Revolutionizing Biomarker Discovery: Harnessing ChatGPT for Genomic Data Processing
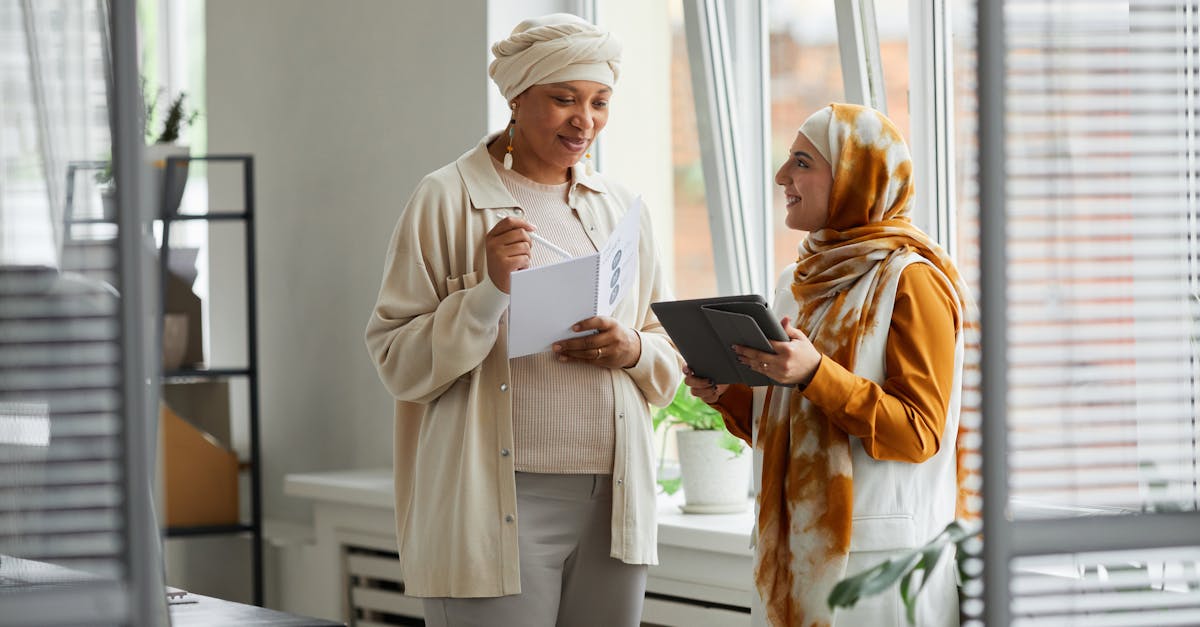
The field of biomarker discovery has gained significant momentum in recent years, with advancements in genomic data processing playing a pivotal role in uncovering valuable insights. As the volume and complexity of genomic data continue to increase, it becomes crucial to utilize sophisticated technologies for efficient data processing and interpretation. One such technology that has shown great promise in this domain is ChatGPT-4, an advanced natural language processing model powered by AI.
Genomic Data Processing and Biomarker Discovery
Genomic data encompasses vast amounts of information present within an individual's DNA sequence. This data holds the key to understanding the underlying genetic basis of various diseases and conditions, as well as identifying potential biomarkers for diagnosis, prognosis, and treatment response. However, large-scale genomic data analysis presents several challenges, such as the need for accurate data preprocessing, feature selection, and complex statistical modeling.
Biomarker discovery involves identifying specific biological markers that can be used to indicate the presence or progression of a disease. These markers are typically derived from genomic data and can provide crucial insights into disease mechanisms, patient stratification, and response to therapies. Efficient processing and interpretation of genomic data are essential for the successful identification and validation of biomarkers.
The Role of ChatGPT-4 in Genomic Data Processing for Biomarker Discovery
ChatGPT-4 combines the power of natural language processing and artificial intelligence to assist researchers and scientists in processing and interpreting genomic data. With its advanced language understanding capabilities, ChatGPT-4 can guide users through various stages of data analysis, from data preprocessing to statistical modeling, and offer valuable insights along the way.
Utilizing ChatGPT-4, researchers can interact with the model using natural language queries, gaining assistance in performing complex data transformations, feature selection, and statistical analyses. This interactive approach simplifies the data processing workflow, allowing researchers to focus on the biological interpretation of their findings.
The vast knowledge base and cognitive abilities of ChatGPT-4 enable it to provide context-specific recommendations and suggestions tailored to the analysis at hand. For example, when investigating potential biomarkers for a specific disease, ChatGPT-4 can offer guidance on appropriate statistical models, identify relevant genetic pathways or functions, and highlight potential confounding factors to consider.
Benefits and Future Implications
The integration of ChatGPT-4 in genomic data processing for biomarker discovery offers several benefits. Firstly, it reduces the time and effort required to process and analyze large-scale genomic data, improving efficiency and scalability of research efforts. Furthermore, it allows researchers with varying levels of computational expertise to interact with complex data analysis techniques, democratizing access to advanced genomic analysis tools.
As AI models continue to evolve and improve, the future implications of ChatGPT-4 and similar technologies in genomic data processing look promising. The assistance provided by AI models can accelerate biomarker discovery efforts, leading to improved disease diagnosis, targeted therapies, and personalized medicine. Additionally, the ability of AI models to mine vast amounts of genomic data can potentially unveil previously unknown biomarkers and genetic associations, advancing our understanding of complex diseases.
Conclusion
In the evolving field of biomarker discovery, efficient genomic data processing is crucial. With the advent of technologies like ChatGPT-4, researchers now have a powerful tool to guide them through the complexities of data analysis and interpretation, ultimately leading to more accurate and impactful biomarker discoveries. Leveraging the capabilities of AI models in genomic data processing opens up new avenues for research and the development of personalized approaches to diagnose and treat diseases.
Comments:
Thank you all for taking the time to read my article on 'Revolutionizing Biomarker Discovery: Harnessing ChatGPT for Genomic Data Processing'. I hope you found it informative and thought-provoking. I look forward to hearing your thoughts and having a fruitful discussion.
Great article, Bridgett! The potential of ChatGPT in genomics is truly exciting. I can see how it can greatly speed up the process of biomarker discovery. Do you think there are any limitations or challenges to be aware of when using ChatGPT in this context?
Thank you, Charles! ChatGPT indeed offers promising possibilities. While it can accelerate biomarker discovery, it's important to consider the limitations. One major challenge is the interpretability of the model's outputs. Deep learning models like ChatGPT can sometimes be seen as 'black boxes' without clear explanations for their decisions, so further research in this area is needed.
Hi Bridgett! Your article is fascinating. I wonder how ChatGPT compares to existing tools or techniques used in biomarker discovery? Are there any specific advantages it offers over traditional methods?
Hello Sophia! Thank you for your kind words. ChatGPT brings unique advantages to the table. Unlike traditional methods that rely on predefined algorithms, ChatGPT can understand natural language queries and adapt to varying input formats. This flexibility is particularly valuable when dealing with complex genomic data that traditional approaches may struggle with.
Thanks for sharing your insights, Bridgett. I'm curious about the ethical implications of using ChatGPT for genomic data processing. What steps should be taken to ensure privacy and security when dealing with sensitive genetic information?
Good question, David. When working with sensitive genetic data, privacy and security are paramount. Proper anonymization techniques and encryption protocols must be employed to protect the information. Additionally, it's crucial to adhere to established ethical guidelines and obtain appropriate consent from individuals sharing their genomic data.
Impressive article, Bridgett. I'm curious to know if ChatGPT is accessible to researchers and organizations with limited computational resources. Are there any strategies to make this technology more widely available?
Thank you, Olivia. Accessibility is important, and efforts are being made to address computational resource limitations. Some approaches include model distillation techniques, which aim to make the models more lightweight and efficient. Additionally, cloud-based services can provide access to ChatGPT's capabilities without requiring extensive local computational resources.
Bridgett, your article is quite intriguing. How do you envision the integration of ChatGPT with other state-of-the-art technologies in the field, such as machine learning algorithms or other AI models?
Thank you, Josephine. The integration of ChatGPT with other technologies holds great potential. Machine learning algorithms can be used for pre-processing and data augmentation, while other AI models can provide complementary insights. The collaboration between various tools can lead to more robust and accurate biomarker discovery.
Hi Bridgett, thanks for writing this informative article. I'm wondering if there are any real-world applications of ChatGPT in genomics that have shown promising results?
Hello Michael! There are indeed several real-world applications of ChatGPT in genomics. For example, it has been successfully used in identifying potential genetic targets for drug development, detecting disease biomarkers, and even assisting in personalized medicine. These applications showcase the versatility and potential of ChatGPT in genomic data processing.
Great article, Bridgett. I can see the tremendous impact of ChatGPT in revolutionizing biomarker discovery. Do you think it will eventually replace existing methods, or will it complement them?
Thank you, Emily. Rather than replacing existing methods, ChatGPT is more likely to complement them. It can offer a valuable addition to the toolkit of researchers and help expedite the process of biomarker discovery. Integrating multiple approaches can lead to more comprehensive and accurate results.
Bridgett, I appreciate your article. ChatGPT seems like a powerful tool, but are there any potential biases in the model that researchers should be cautious of when using it for genomic data processing?
Thank you, Luke. Bias is an important consideration. As ChatGPT learns from existing data, it can inadvertently perpetuate biased patterns present in the training data. Researchers must proactively address this issue by ensuring diverse and representative training datasets and by continually monitoring and mitigating bias during the model's development and deployment.
Fantastic article, Bridgett. I'm curious, how do you see ChatGPT impacting the field of precision medicine? Can it aid in identifying personalized treatment approaches?
Thank you, Sarah. ChatGPT can indeed have a significant impact on precision medicine. By analyzing vast amounts of genomic data, it can aid in identifying specific biomarkers and potential treatment approaches for individual patients. This personalized approach has the potential to revolutionize the field and enhance patient outcomes.
Bridgett, thanks for shedding light on the potential of ChatGPT in biomarker discovery. Are there any ongoing research efforts focused on addressing the limitations or expanding the capabilities of this technology?
You're welcome, Jacob. Ongoing research indeed aims to address the limitations and expand the capabilities of ChatGPT. Researchers are working on improving model interpretability, incorporating domain-specific knowledge, and exploring avenues for better integration with other tools and techniques. The field is advancing rapidly, and we can expect exciting developments in the near future.
Excellent article, Bridgett. Considering the vastness and complexity of genomic data, do you see any challenges in scaling up ChatGPT for large-scale genomic research?
Thank you, Ava. Scaling up ChatGPT for large-scale genomic research does pose challenges. The models can be computationally demanding, requiring substantial resources and infrastructure. Efficient parallelization and distributed computing techniques can help address these challenges and enable the use of ChatGPT in large-scale genomics projects.
Thanks for the insightful article, Bridgett. In your opinion, what are some possible future applications of ChatGPT in genomics beyond biomarker discovery?
You're welcome, Ethan. ChatGPT holds promise for various future applications in genomics. Some potential areas include genomics-driven drug design, genomic data curation and annotation, and even aiding in the development of personalized nutrition plans based on individuals' genetic makeup. The possibilities are vast, and the technology continues to evolve.
Bridgett, your article is thought-provoking. Are there any specific genomic databases or resources that are particularly compatible with ChatGPT?
Thank you, Lillian. ChatGPT can work with multiple genomic databases and resources. Examples include public repositories like GenBank, ENSEMBL, and ClinVar, as well as disease-specific databases and curated datasets. Its flexibility allows researchers to interact with and extract insights from various data sources.
Bridgett, your article highlights the potential of ChatGPT for genomic data processing. I'm curious, how does the fine-tuning process work when applying ChatGPT to genomics?
Hello Nathan. Fine-tuning ChatGPT for genomics involves training the model on a specific dataset, often involving paired questions and answers related to genomics. The model learns from these examples and is fine-tuned using techniques like transfer learning. This process helps adapt ChatGPT to the specific task of genomic data processing.
Bridgett, your article is enlightening. How do you see the deployment of ChatGPT in clinical settings, considering potential challenges with model interpretability and regulatory approvals?
Thank you, Gabriella. The deployment of ChatGPT in clinical settings does indeed have challenges. Model interpretability is crucial for regulatory approvals and building trust. Researchers are actively exploring methods to provide explainable results and ensure transparency in the decision-making process of the model. Addressing these challenges will be vital for regulatory compliance and the successful integration of ChatGPT in clinical workflows.
Interesting article, Bridgett. I'd like to know if there are any known security vulnerabilities associated with using ChatGPT in genomic data processing?
Thanks, Aaron. Security vulnerabilities must be carefully considered. Like any software, there may be potential vulnerabilities associated with ChatGPT, especially when deployed in distributed environments or when dealing with sensitive data. Implementing robust security measures, regular updates, and adherence to security best practices can help mitigate such risks.
Great article, Bridgett. Considering the constantly evolving nature of genomic data and research, how adaptable is ChatGPT to keep up with the advancements in the field?
Thank you, Victoria. ChatGPT's adaptability is one of its strengths. It can be fine-tuned on domain-specific tasks and datasets, allowing it to evolve with advancements in genomics. Regular updates and continuous training on the latest research findings enable ChatGPT to stay up-to-date with the ever-changing landscape of genomic data.
Bridgett, I really enjoyed your article. Has ChatGPT been integrated into any existing bioinformatics tools, or is it primarily used as an independent tool?
Hello Hannah. ChatGPT has primarily been used as an independent tool in genomics research so far. However, there is potential for integration with existing bioinformatics tools to enhance data analysis workflows and improve accessibility. Collaborative efforts between researchers and developers can pave the way for such integrations.
Bridgett, your article provides valuable insights. Considering the large amounts of data involved, have there been any studies on the efficiency of ChatGPT in comparison to other approaches for genomics data processing?
Thank you, Julia. Several studies have demonstrated the efficiency of ChatGPT in genomics data processing. It has been shown to significantly reduce the time required for biomarker discovery and analysis compared to traditional approaches. Its ability to handle large volumes of data and adapt to complex queries contributes to its efficiency, making it a promising tool for genomics research.
Bridgett, thank you for sharing your expertise through this article. Can ChatGPT be trained on proprietary genomic datasets, or does it primarily rely on publicly available data?
You're welcome, Sophie. ChatGPT can be trained on both publicly available and proprietary genomic datasets. While publicly available data offers broad applicability, the use of proprietary datasets can help researchers tailor the model specifically to their domain or focus of interest. The flexibility in training data choice offers opportunities for both general and niche applications.
Impressive article, Bridgett. Could you provide an example of how ChatGPT can handle complex natural language queries related to genomics data processing?
Thank you, Samuel. Certainly! An example query could be: 'Identify potential new drug targets for cancer treatment based on genomics data.' ChatGPT can process such queries, understand the context and requirements, and provide insights on potential genetic targets that could be explored for therapeutic purposes. Its ability to interpret and respond to complex queries makes it a valuable tool in genomics.
Bridgett, your article is enlightening. How does ChatGPT handle uncertainties or missing data in genomic datasets during the processing stage?
Thank you, Liam. ChatGPT can encounter uncertainties and missing data during processing. To handle such situations, researchers can adopt imputation techniques to estimate missing values or leverage probabilistic models to evaluate uncertainties. Handling these challenges effectively is essential to ensure accurate and reliable results from the genomic data analysis process.
Bridgett, your article on ChatGPT and biomarker discovery is intriguing. Besides its benefits in speed and scalability, do you see any other advantages of using ChatGPT over existing methods?
Hello Daniel. Absolutely, ChatGPT offers additional advantages. One notable advantage is its ability to generate explanations or rationales for its predictions, aiding in result interpretation. In contrast, some traditional methods might provide insights without clarifying the underlying reasoning. The explainability aspect sets ChatGPT apart and enhances its usefulness in genomics.
Great article, Bridgett. Considering the potential impact of ChatGPT in genomics, how do you foresee its integration into educational and research institutions?
Thank you, Hailey. ChatGPT has the potential to be integrated into educational and research institutions in multiple ways. It can assist students and researchers in exploring complex questions, providing guidance, and offering valuable insights. Integrating ChatGPT into the educational curriculum can help foster a deeper understanding of genomics and facilitate innovative research in the field.
Bridgett, your article raises an important point. What are some potential applications of ChatGPT in the field of cancer research and treatment?
Hello Isabella. ChatGPT can play a significant role in cancer research and treatment. It can assist in identifying genetic markers associated with different types of cancer, analyzing treatment response patterns, and even suggesting potential therapeutic targets. By leveraging genomics data, ChatGPT can contribute to the development of more effective and personalized cancer treatments.
Bridgett, your article presents an exciting prospect for the genomics field. How does ChatGPT handle noisy or low-quality genomic data during the processing stage?
Thank you, Liam. ChatGPT can encounter noisy or low-quality genomic data during processing. To mitigate these challenges, pre-processing techniques such as data filtering, outlier detection, and error correction can be employed to improve the quality of the input data. These steps help reduce noise and enhance the reliability of the results generated by ChatGPT.
Wonderful article, Bridgett. Could you shed some light on the computational requirements and resources necessary to utilize ChatGPT effectively in genomic data processing?
Thank you, Lucy. Computational requirements for ChatGPT can vary depending on the size and complexity of the genomic data being processed. Models like GPT-3 are computationally expensive and typically require high-performance computing resources or cloud-based services. However, optimizations and model distillation techniques can help make ChatGPT more computationally accessible for researchers with limited resources.
Bridgett, I found your article fascinating. In terms of domain specificity, can ChatGPT be fine-tuned to cater to specific genomics subfields or research areas?
Hello Oliver. Yes, ChatGPT can indeed be fine-tuned to cater to specific genomics subfields or research areas. By training the model on relevant datasets or pre-training it with domain-specific knowledge, researchers can enhance its performance and make it more specialized for particular tasks or areas of interest within genomics.
Bridgett, your article highlights the potential of ChatGPT. Do you foresee any challenges in training or validating models that leverage such vast amounts of genomics data?
Thank you, Emily. Training and validating models with vast amounts of genomics data do pose challenges. Addressing issues like data quality, class imbalance, and selection bias becomes crucial during both the training and validation stages. Researchers must ensure that the models are learning meaningful patterns and that they generalize well to unseen data for robust and reliable genomics data processing.
Bridgett, your article raises interesting prospects for ChatGPT in genomics. Are there any user interface considerations that need to be taken into account when developing ChatGPT tools for genomics?
Good question, Jake. User interface considerations are important for effective utilization of ChatGPT in genomics. The design of the user interface should make it intuitive, easily navigable, and capable of handling complex queries related to genomics data. Usability testing and incorporating user feedback can ensure that the user interface aligns with researchers' workflow and facilitates a seamless experience.
Bridgett, your article is insightful. How can researchers ensure the transparency and reproducibility of the results obtained through ChatGPT in genomics?
Thank you, Mia. Ensuring transparency and reproducibility is crucial. Researchers can achieve this by documenting the training process, including details about the specific model version, hyperparameters, and training data used. Sharing the code and resources can also help others replicate the results. Open communication and collaboration within the research community contribute to transparency and reproducibility in genomics using ChatGPT.
Bridgett, your article on ChatGPT and genomics is captivating. What are the primary computational challenges associated with processing genomic data using deep learning models?
Thank you, Noah. Processing genomic data using deep learning models like ChatGPT comes with computational challenges. The large dimensionality of genomic data, feature extraction complexities, and the need for substantial computing power can pose hurdles. Efficient data representation techniques, parallelization, and utilizing specialized hardware can help overcome these computational challenges and enable effective genomic data processing.
Bridgett, your article provides valuable insights into the potential of ChatGPT in genomics. How do you see the role of human experts alongside ChatGPT in biomarker discovery?
Hello Mason. Human experts play a crucial role alongside ChatGPT in biomarker discovery. Their domain expertise, judgment, and ability to incorporate biological knowledge can effectively complement ChatGPT's capabilities. By combining the strengths of human experts and AI models like ChatGPT, researchers can achieve more comprehensive and accurate biomarker discovery results.
Bridgett, your article is informative. How do you envision the adoption of ChatGPT in genomics by the broader research community or industry?
Thank you, Jacob. The adoption of ChatGPT in genomics by the broader research community and industry is an exciting prospect. As the technology evolves and becomes more accessible, it has the potential to revolutionize genomics research, biomarker discovery, and enable novel applications. Collaborative efforts, user-friendly interfaces, and accessible resources can drive the adoption and integration of ChatGPT into various genomics workflows.
Interesting insights, Bridgett. In terms of challenges, could you elaborate on any ethical considerations related to the use of ChatGPT for genomic data processing?
Thank you, Eva. Ethical considerations are paramount. It's crucial to handle genomic data with care, ensuring privacy, security, and proper consent protocols. Additionally, avoiding biases in the interpretation and output of ChatGPT is essential. Transparency, responsible data usage, and ethical guidelines should underpin the application of ChatGPT in genomics to uphold ethical standards.
Bridgett, thanks for sharing your expertise in this article. Are there any specific challenges associated with deploying ChatGPT for real-time analysis of genomic data?
Hello Lucas. Deploying ChatGPT for real-time analysis of genomic data does present challenges. The computational requirements and processing time can vary depending on the size and complexity of the data. Optimizations and parallelization techniques are essential to ensure timely analysis. Striking a balance between accuracy and speed is crucial when deploying ChatGPT for real-time genomics data analysis.
Bridgett, your article is enlightening. Considering the complexity of genomics, can ChatGPT handle different types of genomic data, such as DNA sequencing data or gene expression data?
Thank you, Owen. Yes, ChatGPT can handle different types of genomic data, including DNA sequencing data and gene expression data. By training models on diverse datasets, ChatGPT can learn patterns and generate insights from various genomic data formats. Its versatility allows researchers to apply it to a wide range of genomics data analysis tasks.
Impressive article, Bridgett. I'm curious, does ChatGPT have any known limitations when it comes to working with large-scale genomic datasets?
Thank you, Isaac. ChatGPT does have limitations when working with large-scale genomic datasets. The computational demands for training and processing such datasets can be substantial, requiring high-performance resources. Efficient data processing techniques and infrastructure optimizations are crucial to ensure that ChatGPT can effectively handle and analyze large-scale genomics data.
Bridgett, your article presents exciting possibilities. Can ChatGPT also assist in identifying potential biomarkers for rare genetic diseases or conditions?
Hello Aaron. ChatGPT can indeed assist in identifying potential biomarkers for rare genetic diseases or conditions. By analyzing genomic data, it can help researchers uncover genetic variations that may be associated with these diseases or conditions. The ability to handle diverse datasets and process complex queries makes ChatGPT a valuable tool in identifying biomarkers, even for rare genetic diseases.
Bridgett, your article is compelling. Could you elaborate on how ChatGPT can contribute to advancing our understanding of the functional elements within the non-coding regions of the genome?
Thank you, Gabriel. ChatGPT can contribute to advancing our understanding of non-coding regions of the genome. By analyzing large amounts of genomic data, it can uncover patterns and potential functional elements within non-coding regions. This can aid in identifying regulatory elements, non-coding RNAs, and other components that play critical roles in gene regulation and overall genomic function.
Bridgett, your article provides valuable insights into ChatGPT's potential in genomics. Could you elaborate on how it has been utilized in clinical decision support systems?
Hello Joseph. ChatGPT has been utilized in clinical decision support systems by providing insights, recommendations, and knowledge-based information for healthcare professionals. By interpreting and analyzing genomics data, it can assist in treatment planning, therapeutic recommendations, and aiding clinical workflow. The integration of ChatGPT into such systems helps combine genomics with patient care to deliver more personalized and informed decisions.
Bridgett, your article on ChatGPT and genomics is insightful. How can researchers ensure the generalization and robustness of ChatGPT when applied to diverse genomics datasets?
Thank you, Sophie. Ensuring generalization and robustness of ChatGPT when applied to diverse genomics datasets is crucial. Researchers must focus on training the model on representative, diverse datasets that cover various genomic variations and contexts. Ensemble approaches and cross-validation techniques can help assess and improve the model's generalization capabilities. Rigorous evaluation and testing across different genomics datasets are essential for ensuring robustness and reliable performance.