Revolutionizing Credit Risk Modeling: Harnessing the Power of ChatGPT in Credit Risk Technology
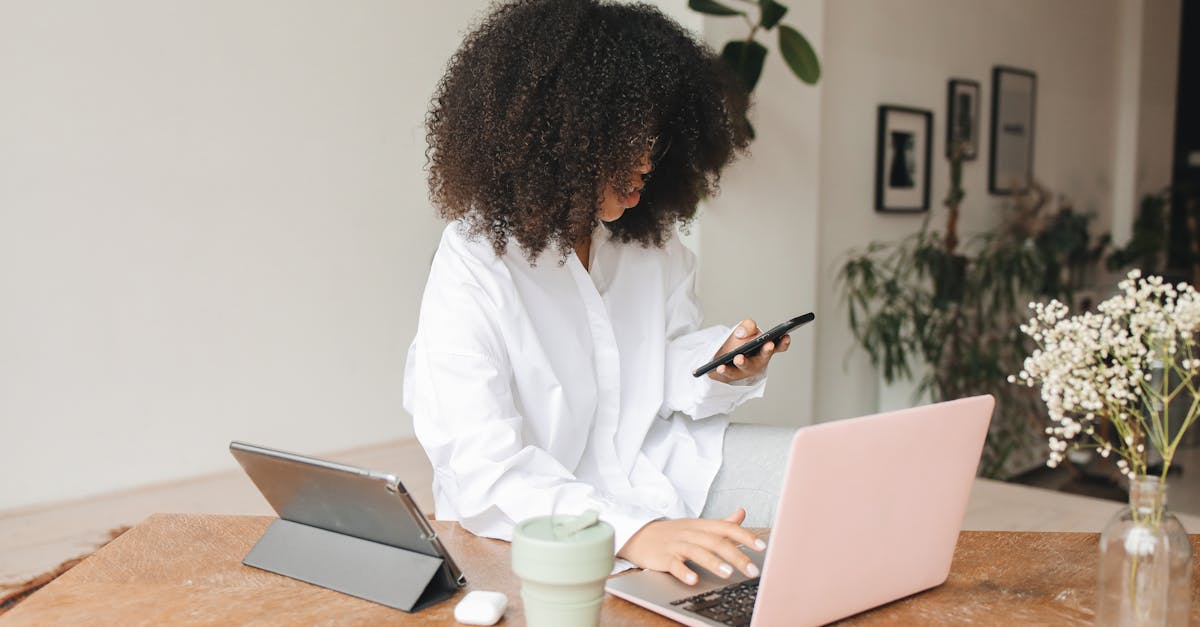
Credit risk modeling plays a crucial role in the finance industry, enabling lenders to analyze the potential risks associated with granting credit to individuals or businesses. Traditionally, credit risk models have been built using statistical methods, but with the advancements in machine learning techniques, lenders now have access to more powerful tools to assess credit risk. One such tool is ChatGPT-4, an AI language model that can assist in building and refining credit risk models using historical data and machine learning algorithms.
Understanding Credit Risk
Credit risk refers to the potential loss a lender may incur if a borrower fails to repay their debt. Lenders need to accurately assess the creditworthiness of borrowers to make informed decisions about lending. This process typically involves analyzing a variety of factors, including the borrower's income, credit history, employment status, and existing financial obligations. Credit risk modeling aims to quantify the probability of default and the potential loss in the event of default.
The Role of Machine Learning
With the proliferation of data and advancements in technology, machine learning has emerged as a valuable tool for credit risk modeling. Machine learning algorithms can analyze large volumes of historical data to identify patterns and relationships that help predict credit risk. These algorithms can consider a wide range of factors and their complex interactions, enabling lenders to develop more accurate and robust credit risk models.
ChatGPT-4: Building and Refining Credit Risk Models
ChatGPT-4, the latest iteration of OpenAI's language model, can assist in the process of building and refining credit risk models. By training the model on historical data, lenders can leverage ChatGPT-4's natural language processing capabilities to extract valuable insights and patterns. The model can understand textual data related to borrowers' financial information, economic indicators, and market trends, helping lenders make data-driven decisions.
Using supervised learning techniques, lenders can provide ChatGPT-4 with labeled examples of credit risk assessments and associated outcomes. The model can learn from this data and generate predictions for new borrowers based on their characteristics. By comparing the model's predictions with actual outcomes, lenders can continuously refine and improve their credit risk models.
The Advantages of ChatGPT-4
One of the key advantages of ChatGPT-4 is its ability to handle unstructured data. Credit risk models often rely on both structured data (e.g., numerical variables) and unstructured data (e.g., text descriptions). ChatGPT-4 excels in understanding and analyzing natural language, enabling lenders to include textual information in their credit risk models effectively.
Additionally, ChatGPT-4 can adapt to changes in the credit landscape. As economic conditions, regulations, and borrower behaviors evolve, lenders need to update their credit risk models accordingly. The flexibility of ChatGPT-4 allows lenders to easily incorporate new information and adapt their models to capture the evolving credit risk landscape.
Conclusion
Credit risk modeling is a vital component of the lending industry. With the advancements in machine learning and the introduction of models like ChatGPT-4, lenders now have a powerful tool to build and refine credit risk models using historical data and machine learning techniques. By leveraging the natural language processing capabilities of ChatGPT-4, lenders can extract valuable insights from textual data and improve the accuracy and robustness of their credit risk assessments. With the ability to handle unstructured data and adapt to changing credit landscapes, ChatGPT-4 is set to revolutionize credit risk modeling.
Comments:
Thank you all for joining the discussion on my article! I appreciate your thoughts and insights.
Great article, Timothy! I've always been interested in how AI can shape credit risk modeling. Do you think ChatGPT can truly revolutionize this area?
Hi Alice! I believe ChatGPT has the potential to revolutionize credit risk modeling. It offers a more interactive and conversational approach to understanding complex credit risk scenarios, making it easier to analyze patterns and make effective predictions.
Interesting concept, Timothy. How do you address concerns about the reliability and accuracy of the models generated by ChatGPT?
Hi Bob! Valid concern. ChatGPT's models undergo rigorous training and testing, which helps ensure reliability and accuracy. However, it's essential to continuously validate and update the models to keep up with changing trends and risks in the credit industry.
I'm curious to know how ChatGPT can handle non-traditional credit data. Can it effectively analyze alternative data sources?
Hi Carol! Absolutely. ChatGPT has the capability to process and analyze a wide range of data types, including non-traditional sources. By incorporating alternative data, we can gain deeper insights into credit risk and make more informed decisions.
The idea of using AI in credit risk modeling is fascinating. However, what about data privacy? How can we ensure that sensitive customer information is protected?
Hi David! Privacy is of utmost importance. When utilizing ChatGPT in credit risk modeling, we adhere to strict data privacy regulations and implement robust security measures to protect sensitive customer information. Confidentiality and compliance are priorities.
I wonder if ChatGPT can account for unforeseen events or black swan events that might impact credit risk models. What are your thoughts?
Hi Elena! Black swan events pose challenges for any credit risk model. While ChatGPT can have difficulty predicting unprecedented events, it can help in quickly analyzing and adapting to emerging scenarios by providing real-time insights and assisting with decision-making.
Do you see ChatGPT completely replacing traditional credit risk models, or is it more of a complementary tool?
Hi Fred! ChatGPT is not intended to replace traditional credit risk models but rather complement them. It offers a new perspective and innovative capabilities that can enhance existing models and help mitigate blind spots. Collaboration between AI and human expertise is the key.
The ethical aspect of AI in credit risk modeling is important. How can we ensure fairness and avoid bias when using ChatGPT?
Hi Grace! Fairness and bias are critical considerations. To address this, we employ rigorous evaluation and testing processes, continually monitor for bias, and take steps to mitigate it. Transparency and accountability are central to ensuring fair credit risk modeling.
It's fascinating to explore the potential of AI in credit risk modeling. Can ChatGPT adapt to ever-changing market conditions effectively?
Hi Henry! ChatGPT's flexibility allows it to adapt to evolving market conditions, making it well-suited for credit risk modeling. By analyzing real-time data and employing continual learning methods, it can provide valuable insights in dynamic market environments.
How do you prevent malicious actors from exploiting ChatGPT's capabilities and using it to manipulate credit risk models?
Hi Isabella! Security is a top concern. We have robust measures in place to prevent misuse and unauthorized access. Regular audits and strict access controls ensure that ChatGPT's capabilities are used ethically and for their intended purposes.
Timothy, what are the potential limitations or challenges when implementing ChatGPT into credit risk technology?
Hi Jack! Implementing ChatGPT into credit risk technology comes with challenges such as model explainability, training data quality, and keeping up with evolving regulatory requirements. Addressing these challenges requires a holistic approach that combines rigorous testing, effective data management, and collaboration with experts.
Could you provide some real-world examples of ChatGPT's application in credit risk modeling?
Hi Kelly! Sure, ChatGPT can be used for applications like credit scoring, fraud detection, and customer sentiment analysis. For instance, it can analyze customer interaction data to detect signals of potential creditworthiness or identify indicators of fraudulent activities.
How does ChatGPT handle data quality issues, such as missing or incomplete data?
Hi Liam! ChatGPT can handle missing or incomplete data to a certain extent. However, it's crucial to ensure data quality and integrity before utilizing it in credit risk modeling. Data preprocessing techniques, such as imputation, can be employed to minimize the impact of missing data.
Timothy, what are some of the key advantages of using ChatGPT in credit risk modeling compared to traditional modeling approaches?
Hi Megan! ChatGPT offers several advantages over traditional modeling approaches. It enables a more interactive and conversational analysis of credit risk scenarios, allowing for better understanding and decision-making. It can also process unstructured data, such as customer chats, which is valuable in gaining comprehensive insights.
What are the considerations when implementing ChatGPT in terms of scalability and computational resources?
Hi Nora! Scalability and computational resources are important considerations. ChatGPT's implementation requires adequate computing power, storage, and memory. Additionally, optimization techniques, like using distributed systems or model compression, can be employed to enhance scalability and efficiency.
Are there any specific industries or sectors where ChatGPT can have a greater impact on credit risk modeling?
Hi Oscar! ChatGPT can have a significant impact in industries where credit risk modeling is crucial, such as banking, lending, insurance, and fintech. The ability to process large amounts of diverse data and provide valuable insights makes it applicable across various sectors.
How do you address concerns about legal and regulatory compliance when using ChatGPT in credit risk technology?
Hi Peter! Legal and regulatory compliance is of utmost importance. When utilizing ChatGPT in credit risk technology, we ensure adherence to relevant laws and regulations governing data usage, privacy, and fair lending practices. Compliance with industry standards and regular audits are essential.
Given the rapidly evolving nature of AI, how do you ensure that ChatGPT remains up to date and relevant in the long term?
Hi Quinn! Continual improvement and adaptation are crucial. To ensure ChatGPT remains up to date, we actively monitor advancements in the AI field, participate in research communities, and engage with industry experts. Regular updates and model refinements help us keep pace with the evolving credit risk landscape.
What are the challenges in obtaining and preparing data for training ChatGPT models in the context of credit risk modeling?
Hi Riley! Obtaining and preparing training data can be challenging. It requires collaboration between domain experts and data scientists to collect and curate relevant and unbiased data. It's crucial to ensure the training data represents the target population and captures diverse credit risk scenarios to build robust models.
Can ChatGPT assist in explaining credit risk models to non-experts, such as customers or regulators?
Hi Sam! ChatGPT can help explain credit risk models to non-experts by providing interactive explanations and answering specific questions. Its conversational nature allows for a more intuitive understanding, making it a valuable tool for communicating complex credit risk concepts to customers, regulators, and other stakeholders.
What are the potential limitations in terms of transparency and interpretability when using ChatGPT in credit risk modeling?
Hi Tina! Transparency and interpretability are indeed challenges. ChatGPT's decision-making process can be less interpretable than traditional models. However, efforts are underway to improve model interpretability, and explainability techniques are being explored to ensure transparency when using ChatGPT in credit risk modeling.
What are some potential future research directions for integrating ChatGPT with credit risk modeling?
Hi Victor! Some future research directions include exploring ways to enhance model explainability, improving interpretability of decision-making, refining domain-specific knowledge integration, and addressing the challenges of real-time model adaptation. These areas of research can further enhance the integration of ChatGPT in credit risk modeling.
How do you validate the performance and accuracy of ChatGPT models in credit risk technology?
Hi Wendy! Validating ChatGPT models involves benchmarking against existing models and evaluating performance measures such as accuracy, precision, recall, and F1 score. Extensive testing is conducted to ensure the reliability and effectiveness of the models in credit risk technology.
What are the collaboration opportunities between researchers, industry experts, and regulators in advancing ChatGPT-based credit risk modeling?
Hi Xavier! Collaboration is crucial for advancing ChatGPT-based credit risk modeling. Researchers, industry experts, and regulators can collaborate in sharing knowledge, identifying challenges, and developing best practices. This collective effort ensures that ChatGPT is effectively integrated into credit risk technology while considering ethical, legal, and regulatory implications.
How does ChatGPT handle interpretability when it comes to regulatory audits and compliance requirements?
Hi Yara! ChatGPT's interpretability is an important consideration in regulatory audits and compliance requirements. Efforts are being made to develop techniques that provide insights into the decision-making process of ChatGPT models, ensuring that they meet the necessary interpretability standards set by regulators.
What steps can organizations take to successfully implement ChatGPT in their credit risk technology?
Hi Zane! Successfully implementing ChatGPT in credit risk technology requires a systematic approach. Organizations should start with defining their goals, secure reliable data sources, collaborate with experts in both AI and credit risk, develop robust model management frameworks, and ensure continual oversight and validation of the models. Clear governance processes and ongoing monitoring are vital to achieving successful integration.