Revolutionizing Book Review Technology: Utilizing ChatGPT for Anomaly Detection
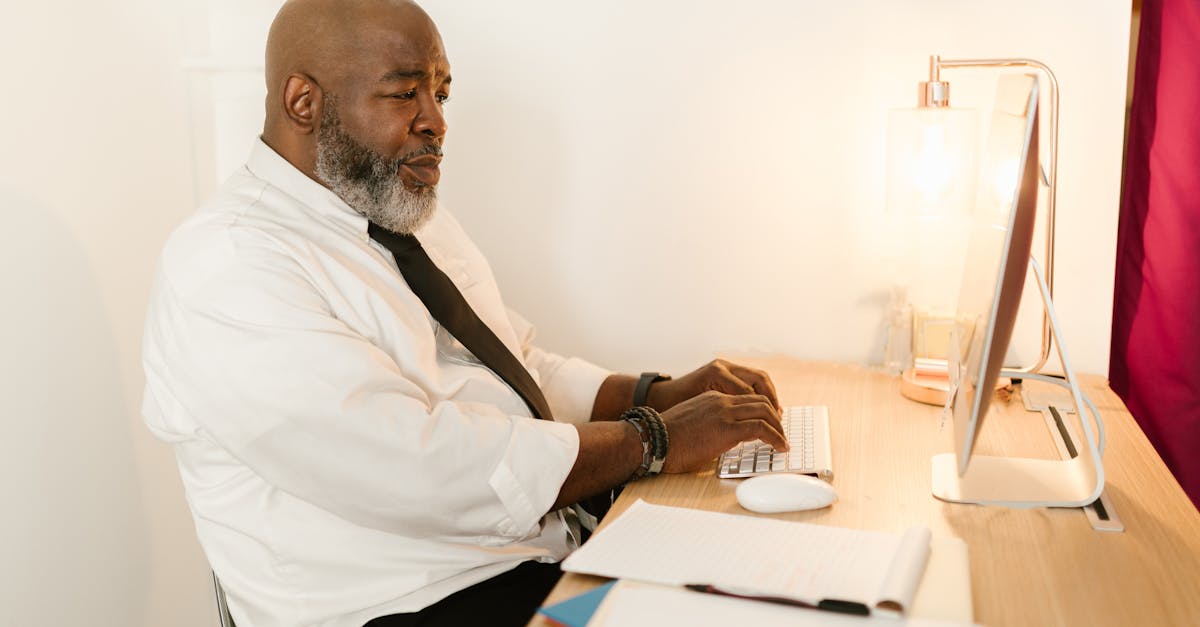
In the digital age, book reviews have become an integral part of the decision-making process for readers. These reviews provide valuable insights into the quality, content, and overall appeal of a book. However, with the rise of fake reviews and biased opinions, it has become increasingly difficult for readers to trust the authenticity of these online reviews. This is where anomaly detection comes into play.
Understanding Anomaly Detection
Anomaly detection is a technology that aims to identify data points or patterns that deviate significantly from what is considered normal or expected. In the context of book reviews, anomaly detection can be used to detect reviews that are outliers in terms of sentiment, writing style, or other relevant criteria. By identifying these anomalous reviews, readers can be equipped with a more accurate understanding of a book's actual quality.
How Anomaly Detection Works in Book Reviews
Anomaly detection in book reviews involves the analysis of various data points associated with each review, such as the ratings, text content, reviewer's history, and more. These data points are then compared to the general patterns observed in the larger set of reviews for a particular book or across multiple books. Anomalies can be identified based on statistical methods, machine learning algorithms, or a combination of both.
For example, if a particular book consistently receives positive reviews with an average rating of 4 stars, and a new review gives it a rating of 1 star, this review may be considered an anomaly. Similarly, if most reviews for a book are filled with praise while one review is excessively critical without any valid reasons, it can also be marked as an anomaly.
Benefits of Anomaly Detection in Book Reviews
The utilization of anomaly detection in book reviews offers several advantages:
- Improved trustworthiness: By identifying and filtering out anomalous reviews, readers can have more confidence in the reliability of the remaining reviews.
- Time-saving: Anomaly detection allows readers to quickly identify the most trustworthy and informative reviews, saving them time and effort in finding the right books to read.
- Greater accuracy: By removing biased or fake reviews from the dataset, anomaly detection ensures that the overall ratings accurately reflect the quality of a book, making it easier for readers to make informed decisions.
- Constant adaptation: Anomaly detection models can be continuously trained and updated to adapt to evolving patterns and new types of anomalies, ensuring their effectiveness in the long run.
Limitations and Challenges
While anomaly detection technology is highly valuable in the realm of book reviews, it is not without its limitations and challenges. Some of these include:
- Data quality: Anomaly detection heavily relies on the availability of accurate and reliable data. If the dataset contains low-quality or biased reviews, the effectiveness of the model may be compromised.
- Human subjectivity: Determining what constitutes an anomaly can be subjective, as different readers may have varying opinions on what is considered normal or abnormal.
- Adversarial attacks: Similar to other applications of artificial intelligence, anomaly detection models for book reviews can be vulnerable to adversarial attacks, where malicious agents attempt to deceive the system by generating fake reviews that go undetected.
Conclusion
Anomaly detection technology in the context of book reviews is a powerful tool that helps readers separate the signal from the noise. By identifying reviews that deviate significantly from the norm, readers are empowered to make more informed decisions about the books they choose to read. While challenges do exist, the benefits of utilizing anomaly detection in book reviews far outweigh the potential drawbacks, ultimately enhancing the trustworthiness and accuracy of online book review platforms.
Comments:
Thank you all for reading my article on revolutionizing book review technology! I'm excited to hear your thoughts and opinions.
Great article, Barbara! I think utilizing ChatGPT for anomaly detection in book reviews is a brilliant idea. It could help identify biased or suspicious reviews.
I agree, Steven. It's important to combat fake reviews and maintain the integrity of the reviewing system. Technology can certainly help with that.
I have mixed feelings about this approach. While it may be useful in detecting anomalies, relying solely on technology might eliminate the human touch that book reviews often offer.
I share your concerns, Sophia. Technology should support human reviewers rather than replacing them completely. A combination of both could be ideal.
But won't this system also flag genuine but unconventional opinions as anomalies? We should be careful not to suppress diverse perspectives.
That's a valid point, David. We don't want to discourage honest reviewers who bring unique insights to the table. Balancing the technology is crucial.
This sounds like a promising development! By automating the detection of suspicious reviews, we can save time and provide readers with more reliable information.
I completely agree, Michelle. It would greatly benefit both authors and readers. Genuine reviews are crucial for making informed decisions.
Absolutely, Peter. Real reviews from real people are what make the book community thrive. Technology is a useful aid, but it should never replace that.
I'm curious to know how accurate the anomaly detection of ChatGPT really is. Are there any benchmarks or case studies available?
Hi Robert! There have been several studies evaluating the accuracy of ChatGPT for anomaly detection in various domains. I'd be happy to share some references with you.
That would be great, Barbara! I'm particularly interested in how it handles subtle anomalies and avoids false positives.
Sure, Robert! I'll send you some research papers later today. Stay tuned!
I worry that relying on technology too much might limit the human interaction between authors and reviewers. Personal opinions and discussions are valuable.
You raise an important point, Linda. The goal is not to eliminate human interaction completely but rather enhance the review process with additional tools.
Indeed, Linda. While technology can improve the efficiency and reliability of book reviews, it shouldn't replace the genuine connections between authors and readers.
I appreciate all your thoughts! It's great to see different perspectives on this topic. Let's continue the discussion and explore further aspects of book review technology.
Barbara, thank you for shedding light on this innovative use of ChatGPT. It's exciting to envision how this technology can shape the future of book reviews.
You're welcome, James! I'm glad you find it exciting. The potential for improving the reviewing system is vast, and I'm optimistic about the future too.
I wonder if there are any ethical concerns regarding the use of AI technology like ChatGPT for anomaly detection. Any thoughts on that?
Ethical considerations are crucial, Emma. While AI technology can provide valuable insights, it's important to ensure fairness, transparency, and avoid potential biases.
AI-powered anomaly detection should be used carefully. We need to address potential risks associated with false positives and protect freedom of expression.
Absolutely, Julia. Striking the right balance between anomaly detection and preserving free expression is essential to make this technology valuable and reliable.
I'm curious if the ChatGPT anomaly detection could also be used for other forms of written content, like movie or product reviews. Any thoughts?
That's an excellent question, Sarah! The principles could certainly be extended to various domains, providing valuable insights and ensuring better review systems overall.
I believe the potential applications are vast. By leveraging advanced language models like ChatGPT, we could improve the credibility of many online review platforms.
Exactly, Daniel. The technology has the potential to revolutionize multiple domains beyond book reviews and help build more trustworthy and transparent platforms.
While anomaly detection is useful, it's critical not to neglect the role of human reviewers in judging the artistic and subjective aspects of a book.
I completely agree, Rachel. The human touch is irreplaceable when it comes to capturing the nuances of artistic works. Technology should be an aid, not a replacement.
Could ChatGPT be used to automatically suggest potential book reviewers based on their writing style and preferences?
That's an intriguing idea, Oliver! While it's possible in theory, there might be challenges in accurately matching preferences and ensuring diversity in the selection process.
Automating reviewer suggestions could be a double-edged sword. It might limit exposure to different perspectives and result in echo chambers.
Indeed, Ethan. Diversity in book reviewers is crucial to maintain a vibrant ecosystem. Technology could assist in the process but should not replace human judgment.
Having technology suggest potential reviewers could be useful, but it should be used as a starting point. Human review and selection are still necessary.
Well said, Lisa! Technology can provide valuable recommendations, but the final decision should always involve human expertise, acknowledging the complexity involved.
What measures can be taken to avoid potential manipulation of the anomaly detection system by malicious actors?
Valid concern, Michael. Building robust defenses against manipulation is crucial. Techniques like adversarial training and continuous monitoring can help mitigate the risks.
Regular updates and improvements to the system would also be necessary to keep up with evolving manipulation techniques.
You're absolutely right, Sophie. As malicious actors evolve, so should our defense mechanisms. Continuous improvement and adaptability are key.
I wonder if ChatGPT could provide real-time feedback to reviewers while they are writing their reviews, helping them avoid potential anomalies from the start.
That's an interesting concept, Nathan! Real-time feedback could indeed assist reviewers in constructing more accurate and anomaly-free reviews. It's worth exploring further.
Barbara, that would be a game-changer! It could help reviewers improve their writing and catch any potential issues as they go.
I'm glad you find it intriguing, Julian! Empowering reviewers with real-time feedback could enhance the review writing experience and ultimately benefit the readers as well.
Thank you all for the lively discussion! I appreciate the valuable insights and different perspectives shared here. Let's continue to explore the potential of book review technology together.