Revolutionizing Credit Scoring: Harnessing ChatGPT for Advanced Data Analysis Technology
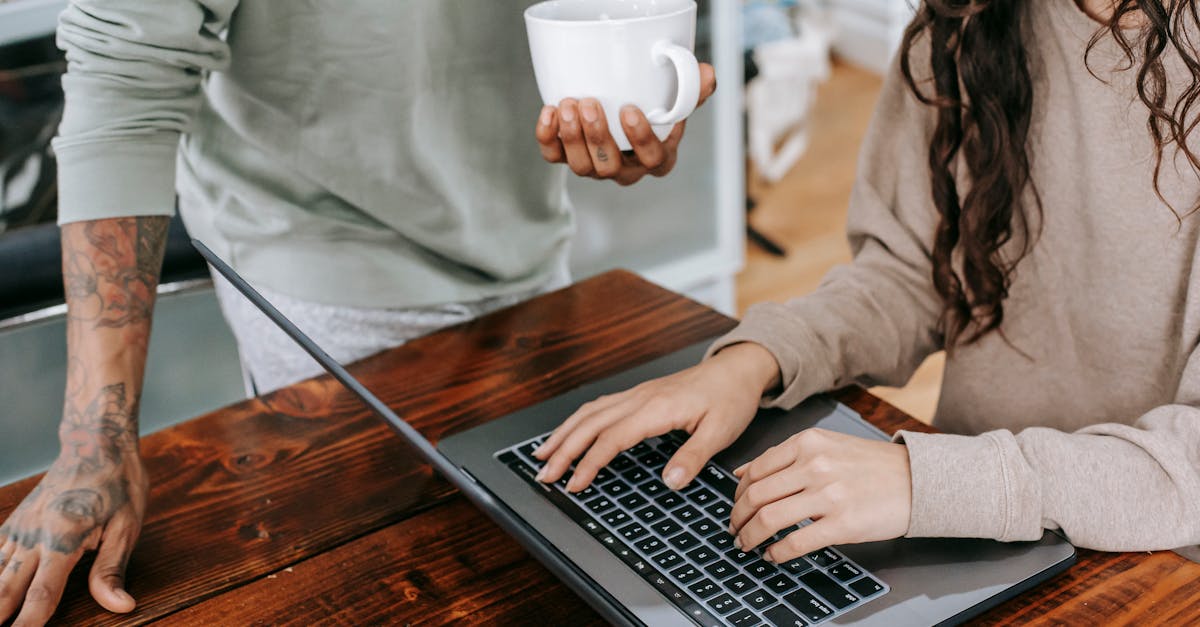
ChatGPT-4, powered by the latest advancements in natural language processing, is revolutionizing the field of credit scoring. With its remarkable ability to predict the probability of default for a given borrowing scenario, ChatGPT-4 offers unprecedented insights into creditworthiness assessment.
The Role of Data Analysis
Data analysis plays a crucial role in credit scoring, enabling lenders to make informed decisions when evaluating the creditworthiness of potential borrowers. Through the utilization of large and diverse datasets, data analysts can uncover patterns, relationships, and risk factors that can help predict the likelihood of borrowers defaulting on their loans.
How ChatGPT-4 Utilizes Data Analysis
Powered by cutting-edge data analysis techniques, ChatGPT-4 has the ability to analyze vast amounts of historical credit data to predict the probability of default for a given borrowing scenario. The model is trained on a wide range of borrower profiles, financial indicators, and economic factors to identify trends and patterns that may indicate a higher risk of default.
Advantages of Using ChatGPT-4
ChatGPT-4 offers several advantages when it comes to credit scoring:
- Accuracy: By leveraging the power of data analysis, ChatGPT-4 can provide highly accurate predictions of default probabilities, enabling lenders to make more informed decisions.
- Efficiency: With its advanced computing capabilities, ChatGPT-4 can perform complex data analysis tasks in a fraction of the time it would take traditional methods.
- Automation: By automating the credit scoring process, ChatGPT-4 eliminates the need for manual data analysis, reducing human errors and ensuring consistency.
- Improved Decision-Making: The insights provided by ChatGPT-4 can help lenders optimize their credit risk management strategies, leading to more effective and profitable lending practices.
The Future of Credit Scoring
The integration of data analysis and artificial intelligence technologies, such as ChatGPT-4, is transforming the credit scoring landscape. As these technologies continue to evolve, we can expect even more accurate and sophisticated credit risk assessment models in the future. Lenders will have access to real-time data and predictive analytics, enabling them to make better-informed lending decisions and reduce the overall risk in their portfolios.
Conclusion
With the advent of ChatGPT-4 and its powerful data analysis capabilities, the credit scoring industry is entering a new era of accuracy, efficiency, and automation. By harnessing the insights provided by this technology, lenders can make better-informed decisions that minimize the risk of default and optimize their lending strategies. The future of credit scoring looks brighter than ever, thanks to the advancements in data analysis and artificial intelligence.
Comments:
Thank you all for taking the time to read my article on revolutionizing credit scoring using ChatGPT for advanced data analysis technology. I'm excited to hear your thoughts and engage in a discussion!
Excellent article, Kerry! It's fascinating to see how AI technology like ChatGPT can be utilized to revolutionize credit scoring. This could bring so much efficiency and accuracy to the process.
Thank you, Mark! I agree, the potential for AI in credit scoring is immense. It can enhance traditional methods by analyzing vast amounts of data and identifying valuable patterns that humans might miss.
I'm a bit concerned about the reliance on AI in credit scoring. What happens if the technology makes mistakes or biases creep in? How can we ensure fairness?
Valid concerns, Emily. Bias in AI is a crucial issue that needs to be addressed. It's crucial to have proper data governance and continuous monitoring to ensure fairness and accountability. Human oversight is also essential to interpret results and challenge potential biases.
I see the advantages AI can bring to credit scoring, but I worry about the potential risks associated with overreliance on technology. What if something goes wrong or the system is hacked?
Great point, Jacob. Ensuring the security of AI systems is crucial. Robust cybersecurity measures, regular audits, and the adoption of best practices can help minimize risks. Additionally, having fallback mechanisms in place, like human expertise and evaluation, is essential to mitigate potential issues.
I love the idea of revolutionizing credit scoring with AI, but what about data privacy? How can we be sure that personal data is adequately protected?
Data privacy is indeed a critical consideration, Sophia. Adhering to data protection regulations, like GDPR, and implementing strict data security measures are vital. Anonymization and encryption techniques can also be utilized to protect personal data while still gaining valuable insights for credit scoring purposes.
ChatGPT sounds promising, but can it truly outperform traditional credit scoring models? What evidence supports its effectiveness?
Great question, Daniel. ChatGPT is still relatively new in the credit scoring domain, but initial studies have shown promising results. While it may not completely replace traditional models, it can augment them by uncovering new insights and improving accuracy. Ongoing research and empirical validation are crucial to further establish its effectiveness.
I'm curious about the interpretability of AI-driven credit scoring models. Can we truly understand how decisions are made and explain them to consumers or regulators?
Interpretability is indeed a challenge with AI models, Emma. Techniques like explainable AI and model-agnostic interpretability can help shed light on decision-making processes. Enhancing transparency and providing understandable explanations are imperative to gain trust from consumers and meet regulatory requirements in the credit scoring domain.
AI-powered credit scoring seems like a double-edged sword. While it brings efficiency and innovation, it may also lead to a dehumanized lending process. How can we strike the right balance?
A valid concern, John. Striking the right balance is crucial to avoid a dehumanized lending process. AI should be seen as a tool to enhance human decision-making, not replace it entirely. Incorporating ethical considerations, continuous human oversight, and involving diverse stakeholders in AI development can help ensure a more balanced approach.
I'm intrigued by the potential benefits of AI in credit scoring, but what about potential job losses in the industry? Could AI lead to fewer employment opportunities for credit scoring professionals?
That's a valid concern, Sarah. While AI may automate certain tasks in credit scoring, it can also create new opportunities. The integration of AI can free up professionals to focus on more complex analysis, customer support, and strategic decision-making. Upskilling and reskilling programs can help professionals adapt and thrive in the evolving landscape.
AI-driven credit scoring may perpetuate existing biases if built on biased historical data. How can we ensure that the models are fair and unbiased?
You raise a crucial point, David. Addressing bias in AI models is essential. By scrutinizing training data, using representative datasets, and applying fairness evaluation metrics, we can work towards more equitable credit scoring models. It's an ongoing journey that requires collaboration between data scientists, domain experts, and regulatory bodies.
I'm excited about the potential of AI in credit scoring, but what about the digital divide? Could reliance on technology exclude certain populations or individuals with limited access to digital resources?
Great point, Melissa. The digital divide is a valid concern. It's important to ensure equitable access to digital resources and alternative methods of assessment for individuals who may be digitally disadvantaged. Employing a hybrid approach that combines AI with traditional methods can help mitigate exclusion and ensure fairness.
I have reservations about relying heavily on AI for credit scoring. Human judgment and empathy are crucial in assessing financial situations. Can AI truly understand complex individual circumstances?
Valid concerns, Brian. While AI can analyze vast amounts of data and identify patterns, it may struggle to fully comprehend nuanced circumstances that require human empathy. That's why I believe a hybrid approach, combining AI's analytical power with human judgment, is key to achieve a more comprehensive and balanced credit scoring process.
AI-powered credit scoring certainly has its benefits, but I worry about potential algorithmic biases. How can we ensure that AI doesn't discriminate against certain groups of individuals?
You're absolutely right, Olivia. Addressing algorithmic biases is crucial. By carefully selecting training data, monitoring and evaluating model outputs regularly, and fostering diversity and inclusivity in AI development, we can work towards more equitable credit scoring systems that minimize discrimination and promote fairness.
AI-driven credit scoring could potentially benefit small businesses and startups. Traditional scoring methods often overlook their potential. Do you think AI can help level the playing field?
Absolutely, Michelle. AI can analyze a wide range of data sources and provide more comprehensive insights into the creditworthiness of small businesses and startups. By considering non-traditional variables and patterns, it can help level the playing field and provide better access to credit opportunities for these entities.
I'm concerned about AI impacting customer trust. Many already have reservations about sharing personal data. Won't reliance on AI for credit scoring further increase privacy concerns?
Valid concern, Hannah. Building and maintaining customer trust is vital. Transparency in how personal data is collected, used, and protected is crucial. Clear communication and educating customers about the benefits and safeguards around AI, along with robust data protection practices, can help alleviate privacy concerns and enhance trust.
What about potential regulatory challenges in implementing AI-driven credit scoring? Do you think existing regulations are sufficient, or is there a need for new frameworks?
Regulatory challenges are indeed a key consideration, Andrew. Existing regulations may need to evolve to address the unique aspects of AI-driven credit scoring adequately. Collaboration between industry stakeholders, policymakers, and regulatory bodies is necessary to establish appropriate frameworks that balance innovation, consumer protection, and responsible use of AI in the credit scoring domain.
AI-driven credit scoring sounds promising, but what about outliers or uncommon situations that may not fit within predefined models? Can AI adapt to handle such cases effectively?
Good question, Alex. Outliers and uncommon situations can indeed pose challenges. While AI is powerful in uncovering patterns, it may struggle with extreme cases or unique scenarios. However, continuous learning and updating of models can help the system adapt and improve its handling of such cases over time.
AI-powered credit scoring may improve efficiency, but could it lead to a reduction in personalized interactions between lenders and borrowers? Building relationships and understanding individual needs are important in lending decisions.
You make a valid point, Sophie. Personalized interactions and understanding individual needs are valuable aspects of lending decisions. AI should be seen as a complement rather than a replacement for such interactions. By automating routine tasks, AI can free up time for lenders to focus on building relationships and providing personalized support to borrowers.
What about the cost of implementing AI-driven credit scoring? Will it be affordable for small or regional lenders?
Affordability is a valid concern, Jason. While AI implementation costs can vary, there are opportunities for collaboration, cloud-based solutions, and scalability that can make it more accessible to small or regional lenders. Additionally, as AI adoption continues to grow, the costs are likely to decrease over time.
AI-driven credit scoring sounds promising, but what about the transparency of the models? How can we ensure that lenders and borrowers understand the criteria used and trust the results?
Transparency is crucial, Sophia. Lenders have a responsibility to explain the criteria used in credit scoring models. Embracing explainable AI techniques can help shed light on the decision-making process and build trust. Clear communication, transparency reports, and accessible information can empower both lenders and borrowers to understand and trust the results.
AI-driven credit scoring could lead to more consistent and standardized evaluations. However, should we be wary of sacrificing flexibility and adaptability in the pursuit of consistency?
Valid concern, Jacob. Striking the right balance between consistency and flexibility is essential. While AI can bring standardization, it's crucial to have room for adaptability and human judgment. Continuous monitoring, periodic model updates, and incorporating feedback loops can help achieve a balance that preserves both consistency and the ability to adapt to changing circumstances.
AI can certainly expedite credit scoring processes, but what about the potential for fraud? How can we ensure that AI-powered systems aren't manipulated by malicious actors?
Great point, Gabriel. Preventing fraud is crucial. Implementing robust fraud detection mechanisms, continuous monitoring, and access controls can help minimize the risk of manipulation. Regular audits and the adoption of secure AI development practices are also essential to establish trust and reduce the potential for fraudulent activities.
AI-powered credit scoring could benefit lenders, but what about borrowers? How can we ensure that AI doesn't lead to predatory lending practices or exclusion of certain communities?
You raise a critical concern, Isabella. A responsible and ethical approach to AI-powered credit scoring is paramount. Regulatory frameworks, industry best practices, and ongoing monitoring can help mitigate the risk of predatory lending. Additionally, diversity in AI development teams and engaging communities can help ensure fair and inclusive credit access.
AI-driven credit scoring certainly offers potential, but how can we ensure that the models are continuously updated to adapt to evolving economic and social contexts?
Excellent point, Liam. Continuous model updates are crucial to keep pace with evolving economic and social contexts. Monitoring key performance metrics, incorporating new data sources, and considering stakeholder feedback can help ensure the models remain relevant and effective in a changing landscape.
I'm excited about the potential of AI in credit scoring, but what are the key challenges in implementing AI on a large scale across the industry?
Good question, Lucas. Some key challenges in large-scale AI implementation include data quality and availability, organizational readiness, governance frameworks, and regulatory compliance. Collaboration between industry stakeholders, rigorous testing, and phased rollouts can help overcome these challenges and ensure successful adoption at scale.
Thank you all for your insightful comments and questions! It has been a pleasure discussing the potential and challenges of revolutionizing credit scoring with AI. Your engagement is much appreciated!