Revolutionizing Data Analysis: Leveraging ChatGPT for SFR Technology
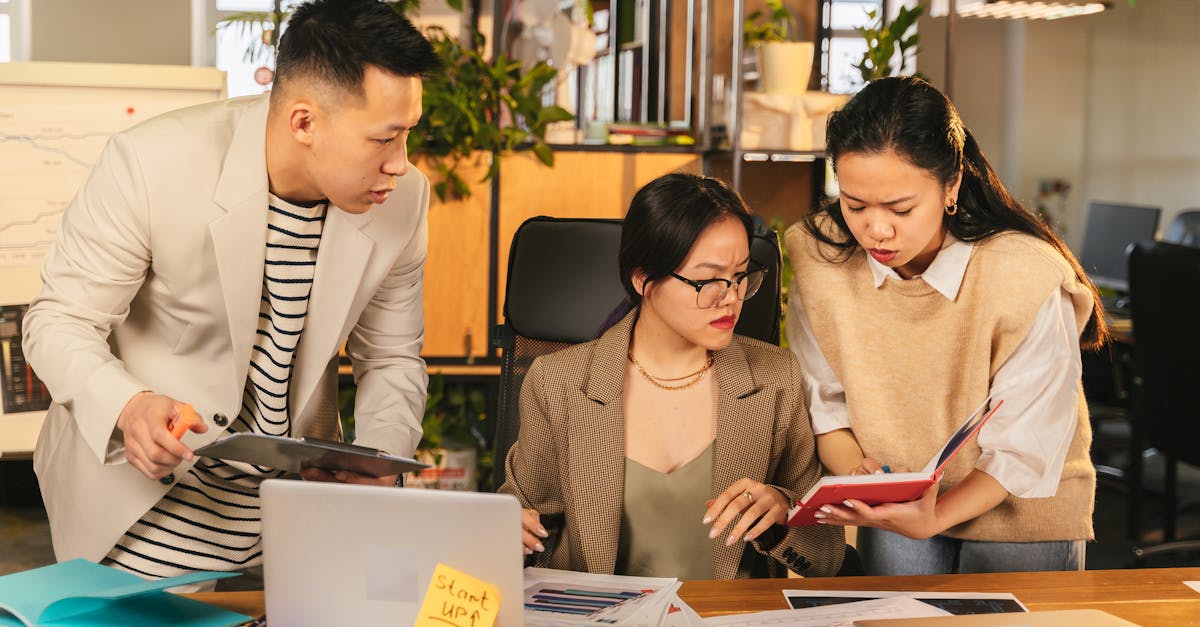
Introduction
SFR, which stands for Statistical Function for R, is a powerful technology used in the field of data analysis. It assists in analyzing data sets, interpreting statistical analyses, and summarizing data reports. With its robust features and capabilities, SFR is a valuable tool for professionals working with large amounts of data.
How does SFR work?
SFR is built upon the R programming language, a widely-used language for statistical computing and graphics. It leverages R's extensive package ecosystem, allowing users to access numerous statistical functions and visualization tools. By combining these packages with its own functionalities, SFR provides a comprehensive solution for data analysis tasks.
Key Features of SFR
- Data Set Analysis: SFR enables users to explore data sets by calculating descriptive statistics such as mean, median, standard deviation, and variance. These measures provide insights into the central tendencies and variabilities of the data.
- Statistical Analysis: SFR facilitates a wide range of statistical analyses, including hypothesis testing, analysis of variance (ANOVA), regression analysis, and t-tests. These analyses help researchers draw meaningful conclusions and make data-driven decisions.
- Data Summarization: SFR's summarization capabilities allow users to generate summary reports, visualizations, and data dashboards. These summaries provide concise representations of complex data, making it easier to communicate insights to stakeholders.
- Integration with Other Tools: SFR seamlessly integrates with other technologies commonly used in data analysis, such as database management systems and visualization tools. This integration enables efficient data processing and enhances collaboration among team members working on different aspects of the analysis.
Benefits of SFR in Data Analysis
SFR offers several benefits for professionals engaged in data analysis:
- Efficiency: SFR automates repetitive data analysis tasks, saving time and effort for analysts. Its extensive library of functions eliminates the need for manual calculations, allowing analysts to focus on interpreting results and drawing meaningful insights.
- Accuracy: SFR follows standardized statistical methodologies, ensuring accuracy and reliability in data analysis. By minimizing human errors and biases, it enhances the credibility of research findings and business decisions.
- Scalability: SFR is designed to handle large and complex data sets. Its optimized algorithms and parallel processing techniques enable efficient analysis of massive volumes of data, making it suitable for big data projects.
- Flexibility: SFR provides a wide range of functions and algorithms to handle different types of data analysis tasks. It supports various statistical models, allowing analysts to adapt their approaches based on the specific characteristics of the data and research objectives.
- Reproducibility: SFR allows analysts to document their analysis workflows and share them with others. This promotes transparency and reproducibility in research, enabling others to validate and build upon previous analyses.
Conclusion
SFR is a valuable technology in the field of data analysis. Its powerful features, including data set analysis, statistical analysis, and data summarization, make it an essential tool for professionals working with data. By leveraging the capabilities of SFR, analysts can gain meaningful insights, make informed decisions, and communicate their findings more effectively. Whether it's in research, business, or any other data-driven domain, SFR provides the necessary tools to unlock the potential of data.
Comments:
Thank you all for your interest in my blog article on Revolutionizing Data Analysis. I'm excited to discuss the advancements in SFR technology with you!
Great article, Neil! The use of ChatGPT for data analysis is indeed a game-changer. It has significantly enhanced our team's efficiency, allowing us to process and analyze large datasets with ease.
I agree, Rachel. ChatGPT's natural language processing capabilities have made data analysis more accessible for non-technical users as well. It's impressive to see how it simplifies complex tasks.
Absolutely, Eric! It's a powerful tool for democratizing data analysis. Companies can now empower employees from various departments to gain insights and make data-driven decisions.
I'm intrigued by the potential of ChatGPT for SFR technology. Neil, can you elaborate more on how it can revolutionize data analysis specifically in the SFR domain?
Of course, Tom! In the SFR (Structured Financial Reporting) domain, ChatGPT can streamline the analysis of complex financial data. It can assist in tasks like identifying patterns, detecting anomalies, and generating financial reports in a more efficient, accurate, and timely manner.
That sounds incredibly promising, Neil. The potential time savings and improved accuracy in financial reporting would be invaluable for organizations. Are there any limitations or challenges that we should be aware of when using ChatGPT in SFR analysis?
Great question, Tom! One of the challenges with ChatGPT is ensuring that it understands and contextualizes financial jargon accurately. It requires careful training and fine-tuning to handle the nuances of the SFR domain effectively.
Thank you for clarifying, Neil. That's an important consideration when implementing ChatGPT in financial analysis. It seems like the benefits outweigh the challenges as long as we invest in proper training and validation.
I'm curious about the potential security implications of using ChatGPT for data analysis. Neil, could you share any insights on how organizations can address data privacy concerns when leveraging ChatGPT?
Certainly, Linda. When using ChatGPT or any AI technology, organizations need to ensure proper data anonymization and implement secure access controls. Additionally, rigorous testing and auditing should be conducted to maintain compliance with data privacy regulations.
Thank you for addressing my concern, Neil. Data privacy is crucial, especially when dealing with sensitive information. It's reassuring to know that there are measures to mitigate the associated risks.
Neil, I'm interested in the scalability of using ChatGPT for SFR analysis. Have you encountered any limitations in terms of handling large volumes of data?
That's a great point, Mark. While ChatGPT can handle large volumes of data, it's crucial to ensure sufficient computational resources to process the information efficiently. The hardware infrastructure needs to be appropriately provisioned to maintain smooth performance.
Hi, Neil! I'm impressed with the potential of ChatGPT. However, what would you say to those who argue that relying on AI for data analysis may diminish human intuition and critical thinking?
Hello, Ruth! It's a valid concern. While AI can enhance efficiency and accuracy, human intuition and critical thinking remain essential. AI tools like ChatGPT should be seen as complementary, aiding humans in gaining insights from data rather than replacing their cognitive abilities.
Neil, I'm curious about the future potential of ChatGPT and SFR technology. Are there any upcoming advancements or features we can look forward to?
Great question, Jamie! The field of natural language processing is evolving rapidly, and we can expect continuous improvements in ChatGPT's ability to handle domain-specific jargon, understand complex queries, and provide more accurate and nuanced responses. Ongoing research will drive its advancements!
Neil, do you foresee any challenges in implementing ChatGPT for organizations with limited technical expertise or resources?
That's a valid concern, David. Implementing ChatGPT can be challenging for organizations without extensive technical expertise. However, as the technology matures, we can expect the development of user-friendly interfaces and pre-trained models that make it more accessible for non-experts.
Neil, I found your article informative. Could you recommend any additional resources or research papers to dive deeper into the topic?
Absolutely, Michael! I recommend exploring research papers on transformer architectures, natural language processing, and applications of AI in finance. Some notable papers include 'Attention Is All You Need' by Vaswani et al. and 'BERT: Pre-training of Deep Bidirectional Transformers for Language Understanding' by Devlin et al.
Neil, can you share any real-world examples or case studies where ChatGPT has been successfully applied to SFR analysis?
Certainly, Karen! One notable example is a financial institution that used ChatGPT to automate the identification of outliers in financial reports. By leveraging the model's natural language processing capabilities, they achieved greater accuracy and efficiency in detecting irregularities.
Neil, how can organizations ensure the reliability and interpretability of ChatGPT's outputs in SFR analysis?
Great question, Emily! It's crucial to implement robust validation processes and sanity checks to ensure the outputs align with expected results. Additionally, organizations should document and explain the decision-making processes involved in using ChatGPT for SFR analysis, providing transparency and improving interpretability.
Thank you for the clarification, Neil. Reliable and interpretable outputs are essential, especially in financial analysis. Trusting the AI's decisions is crucial for organizations to make informed decisions based on the analysis.
Neil, as AI continues to advance, what potential ethical considerations should organizations keep in mind when implementing ChatGPT for data analysis?
Ethical considerations are paramount, Samantha. Organizations must ensure fairness, avoid biases in training data, and regularly assess the performance of AI systems to prevent unintended consequences. Transparency, accountability, and privacy should be at the forefront of AI implementation.
Neil, I'm curious if there are any specific industries that have particularly benefited from leveraging ChatGPT for SFR analysis?
Certainly, Benjamin! The banking and financial services industry, insurance sector, and regulatory bodies such as government agencies have shown significant interest and benefit from using ChatGPT for SFR analysis. However, the potential applications span across industries that deal with structured financial reporting.
Neil, how do you see the future of ChatGPT in the context of the overall data analytics landscape?
Great question, Julia! ChatGPT demonstrates the potential of natural language processing in data analytics. As the technology evolves, we can expect more sophisticated and specialized models that not only assist humans in analysis but automate complex data tasks, enabling deeper insights and informed decision-making.
Neil, could you briefly explain the difference between ChatGPT and traditional analytical tools like business intelligence platforms?
Certainly, Grace! Traditional analytical tools like business intelligence platforms focus on processing structured data through predefined queries and visualizations. On the other hand, ChatGPT operates on unstructured and semi-structured data, providing a conversational interface that allows users to ask questions and receive responses in natural language, enhancing the exploration and understanding of data.
Neil, what are some key considerations organizations should keep in mind when integrating ChatGPT into existing data analysis workflows?
Great question, Oliver! Organizations should conduct a thorough analysis of their data analysis workflows, identify suitable use cases for ChatGPT, and ensure proper integration with existing systems. They should also provide user training and monitor its impact on overall productivity and data quality.
Neil, what kind of data preprocessing or data cleaning steps are typically required before leveraging ChatGPT for SFR analysis?
Good question, Sophia! Data preprocessing and cleaning play a vital role in ensuring accurate analysis. Common steps include removing irrelevant or duplicated data, handling missing values, and standardizing formats. Additionally, domain-specific preprocessing steps can help tailor ChatGPT to handle the intricacies of SFR analysis more effectively.
Thank you all once again for participating in this discussion. Your questions and insights have enriched the conversation around leveraging ChatGPT for SFR technology. Feel free to reach out if you have any more questions or ideas!