliability of AI models like ChatGPT? Are there specific validation techniques or best practices?
Emily, validating AI models requires rigorous testing and evaluation. Techniques such as cross-validation, benchmarking against existing datasets, and comparing results with domain experts' assessments can help validate the reliability of AI models like ChatGPT. It's an ongoing process that involves continuous monitoring and improvement.
Bridgett, what measures can researchers take to ensure AI models like ChatGPT don't perpetuate biases present in existing datasets?
Emily, addressing biases requires careful curation of training datasets, considering diverse perspectives, and ensuring representative samples. Periodic evaluations of AI models, both during development and when deployed, can help identify and rectify any biases that might arise. Transparency in AI decision-making processes is vital to address and mitigate biases.
Bridgett, how can organizations encourage transparency in AI decision-making processes? Are there any frameworks or guidelines available?
Emily, organizations can encourage transparency by adopting standards and guidelines like the AI Transparency and Accountability Framework proposed by the IEEE. These frameworks emphasize the importance of explaining AI's decision logic, data sources, and potential limitations. Furthermore, promoting research on interpretability and explainability of AI models can drive progress in this domain.
Bridgett, what role can professional organizations play in promoting transparency and accountability in AI decision-making processes?
Emily, professional organizations can play a crucial role by establishing guidelines, sharing best practices, and offering training programs that emphasize the importance of transparency and accountability in AI decision-making. They can organize conferences, workshops, and knowledge-sharing platforms that facilitate discussions and collaborations among AI developers, researchers, and policymakers, fostering a culture of responsible AI use.
Bridgett, professional organizations can indeed serve as catalysts for promoting responsible AI practices. Their initiatives can foster a sense of community and shared responsibility among AI stakeholders, furthering transparency and accountability in AI decision-making.
Exactly, Emily! Professional organizations play a crucial role in creating a supportive ecosystem, where knowledge sharing, collaboration, and responsible AI practices are encouraged. By bringing stakeholders together, these organizations can drive positive change and enable AI to address complex challenges in biomarker discovery and beyond.
Bridgett, creating a supportive ecosystem through professional organizations ensures that AI in biomarker discovery is developed responsibly. Collaboration and collective efforts are necessary to address the challenges and opportunities posed by AI technology.
Exactly, Emily! Collaboration and fostering a supportive ecosystem through professional organizations enable us to collectively overcome challenges and harness AI's potential in biomarker discovery. By working together, we can unlock new insights, drive innovation, and make a positive impact on healthcare research and patient care.
I'm curious about the computational requirements of using AI models like ChatGPT. Do you think it's accessible to most researchers in terms of computing power?
George, that's an important consideration. The computational requirements can be a challenge for some researchers. However, with advancements in cloud-based computing services and the availability of pre-trained models, the accessibility of AI technology is increasing, making it more feasible for researchers.
Bridgett, with the increasing popularity of AI in various fields, do you foresee more researchers adopting AI technology like ChatGPT in biomarker discovery?
George, as AI technology continues to advance and demonstrate its potential, I believe more researchers will be inclined to adopt it in biomarker discovery. The key is ensuring accessibility, providing user-friendly interfaces, and promoting collaborations that bridge the gap between AI developers and domain experts.
Bridgett, you mentioned the importance of user-friendly interfaces. How can AI developers make their tools more accessible to researchers who may not have extensive AI expertise?
George, user-friendly interfaces can simplify the adoption of AI tools by researchers. Providing clear documentation, intuitive interfaces, and incorporating feedback from end-users can make AI models more accessible. Collaborations between AI developers and domain experts during the development process can ensure these tools cater to the needs of researchers without extensive AI expertise.
Bridgett, what steps can be taken to ensure AI model developers and researchers effectively communicate and understand each other's needs during the development process?
George, effective communication can be fostered by involving researchers as stakeholders in the development process itself. Regular meetings, clear documentation, and understanding researchers' specific requirements can bridge the communication gap. Actively seeking feedback, addressing concerns, and iteratively refining AI models can lead to productive collaborations and successful deployment in biomarker discovery.
Bridgett, you mentioned involving researchers as stakeholders. How can AI developers identify and understand researchers' specific needs and challenges?
George, understanding researchers' needs requires active communication and collaboration from the early stages. AI developers can engage with researchers through surveys, interviews, and workshops to gain insights into their workflows, challenges, and pain points. By involving researchers as partners throughout the development process, AI developers can tailor their solutions to meet specific needs, fostering a user-centric approach.
Bridgett, involving researchers as stakeholders can help ensure AI developers truly understand the end-users' needs. Collaboration and communication are vital for successful AI integration in biomarker discovery workflows.
Absolutely, George! Collaboration and effective communication between AI developers and researchers are essential ingredients for successful integration. Understanding the unique needs, challenges, and requirements of researchers enables AI developers to create solutions that have a genuine impact and seamlessly fit into biomarker discovery workflows.
Bridgett, communication and collaboration between AI developers and researchers can ensure that AI integration in biomarker discovery is a seamless and impactful process. Together, we can leverage AI's capabilities to advance medical research.
Absolutely, George! Effective communication and collaboration enable us to bridge the gap between AI developers and researchers, creating seamless integration and maximizing AI's potential in biomarker discovery. Together, we can accelerate medical research and unlock new frontiers in healthcare.
Thank you all for taking the time to read my article on revolutionizing biomarker discovery using ChatGPT! I'm excited to hear your thoughts and answer any questions you may have.
This is a fascinating application of language models like ChatGPT. It's amazing to see how AI is transforming various industries.
Indeed, Josephine! AI has the potential to revolutionize the way we approach biomarker discovery, which in turn can have a significant impact on healthcare and medical research.
I'm curious to know how reliable the biomarker predictions made by ChatGPT are. Are there any studies or results validating its effectiveness?
That's a great question, Michael. Recent studies have shown promising results in using ChatGPT for identifying potential biomarkers, but further research and validation are ongoing to ensure its reliability and accuracy.
I'm impressed by the potential of ChatGPT in streamlining the biomarker discovery process. It could save so much time and effort compared to traditional methods.
Absolutely, Sarah! The ability of ChatGPT to process and analyze vast amounts of biomedical literature can greatly accelerate the identification of potential biomarkers, leading to more efficient research and development.
I wonder if ChatGPT can also be used for other areas of medical research besides biomarkers. The possibilities seem endless!
You're absolutely right, David! ChatGPT has the potential to be applied to various areas of medical research, such as drug discovery, clinical decision support, and even personalized medicine.
While the possibilities are exciting, we should also consider the ethical implications of relying heavily on AI systems like ChatGPT. Human oversight and accountability are crucial.
That's an important point, Victoria. While AI can be a powerful tool, it should always be used in conjunction with human expertise and judgment. Striking the right balance between automation and human oversight is key.
I'm curious to know how accessible ChatGPT is for researchers who might not have extensive AI or programming backgrounds.
Great question, Jason! OpenAI is actively working on improving the accessibility of ChatGPT to empower researchers with diverse backgrounds to leverage its capabilities without needing advanced AI or programming knowledge.
The potential applications of ChatGPT in biomarker discovery are immense, but we should also be cautious about potential biases in the data that could propagate through the AI system.
You raise a valid concern, Sophia. It's crucial to ensure the training data used for ChatGPT is representative and diverse, to avoid biased predictions. Mitigating biases in AI systems is an ongoing challenge that requires continuous effort.
Can ChatGPT be used to analyze real-time patient data and provide instant biomarker predictions?
That's an interesting idea, Jennifer. While ChatGPT's capabilities are impressive, real-time analysis of patient data requires stringent security and privacy considerations. It's a possibility, but it would require careful implementation and safeguards.
The potential applications of ChatGPT for biomarker discovery seem promising, but we should also be mindful of the limitations of language models when it comes to truly understanding the underlying biological mechanisms.
Very true, Maxwell. Language models like ChatGPT excel at processing and analyzing text but may not have a deep understanding of the underlying biological processes. It's important to leverage their capabilities while considering domain expertise for comprehensive biomarker discovery.
As a healthcare professional, I'm optimistic about the potential of AI in improving patient care and advancing medical research. Exciting times!
Absolutely, Emily! AI has the power to transform the healthcare industry and benefit both patients and healthcare providers. It's an exciting journey ahead.
I wonder if ChatGPT can be used in conjunction with other biomarker discovery techniques to complement and enhance the results.
That's an excellent point, Oliver. The integration of ChatGPT with existing biomarker discovery techniques can potentially offer a more comprehensive and reliable approach, making use of the strengths of both AI and traditional methods.
What are some of the challenges you foresee in scaling up ChatGPT for widespread biomarker discovery adoption?
Great question, Linda. Scaling up ChatGPT for widespread adoption comes with challenges like model accuracy, computational resources, addressing biases, and handling ethical concerns. Addressing these challenges will be crucial for its successful integration into the biomarker discovery process.
I'm impressed by the potential speed at which ChatGPT can analyze vast amounts of literature. It can definitely expedite the pace of biomarker discovery.
Indeed, Robert! The speed and efficiency of ChatGPT in processing vast amounts of biomedical literature can help researchers explore a broader space of potential biomarkers, accelerating the pace of discovery and innovation.
Could ChatGPT be incorporated into existing research platforms or software tools to enhance their capabilities?
Absolutely, Hannah! Integration of ChatGPT with existing research platforms or software tools can augment their capabilities and provide researchers with an AI-powered tool to assist in biomarker discovery, making the process more efficient and effective.
Are there any current collaborations between AI researchers and biomarker discovery experts to further refine the application of ChatGPT?
Definitely, Timothy! AI researchers are actively collaborating with biomarker discovery experts and domain specialists to refine the application of ChatGPT. These collaborations are vital to harness the full potential of AI in biomarker research and ensure its alignment with real-world needs.
I'm curious about the training process of ChatGPT. How was it taught to understand biomedical literature?
Great question, Julia! ChatGPT's training involves pre-training on a diverse range of internet text, which includes biomedical literature. This exposure helps it to acquire a broad understanding of various domains, including the language and context specific to the biomedical field.
The potential benefits of ChatGPT for biomarker discovery are immense, but how accessible is it to organizations and researchers, considering the computational resources required?
Valid point, Daniel. The computational resources necessary to leverage ChatGPT's capabilities can be a challenge. However, OpenAI is working on exploring options to make it more accessible and cost-effective, enabling more organizations and researchers to benefit from its potential.
I'm intrigued by the potential impact of ChatGPT on personalized medicine. It could potentially aid in identifying customized biomarkers for individual patients.
Absolutely, Ava! ChatGPT's ability to process and analyze vast amounts of biomedical literature can contribute to personalized medicine by assisting in the identification and prioritization of relevant biomarkers tailored to individual patients, ultimately leading to more precise and effective treatments.
With the rapid advancement of AI and language models, do you envision a future where biomarker discovery is predominantly driven by AI systems like ChatGPT?
That's an interesting question, Isabella. While AI systems like ChatGPT have the potential to transform biomarker discovery, I believe the future lies in striking a balance between AI-driven processes and the valuable expertise of researchers and domain specialists. Collaboration between humans and AI will be key for comprehensive and reliable biomarker discovery.
Thank you all for your insightful comments and questions! It's been a pleasure discussing the potential of ChatGPT in revolutionizing biomarker discovery. Let's continue advancing this exciting field together!