Revolutionizing Database Administration: Leveraging ChatGPT for Advanced Database Clustering and Replication
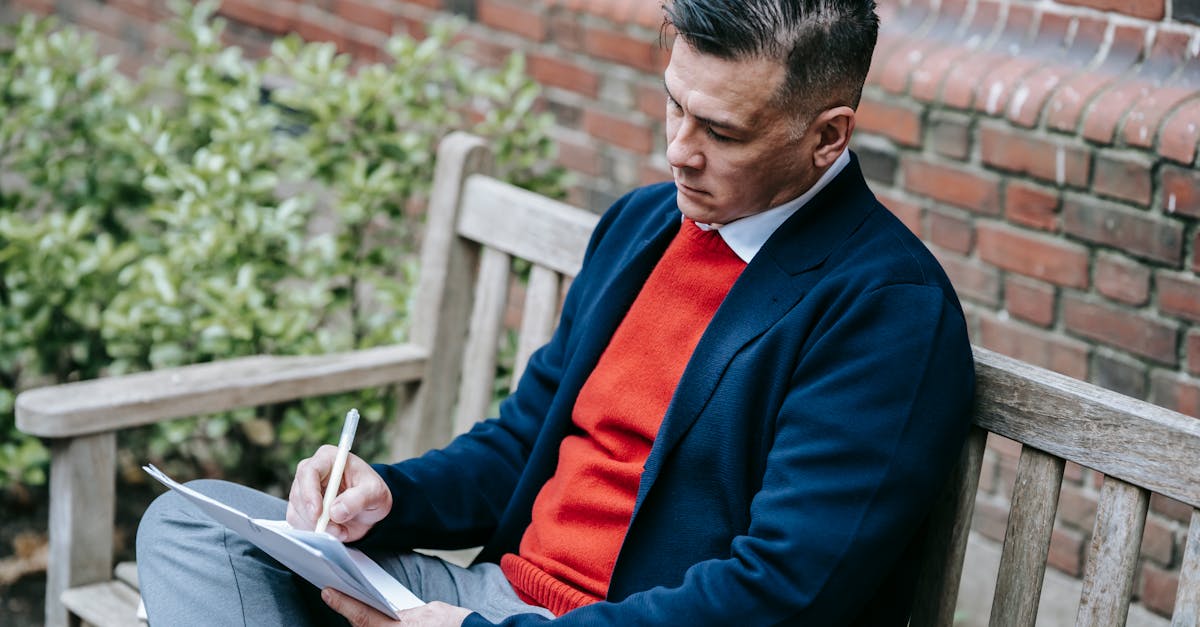
Introduction
Database clustering and replication are essential techniques in the field of database administration. These techniques help improve the availability, scalability, and reliability of databases, ensuring optimal performance even during peak loads or in the case of hardware failures. In this article, we will explore the benefits of database clustering and replication, provide implementation guidance, and address common troubleshooting scenarios.
Benefits of Database Clustering and Replication
High Availability: Clustering and replication techniques ensure that even if one database node fails, the system can continue to operate smoothly. This improves the availability of the database, reducing downtime and maintaining consistent application access.
Scalability: By distributing the database workload across multiple nodes, clustering and replication allow for horizontal scalability. This means that as the workload increases, additional nodes can be added to handle the increased load, ensuring optimal performance.
Improved Performance: Database clustering and replication techniques enhance performance by distributing the processing load across multiple nodes. This reduces the workload on individual nodes, resulting in faster query response times and improved overall system performance.
Implementation Guidance
When implementing database clustering and replication, there are several key considerations to keep in mind:
- Topology: Choose the appropriate clustering and replication topology based on your specific requirements. Common topologies include master-slave replication, master-master replication, and multi-master replication.
- Hardware and Network: Ensure that the hardware and network infrastructure can support the increased demands of clustering and replication. This may involve using high-performance servers, redundant network connections, and fast storage systems.
- Data Consistency: Implement mechanisms to ensure data consistency across nodes. This may involve using synchronous or asynchronous replication and employing techniques like write locks, transaction logs, or conflict resolution algorithms.
- Monitoring and Maintenance: Regularly monitor the health and performance of the clustered and replicated database. Perform routine maintenance tasks such as node failure detection, failover testing, and data backup and recovery.
Troubleshooting Scenarios
Despite the benefits and careful implementation, issues may arise in database clustering and replication environments. Some common troubleshooting scenarios include:
- Node Failure: When a database node fails, it is important to quickly detect the failure and initiate failover mechanisms to ensure uninterrupted service. Regularly test failover mechanisms to ensure they perform as expected in real-world scenarios.
- Data Inconsistency: In distributed database environments, data inconsistencies may occur due to network disruptions, conflicts in data updates, or hardware failures. Employ appropriate mechanisms such as automatic synchronization or manual intervention to resolve data inconsistencies.
- Performance Bottlenecks: Identify and address performance bottlenecks that may arise due to uneven workload distribution, network latency, or inefficient query execution plans. Monitor the system performance, implement load balancing, and tune the database configuration to optimize performance.
- Security: Ensure proper security measures are in place to protect the database and its replicated nodes. Implement firewall rules, encryption, access controls, and regular security audits to safeguard sensitive data.
Conclusion
Database clustering and replication techniques play a crucial role in ensuring high availability, scalability, and performance of databases. By distributing the workload and providing redundancy, clustering and replication techniques enable efficient and reliable data processing. However, careful planning, implementation, monitoring, and troubleshooting are essential for a successful clustering and replication setup. By following best practices and addressing potential challenges, organizations can harness the benefits of these techniques to establish a robust and resilient database infrastructure.
Comments:
Thank you all for taking the time to read my article on leveraging ChatGPT for advanced database clustering and replication.
This is an interesting concept! I've never thought about using AI chatbots for database administration.
Yes, it's an innovative approach. It could potentially bring efficiency and automation to database management.
I'm curious about the scalability of using ChatGPT for large-scale databases. Has anyone tested it on extensive datasets?
I believe OpenAI has conducted some experiments on large datasets. It would be great to hear more details about the performance.
While the idea seems promising, I wonder about the security implications. How can we ensure the chatbot doesn't expose sensitive data?
That is an important concern, Mark. To address it, the chatbot implementation should carefully consider authentication and access controls to prevent unauthorized information disclosure.
I'm curious about the computational resources required for running ChatGPT alongside database operations. Could it introduce performance issues?
Good point, Hannah. ChatGPT can be resource-intensive, so optimizing the infrastructure and considering the server load is crucial.
Agreed, Michael. It's vital to assess the impact on database performance and ensure scalability.
Are there any real-life use cases of ChatGPT being implemented for database clustering and replication?
I came across a case study where a company used ChatGPT to optimize their database replication strategy, resulting in faster synchronization and improved availability.
That sounds promising, Jonathan. Do you have any more details about that case study?
Unfortunately, I don't have the link handy, but I can try to find it and share it here later.
This article raises interesting possibilities for automation in database administration. However, how does ChatGPT handle complex database queries?
Complex queries can be challenging. ChatGPT's underlying language model might not always grasp the full context effectively. It's crucial to train the chatbot on a diverse dataset.
That makes sense, Gary. So, human supervision and fine-tuning are still important to ensure accurate responses for complex queries?
Absolutely, Sarah. Combining AI with human expertise can enhance the chatbot's understanding and provide reliable results.
What programming languages would be suitable for developing this kind of chatbot for database administration?
Python is commonly used for natural language processing and AI tasks. It would be a good choice, I believe.
Java could also be considered as it has robust libraries for working with databases and handling concurrent operations.
Node.js can be another option, especially if you want to leverage JavaScript for both frontend and backend development.
Ultimately, the choice of programming language would depend on the specific requirements and expertise within the development team.
I'm concerned about potential biases arising from training the chatbot. How can we ensure the responses are fair and unbiased?
Addressing biases is crucial. By carefully curating the training data, involving a diverse group of people, and implementing bias mitigation strategies, fairness can be improved.
I'm curious about the implementation of natural language understanding in ChatGPT for database queries. How does it interpret user intentions accurately?
ChatGPT relies on a powerful language model that can identify patterns and contexts to understand user intentions. Additionally, fine-tuning can be performed to improve domain-specific understanding.
I wonder if integrating ChatGPT with voice assistants like Siri or Alexa could enhance the user experience in database administration. What do you think?
That's an intriguing idea, Jonathan! Voice interfaces can provide more natural and intuitive interactions with the chatbot, bringing convenience for users.
Integrating with voice assistants could also make database administration accessible hands-free, which is beneficial for certain use cases.
While the concept seems promising, I wonder if AI-driven chatbots for database administration could completely replace human DBAs in the future?
Indeed, Sarah. DBAs would continue to play a vital role in data governance, security, and handling non-routine scenarios.
I believe AI chatbots can augment DBAs and automate routine tasks, but human expertise and critical decision-making will still be indispensable.
There will always be a need for human DBAs to handle complex situations, troubleshoot issues, and ensure the overall system's reliability.
I agree, Michael. Database management can benefit from leveraging AI to enhance efficiency.
Exactly, Emily. Ensuring scalability is a key factor in implementing AI solutions.
How do you see the future of database administration evolving with the integration of AI technologies like ChatGPT?
I think AI could automate repetitive tasks, enabling DBAs to focus more on strategic decision-making and addressing complex challenges.
Agreed, Mark. DBAs could leverage AI to gain deeper insights from data and ensure optimal database performance.
AI-driven solutions like ChatGPT have the potential to revolutionize the scalability, efficiency, and availability of databases.
The integration of AI technologies can bring innovative approaches that streamline operations, making database administration more agile and adaptive.
Thank you all once again for your valuable insights and questions. It's been a great discussion on the future of database administration with AI. Feel free to reach out to me if you have further queries.
Absolutely, access controls and authentication are crucial to protect sensitive data.
So, starting with a well-curated training dataset is important when dealing with complex queries.
Python's NLP libraries and vast community support make it a sound choice for this kind of development.
Addressing biases in AI systems is indeed critical to ensure ethical and fair outcomes.
Fine-tuning the language model can significantly enhance accuracy and interpretation.
Integrating voice assistants could simplify the user experience and make it more accessible.
A balance between AI automation and human expertise can lead to optimal database management.
AI technologies can empower DBAs to focus on strategic tasks, driving innovation.