Revolutionizing Customer Lifetime Value Analysis: Leveraging ChatGPT for Revenue Analysis
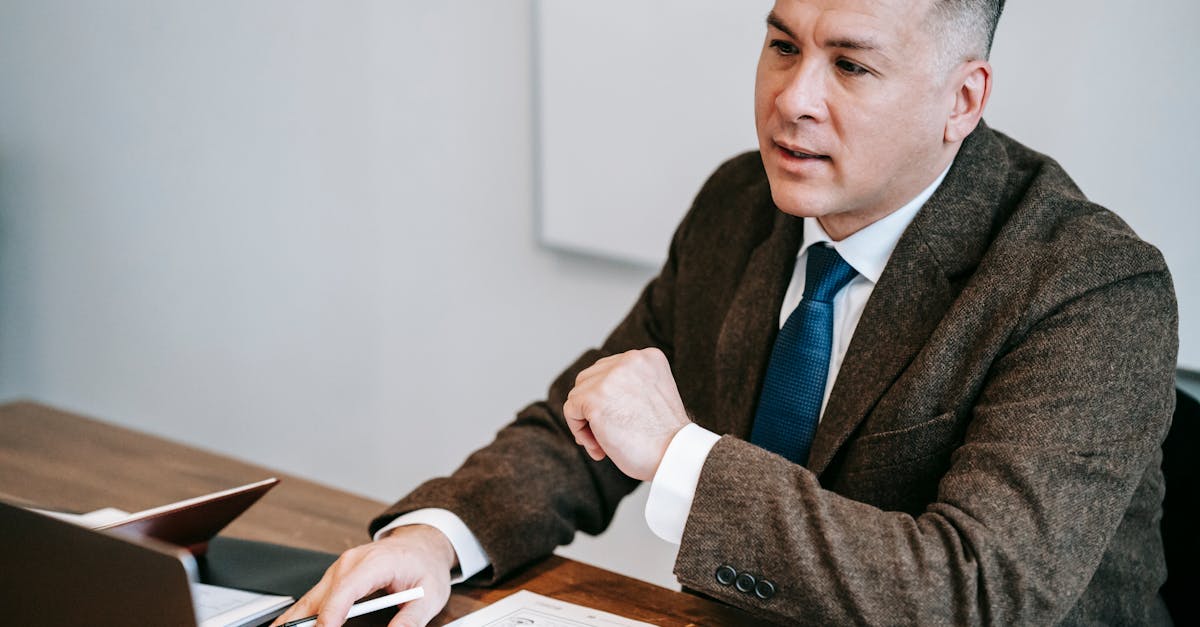
Introduction
Technology and data analysis have become integral parts of modern business strategies. One such analysis that has gained prominence in recent years is the Customer Lifetime Value (CLV) analysis. With the advent of advanced language models like ChatGPT-4, revenue analysis has become more streamlined and effective.
What is Customer Lifetime Value (CLV)?
Customer Lifetime Value, or CLV, is a metric used by businesses to assess the worth of a customer throughout their relationship with the company. It calculates the predicted revenue generated by a customer over their entire lifetime by considering various factors such as purchase history, average order value, frequency of purchases, customer behavior, and more.
Utilizing ChatGPT-4 for Revenue Analysis
ChatGPT-4, a state-of-the-art language model powered by artificial intelligence, can play a significant role in revenue analysis by accurately calculating CLV. By leveraging its capability to analyze large amounts of customer data, ChatGPT-4 can provide valuable insights to businesses.
Analyzing Customer Behavior
One of the key aspects of revenue analysis is understanding customer behavior patterns. ChatGPT-4 can process and analyze vast datasets, including customer interactions, purchase history, feedback, and browsing behavior. By extrapolating trends and identifying patterns, the model can predict future behavior and optimize revenue-generating opportunities.
Predicting Revenue Potential
Using the insights gained from customer behavior analysis, ChatGPT-4 can accurately forecast the potential revenue a customer might generate over their lifetime. This prediction enables businesses to allocate resources effectively, tailor marketing strategies, and prioritize customer engagement activities for maximum profitability.
Optimizing Resource Allocation
With the help of ChatGPT-4, companies can optimize their resource allocation strategies. By identifying high-value customers with substantial revenue potential, businesses can focus their attention on retaining and nurturing these valuable relationships. Additionally, the model can identify areas where resources may be wasted, allowing for efficient cost management.
Conclusion
Customer Lifetime Value analysis has become an essential component of revenue analysis for businesses in various industries. Leveraging advanced language models like ChatGPT-4 provides companies with accurate predictions and valuable insights into customer behavior and revenue potential. By utilizing these insights, companies can develop effective strategies to maximize CLV and drive sustainable growth.
Comments:
Great article, Hitesh! Leveraging ChatGPT for revenue analysis is indeed a revolutionary approach. It seems like a promising technology for businesses to improve customer lifetime value analysis. Can you share any specific use cases or success stories?
I agree with Hannah. This article is very insightful, and I'm particularly interested in learning about practical applications. Hitesh, could you provide some guidance on implementing this approach within different industries?
Hi Michael! I can give you an example of implementing ChatGPT in the e-commerce industry. By analyzing customer chat logs, it helps in personalizing product recommendations, improving customer support, and increasing overall sales. It's quite versatile!
Thanks, Sarah! That example showcases the potential impact of ChatGPT in e-commerce. It's fascinating how it can optimize multiple aspects of the customer experience using natural language processing.
Thanks for this article, Hitesh! Leveraging AI for revenue analysis is indeed a game-changer. Does ChatGPT help in identifying patterns or trends in customer behavior that were previously difficult to uncover?
Hello Emma! Yes, ChatGPT can identify patterns and trends in customer behavior by analyzing a large volume of chat data. It can unveil insights that were previously challenging to uncover manually. This enables businesses to make data-driven decisions to enhance customer lifetime value.
Thanks for offering more insights, Hitesh! I would love to learn more about how ChatGPT can optimize pricing strategies. Are there any notable case studies you can share?
Certainly, Hannah! In one case study, a company used ChatGPT to optimize their pricing strategy by analyzing competitor chat data. This allowed them to identify price-sensitive customer segments and adjust their pricing to increase profits without compromising customer satisfaction.
Thanks for sharing the case study, Hitesh! Optimizing pricing strategies through chat analysis sounds promising. It seems like ChatGPT can extract valuable insights from unstructured data and contribute to business growth.
Hitesh, the case study you shared about optimizing pricing strategies sounds intriguing. Do you have any insights into the potential impact on customer satisfaction when using ChatGPT for pricing adjustments?
Emma, adjusting pricing by leveraging ChatGPT can have positive impacts on customer satisfaction. By analyzing chat data, businesses can understand customer preferences, willingness to pay, and price sensitivities. This enables them to align their pricing with customer expectations, striking a balance between profitability and customer satisfaction.
Thank you, Hannah and Michael, for your kind words! Absolutely, there are several use cases. One example is optimizing pricing strategies by analyzing customer conversations. Another application is predicting customer churn based on chat history. I can provide more details if you're interested!
This article raises an important point about using AI in revenue analysis. Hitesh, can you discuss any potential limitations or challenges businesses might face while implementing ChatGPT for customer lifetime value analysis?
Certainly, Alex! While ChatGPT is powerful, it does have a few limitations. It might generate responses that are plausible but incorrect, and it heavily relies on the quality and representativeness of training data. Validating generated insights is crucial to avoid misleading conclusions.
Hitesh, great article! Do you think ChatGPT could also be applied to analyze customer sentiment and preferences? Would it enable businesses to tailor their offerings accordingly?
Absolutely, David! ChatGPT can analyze customer sentiment and preferences by processing chat data. This enables businesses to gain a deeper understanding of their customers and tailor their offerings to meet their expectations. It's a valuable tool for personalized marketing.
Hitesh, this article got me really interested in the topic. Is ChatGPT only applicable to analyzing chat data, or can it also be used with other customer data sources like surveys or social media?
Good question, Julia! ChatGPT can indeed be used with other customer data sources like surveys or social media. By incorporating diverse data, businesses can gain a holistic understanding of customer behavior, sentiment, and preferences, resulting in better-informed decision-making.
Great article, Hitesh! I believe implementing ChatGPT for revenue analysis will give companies a competitive advantage by extracting valuable insights. Are there any specific industries where this approach has already shown remarkable results?
Certainly, Sophia! Many industries have witnessed remarkable results with ChatGPT. Retail, e-commerce, and customer support are some of the sectors where this approach has been successfully utilized to enhance revenue analysis and customer engagement.
Moreover, human involvement is critical to avoid bias or misinformation from the AI-generated insights. Businesses need to ensure that the AI models are trained on diverse and representative data to mitigate potential issues.
Hitesh, your article provides valuable insights into leveraging AI for revenue analysis. In terms of implementation, what data requirements are necessary? Are there any specific data preparation steps to consider before applying ChatGPT?
Thank you, Oliver! To implement ChatGPT, a large corpus of chat data is required to train the model effectively. The data should be diverse and representative of the target customer base. Additionally, preprocessing steps like cleaning and anonymization are usually carried out to protect privacy and ensure data quality.
Hitesh, I find this article very intriguing. Can you give some guidance on the computational resources or infrastructure needed to deploy ChatGPT effectively for revenue analysis?
Hi Emily! Deploying ChatGPT effectively requires substantial computational resources. Training the model involves using powerful GPUs or even distributed computing setups. For smaller-scale deployments, cloud-based AI platforms can be a practical choice.
Hitesh, your article highlights the potential of AI in revenue analysis. I'm curious, what are the key steps involved in implementing ChatGPT for customer lifetime value analysis? Are there any best practices to follow?
Hello Daniel! Implementing ChatGPT for customer lifetime value analysis involves several steps. Firstly, gathering and preprocessing relevant chat data. Then, training the model on this data to generate valuable insights. Finally, integrating these insights into existing revenue analysis frameworks. As for best practices, it's crucial to validate the generated insights, involve domain experts, and align the analysis with business objectives.
Good point, Hitesh! Retail and customer support seem like obvious use cases for ChatGPT in revenue analysis. Are there any surprising or non-conventional industries that can also benefit from this approach?
Sophia, absolutely! Surprising industries that can benefit from ChatGPT in revenue analysis include hospitality, travel, and even healthcare. In these sectors, analyzing chat data can uncover valuable insights about customer preferences, satisfaction levels, and pain points.
Hitesh, great article! Are there any privacy concerns associated with analyzing customer chat data using ChatGPT? How are businesses addressing these concerns while leveraging this technology for revenue analysis?
Jason, privacy is a crucial aspect while analyzing customer chat data. Businesses must ensure that customer privacy is protected and comply with data protection regulations. Anonymization techniques can be applied to remove personally identifiable information, and access to sensitive data should be strictly controlled.
Great article, Hitesh! I'm curious about the scalability of using ChatGPT for revenue analysis. Can it handle large volumes of chat data efficiently?
Hitesh, this article is enlightening! Could you elaborate on the benefits of incorporating ChatGPT into traditional revenue analysis methods? How can it complement or enhance existing approaches?
Hi Lucas! Incorporating ChatGPT into traditional revenue analysis methods brings several benefits. It can provide new insights by analyzing unstructured chat data that was previously challenging to process. This enhances the quality and completeness of revenue analysis, enabling businesses to make more informed decisions about customer segmentation, pricing, and customer satisfaction.
Hitesh, this article is thought-provoking! Is there any recommended approach to validate the accuracy of insights generated by ChatGPT during customer lifetime value analysis?
Validating the accuracy of insights generated by ChatGPT is crucial, Melissa. Several approaches can be employed, such as comparing the generated insights with domain knowledge, involving human experts in the validation process, and conducting A/B testing to evaluate the impact of AI-generated recommendations or strategies.
Hitesh, since ChatGPT relies on training data, how do businesses handle biases that may exist in the training data? Is there a risk of perpetuating existing biases or extracting biased insights?
Sophia, I believe education could also be a surprising industry that can leverage ChatGPT for revenue analysis. Analyzing chat data from online learning platforms could provide insights into student engagement, preferences, and learning outcomes. It could help design personalized educational experiences based on individual needs.
Incorporating a technology like ChatGPT into traditional revenue analysis can lead to a more holistic understanding of customer behavior. By gaining insights from chat data, businesses can make data-driven decisions that align with customers' needs and preferences. It complements existing approaches by adding a new dimension to the analysis.
It's interesting how computational resources and infrastructure play a vital role in effectively deploying ChatGPT. Can cloud-based AI platforms handle the scale and complexity of revenue analysis using ChatGPT?
Hitesh, fascinating article! What steps should businesses take to ensure ethical use of ChatGPT and prevent any unintended consequences related to revenue analysis?
Thank you, Andrew! Ethical use of ChatGPT is essential. Businesses should establish clear guidelines for AI-generated insights and ensure transparency in their analysis methods. It's important to monitor and address any unintended consequences, such as biased outcomes or reliance on AI-generated insights without human oversight. Regular audits and involving ethics experts can help in promoting responsible usage.
Hitesh, this article is insightful! Can ChatGPT be integrated into real-time systems for revenue analysis? How quickly can the generated insights be made available to businesses for decision-making?
Hi Sophie! ChatGPT can indeed be integrated into real-time systems for revenue analysis. The speed at which generated insights are available depends on the complexity of the analysis and the computational resources deployed. With efficient infrastructure, it's possible to make the insights available in near real-time, enabling businesses to respond quickly to changing customer dynamics.
To ensure ethical usage of AI-generated insights, what strategies are recommended for businesses to communicate the limitations and potential risks associated with ChatGPT deployments for revenue analysis?
Lucy, communication is key when highlighting the limitations and potential risks. Businesses should clearly communicate the uncertainties associated with AI-generated insights, emphasize the need for human validation and decision-making, and address the potential biases or inaccuracies that might arise. Being transparent about the technology's capabilities and limitations fosters trust and informed decision-making.
Hitesh, you mentioned analyzing customer conversations for predicting churn. How accurate is ChatGPT in churn prediction, and what strategies can businesses adopt to leverage this capability?
Lucas, ChatGPT can provide valuable insights for churn prediction by analyzing customer conversations. However, it's important to note that accuracy depends on various factors like the quality and representativeness of training data, as well as domain-specific factors. To leverage this capability effectively, businesses can implement proactive customer retention strategies based on insights from customer conversations, such as personalized offers or targeted interventions.
Lucas, in terms of leveraging ChatGPT for churn prediction, it is essential to consider a combination of predictors, such as chat sentiment analysis, chat frequency, and specific keywords or patterns in chat conversations. By incorporating a multi-dimensional approach, businesses can enhance the accuracy of churn predictions and take proactive measures to retain valuable customers.
Hitesh, thank you for providing comprehensive insights into leveraging ChatGPT for revenue analysis. What key considerations should businesses keep in mind while evaluating the cost-benefit analysis of implementing this approach?
You're welcome, Hannah! When evaluating the cost-benefit analysis of implementing ChatGPT for revenue analysis, businesses should consider factors like the availability and quality of training data, the required computational resources, and the potential return on investment. It's crucial to assess whether the insights gained from ChatGPT justify the associated costs and align with the organization's revenue goals and long-term strategy.