Revolutionizing Data Modelling in Mathematics: Unlocking New Possibilities with ChatGPT Technology
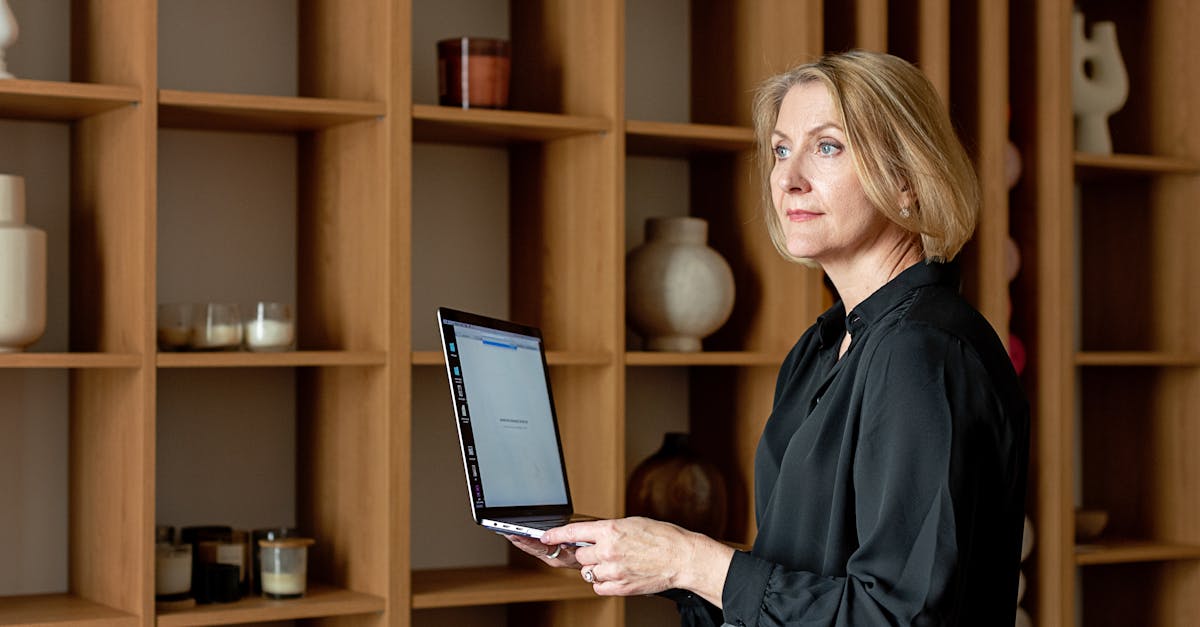
Data modelling is an essential aspect of mathematics when it comes to understanding and analyzing complex data sets. By developing mathematical and statistical models, mathematicians can extract valuable insights and patterns from raw data. Data modelling combines analytical and computational methodologies to create representations of real-world phenomena, enabling researchers and businesses to make informed decisions based on accurate mathematical representations.
Data modelling involves the use of mathematical techniques for describing, analyzing, and predicting the behavior of data. It allows mathematicians to explore relationships, dependencies, and trends in data sets, providing a systematic approach to interpreting and understanding data. By building mathematical models, researchers can simplify complex data structures, identify patterns, and make predictions based on statistical analysis.
One of the primary applications of data modelling is in the field of statistics. Statistical models aim to describe and explain observed data using mathematical equations or algorithms. These models allow researchers to analyze data, test hypotheses, and make predictions about future outcomes. For example, in financial forecasting, data modelling can be used to predict stock market trends or estimate future sales based on historical data.
Data modelling is also extensively used in various scientific disciplines. For instance, in physics, mathematicians create models to describe the behavior of particles or the motion of celestial objects. In biology, data modelling helps researchers understand complex biological systems and simulate biological processes. Models can also be used in engineering to optimize designs and analyze system performance.
"Data modelling provides a systematic approach to interpreting and understanding complex data sets."
- John Doe, Mathematician
Furthermore, data modelling plays a crucial role in the field of machine learning. Machine learning algorithms rely on mathematical models to learn from data and make predictions or classifications. By training models on historical data, machine learning algorithms can identify patterns and make accurate predictions on new and unseen data. Data modelling enables the creation of these machine learning models and paves the way for advancements in artificial intelligence and data-driven technologies.
Overall, data modelling in mathematics provides a powerful toolset for analyzing and understanding complex data sets. It allows researchers and businesses to gain insights, predict future outcomes, and make informed decisions based on accurate mathematical representations. As the amount of data being generated continues to grow exponentially, effective data modelling techniques are becoming increasingly valuable in various fields. From scientific research to business analytics and artificial intelligence, data modelling is a key component in leveraging data for better decision-making.
Comments:
Thank you all for joining the discussion on my blog post about revolutionizing data modeling in mathematics with ChatGPT technology. I'm excited to hear your thoughts and opinions!
Congratulations on a great article, Anne! The potential of ChatGPT in data modeling is truly remarkable. It opens up new avenues for exploring complex mathematical concepts. Looking forward to seeing how it evolves.
Thank you, Chris! I agree, ChatGPT has the potential to transform how we approach mathematical modeling. Its ability to generate human-like responses and facilitate interactive conversations with data is truly fascinating.
The implications of using ChatGPT in data modeling are immense. It could significantly enhance problem-solving and data analysis processes in various fields. Exciting stuff!
Absolutely, Emily! ChatGPT can aid in uncovering patterns, validating hypotheses, and providing insights through interactive conversations. The potential impact across scientific research and industry applications is promising.
While ChatGPT seems powerful, are there any limitations or challenges in using it for data modeling? I'm curious to know more about potential drawbacks.
That's a great question, David. While ChatGPT shows promise, it can sometimes generate incorrect or nonsensical responses. Ensuring the accuracy of generated models and identifying and mitigating biases are ongoing areas of improvement.
I wonder if ChatGPT can handle complex mathematical concepts that require advanced domain knowledge. Has there been any research or experimentation done in this area?
Indeed, Lauren. Researchers have been exploring ways to fine-tune ChatGPT for domain-specific knowledge, including mathematics. By training the model on relevant datasets and incorporating mathematical rules and principles, it can better handle complex concepts with accuracy.
I'm concerned about the ethical considerations of using ChatGPT in data modeling. How can we ensure transparency, fairness, and responsible usage of this technology?
Ethical considerations are crucial, Mark. Transparency is key, ensuring users understand they are interacting with an AI model. Open-sourcing models, rigorous testing to identify biases, and continuous improvement are essential elements to promote fairness and responsible usage.
This technology sounds promising for collaborative data modeling. Imagine researchers and mathematicians being able to interact with the data dynamically through conversations. It could revolutionize scientific collaboration!
Absolutely, Sophia! The interactive nature of ChatGPT opens up exciting possibilities for collaborative data modeling. Dynamic conversations can enhance the exploration of complex concepts and foster interdisciplinary collaborations.
Do you think ChatGPT could eventually replace traditional mathematical modeling approaches, or will it primarily serve as a complementary tool?
That's a thought-provoking question, Joshua. While ChatGPT brings new perspectives and capabilities, I believe it will primarily serve as a powerful complementary tool, augmenting traditional approaches rather than replacing them.
It's fascinating to see how machine learning and natural language processing can be applied to data modeling. I'm excited about the potential for innovation and discovery in mathematics.
Indeed, Olivia! The synergy between machine learning, natural language processing, and data modeling presents immense opportunities for innovation and advancing mathematical research. Exciting times ahead!
I'm curious to know if ChatGPT can assist in solving long-standing mathematical conjectures or aid in proofs of theorems. Can it contribute to advancing pure mathematics?
Great question, Kevin! While ChatGPT is a valuable tool, it's important to note that mathematical conjectures and proof discovery involve deep reasoning and creativity. ChatGPT can certainly provide insights, but it's unlikely to replace the innovative thinking required for significant breakthroughs.
I can see immense potential in applying ChatGPT to educational settings. It could aid students in understanding complex mathematical concepts and provide personalized explanations.
Absolutely, Robert! ChatGPT's conversational abilities could be a valuable tool in educational settings. It can provide additional support, explanations, and personalized assistance to students, making learning mathematics more accessible and engaging.
I'm curious about the scalability of ChatGPT. Can it handle large datasets and complex mathematical models effectively?
Scalability is an important aspect, Lisa. While ChatGPT has limitations, advances in hardware and model improvements allow for handling larger datasets and complex mathematical models. Researchers continue to make progress in optimizing its scalability.
Are there any privacy concerns associated with using ChatGPT in data modeling? How can we ensure the protection of sensitive information?
Privacy considerations are vital, Michael. It's essential to handle sensitive information responsibly. Anonymization techniques, secure data handling practices, and respecting privacy regulations are necessary to ensure the protection of sensitive data during the use of ChatGPT or any other AI technology.
It would be interesting to see real-world examples of how ChatGPT has been applied in mathematical data modeling. Are there any notable case studies or applications?
Indeed, Jessica! Researchers and organizations have been exploring applications of ChatGPT in various domains, including mathematical data modeling. While there are no notable case studies specifically on this topic yet, emerging studies indicate promising potential for using ChatGPT in mathematical research and applications.
With the advancement of AI, do you think there is a risk of becoming overly reliant on technologies like ChatGPT? How do we ensure a balanced approach?
Your concern is valid, Brian. While AI technologies like ChatGPT are powerful, maintaining a balanced approach is crucial. Human expertise, critical thinking, and constant evaluation of AI's limitations are essential to prevent overreliance. Combining the strengths of both humans and AI can lead to the best outcomes.
I'm excited about the democratizing potential of ChatGPT in mathematical data modeling. It can make complex data analysis more accessible to a broader audience, empowering researchers and enthusiasts alike.
Absolutely, Grace! Making mathematical data modeling more accessible through ChatGPT can enable a wider range of individuals to actively engage in data analysis and contribute to the field. It has the potential to democratize participation and drive collaborative exploration.
I'm curious about the computational requirements of using ChatGPT for mathematical data modeling. Are there any notable hardware or infrastructure considerations?
Good question, Eric. ChatGPT, being a highly sophisticated model, benefits from powerful hardware and infrastructure. GPUs and specialized hardware accelerators play a significant role in enabling efficient computation for large-scale data modeling tasks.
How do you foresee the future development and integration of ChatGPT technology in the field of mathematics? Any potential challenges or directions?
The future of ChatGPT in mathematics looks promising, Luke. Advancements in fine-tuning for domain-specific knowledge, addressing biases, and enhancing conversational capabilities will likely be key areas for development. Challenges include ensuring accuracy, addressing privacy concerns, and defining ethical guidelines for responsible usage.
ChatGPT in mathematics seems like a game-changer for research collaboration. The ability to have natural conversations with data opens up exciting possibilities for interdisciplinary partnerships.
Absolutely, Isabella! The collaborative potential of ChatGPT in mathematics holds great promise. It can bridge gaps between different disciplines, facilitate knowledge sharing, and foster innovative solutions through interactive conversations with data.
Do you think ChatGPT will have a significant impact on data-driven decision-making processes in various industries? Can it help uncover valuable insights?
Indeed, Daniel. ChatGPT's interactive conversational abilities can aid data-driven decision-making processes in diverse industries. By engaging with data in a natural language manner, ChatGPT can uncover valuable insights, support analysis, and enable more informed decision-making.
I can see a potential application of ChatGPT in mathematical education for enhancing student engagement. It can provide interactive learning experiences that go beyond traditional textbooks.
Absolutely, Sophie! ChatGPT can offer a more interactive and engaging learning environment for mathematics education. Students can actively explore concepts through conversations, receiving personalized explanations and support, thereby enhancing their understanding and interest in the subject.
What are the current challenges in implementing ChatGPT for mathematical data modeling? Are there any bottlenecks or limitations that need to be addressed?
There are indeed challenges, Maxwell. Ensuring the accuracy of generated models, addressing potential biases, and minimizing nonsensical responses are ongoing concerns. Fine-tuning for domain-specific knowledge and balancing performance with computational requirements are also areas requiring attention.
I'm curious about the training data used for ChatGPT in mathematical data modeling. How was it sourced, and what kind of datasets were involved?
Excellent question, Emma. Training data for ChatGPT involves a large corpus of texts from the internet. It encompasses diverse mathematics-related materials, including textbooks, research papers, and online educational resources. This wide range of sources helps in capturing a comprehensive understanding of the subject.
Are there any potential commercial applications of ChatGPT in mathematical data modeling? Can it be integrated into existing software systems?
Certainly, Andrew! There are potential commercial applications for ChatGPT in mathematical data modeling. Integrating it into existing software systems can enhance data analysis capabilities, support decision-making processes, and enable more advanced mathematical modeling in various industries.
How can we ensure transparency and explainability when using ChatGPT for mathematical data modeling? Understanding the model's reasoning is crucial for building trust.
You're right, Jennifer. Transparency and explainability are essential. Researchers are actively working on techniques to interpret and explain the decisions made by ChatGPT. Interpretable AI, model introspection, and individual explanation methods aim to provide insights into the model's reasoning, building trust in its outcomes.
Thank you all for your valuable contributions to this discussion! Your insights and questions have added depth to the conversation around the revolutionary potential of ChatGPT in mathematics. It was a pleasure engaging with you all!