Revolutionizing Cataloging: Harnessing ChatGPT for Efficient Technology Categorization
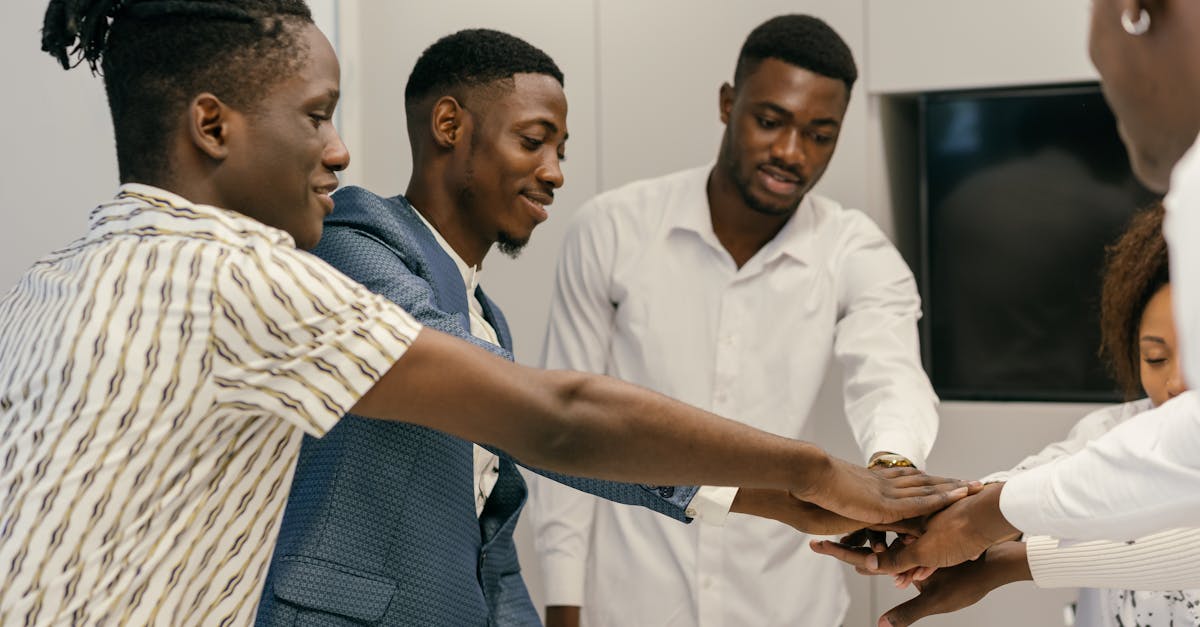
Introduction
In the digital age, libraries face the challenge of managing large volumes of data efficiently. Cataloging plays a crucial role in organizing and categorizing information in library databases. To enable accurate and swift classification, chatbot technology, such as GPT-4, has emerged as a valuable tool. This article explores how chatbots enhance the process of library database classification and highlights their significance in improving information management.
The Role of Chatbots in Library Database Classification
Chatbots equipped with advanced machine learning algorithms have the capability to analyze and interpret vast amounts of textual information. By training them on existing library databases, these chatbots can learn and understand the intricacies of library database classification systems.
Utilizing natural language processing techniques, chatbots can identify key elements within texts such as titles, authors, subjects, and categorize them accordingly. This eliminates the need for manual intervention, reducing human error and enhancing efficiency.
Benefits of Chatbot Technology in Library Database Classification
1. Accurate and Consistent Categorization: Chatbots excel at providing accurate and consistent categorization of textual data in library databases. By eliminating human biases and inconsistencies, they ensure a reliable and standardized classification system.
2. Time Efficiency: Manual classification of library databases can be a time-consuming process, especially when dealing with large volumes of data. With the assistance of chatbots, the classification process can be automated, enabling libraries to categorize and store information swiftly, saving valuable time and resources.
3. Scalability and Adaptability: As libraries continue to amass an ever-increasing amount of digital content, chatbots offer a scalable solution. By leveraging their ability to handle extensive datasets, chatbots can effortlessly adapt to the growing demands of library database classification.
4. Enhanced User Experience: By streamlining the classification process, chatbots allow library patrons to locate information more efficiently. The ease of navigation provided by well-organized databases enhances the user experience, making libraries more accessible and user-friendly.
Challenges and Future Outlook
While chatbot technology brings numerous benefits to library database classification, there are challenges that need to be addressed. Ensuring the accuracy and reliability of chatbot categorizations remains a primary concern. Continuous monitoring and improvement of the chatbot's algorithms are necessary to maintain the integrity of the database.
Looking ahead, advancements in artificial intelligence and machine learning will likely lead to more sophisticated chatbot technology. The development of GPT-4 and subsequent iterations will further enhance the capabilities of library database classification systems, enabling more accurate and efficient categorization.
Comments:
Thank you all for reading my article on 'Revolutionizing Cataloging: Harnessing ChatGPT for Efficient Technology Categorization'. I hope you found it interesting! Feel free to share your thoughts and comments below.
Great article, Saad! The potential of ChatGPT in revolutionizing cataloging is impressive. I can see it significantly improving the efficiency of technology categorization. Are there any specific limitations or challenges that you foresee in implementing this approach?
Thanks, Paula! You raise a good point about the limitations of ChatGPT. While the model performs admirably, it may still struggle with understanding complex technical jargon and nuances. Human oversight and continuous training will be crucial to ensure accurate categorization. Additionally, scaling this approach to handle large catalogs could pose challenges. However, with further refinement, I believe this technology has the potential to overcome these limitations.
Impressive work, Saad! I can see how ChatGPT's natural language processing abilities can greatly enhance the accuracy and speed of technology categorization. Do you think this approach could be applied to other domains beyond technology?
Thank you, Philip! Absolutely, the approach of harnessing ChatGPT can indeed be extended to other domains. Any field that requires the categorization of large amounts of textual data can benefit from the capabilities of this technology. With appropriate fine-tuning and domain-specific training data, the potential applications are vast!
Hi Saad, thank you for sharing your insights on this fascinating topic. I can see how ChatGPT can streamline the cataloging process, but I'm curious about potential biases in the categorization. How do you ensure fairness and mitigate any inherent biases that may exist in the data or model?
Hi Emily, that's an important concern. Bias mitigation is a critical aspect while using AI models. When training ChatGPT, we strive to use diverse and representative data to avoid perpetuating bias. Rigorous evaluation processes are in place to identify any biases that may arise. Additionally, actively involving human reviewers helps in identifying and correcting any potential bias. Transparency and continuous improvement are key principles in ensuring fairness in categorization.
Excellent article, Saad! I can see immense potential in using ChatGPT for technology categorization. However, how would you address the challenge of CPG (Common Pool Goods), where information might be proprietary or closely guarded by companies? Does ChatGPT rely heavily on publicly available information?
Thank you, Michael! You raise an important consideration. While ChatGPT can leverage publicly available information for categorization, it doesn't rely solely on it. In scenarios where proprietary or closely guarded information is involved, organizations can restrict the training data to avoid exposing sensitive details. The model, even with limited access, can still benefit from the general knowledge and understanding of technology without breaching proprietary information.
Hi Saad, fantastic article! I'm curious about the scalability of ChatGPT for large catalogs. Can it handle thousands of technology items and maintain efficient categorization? Or are there practical limits to consider?
Hi Jasmine, scalability is indeed a crucial factor. While ChatGPT can handle a significant amount of data, there may be practical limits when it comes to extremely large catalogs with thousands of items. However, by leveraging distributed computing resources and optimized implementation, it's possible to enhance its scalability. Building on the existing capabilities, future developments can further enhance ChatGPT's ability to efficiently categorize large catalogs.
Impressive research, Saad! I'm curious about the training process behind ChatGPT. How do you ensure that the model achieves accurate categorization? Are there any challenges in obtaining sufficient training data?
Thank you, Daniel! Training ChatGPT involves feeding it large amounts of diverse and high-quality data, including technology-related texts and categorization labels. The model learns to associate the textual patterns with appropriate categories. Obtaining high-quality training data can sometimes pose challenges, especially for specialized domains or specific categories. Careful data curation and involvement of human reviewers for validation and iterative improvement are used to ensure accurate categorization.
Very informative article, Saad! I was wondering, what are the advantages of using ChatGPT for technology categorization compared to more traditional rule-based approaches?
Thank you, Hannah! One of the advantages of ChatGPT is its ability to learn categorization based on contextual understanding from vast amounts of data. Traditional rule-based approaches are often limited by predefined rules and may not handle complex language patterns effectively. ChatGPT's flexibility, adaptability, and ability to improve with new datasets make it a compelling option for technology categorization, especially when dealing with diverse and constantly evolving products and technologies.
Great article, Saad! How does ChatGPT handle ambiguous or unclear descriptions of technology items? Can it accurately categorize them?
Thanks, Larry! ChatGPT can handle to some extent ambiguous or unclear descriptions of technology items. However, it may encounter challenges in accurately categorizing such instances without additional context or clarification. This is where human reviewers play a crucial role in providing feedback and helping the model make more informed categorization decisions. Iterative improvement and active learning from ambiguity are key aspects of refining the accuracy and understanding of ChatGPT.
Fantastic read, Saad! How do you see the future of cataloging evolving with the emergence of AI technologies like ChatGPT? Will it completely replace manual categorization efforts?
Hi Sophia, AI technologies like ChatGPT hold immense promise in transforming cataloging processes. While they can greatly enhance efficiency, accuracy, and scalability, complete replacement of manual categorization efforts may not be realistic. Human expertise will continue to play a vital role, especially in refining categorization models, addressing edge cases, and ensuring fairness. The future lies in leveraging the complementary strengths of AI and human intelligence to achieve optimal results.
Great insights, Saad! I can see the potential of ChatGPT in revolutionizing cataloging. With the continuous advancements in AI, how do you plan to address the challenge of keeping up with new technologies and terminology?
Thank you, Melissa! Staying up-to-date with new technologies and terminology is indeed a challenge. As the field evolves, it's crucial to continuously train and fine-tune the categorization models with new datasets. Having an iterative feedback loop involving domain experts, industry collaboration, and technology tracking mechanisms can help address this challenge. The flexibility of ChatGPT allows for quick updates and adaptability to changing technology landscapes.
Excellent article, Saad! Could you provide more insight into the potential applications beyond cataloging where ChatGPT's capabilities can be leveraged?
Thank you, Robert! ChatGPT's capabilities have broad applications beyond cataloging. It can be utilized in customer support chatbots, content analysis, information retrieval, and even creative writing assistance, among others. The ability to understand and generate human-like text makes it a versatile language model. As research progresses and models improve, we can expect to witness ChatGPT's impact in various domains where natural language understanding and generation are valuable.
Wonderful article, Saad! With the increasing concerns about privacy and data security, how does ChatGPT handle sensitive information while categorizing technology?
Thank you, Megan! Privacy and data security are vital considerations. ChatGPT categorizes technology based on patterns and knowledge learned from training data, but it doesn't store or retain sensitive information itself. Organizations implementing ChatGPT would need to handle any sensitive data appropriately and take measures to safeguard privacy, ensuring compliance with relevant regulations and industry best practices.
Great insights, Saad! Can ChatGPT be fine-tuned to cater to specific domain requirements, such as medical technology or finance, for more accurate categorization?
Thanks, Liam! Absolutely, ChatGPT's fine-tuning can be tailored to specific domain requirements. By providing domain-specific training data and categorization labels, the model's performance and accuracy in categorizing medical technology or finance-related items can be enhanced. Fine-tuning with narrower categorization scopes enables better alignment with the nuances and specific language patterns in different domains.
Impressive work, Saad! How does ChatGPT handle multilingual technology items and categorization in languages other than English?
Thank you, Isabella! ChatGPT's capabilities extend beyond English, and it can handle categorization in multiple languages. However, the model's performance may vary depending on the availability of training data in different languages. Adequate training data for various languages and continuous improvement efforts can help ensure accurate categorization across a range of languages.
Very thought-provoking article, Saad! How do you see the collaboration between AI models and human experts evolving in the future for efficient technology categorization?
Thank you, Oliver! Collaboration between AI models and human experts will continue to evolve and become more synergistic. While AI models like ChatGPT can automate categorization at scale, human experts bring invaluable domain knowledge, context, and judgment. The future lies in seamless interaction and feedback loops. Human experts can guide and train the models, identify edge cases, and ensure fairness, while AI systems assist in streamlining the categorization process and reducing manual effort.
Fantastic insights, Saad! When implementing ChatGPT for technology categorization, how would you balance the need for speed and efficiency with the requirement for accurate categorization?
Thank you, Victoria! Balancing speed and efficiency with accurate categorization is indeed crucial. Initially, the model's performance may rely more on human reviewers to ensure accuracy. As the system gains experience and knowledge, it can progressively handle more categorization tasks independently, reducing the need for human intervention. Continuous training, feedback loops, and iterative improvements allow striking the right balance between speed, efficiency, and accuracy.
Great article, Saad! As ChatGPT is trained on existing data, how does it handle technology items that are new or not present in the training data?
Thanks, Lucas! ChatGPT can encounter challenges with technology items that are not present in the training data or are relatively new. In such cases, the model's categorization may rely more on general knowledge and patterns learned from training on existing data. Human reviewers play a valuable role in providing feedback and helping the model handle novel items. Continuous model updates and incorporating new relevant data can also improve its ability to categorize emerging technologies effectively.
Very interesting article, Saad! How do you ensure the transparency of the categorization logic and decision-making process employed by ChatGPT?
Thank you, Grace! Transparency is vital when it comes to AI models. While exact details of the categorization logic are complex and may not be explicitly defined, ChatGPT's training process involves providing categorization labels for the model to learn from. To ensure transparency, organizations employing ChatGPT can document guidelines, involve human reviewers in iterative feedback, and have processes in place to review and explain the model's decision-making when needed.
Wonderful insights, Saad! How do you envision the future evolution of ChatGPT in terms of improvements and addressing the limitations you mentioned?
Hi Sophie, the future of ChatGPT holds exciting possibilities. Improvements will focus on scaling the system to handle larger catalogs, refining its understanding of complex technical jargon, and minimizing biases. Increased fine-tuning options for specific domains and languages, better support for handling ambiguity, and more user-friendly interfaces are likely areas of improvement. Continuous research, development, and collaboration with the AI community and user feedback will drive the evolution of ChatGPT to address limitations and unlock further potential.
Impressive work, Saad! Is ChatGPT primarily focused on categorizing technology products, or can it be applied to other types of items as well?
Thank you, Ethan! While the article primarily focuses on technology categorization, the methodology can be applied to various types of items beyond technology. ChatGPT's ability to learn from textual patterns and make informed categorization decisions can benefit diverse domains, such as consumer goods, services, or any domain involving large catalogs that require efficient categorization.
Great insights, Saad! What are some of the real-world applications where ChatGPT's efficient technology categorization can have a significant impact?
Thanks, Maria! ChatGPT's impact can be significant in real-world applications involving technology categorization. E-commerce platforms can leverage it to enhance search accuracy and product recommendations. Technology review websites can benefit from efficient categorization for improved organization and searchability. IT departments can streamline inventory management and asset tracking. Overall, any system dealing with large technology catalogs can find value in leveraging ChatGPT's efficient categorization capabilities.
Fantastic article, Saad! How does ChatGPT handle cases where technology items belong to multiple categories or subcategories?
Thank you, Dylan! Handling cases where technology items have multiple categories or subcategories can be challenging. ChatGPT, in its current form, primarily associates items with specific categories. However, by incorporating additional signals or using hierarchical categorization schemes, it could be possible to handle multi-categorization scenarios more effectively. This is an area where the model's capabilities can be further explored and refined to provide more accurate results.
Very insightful article, Saad! How do you ensure efficient training of the model without sacrificing the quality and accuracy of categorization?
Thank you, Sarah! Efficient training while maintaining quality and accuracy is a delicate balance. Initial training involves a large dataset, but to ensure accuracy, human reviewers play a crucial role by providing validation and feedback. By combining automated systems with human guidance, it is possible to strike that balance. Iterative improvements and training on relevant subsets of data can help further refine the model's understanding while achieving efficient categorization.
Wonderful insights, Saad! How do you see the role of AI in cataloging evolving in the next few years, and what are the potential challenges that need to be addressed?
Hi Olivia, AI's role in cataloging will likely continue to transform the process in the coming years. With advancements in natural language processing and models like ChatGPT, automation and efficiency will increase. However, challenges such as bias mitigation, privacy concerns, scalability, and accurate handling of novel and ambiguous items will need to be addressed. Collaboration between AI researchers, domain experts, and industry stakeholders will be critical in driving positive and responsible developments in cataloging.
Great article, Saad! How do you handle user feedback during the implementation of ChatGPT for technology categorization?
Thanks, Emma! User feedback is invaluable in the implementation of ChatGPT for technology categorization. It helps in identifying areas of improvement, assessing accuracy, and addressing specific user needs. Feedback mechanisms, such as user surveys, crowdsourced annotations, or direct communication channels, can be established to collect, analyze, and act upon user feedback. Incorporating user insights and continuously fine-tuning the model based on user experiences is a crucial part of refining and enhancing the system.
Very informative article, Saad! Does ChatGPT have any mechanisms in place to handle potential adversarial inputs or attempts to manipulate categorization results?
Thank you, Zoe! ChatGPT does have some inherent defenses against adversarial inputs and manipulation attempts. The model's training involves exposure to a wide range of data and patterns, which allows it to handle a certain level of noise or ambiguous input. Additionally, the involvement of human reviewers helps in detecting and mitigating potential manipulations. Continuous monitoring, scrutiny, and improvement processes are essential in maintaining the integrity and reliability of ChatGPT's categorization results.
Impressive insights, Saad! How would you compare ChatGPT's performance in technology categorization to other AI models or approaches in the field?
Thanks, Benjamin! Comparing ChatGPT's performance to other AI models or approaches would require specific benchmarking and evaluation in the field of technology categorization. While ChatGPT has shown promising capabilities, it's important to consider alternative models, rule-based approaches, and domain-specific optimization techniques. Each approach may have its unique strengths and limitations, making it essential to evaluate performance in context-specific scenarios.
Hi Saad, great article! How do you ensure the fairness and inclusivity of ChatGPT's categorization, especially when dealing with different cultural perspectives and biases?
Hi Jordan, ensuring fairness and inclusivity in categorization is crucial. When training ChatGPT, efforts are made to use diverse and representative data to avoid perpetuating bias. Human review processes play a vital role in identifying and correcting any potential biases. Moreover, engaging reviewers from diverse backgrounds and cultural perspectives helps diminish biases and ensures a more inclusive understanding of technology. Continuous evaluation and improvement are key to maintaining fairness in categorization.
Very thought-provoking article, Saad! How do you handle cases where the same technology item can be categorized differently by different experts or reviewers?
Thank you, Adam! The potential for different categorizations by different experts or reviewers exists due to subjectivity or varying interpretations. To handle such cases, organizations implementing ChatGPT can establish clear guidelines and train reviewers to ensure consistency in categorization decisions. Implementing feedback loops, maintaining open communication channels, and addressing edge cases through collective learning contribute to minimizing inconsistencies and enhancing the accuracy of categorization.
Wonderful article, Saad! Can ChatGPT assist in automatically generating or suggesting category labels for technology items, or is it primarily focused on categorization?
Thank you, Sophie! ChatGPT's capabilities extend beyond just categorization. In addition to categorizing technology items, it can potentially assist in generating or suggesting category labels. By leveraging both its language understanding and generation capabilities, it can facilitate the process of labeling technology items, potentially saving significant manual effort in managing and organizing large catalogs.
Great article, Saad! How does ChatGPT handle instances where a technology item could be categorized under multiple relevant categories?
Thanks, Grace! In cases where a technology item could belong to multiple categories, ChatGPT, in its current form, primarily associates items with specific categories. However, by utilizing hierarchical categorization structures or incorporating additional signals, it could be possible to expand its capabilities to handle multi-category scenarios more effectively. Future research and refinements can address this aspect to provide more nuanced and accurate categorization results.
Fantastic insights, Saad! What are the potential ethical considerations to keep in mind while implementing AI models like ChatGPT for technology categorization?
Thank you, Joshua! Implementing AI models like ChatGPT requires careful ethical considerations. Transparency, bias mitigation, privacy protection, and fairness are foremost concerns. By following established ethical guidelines, organizations can ensure transparency of the model and decision-making process. Continuous monitoring, rigorous evaluations, and diverse human feedback help identify and mitigate biases. Safeguarding user privacy, handling sensitive data responsibly, and complying with regulations play a critical role in maintaining ethical standards.
Very informative article, Saad! How do you evaluate the accuracy and effectiveness of ChatGPT's categorization, especially for diverse sets of technology items?
Thank you, Lucy! Evaluating the accuracy and effectiveness of ChatGPT's categorization involves multiple aspects. Human reviewers are involved in providing validation and feedback during training, which helps assess initial accuracy. Ongoing monitoring, periodic evaluations using diverse test sets, and comparison against human benchmarks contribute to accuracy assessment. Organizations can also leverage user feedback and gather validation data to continuously improve and validate the effectiveness of the categorization process.
Very informative article, Saad! How do you handle cases where technology items include user-generated content or non-standard language usage?
Thanks, Oliver! User-generated content and non-standard language usage can indeed pose challenges in technology categorization. While ChatGPT has the ability to handle a certain degree of variation, human reviewers play a vital role in identifying and addressing non-standard language patterns or jargon. Reviewers help inform the model's understanding of such instances, enabling it to effectively categorize technology items with user-generated content or deviations from standard language usage.
Great insights, Saad! How do you address user trust and explainability of ChatGPT's categorization results, especially when dealing with complex categorization decisions?
Thanks, Liam! User trust and explainability are essential. While explaining complex categorization decisions can be challenging, organizations can adopt strategies to ensure user trust. Providing transparency on the general methodology, guidelines, and interoperability with human reviewers helps build trust. Organizations can also explore techniques to generate explanations to offer insights into how ChatGPT arrived at specific categorization decisions, making the process more understandable and trustworthy to users.
Very thought-provoking article, Saad! Are there any specific technologies or frameworks that complement ChatGPT in improving technology categorization?
Thank you, Mia! Several technologies and frameworks can complement ChatGPT in technology categorization. Natural language processing libraries like spaCy or NLTK help with text preprocessing and linguistic analysis. Embedding techniques like word2vec or BERT enhance the representation of text. For large-scale distributed computing, frameworks like Apache Spark or TensorFlow help optimize performance. Integration with existing knowledge graphs and ontologies can further enhance categorization accuracy and alignment with established standards.
Impressive work, Saad! How do you handle cases where technology items have inconsistencies or inaccuracies in their descriptions?
Thank you, Ella! Handling inconsistencies or inaccuracies in technology item descriptions is important in maintaining categorization accuracy. ChatGPT, in its current form, handles text patterns and relies on training data for categorization. However, to address inconsistencies or inaccuracies, human reviewers help provide feedback, identify potential issues, and help improve the model's understanding. Continuous updates, feedback loops, and user input allow refining the system to handle cases with inconsistencies or inaccuracies more effectively.
Great insights, Saad! How does ChatGPT handle abbreviations, acronyms, or industry-specific jargon commonly used in technology descriptions?
Thanks, Lily! ChatGPT's training exposes it to a wide range of language patterns, including abbreviations, acronyms, and industry-specific jargon. While it can handle many such cases, it may still encounter challenges with less common or domain-specific terms. Human reviewers and feedback play an instrumental role in addressing such challenges, as their expertise and knowledge help the model improve its understanding and categorization of specialized terminology used in technology descriptions.
Impressive article, Saad! Can ChatGPT adapt to updates in technology categorization standards or new categorization frameworks?
Thank you, Ruby! ChatGPT's adaptability allows it to incorporate updates in technology categorization standards or new frameworks to some extent. By retraining the model with updated data and categorization labels, it can align with evolving standards. However, significant shifts in categorization frameworks or entirely new frameworks may require more extensive model updates or fine-tuning. The flexibility of ChatGPT enables it to adapt and benefit from advancements in technology categorization methodologies.
Great article, Saad! How would you compare ChatGPT's categorization speed and efficiency to traditional manual categorization efforts?
Thanks, Eva! ChatGPT's categorization speed and efficiency compared to traditional manual efforts can be significantly higher. Once adequately trained, the model can rapidly process and categorize large quantities of technology items. Manual categorization efforts involve substantial time and effort, whereas ChatGPT's automated approach offers speed and scalability. However, human expertise is still vital for refining results, resolving edge cases, and ensuring quality, making ChatGPT a powerful tool in augmenting, rather than replacing, manual categorization.
Very informative article, Saad! How does ChatGPT ensure consistency and accuracy in categorization across different reviewers or users?
Thank you, Aiden! Ensuring consistency and accuracy across different reviewers or users is a crucial aspect. By establishing clear guidelines and providing training to reviewers, organizations can promote consistency. Feedback loops, open communication channels, and regular meetings or discussions help align the categorization decisions and address any inconsistencies or questions. Periodic calibration sessions where reviewers compare and discuss outcomes further enhance the accuracy and consistency of categorization.
Impressive work, Saad! How can organizations ensure the continuous improvement and accuracy of ChatGPT's categorization in real-world scenarios?
Thank you, Daniel! Continuous improvement and accuracy of ChatGPT's categorization can be achieved through multiple strategies. User feedback collection and incorporation, periodic human reviewer calibration, and training and fine-tuning with new or refined datasets all contribute to improvement. Maintaining open channels for user input, actively tracking categorization accuracy metrics, and prioritizing areas for refinement based on established benchmarks help ensure the ongoing accuracy and relevancy of ChatGPT's categorization performance in real-world scenarios.
Great insights, Saad! How does ChatGPT handle categorization in edge cases or technology items that do not neatly fit into existing predefined categories?
Thanks, Leo! In edge cases or instances where technology items don't fit into existing categories, ChatGPT's categorization may rely on general knowledge and patterns learned from the training data. Human reviewers play a vital role in addressing such cases by providing feedback and helping the model make more informed decisions. Continuous improvement, ongoing feedback loops, and including user input assist in refining the model's understanding and enhancing its ability to handle edge cases effectively.
Fantastic article, Saad! How can organizations ensure the privacy of user data while leveraging ChatGPT for technology categorization?
Thank you, Max! Ensuring privacy when leveraging ChatGPT for technology categorization is essential. Organizations must implement appropriate measures to protect user data. Limiting data access to relevant teams, adopting privacy protection techniques, and complying with privacy regulations minimize privacy risks. Organizations can also explore privacy-preserving methods, such as federated learning or on-device computations, to further safeguard user data. By prioritizing privacy and following secure data handling practices, organizations can instill confidence in users while utilizing ChatGPT for categorization.
Great insights, Saad! What are the key factors to consider when selecting or creating the training data for ChatGPT's technology categorization?
Thanks, Michael! Key factors in selecting or creating training data for ChatGPT's technology categorization include diversity, representativeness, and relevance. The data should cover a wide range of technology items, categories, and subcategories. It also helps to include specificity in terms of domains or industries when creating labels. Striving for a mix of real-world examples, industry-specific texts, and diverse sources assists in capturing varied language patterns. High-quality and curated data contribute to the accurate learning and categorization of technology items.
Impressive work, Saad! Can ChatGPT be fine-tuned to handle different classification hierarchies or taxonomies for technology categorization?
Thank you, Nathan! ChatGPT's flexibility allows it to be fine-tuned for different classification hierarchies or taxonomies. By modifying the training data and labels, the model can be adapted to handle specific categorization hierarchies relevant to different industries or applications. Fine-tuning allows aligning ChatGPT's categorization capabilities according to the needs of the context and desired taxonomies, enabling more customized and accurate results for technology categorization across different domains.
Great article, Saad! How do you ensure the generalizability of ChatGPT's categorization across different types and subtypes of technology items?
Thanks, Nicholas! Ensuring the generalizability of ChatGPT's categorization involves training the model on diverse and representative datasets covering a wide range of technology types and subtypes. The learning from this diverse training data enables the model to generalize across different items. Regular evaluations using diverse test sets help measure and improve the generalization capabilities. Inviting feedback from users and domain experts assists in identifying and addressing any gaps or limitations in the generalizability of the model's categorization.
Impressive insights, Saad! When implementing ChatGPT, how do you ensure the security and integrity of the data used for training and categorization?
Thank you, Samuel! Data security and integrity are vital considerations. Organizations implementing ChatGPT should follow best practices for securing training data, especially if it contains sensitive information. Measures like data encryption, access controls, and maintaining audit records help protect data integrity. Applying privacy protection techniques such as anonymization or differential privacy can further enhance security. Compliance with relevant regulations and periodic security assessments contribute to maintaining the security and integrity of the data used in training and categorization.
Very thought-provoking article, Saad! In cases where ChatGPT encounters uncertain categorization, can it seek clarification from users or request additional information?
Thanks, Emily! In its current form, ChatGPT doesn't have a built-in ability to seek clarification from users or request additional information. However, users can potentially engage in a dialog with clarifying questions to assist in categorization. Organizations can design interactive interfaces or systems to facilitate such clarification interactions. Incorporating user feedback and exploring ways to enhance ChatGPT's ability to handle uncertainty are avenues for future development, improving its capability to seek clarification during categorization.
Wonderful article, Saad! Can ChatGPT be integrated into existing cataloging systems, or is it a standalone solution?
Thank you, Ava! ChatGPT can be integrated into existing cataloging systems as part of a more comprehensive solution. Through suitable APIs or integration frameworks, organizations can leverage ChatGPT's categorization capabilities within their existing systems. This integration can streamline the categorization process, enhance search accuracy, or automate workflows. Whether used as a standalone solution or integrated into existing systems, ChatGPT's flexible deployment options empower organizations to align it with their specific cataloging requirements.
Thank you all for taking the time to read my article on revolutionizing cataloging with ChatGPT! I'm excited to hear your thoughts and answer any questions you may have.
Great article, Saad! I found your ideas on using ChatGPT for technology categorization really fascinating. It definitely seems like a promising approach for streamlining the process.
I agree, Emily! The potential of ChatGPT in revolutionizing cataloging is immense. Saad, could you provide more examples of how ChatGPT can be applied in this context?
Absolutely, Michael! One example would be using ChatGPT to categorize and tag technology products based on customer queries. By training the model on customer conversations, it can identify the most relevant categories and tags for different products.
Interesting concept, Saad! I can see how using ChatGPT to analyze customer queries and provide accurate categorization can greatly improve the efficiency of technology cataloging. Are there any limitations or challenges to consider?
Good question, Julia! While ChatGPT is powerful, it may struggle with interpreting ambiguous queries or understanding nuanced differences between categories. Additional fine-tuning and human oversight can help address these challenges.
Julia and Saad, how would ChatGPT handle cases where multiple categories are applicable to a query? Is there a prioritization process?
Good question, Paul. In cases where multiple categories apply, ChatGPT can offer suggestions to users and allow them to select the most accurate category themselves. Manual input and feedback play a crucial role in improving the categorization process.
Thank you, Paul and Saad! Allowing users to choose the most accurate category themselves sounds like a user-friendly approach. It adds a level of customization to the process.
I agree, Alice! Empowering users to make the final decision ensures a personalized experience and reduces the chances of misclassification. It's a win-win!
Saad, have you considered potential biases in the training data that could affect the categorization accuracy of ChatGPT?
That's an important point, Jonathan. Biases in training data can indeed impact accuracy. To mitigate this, it's crucial to have diverse and representative training data that includes a wide range of perspectives.
Jonathan, biases are a relevant concern. Saad, could you elaborate on how biases can be addressed during model training and implementation?
Certainly, Emma. To address biases, it's necessary to curate a diverse dataset during model training. This dataset should not favor any specific group or viewpoint, ensuring fair representation. Additionally, regular evaluation and bias detection can aid in minimizing biases during implementation.
I appreciate the thorough explanation, Saad. It's exciting to see how NLP models like ChatGPT can revolutionize cataloging processes. How are accuracy and performance measured in this context?
Thank you, Robert! Accuracy and performance in technology categorization can be measured using metrics like precision, recall, and F1-score. These metrics help determine the model's ability to correctly assign categories and tags to different products.
Robert, apart from precision and recall, are there any other metrics that can be used to evaluate the performance of ChatGPT in technology categorization?
Good question, Daniel. Besides precision and recall, other metrics like accuracy, confusion matrix, and mean average precision can provide additional insights into the model's performance.
Daniel, could you briefly explain the mean average precision metric and its significance in evaluating the performance of ChatGPT?
Certainly, Sophie! Mean average precision (MAP) considers both precision and recall at various thresholds. It calculates the average precision across all relevant categories, providing a comprehensive evaluation of the model's effectiveness in categorizing technology products.
Saad, I'm curious about the scalability of using ChatGPT for cataloging large volumes of products. Can it handle the high workload efficiently?
Great question, Nadia! ChatGPT can handle large volumes, but efficiency can vary depending on the hardware and resources available. Optimizing the infrastructure and model architecture can make the cataloging process more scalable.
Nadia and Saad, how can we ensure that the workload doesn't overwhelm the system, particularly during peak cataloging periods?
Emma, scaling infrastructure and optimizing resource allocation are key to handling peak cataloging periods efficiently. Load balancing techniques and parallel processing can help distribute the workload effectively.
Emma, Saad made an important point about having a diverse dataset during training. It helps prevent biases and ensures fairness in technology categorization. Transparency throughout the process is also crucial to address ethical concerns.
Absolutely, James! Transparency and fairness should be at the core of implementing AI-based models for cataloging. Continuous monitoring and auditing can help maintain ethical standards.
Emma and Saad, in terms of workload management, are there any techniques to prioritize time-sensitive cataloging tasks without compromising accuracy?
Good question, Olivia. Prioritizing time-sensitive cataloging can be done through task scheduling algorithms that consider both urgency and required accuracy. Smart task assignment ensures timely completion while maintaining high accuracy.
Saad, how often is retraining necessary to keep the cataloging model up to date? Technology advances quickly, so staying accurate is crucial.
Excellent point, Javier. Retraining is an ongoing process, especially for evolving technology categories. Regularly incorporating new data and fine-tuning the model ensures that it stays up to date with the latest advancements.
Javier, in addition to retraining, are there any other ways to keep the cataloging model up to date with the latest technology advancements?
Absolutely, Lucas. Apart from retraining, model performance can be continuously improved by regularly fine-tuning it with smaller updates using newly annotated data or through active learning techniques.
Saad, what are your thoughts on potential ethical concerns when using AI-based models for cataloging? Are there any risks to be mindful of?
Ethical considerations are vital, Sophia. AI models should be transparent, fair, and accountable. It's important to regularly review potential biases and ensure the technology categorization process doesn't inadvertently discriminate against any group.
Saad, I'm curious about your personal experience with implementing ChatGPT for technology cataloging. Have you seen significant improvements in efficiency and accuracy firsthand?
Thanks for asking, Oliver. As an early adopter, I've witnessed promising improvements in both efficiency and accuracy. ChatGPT has shown great potential for revolutionizing technology cataloging, but it's essential to continue refining the approach.
Saad, I'm wondering if there are any potential applications beyond technology cataloging where ChatGPT could be utilized effectively?
Absolutely, Alice! ChatGPT's capabilities extend beyond cataloging. It can be leveraged for customer support, content generation, and even personal assistants. The potential is vast!
Alice and Saad, could ChatGPT be utilized for sentiment analysis in customer reviews to enhance cataloging?
Certainly, Liam! ChatGPT can indeed be used for sentiment analysis. By understanding customer sentiment through their reviews, it can provide valuable insights to enhance cataloging and improve customer experience.