Revolutionizing Data Warehousing: Unleashing the Power of ChatGPT for Big Data Technology
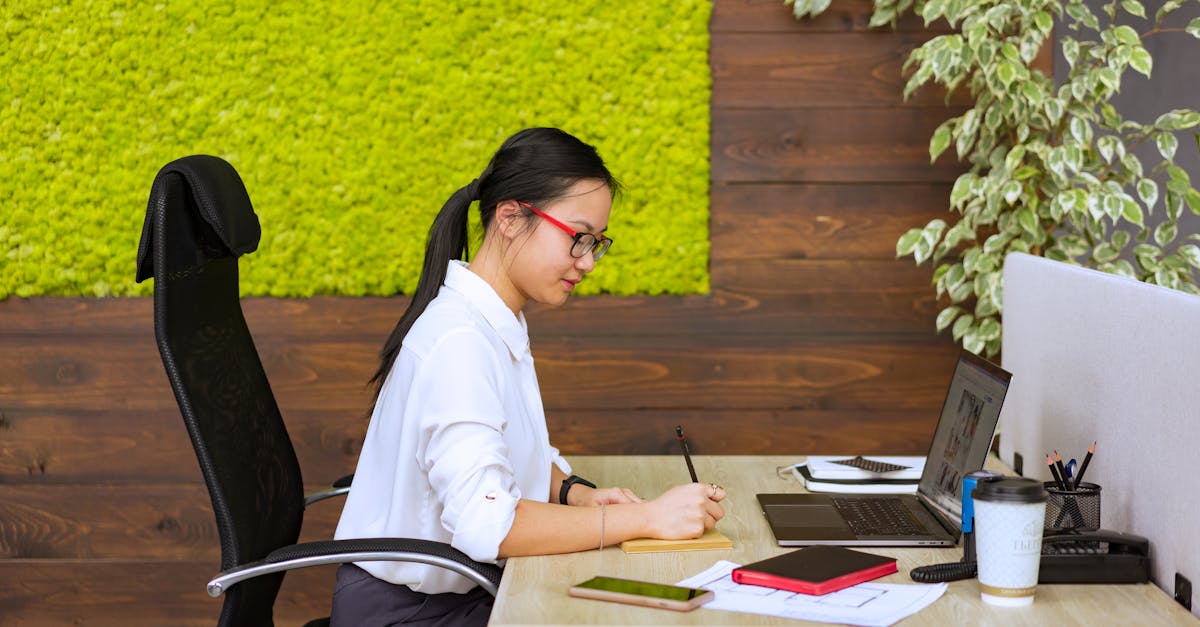
Introduction
Data warehousing is a crucial aspect of modern information systems. It involves consolidating data from various sources into a centralized repository for analysis and reporting purposes. With the advent of big data, the volume, variety, and velocity of data have increased exponentially, posing new challenges and opportunities for the field of data warehousing.
What is Big Data?
Big Data refers to extremely large and complex datasets that cannot be easily managed, processed, or analyzed using traditional databases and analytical tools. These datasets often consist of unstructured and semi-structured data from different sources such as social media, sensors, logs, and other digital channels. Big Data technologies enable organizations to extract insights from these massive datasets and make data-driven decisions.
Big Data in Data Warehousing
ChatGPT-4, an advanced natural language processing model, can greatly assist in designing efficient data warehousing architectures. It can suggest data partitioning strategies based on the characteristics of the dataset and the intended analytical workload. Furthermore, ChatGPT-4 can provide valuable advice on indexing techniques to optimize query performance in a big data environment. The integration of Big Data technologies like Hadoop and Spark allows data warehousing systems to handle massive volumes of data efficiently.
Benefits of Big Data in Data Warehousing
The usage of Big Data in data warehousing brings numerous advantages:
- Scalability: Big Data technologies enable horizontal scalability, allowing organizations to store and process vast amounts of data.
- Faster Insights: With parallel processing capabilities, Big Data technologies can expedite data analysis and provide near-real-time insights.
- Cost Efficiency: The ability to leverage commodity hardware and distributed computing reduces infrastructure costs.
- Enhanced Decision-Making: Big Data analytics can uncover hidden patterns and correlations in data, enabling organizations to make informed decisions.
Future Trends
The field of Big Data in data warehousing is continuously evolving. Some of the emerging trends include:
- Advanced Analytics: Incorporating machine learning and artificial intelligence techniques will enable sophisticated analysis and predictive modeling.
- Real-Time Data Warehousing: The ability to process and analyze data in real-time will become increasingly important.
- Data Integration: Integrating data from various sources, including IoT devices and cloud-based systems, will provide a comprehensive view for analysis.
- Data Governance: Establishing robust data governance frameworks to ensure data quality, security, and compliance.
Conclusion
The role of Big Data in data warehousing is pivotal in managing the immense volume and complexity of data in today's digital world. With the assistance of advanced technologies like ChatGPT-4, organizations can design efficient data warehousing architectures, optimize performance, and gain valuable insights from their vast datasets. As Big Data continues to evolve, data warehousing practices will need to adapt to leverage the full potential of this technology.
Comments:
Thank you all for joining the discussion on my article 'Revolutionizing Data Warehousing: Unleashing the Power of ChatGPT for Big Data Technology'. I'm excited to hear your thoughts!
Great article, Tony! I'm particularly interested in how ChatGPT can assist in handling unstructured data. Can you share some insights on that?
Certainly, Anne! ChatGPT's ability to understand and generate human-like responses makes it well-suited for analyzing unstructured data. It can uncover patterns and extract information from vast amounts of text, improving data warehousing processes. The natural language processing capabilities of ChatGPT make it an excellent tool for tackling unstructured data challenges.
Thank you for the clarification, Tony. It's fascinating to see how ChatGPT can unlock insights from unstructured data. This opens up new possibilities in data analysis and decision-making.
Thank you for clarifying, Tony. I can see the tremendous value ChatGPT can bring to the analysis of unstructured data. Truly transformative!
I'm curious about the scalability of ChatGPT in big data scenarios. Can it handle large volumes of data efficiently?
That's a great question, Robert. ChatGPT's scalability largely depends on the underlying infrastructure it is built upon. By leveraging distributed systems and parallel processing, ChatGPT can efficiently handle big data scenarios. Additional optimization techniques, such as precomputing certain computations and using efficient data storage techniques, are also employed. These factors together contribute to ChatGPT's ability to handle large volumes of data effectively.
Thanks, Tony! The scalability aspect is crucial when dealing with large-scale data. It's impressive how ChatGPT utilizes distributed systems for efficient processing.
Thanks for explaining the scalability aspect, Tony. Utilizing distributed systems and optimization techniques is essential when dealing with massive data volumes.
I'm interested in understanding the advantages of using ChatGPT for data warehousing compared to other traditional methods. Any insights?
Certainly, Elena! One of the major advantages of using ChatGPT for data warehousing is its ability to process and understand natural language. Unlike traditional methods that require predefined structures or schemas, ChatGPT can handle unstructured data, providing more flexibility and adaptability. Additionally, ChatGPT's conversational abilities allow for interactive queries and insights generation, enhancing data exploration and analysis. These advantages make ChatGPT a powerful tool in revolutionizing data warehousing.
Thank you for explaining the advantages, Tony. The flexibility and interactive nature of ChatGPT indeed make it a valuable tool in data warehousing.
Thanks for shedding light on the advantages, Tony. ChatGPT's flexibility and ability to handle unstructured data make it a powerful addition to traditional methods.
ChatGPT sounds intriguing, but are there any limitations to its applicability in big data technology?
Indeed, Sophia, while ChatGPT offers numerous benefits, it also has limitations. One limitation is the need for substantial computational resources, especially when handling massive volumes of data. Additionally, as an AI model, it is not immune to biases present in the training data. Careful considerations should be taken to limit biases and ensure fairness. Lastly, it's important to note that ChatGPT is not a standalone solution but rather an additional tool that can enhance existing data warehousing processes.
I'm curious about the security aspects of using ChatGPT for big data technology. Are there any concerns related to data privacy and potential breaches?
Valid point, Alexandra. When integrating ChatGPT into data warehousing systems, ensuring data privacy and security is crucial. Access controls, encryption mechanisms, and secure communication protocols should be implemented to prevent potential breaches. Additionally, data anonymization techniques can be employed to safeguard sensitive information. While ChatGPT itself doesn't inherently pose security threats, maintaining secure infrastructure and following best practices is vital.
Appreciate your insights on data privacy and security, Tony. Safeguarding sensitive information should always be a top priority.
Tony, how do you envision the future of ChatGPT in the field of big data? Any upcoming advancements we can look forward to?
Great question, William! The future of ChatGPT in big data is promising. Advancements in the underlying architectures, such as more efficient model training and better resource management, can enhance its scalability and performance. Additionally, researchers are working on reducing biases and improving fairness in AI models. Integration with other cutting-edge technologies, like graph databases and real-time analytics, can further unlock the potential of ChatGPT in revolutionizing data warehousing. Exciting times lie ahead!
Exciting times indeed, Tony! I'm curious to see how ChatGPT evolves and integrates with other emerging technologies in the future.
Tony, do you think ChatGPT has the potential to replace traditional data warehousing methods entirely?
That's an interesting question, Michael. While ChatGPT brings significant advancements and flexibility, I don't foresee it completely replacing traditional data warehousing methods. Instead, I believe ChatGPT will act as a complementary tool, augmenting existing processes and empowering data analysts and scientists. Traditional methods still provide robustness and reliability, whereas ChatGPT excels in handling unstructured data and enabling interactive data exploration. It's about finding the right balance!
In your article, you mentioned 'unleashing the power of ChatGPT.' Can you elaborate on what makes ChatGPT powerful in the context of big data technology?
Certainly, Emily! ChatGPT's power lies in its ability to process and generate human-like responses in natural language. This enables data analysts and scientists to interact with data in a conversational manner, making exploration and analysis more intuitive. Furthermore, ChatGPT's adaptability and flexibility in handling unstructured data bring new possibilities for discovering insights from vast amounts of unstructured information. By leveraging these capabilities, ChatGPT can revolutionize data warehousing processes and contribute to better decision-making.
Thank you for elaborating on ChatGPT's power, Tony. The human-like conversational capabilities make it an exciting tool for data exploration.
Are there any examples or use cases where ChatGPT has already been successfully employed in the field of big data?
Absolutely, David! ChatGPT has already shown promise in various big data applications. For example, it has been utilized for data classification and clustering tasks, where its ability to understand unstructured data enables efficient categorization. Additionally, ChatGPT has been leveraged for sentiment analysis and summarization of large text datasets, allowing for quick insights extraction. These are just a few examples, and as ChatGPT continues to evolve, its potential use cases in big data technology will expand further.
The use cases you mentioned, Tony, show the versatility of ChatGPT in big data applications. It's amazing to see its potential.
Tony, can ChatGPT assist in real-time data processing, or is it more suitable for batch processing?
Good question, Sara. While ChatGPT can be used for real-time data processing to a certain extent, its strength lies in interactive data exploration and ad hoc queries. For applications requiring real-time processing, other specialized systems like stream processing frameworks are more suitable. By integrating ChatGPT alongside these systems, real-time insights generation becomes possible. It's about leveraging the strengths of different technologies to create a comprehensive and efficient big data ecosystem.
Integrating ChatGPT with specialized real-time processing systems seems like a smart approach to get the best of both worlds. Thank you for clarifying, Tony.
Finding the balance between real-time processing and interactive data exploration seems key. Integrating ChatGPT with other technologies can enable comprehensive big data processing. Thanks, Tony!
Considering the ever-increasing amount of data, how does ChatGPT handle data quality issues and noise in big data?
Valid concern, Oliver. ChatGPT's performance can be affected by data quality issues and noise present in big data. Preprocessing techniques like data cleaning, outlier detection, and noise reduction are essential to mitigate these challenges. Filtering irrelevant data and ensuring data correctness are crucial steps to improve the accuracy of insights generated by ChatGPT. As the quality of data improves, ChatGPT becomes more reliable in extracting meaningful information and patterns from big data.
Thanks for addressing the data quality issues, Tony. ChatGPT's reliability improves as the quality of input data improves.
Preprocessing techniques play a vital role in improving ChatGPT's performance with respect to data quality. Thanks for the insights, Tony.
The ethical aspects of using AI models like ChatGPT are often discussed. What ethical considerations should be taken into account when applying ChatGPT to big data technology?
An important question, Isabella. Ethical considerations are crucial when working with AI models like ChatGPT. Firstly, dealing with biases in training data is vital to ensure fairness in decision-making. Transparent documentation and explainability mechanisms can help in identifying biases and addressing potential issues. Additionally, data privacy and protection of sensitive information should be prioritized. It's crucial to have clear policies and guidelines in place to handle data responsibly and protect user privacy. Ethics should always be a fundamental aspect of AI deployments.
I appreciate your emphasis on ethics, Tony. It's essential to ensure AI models like ChatGPT are deployed responsibly and with proper oversight.
Transparency, fairness, and responsible data handling are crucial in the deployment of AI models. Thank you for emphasizing ethical considerations, Tony.
Tony, do you think the integration of ChatGPT will require significant changes in the existing infrastructure of data warehousing systems?
Great question, Gabriel. The integration of ChatGPT does require some changes in the existing infrastructure of data warehousing systems, but it can often be done without significant disruptions. Depending on the specific implementation, integrating ChatGPT may involve setting up the necessary computational resources, incorporating natural language processing pipelines, and establishing communication channels with existing data warehousing components. With careful planning and appropriate technical expertise, integrating ChatGPT can be a smooth process.
Regarding the biases you mentioned, Tony, what steps can be taken to address them and ensure fairness in AI applications?
Excellent question, Lucas. There are several steps that can be taken to address biases and ensure fairness in AI applications. Firstly, diverse and representative training datasets should be used to minimize bias at the source. Secondly, continuous monitoring and analysis of model outputs can help identify potential biases and mitigate them through fine-tuning and retraining. Additionally, involving ethicists and domain experts in the development and evaluation process can provide valuable perspectives on fairness. Addressing biases requires a multi-dimensional approach involving diverse stakeholders and ongoing efforts.
Great article, Tony! I appreciate the insights on using ChatGPT for data warehousing. It seems like a promising approach that can bring significant value to big data technology.
I totally agree, Javier. ChatGPT's capabilities for handling unstructured data and interactive querying can truly revolutionize data warehousing. Exciting times ahead!
Addressing biases requires a holistic approach involving diverse perspectives. It's important to continuously work towards developing fair AI applications. Thanks for the insights, Tony.
Thank you for addressing my concern, Tony. It's reassuring to know that the integration process can be smooth with the right planning and expertise.
Finding the right balance between traditional methods and new advancements is key. ChatGPT's ability to handle unstructured data definitely adds value.