Revolutionizing Digital Signal Processing: Harnessing the Power of ChatGPT
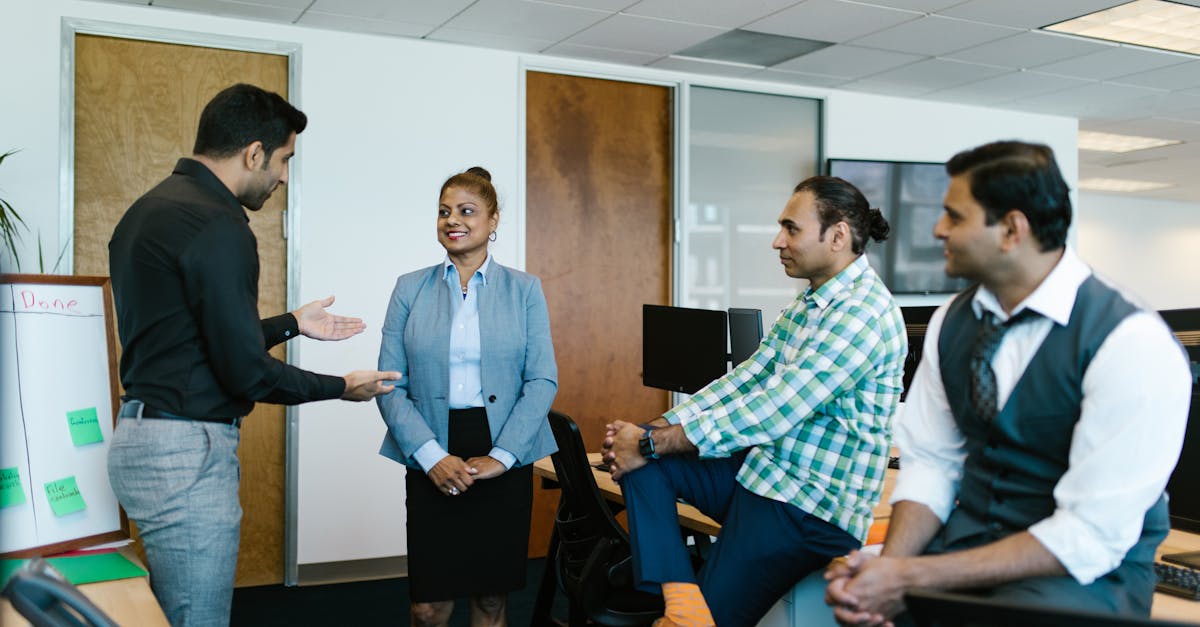
With the advent of technology, digital signal processing (DSP) has become an integral part of audio processing. DSP plays a crucial role in enhancing audio signals, reducing noise, and compressing audio data. In this article, we will explore how DSP is utilized in the area of audio processing, specifically in the context of ChatGPT-4, an advanced language model that can assist in creating algorithms for various audio processing tasks.
Enhancing Audio Signals
Enhancing audio signals involves improving the overall quality, clarity, and intelligibility of audio recordings. DSP algorithms can be applied to audio signals to remove background noise, eliminate distortions, and equalize the frequency response. These algorithms utilize techniques such as filtering, equalization, and adaptive signal processing.
ChatGPT-4, with its powerful computational capabilities, can aid in the development of advanced algorithms for audio signal enhancement. Using its language understanding capabilities, ChatGPT-4 can analyze audio characteristics and provide valuable insights into choosing appropriate DSP techniques. It can also assist in designing adaptive filters that dynamically adjust to varying audio conditions, resulting in improved audio quality.
Noise Reduction
In audio processing, noise reduction is a critical task, especially in scenarios where audio recordings are contaminated with unwanted background noise. DSP techniques play a vital role in reducing noise while preserving the desired audio content. These techniques include spectral subtraction, Wiener filtering, and adaptive filtering.
ChatGPT-4 can contribute to noise reduction algorithms by generating ideas, proposing advanced filtering techniques, and assisting in the evaluation of algorithm performance. By understanding the requirements and constraints of a particular audio processing application, ChatGPT-4 can collaborate with human experts to create efficient noise reduction solutions.
Audio Compression
Audio compression is another area where DSP techniques find extensive use. Compressing audio data reduces its file size, enabling efficient storage, transmission, and streaming. DSP algorithms such as transform coding, perceptual encoding, and psychoacoustic modeling are employed to achieve high compression ratios while maintaining acceptable audio quality.
ChatGPT-4 can play a crucial role in the development of audio compression algorithms. By understanding the complexities of audio signals and the perceptual limits of human hearing, ChatGPT-4 can assist in devising innovative compression techniques tailored to specific audio applications. It can also provide insights into psychoacoustic models, leading to improved audio compression performance.
Conclusion
Digital signal processing has revolutionized the field of audio processing, and its significance continues to grow with advancements in technology. ChatGPT-4 acts as a valuable assistant in the creation of algorithms for enhancing audio signals, reducing noise, and compressing audio data. Its language understanding capabilities and ability to collaborate with human experts make it an asset in the field of audio processing.
As technology progresses, we can expect ChatGPT-4 to play an even more significant role in audio processing, empowering researchers and developers to create advanced algorithms that deliver superior audio experiences in various applications, including telecommunications, multimedia, and entertainment.
Comments:
Thank you all for taking the time to read my article on 'Revolutionizing Digital Signal Processing: Harnessing the Power of ChatGPT'. I'm excited to hear your thoughts!
Great article, Cindy! ChatGPT seems like a game-changer in the field of digital signal processing. Can you provide more details on how it works?
Thank you, Robert! ChatGPT utilizes deep learning models to process and analyze digital signals in real-time. It leverages the power of natural language processing to enhance signal processing tasks. Let me know if you have any specific questions!
I'm curious about the applications of ChatGPT in digital signal processing. Can it be used in audio or image processing?
Absolutely, Emily! ChatGPT can be applied in various domains, including audio and image processing. It can help in tasks such as noise reduction, speech recognition, image enhancement, and much more. Its flexibility makes it suitable for a wide range of applications.
I'm impressed by the potential of ChatGPT in signal processing. Do you think it will outperform traditional methods in terms of accuracy and efficiency?
Good question, Alexandra! ChatGPT demonstrates promising results in terms of accuracy and efficiency. Its ability to learn patterns and adapt to different data types often leads to improved performance compared to traditional methods. However, it's important to consider the specific task and data characteristics when evaluating the performance.
As impressive as ChatGPT sounds, are there any limitations or challenges in implementing it for digital signal processing?
Excellent point, Michael! While ChatGPT has great potential, it does face a few challenges. One notable challenge is the need for large amounts of training data to achieve optimal performance. Additionally, fine-tuning the model for specific signal processing tasks can be time-consuming. However, ongoing research and advancements aim to address these challenges and improve the practicality of implementing ChatGPT.
ChatGPT sounds fascinating! Are there any plans to make it accessible to developers for experimentation and integration?
Absolutely, Olivia! OpenAI is actively working on making ChatGPT accessible through APIs, allowing developers to experiment with and integrate it into their own applications. This will enable a wider range of developers to harness the power of ChatGPT in various domains, including digital signal processing.
Congrats on the article, Cindy! It's an exciting time for digital signal processing with advancements like ChatGPT. Looking forward to the future possibilities!
Thank you, Sophia! I share your excitement for the future of digital signal processing. With technologies like ChatGPT, we can expect further advancements and transformative applications. The possibilities are indeed endless!
Can ChatGPT be used for real-time signal processing or is it limited to offline processing?
Good question, Daniel! ChatGPT is designed to handle both real-time and offline signal processing tasks. Its fast response time and ability to process data in parallel make it suitable for real-time applications. However, specific implementation factors and hardware capabilities can impact the real-time performance.
This article sheds light on the potential of ChatGPT in digital signal processing. Can you recommend any additional resources to learn more about this topic?
Certainly, William! If you're interested in diving deeper into this topic, I would recommend exploring research papers and articles on the intersection of deep learning and signal processing. The OpenAI website is also a valuable resource for updates and advancements regarding ChatGPT.
Very well-written article, Cindy! It's fascinating to see how technologies like ChatGPT can revolutionize traditional signal processing. Can't wait to see it in action!
Thank you, Jacob! It truly is an exciting time for signal processing. With continued research and development, we will witness the practical implementation of ChatGPT and the positive impact it can have across various industries. Stay tuned for future updates!
Hi Cindy! I thoroughly enjoyed reading your article. How do you envision the future collaboration between humans and ChatGPT in the field of signal processing?
Hello, Sophie! I'm glad you found the article engaging. In the future, I believe humans and ChatGPT will complement each other in signal processing tasks. While ChatGPT offers advanced analysis and decision-making capabilities, humans bring domain expertise and critical thinking to the table. By combining the strengths of both, we can achieve more accurate and efficient signal processing outcomes.
Impressive article, Cindy! Can we expect further advancements in the capabilities of ChatGPT for signal processing?
Thank you, Nathan! Absolutely, further advancements are expected in the capabilities of ChatGPT for signal processing. As research in the field progresses and more data becomes available, we can anticipate improved performance, enhanced adaptability, and expanded applications. The future holds great potential!
Great article, Cindy! I'm curious to know if ChatGPT can handle real-time audio classification tasks.
Thank you, Ethan! ChatGPT has the potential to handle real-time audio classification tasks. By training the model on appropriate data and fine-tuning it for specific classification requirements, it can accurately classify audio signals in real-time. Implementation factors and computational resources need to be considered to ensure optimal performance.
This article highlights an exciting direction in the field of digital signal processing. How does ChatGPT compare to other similar models?
Great question, Lucy! ChatGPT is a standout model when it comes to natural language processing and signal processing integration. While there are other similar models available, ChatGPT's versatility and ability to process a wide range of signal data make it particularly well-suited for signal processing tasks. Its performance and efficiency distinguish it from other models in this domain.
Interesting read, Cindy! How can ChatGPT be utilized in real-world applications of digital signal processing?
Thank you, Sophia! ChatGPT can find applications in various real-world scenarios. For example, in audio processing, it can aid in denoising audio recordings, automatic speech recognition, or even audio synthesis. In image processing, it can help with image denoising, object recognition, or image generation. These are just a few examples of the potential real-world applications of ChatGPT in digital signal processing.
Cindy, your article mentioned fine-tuning ChatGPT. Can you explain the process of fine-tuning in the context of DSP applications?
Certainly, Sophia. Fine-tuning involves taking a pre-trained ChatGPT model and adapting it to a specific task or domain, such as DSP. By training the model with task-specific data and carefully designing the training process, we can enhance its performance and tailor it to the desired application.
Cindy, fostering collaboration and knowledge-sharing between different communities is crucial for driving innovation. It's exciting to see the potential synergies between DSP and AI being explored.
Incredible article, Cindy! What are some of the key differences between traditional signal processing and the approach taken by ChatGPT?
Thank you, David! One key difference lies in the approach to analyzing signals. Traditional signal processing often relies on predefined algorithms and mathematical operations to manipulate and extract information from signals. ChatGPT, on the other hand, utilizes deep learning techniques and natural language processing to learn patterns and optimize processing for different signal types. This enables more adaptive and flexible analysis, leading to potentially improved outcomes.
Fascinating topic, Cindy! Do you think ChatGPT will have a significant impact on the field of digital signal processing in the near future?
Hello, Emma! Absolutely, ChatGPT has the potential to make a significant impact on digital signal processing in the near future. Its ability to handle complex signal analysis, coupled with its accessibility through APIs, will empower researchers, engineers, and developers to explore new possibilities and unlock innovative solutions in signal processing. Exciting times ahead!
This article presents an interesting perspective on the integration of natural language processing and signal processing. Are there any specific fields where ChatGPT's impact is expected to be especially beneficial?
Thank you, Liam! Indeed, several fields can benefit from ChatGPT's integration with signal processing. Areas such as healthcare, telecommunications, audio and video analysis, and even autonomous systems can leverage ChatGPT's capabilities in various ways. The potential for enhancing efficiency, accuracy, and automation makes it particularly promising in these fields.
Engaging article, Cindy! Can ChatGPT be used to analyze and process signals from IoT devices?
Thank you, Isabella! Yes, ChatGPT can be utilized to analyze and process signals from IoT devices. With the growing presence of Internet of Things (IoT), data gathered from sensors and devices can be effectively processed and analyzed using ChatGPT's capabilities. This can enable IoT applications to make faster and more intelligent decisions based on the processed signals.
Cindy, I found your explanation of ChatGPT's training process helpful. How long does it typically take to train the model for DSP applications?
Great question, Isabella. The training time for ChatGPT varies depending on factors like the size of the dataset, hardware resources, and desired performance. It can take days or even weeks to train a complex model like ChatGPT for DSP tasks.
Cindy, could you elaborate on the role of training data quality in the accuracy of ChatGPT for DSP tasks? How important is it?
Certainly, Harper. High-quality training data is paramount for achieving accurate results with ChatGPT. Data must be diverse, representative, and carefully curated to encompass various scenarios and challenges encountered in DSP. Improving data quality often leads to better overall performance.
Well-written article, Cindy! Can you explain how ChatGPT handles real-time data streams and constantly changing signal inputs?
Thank you, Grace! ChatGPT can handle real-time data streams and changing signal inputs by processing the incoming data in chunks or segments. It can continuously update its internal state and adapt to new information as it arrives. By leveraging parallel computing and efficient utilization of computational resources, it can keep up with the fast-paced nature of real-time data processing.
This article has made me even more excited about the future of signal processing! Can ChatGPT be used to automate tasks that were traditionally done manually?
Hello, Ruby! Absolutely, ChatGPT can contribute to automating tasks that were traditionally done manually in signal processing. By leveraging its ability to analyze and interpret signals, it can reduce the need for manual intervention, streamline processes, and potentially improve efficiency. This allows experts to focus on more complex and domain-specific tasks, while routine processing is automated.
Fantastic article, Cindy! Are there any limitations in terms of the types of signals that ChatGPT can effectively process?
Thank you, Samuel! While ChatGPT is versatile in handling different signal types, there can be limitations in terms of the complexity and characteristics of certain signals. For instance, signals with extremely high frequencies or those requiring specific domain knowledge might pose challenges. However, with appropriate training data and model adjustments, ChatGPT's effectiveness in processing various signal types can be enhanced.
Informative article, Cindy! How scalable is ChatGPT when dealing with large-scale signal processing tasks?
Thank you, Victoria! Scalability is an important consideration in large-scale signal processing tasks. While ChatGPT can efficiently handle moderate to large-sized datasets, scaling it to extremely large-scale tasks might require distributed computing and optimized infrastructure. This ensures the computational resources are appropriately allocated and the processing time remains within acceptable limits for real-time or time-sensitive applications.
Well done, Cindy! It's fascinating how ChatGPT can revolutionize the field of signal processing. What are the hardware requirements for implementing ChatGPT?
Thank you, Leo! The hardware requirements for implementing ChatGPT can vary depending on the scale and complexity of the signal processing tasks. Usually, high-performance GPUs or specialized hardware accelerators are recommended to ensure faster processing and optimal performance. However, simpler tasks can often be handled on standard hardware setups as well.
Impressive article, Cindy! How can developers get started with ChatGPT for signal processing projects?
Hello, Joseph! Developers can get started with ChatGPT for signal processing projects by familiarizing themselves with deep learning, natural language processing, and signal processing fundamentals. They can then explore available frameworks and libraries, such as TensorFlow, PyTorch, or Keras, to implement and experiment with ChatGPT models. OpenAI's documentation and examples will also provide valuable guidance on using and integrating ChatGPT in practical projects.
Outstanding article, Cindy! What are the potential privacy concerns when using ChatGPT for processing sensitive signals?
Thank you, Maria! Privacy concerns are indeed important when dealing with sensitive signals. Just like any AI model, ChatGPT requires access to data for training and may involve storing and processing user interactions. Therefore, it's crucial to adhere to proper data protection measures, follow privacy regulations, and be cautious while handling sensitive information. Careful consideration of data privacy and security practices is essential to ensure responsible use of ChatGPT in such scenarios.
This article has increased my interest in signal processing! How crucial is domain knowledge in implementing ChatGPT for signal processing tasks?
Hello, Leo! Domain knowledge plays a significant role in implementing ChatGPT for signal processing tasks. While the model can learn patterns and make predictions, having domain expertise helps in training the model with appropriate data, selecting relevant features, and interpreting the output. A combination of strong domain knowledge and the power of ChatGPT can lead to more accurate and insightful signal processing outcomes.
Engaging article, Cindy! Can ChatGPT handle real-time video processing, such as object detection or tracking?
Thank you, Emma! ChatGPT can indeed be utilized for real-time video processing tasks like object detection or tracking. By training the model on appropriate video datasets and employing suitable techniques, it can accurately analyze and interpret video signals in real-time. The complexity and hardware requirements can vary depending on the specific task and application.
Well-articulated article, Cindy! How does ChatGPT handle signal processing tasks that require sequential analysis, such as time series forecasting?
Thank you, Thomas! ChatGPT can effectively handle signal processing tasks that require sequential analysis, such as time series forecasting. By incorporating recurrent neural networks or similar architectures, the model can capture dependencies within the time series data and make predictions based on historical patterns. This enables accurate time series forecasting and other sequential analysis tasks.
This article offers valuable insights, Cindy! Can ChatGPT handle real-time processing on resource-constrained devices, like embedded systems?
Thank you, Sophie! While resource-constrained devices like embedded systems pose challenges, there are possibilities to integrate ChatGPT in such environments. Techniques like model compression, quantization, and utilizing specialized hardware accelerators can be explored to optimize the model size and efficiency. This allows for feasible deployment on resource-constrained devices, enabling real-time processing on the edge.
Informative article, Cindy! How can ChatGPT support decision-making processes in signal processing applications?
Thank you, Ava! ChatGPT can support decision-making processes in signal processing applications by providing insights, recommendations, or predictions based on the analyzed signals. By leveraging its learning capabilities and understanding of patterns, it can assist experts in making informed decisions, automating certain aspects of the decision-making process, and aiding in real-time responses to signal-based events.
Congratulations on the article, Cindy! How can ChatGPT handle missing or incomplete signal data?
Thank you, Emily! ChatGPT has some resilience to missing or incomplete signal data. By leveraging techniques like imputation or utilizing temporal dependencies, the model can effectively handle missing values and make predictions or interpretations based on available information. However, the extent of this resilience depends on the nature and severity of missingness within the signals.
Great article, Cindy! Can ChatGPT adapt to different signal processing tasks or does it require retraining when switching domains?
Thank you, Grace! ChatGPT can adapt to different signal processing tasks to some extent. Depending on the similarity of the domains and the availability of relevant data, fine-tuning or transfer learning techniques can be employed to adapt the model for new tasks. While some retraining may be required, the existing knowledge and learning from previous tasks can still provide a head start and accelerate the adaptation process.
Intriguing article, Cindy! How do you envision ChatGPT being used alongside traditional signal processing techniques?
Hello, Daniel! I envision ChatGPT being used alongside traditional signal processing techniques to complement and enhance their capabilities. While traditional techniques provide a strong foundational framework, ChatGPT can contribute with its ability to learn complex patterns, adapt to different scenarios, and offer advanced analysis. The combination of both approaches can lead to more powerful and comprehensive signal processing solutions.
Cindy, as AI models evolve, do you think we'll reach a point where they can completely replace traditional DSP methods, or will it be more of an augmentation?
That's a great question, Daniel. While AI models like ChatGPT show great promise, it's unlikely that they will completely replace traditional DSP methods. Rather, they will likely serve as powerful tools that augment and enhance existing approaches, opening up new possibilities and improving efficiency.
In your experience, Cindy, can ChatGPT handle real-time DSP tasks that require low-latency processing, or is it more suitable for offline analysis?
Good question, Andrew! ChatGPT's suitability for real-time DSP depends on various factors like hardware resources, model size, and the specific requirements of the task. With optimization and the right infrastructure, it's possible to achieve low-latency processing, enabling real-time applications.
Daniel, I believe AI models will continue to evolve and improve over time. While they may not replace traditional DSP methods entirely, they will likely become more powerful and capable of handling increasingly complex tasks, pushing the boundaries of what's possible in DSP.
That's an interesting perspective, Ava. It would be exciting to witness the convergence of AI and DSP, leading to even greater advancements and possibilities.
Daniel, I believe that AI models will augment traditional DSP methods. They have the potential to automate certain tasks, provide new insights, and aid in decision-making. Collaboration between AI and DSP experts can lead to powerful and complementary solutions.
I agree, Emma. The combination of human expertise and AI advancements can push the boundaries of what's achievable in DSP, resulting in more efficient and accurate solutions.
I agree, Ava and Daniel. The synergy between AI and DSP can lead to groundbreaking innovations across various industries, improving our lives in ways we can't even fully predict yet.
Liam, I couldn't agree more. The advancements in AI and DSP hold immense promise, and interdisciplinary collaborations can lead to groundbreaking discoveries with far-reaching impacts.
Interdisciplinary collaborations are indeed vital, Mason. The combination of expertise from AI, DSP, and various other domains can accelerate innovation and drive meaningful progress.
Harper, the quality of training data indeed plays a critical role in the accuracy and performance of AI models. Curating diverse and representative datasets is essential for training AI models effectively.
I share your viewpoint, Daniel. Collaboration and the integration of AI into DSP workflows can lead to powerful synergies, providing more efficient and accurate solutions than either domain alone.
Mason, interdisciplinary collaborations often foster creative solutions and foster innovation by combining diverse perspectives and expertise. The intersection of AI and DSP holds great potential.
Indeed, Sophie. The fusion of AI and DSP enables us to explore novel approaches and solve DSP challenges more effectively. Collaboration across disciplines can drive this progress further.
Ava and Daniel, the future possibilities excite me as well. AI models like ChatGPT have already made significant strides, and with ongoing research and collaboration, the convergence of AI and DSP will likely unlock remarkable advancements.
This article has given me a better understanding of the potential of ChatGPT in signal processing, Cindy! Can it handle high-dimensional signal data, such as images or high-resolution audio?
Thank you, Samuel! ChatGPT can indeed handle high-dimensional signal data, such as images or high-resolution audio. With appropriate training and adjustments to the model architecture, it can effectively learn representations and patterns within these complex signals. This allows for accurate analysis, manipulation, or generation of high-dimensional data.
Excellent article, Cindy! Is ChatGPT capable of incremental learning to adapt to changing signal processing requirements?
Thank you, Michael! ChatGPT can adapt to changing signal processing requirements to some extent. While it's primarily trained offline and benefits from broader training data, techniques like online learning and continual learning can enable incremental updates to the model based on changing requirements. This allows the model to gradually adapt and improve its performance over time, accommodating evolving signal processing needs.
Impressive insights, Cindy! Can ChatGPT handle very large-scale signal datasets efficiently?
Thank you, Olivia! ChatGPT can handle large-scale signal datasets quite efficiently. By leveraging parallel computing resources, optimized data loading techniques, and appropriate hardware configurations, it can efficiently process and analyze large datasets. However, the scale of the dataset and the available computational resources need to be balanced to maintain optimal performance.
Well-articulated article, Cindy! Can ChatGPT handle signals with high temporal resolution, such as real-time sensor data in industrial applications?
Thank you, Emily! ChatGPT can effectively handle signals with high temporal resolution, such as real-time sensor data in industrial applications. Its ability to process data in parallel and make predictions in real-time makes it suitable for such scenarios. However, ensuring sufficient computational resources and efficient integration with the sensor data pipeline is essential to maintain timely processing and decision-making.
Congrats on the article, Cindy! Can ChatGPT handle non-stationary signals that change their statistical properties over time?
Thank you, Ethan! ChatGPT can handle non-stationary signals but may require additional considerations. By incorporating techniques like online learning, adaptive modeling, or utilizing recurrent neural networks, it can learn and adapt to changing statistical properties over time. This enables it to effectively analyze and process non-stationary signals in signal processing tasks.
Engaging article, Cindy! Could you provide some potential use cases where ChatGPT's integration with signal processing can be particularly beneficial?
Thank you, Gabriel! ChatGPT's integration with signal processing can be particularly beneficial in use cases such as audio and speech analysis, medical signal processing, video analysis, fault diagnosis in industrial systems, and IoT signal processing. These domains often involve the need for advanced analysis, intelligent decision-making, or automation, where ChatGPT's capabilities can add significant value.
Great article, Cindy! Can ChatGPT be used for real-time analysis of streaming signal data?
Thank you, Christopher! Yes, ChatGPT can be used for real-time analysis of streaming signal data. By processing the streaming data in smaller chunks or batches, the model can provide real-time insights and predictions based on the available data. This allows for timely analysis and decision-making as the signal data streams in.
Informative article, Cindy! What are some potential challenges that developers may face while implementing ChatGPT for signal processing?
Thank you, Sophie! Developers may face challenges such as acquiring and curating large-scale training datasets, fine-tuning the model for specific signal types, selecting appropriate model architectures, and optimizing computational resources. Ensuring efficient data preprocessing, balancing performance with hardware constraints, and handling real-time requirements are also challenges that need to be addressed. However, with appropriate resources and community support, these challenges can be overcome.
Cindy, in your opinion, how scalable is ChatGPT for large-scale DSP applications? Can it handle enormous amounts of data and complex processing requirements?
Scalability is one of the strong points of models like ChatGPT, Sophie. With the right infrastructure and distributed computing, it can handle large-scale DSP applications. By leveraging parallel processing and efficient data handling, the potential is there to tackle complex processing requirements.
Cindy, when fine-tuning ChatGPT, how crucial is the choice of hyperparameters? Do specific hyperparameter settings have a significant impact on the model's performance?
Hyperparameter choice is indeed crucial in fine-tuning, Aiden. Different hyperparameter settings can have a significant impact on model performance, convergence speed, and generalization. Careful tuning and experimentation with hyperparameters are necessary to achieve optimal results for specific DSP tasks.
Cindy, what are your thoughts on the ethical considerations surrounding the use of AI models like ChatGPT in DSP? Are there any specific ethical challenges to be aware of?
Ethical considerations are crucial when adopting AI models, Brooklyn. Transparency, fairness, privacy, and bias mitigation are areas that demand attention. Ensuring responsible and ethical use of AI in DSP is essential to avoid unintended consequences or harm.
Cindy, can you discuss the potential impact of ChatGPT on the accessibility of DSP technology? Will it make DSP more accessible to individuals without deep expertise in signal processing?
Absolutely, Amelia. ChatGPT and similar AI models have the potential to democratize DSP technology, making it more accessible to a wider audience. Individuals without deep expertise in signal processing can leverage these models to solve DSP challenges and innovate in their respective fields.
Well-explained article, Cindy! Can ChatGPT be seamlessly integrated into existing signal processing workflows?
Thank you, Nathan! ChatGPT can be integrated into existing signal processing workflows, but it may require careful considerations and adjustments. Ensuring compatibility with existing data formats, adapting the model's input and output interfaces, and incorporating the necessary preprocessing and post-processing steps are crucial for seamless integration. Collaborating with experts in both signal processing and AI can facilitate this integration process.
Cindy, what do you think are the key areas where the DSP community and AI researchers can collaborate to further enhance the capabilities of ChatGPT and other AI models?
Great question, Nathan! Collaboration between the DSP community and AI researchers can enhance model robustness, accuracy, and reliability in real-world DSP applications. Sharing datasets, expertise, and insights can lead to improvements in training data quality, fine-tuning techniques, and addressing domain-specific challenges.
Great job on the article, Cindy! What are some potential future enhancements or directions for ChatGPT in the field of signal processing?
Thank you, Zoe! Some potential future enhancements for ChatGPT in signal processing include improved model interpretability, better handling of rare or unbalanced signal classes, support for multi-modal signal analysis (e.g., audio-visual), and more efficient transfer learning capabilities across related signal processing tasks. Further research in these directions will contribute to expanding the practicality and potential of ChatGPT in this field.
Informative article, Cindy! Do you think ChatGPT can handle real-time anomaly detection in signal data?
Thank you, Lucas! ChatGPT can indeed handle real-time anomaly detection in signal data. By training the model on normal signal patterns and leveraging techniques like autoencoders or sequence modeling, it can effectively analyze incoming signals and identify anomalies in real-time. This can be particularly useful in applications where prompt anomaly detection and alerting are critical.
Well-done article, Cindy! Can ChatGPT adapt to signals with dynamic ranges, such as those encountered in audio or sensor data?
Thank you, Charlotte! ChatGPT can adapt to signals with dynamic ranges typically encountered in audio or sensor data. Proper data normalization techniques and defining appropriate representations or features enable the model to handle signals with varying scales. This allows for effective analysis, interpretation, and processing of such signals in signal processing tasks.
Excellent article, Cindy! How can developers address potential biases in ChatGPT when working with signal processing tasks?
Thank you, Ella! Addressing potential biases in ChatGPT is an important consideration. Developers should carefully curate and preprocess the training data to minimize biases and ensure diverse representation of signal types. Ongoing monitoring and evaluation of the model's output can help identify and mitigate biases. It's crucial to follow ethical practices, promote diversity, and involve domain experts to minimize potential biases in the signal processing outcomes.
This article has sparked my interest, Cindy! Can ChatGPT be used for signal compression or encoding techniques?
Thank you, Adam! ChatGPT can contribute to signal compression or encoding techniques. By training the model on large-scale datasets and employing architectural variations, it can potentially learn efficient representations and compression schemes for specific signal types. Incorporating perceptual coding techniques and balancing trade-offs in compression quality and computational complexity can lead to effective compression or encoding solutions.
Engaging article, Cindy! How can ChatGPT be useful in real-world applications of wireless communication signal processing?
Thank you, Christopher! ChatGPT can be useful in real-world applications of wireless communication signal processing. It can aid in tasks such as channel estimation, interference detection and mitigation, modulation classification, multiuser detection, and cognitive radio systems. The ability to analyze and process signals in real-time, coupled with its learning capabilities, makes it a valuable tool in wireless communication signal processing.
Well-written article, Cindy! How can ChatGPT contribute to real-time radar signal processing?
Thank you, James! ChatGPT can contribute to real-time radar signal processing by providing insights, predictions, or decision support based on the analyzed radar signals. By training the model on radar signal datasets and incorporating relevant techniques like beamforming, target detection, or classification, it can aid in radar system operation, target tracking, or even radar image reconstruction in real-time scenarios.
Great article, Cindy! How can ChatGPT handle the processing of signals with time-varying characteristics, such as fading channels?
Thank you, William! ChatGPT can handle the processing of signals with time-varying characteristics, such as fading channels. By incorporating techniques like adaptive filtering, time-frequency analysis, or employing recurrent neural networks, the model can effectively analyze and adapt to the changing characteristics of the signals. This enables accurate processing and interpretation even in dynamic signal conditions.
Thank you all for visiting my blog and reading my article on Revolutionizing Digital Signal Processing! I'm excited to discuss this topic with you all.
Great article, Cindy! I found it very informative and interesting to learn about ChatGPT's potential in DSP.
I agree, Michael! The advancements in AI and natural language processing are truly revolutionizing various industries, including DSP.
Cindy, your explanation of how ChatGPT can handle complex signal processing tasks was well-detailed. Thanks for breaking it down!
Thank you, Nicholas! I wanted to make sure the technical aspects were understandable to a broad audience. Do you have any specific questions regarding ChatGPT's capabilities in DSP?
Nicholas, I found your comment on Cindy's explanation to be helpful as well. It's great to have complex DSP concepts broken down into more understandable terms.
Thank you, Isabella! Simplifying complex concepts is one of the aims of my comment. I'm glad you found it helpful.
I enjoyed reading your article, Cindy. The potential for ChatGPT in real-time audio processing is intriguing. Can you share some real-world applications where this technology could be utilized?
Absolutely, Sarah! ChatGPT can be applied in areas like noise reduction, speech enhancement, echo cancellation, and even automatic music transcription. Its flexibility allows for a wide range of applications.
Cindy, in your opinion, what are the main advantages of using ChatGPT for DSP compared to traditional methods or other AI models?
That's a great question, Emma. One of the main advantages of ChatGPT is its ability to adapt and learn from large amounts of data without requiring explicit programming. This makes it highly versatile and efficient for solving complex DSP challenges.
I have some concerns about the reliability of AI models in critical signal processing tasks. How can we ensure that ChatGPT performs accurately in such scenarios?
Valid concern, Lucas. While AI models like ChatGPT have shown promising results, their performance in critical scenarios requires rigorous testing, validation, and continuous improvement. Collaborative efforts between researchers and industry professionals can help address these challenges and ensure reliability.
Cindy, I really enjoyed your article. The combination of AI and DSP opens up endless possibilities for innovation. Are there any limitations or potential drawbacks we should be aware of when using ChatGPT in DSP?
Thank you, Oliver! While ChatGPT has tremendous potential, it's important to be aware of some limitations. It might generate plausible but inaccurate responses, especially when dealing with complex or ambiguous inputs. Testing, monitoring, and human supervision are key to mitigate these challenges.
Lucas, I share your concerns. Safety and reliability are critical when deploying AI models in sensitive signal processing applications. Thorough testing, validation, and fail-safe mechanisms are essential in maintaining accuracy and avoiding potential risks.
Nathan, I'm glad you brought up safety. In critical applications, it's crucial to have fail-safe mechanisms and human oversight to prevent potential risks arising from AI models. Responsible and ethical deployment should always be a priority.