Revolutionizing Emergency Response Planning: Harnessing the Power of ChatGPT for Cutting-Edge Traffic Analysis Technology
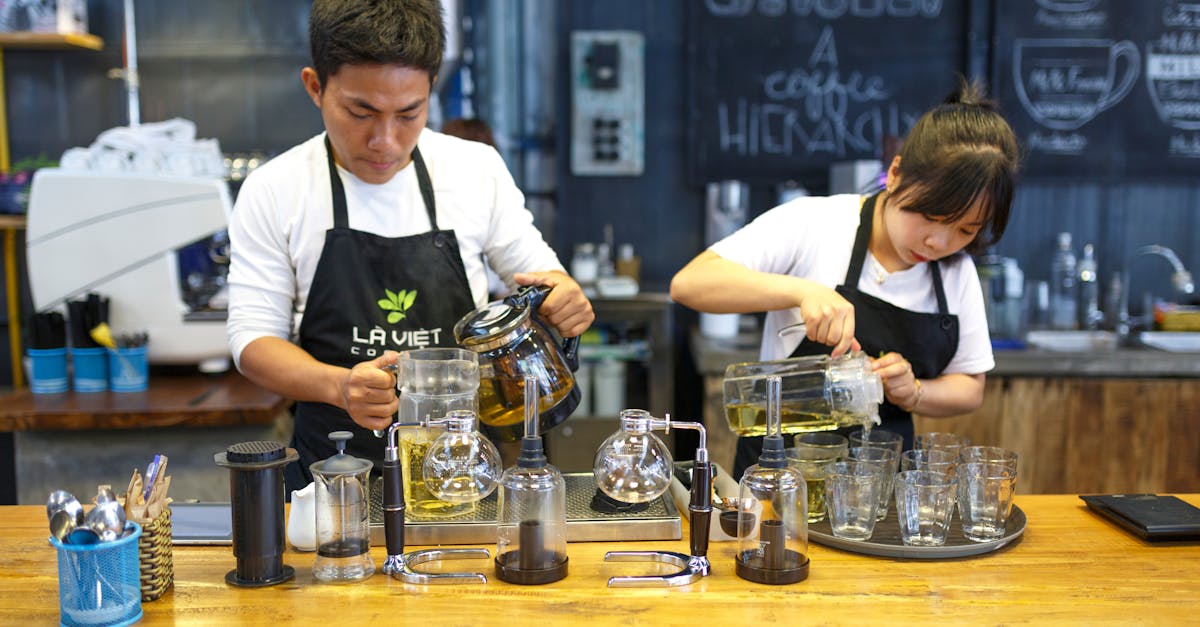
In the world of emergency response planning, every second counts. When lives are at stake, having efficient routes for emergency vehicles can make all the difference. This is where traffic analysis technology comes into play.
Traffic analysis is a method used to study and analyze traffic patterns, flow, and congestion on road networks. It involves collecting real-time and historical traffic data and using advanced algorithms to predict the best routes for emergency vehicles, ensuring swift and effective response times.
Utilizing Real-Time Data
One of the key advantages of traffic analysis technology is its ability to utilize real-time traffic data. By collecting information from various sources such as roadway sensors, GPS devices, and traffic management centers, the system can constantly update and adjust the predicted routes for emergency vehicles.
Real-time data allows the analysis system to consider and adapt to changing traffic conditions, such as accidents, construction, or congestion. By integrating this data into the emergency response planning process, dispatchers and responders can make informed decisions about the most efficient routes to reach their destinations.
Harnessing Historical Traffic Data
In addition to real-time data, traffic analysis technology also makes use of historical traffic data. This data includes information about traffic patterns at different times of the day, week, month, or year, as well as data from previous emergency response scenarios.
By analyzing historical traffic data, the system can identify recurring patterns and trends, such as rush hour congestion or areas prone to accidents. This knowledge helps emergency response planners create effective strategies and optimize routes for emergency vehicles.
Benefits for Emergency Response Planning
The usage of traffic analysis technology in emergency response planning brings several notable benefits:
- Improved response times: By predicting the best routes based on real-time and historical traffic data, emergency vehicles can reach their destinations faster, potentially saving lives.
- Efficient resource allocation: Optimal routing helps emergency response agencies allocate their resources more effectively, ensuring that vehicles are deployed where they are needed most.
- Enhanced situational awareness: Traffic analysis technology provides dispatchers and responders with up-to-date information on traffic conditions, allowing them to adapt their plans and make informed decisions.
- Reduced congestion: By directing emergency vehicles along the most efficient routes, traffic analysis technology can help minimize traffic congestion and prevent further delays.
In Conclusion
Traffic analysis technology plays a crucial role in emergency response planning, offering a systematic approach to predicting the best routes for emergency vehicles. By utilizing real-time and historical traffic data, this technology improves response times, enhances situational awareness, and aids in efficient resource allocation.
As technology continues to evolve, we can expect traffic analysis systems to become even more sophisticated, providing further benefits to emergency response planning and ultimately contributing to the overall safety and well-being of communities.
Comments:
Thank you all for your interest in my article! I'm excited to hear your thoughts and answer any questions you may have.
This is a fascinating article, Rene! ChatGPT has really opened up new possibilities for various applications. Have you considered any potential challenges in implementing this technology for traffic analysis?
Thanks, Michael! Implementing ChatGPT for traffic analysis does come with some challenges. One of them is the need for detailed training data that includes various traffic scenarios, which can be time-consuming to collect and label.
Rene, have you encountered any challenges in obtaining and maintaining the required datasets for training ChatGPT in the traffic analysis domain?
Michael, collecting and maintaining high-quality training datasets is indeed a challenge. Traffic data can vary across different regions, and obtaining representative samples without biases can be tricky. Additionally, ensuring data privacy and security is also a crucial consideration.
Rene, I agree that maintaining data privacy and avoiding biases in training datasets are critical. Have you explored any techniques to mitigate these challenges?
Michael, to mitigate biases, it's essential to ensure diversity in the training data sources. Collaborating with various city authorities, traffic management agencies, and data providers can help obtain representative datasets and minimize biases. Additionally, conducting regular audits and addressing potential biases is crucial throughout the system's development and deployment.
Rene, you bring up a good point. Ensuring the model's safety and ability to handle unexpected scenarios is crucial. How do you plan to address this challenge?
Michael, to address safety concerns and handling unexpected scenarios, a robust feedback loop is crucial. Continuously monitoring and collecting user feedback will help identify potential risks, update the training dataset, and improve the model's performance. Regular evaluations and incorporating safety mechanisms into the system will be part of the ongoing efforts.
Rene, incorporating a robust feedback loop for safety is essential. How do you plan to engage with users to gather feedback and ensure their trust in the system?
Michael, user engagement will be a key factor in gathering feedback and fostering trust. Implementing user feedback channels, conducting surveys, and collaborating with city authorities, emergency services, and other stakeholders will help address concerns and gain insights into users' experiences. Transparency about the model's capabilities and limitations will also contribute to building trust.
Rene, ensuring user safety is paramount. Integrating context-aware response filtering mechanisms sounds like a great approach. Are there any ongoing efforts to enhance model interpretability?
Michael, indeed, model interpretability is an important aspect. Ongoing research focuses on developing techniques to enhance interpretability, such as attention mechanisms, explainable AI methods, and interactive interfaces that allow users to understand the model's decision-making process. The goal is to strike a balance between accuracy and clarity in model output.
Rene, transparency about the model's capabilities and limitations is crucial for building user trust. How can transparency be effectively communicated to users and stakeholders?
Michael, effective communication of transparency can be achieved through various channels. Developing user-friendly documentation, clear guidelines, and informative visualizations that explain the model's behavior can aid in transparently communicating its capabilities and limitations. Regular updates, public presentations, and accessible platforms for questions and answers can further enhance transparency and build trust.
Great article, Rene! I'm particularly interested in how ChatGPT can be leveraged for emergency response planning. Can you provide an example of how this technology can improve existing systems?
Emily, thank you! In emergency response planning, ChatGPT can assist in assessing traffic conditions during crises, suggest optimal routes, and provide real-time updates to emergency services. This can help save valuable time and enhance coordination efforts.
Rene, that sounds very promising! By leveraging ChatGPT's capabilities, emergency services can make more informed decisions in critical situations. Do you think this technology will be scalable for large metropolitan areas?
Emily, absolutely! With advancements in computational power and optimizations in the underlying models, ChatGPT can be scaled to handle traffic analysis in large metropolitan areas. However, it's important to consider infrastructure requirements and computational constraints.
That's great to hear, Rene! As cities continue to grow, having scalable technologies like ChatGPT will be imperative for effective traffic management in emergencies. What are some limitations that should be considered with this technology?
Emily, while ChatGPT shows promise, it's important to consider its limitations. The model's responses heavily rely on the training data it has seen, so it may struggle with handling novel or out-of-distribution scenarios accurately. Additionally, it's essential to handle user queries that exploit the model's weaknesses or attempt to generate unsafe instructions.
Rene, addressing biases and ensuring diversity in training data sources are crucial. How can we ensure that the data collected accurately represents the complexities of different city traffic conditions?
Emily, accurately representing the complexities of different city traffic conditions requires thoughtful data collection strategies. Collaborating with multiple cities and traffic management authorities, covering diverse urban areas, and considering various traffic scenarios will aid in capturing the necessary intricacies. Additionally, continuous evaluation and refining of the training data will help address any potential gaps and improve accuracy.
Rene, regarding users exploiting weaknesses or generating unsafe instructions, what measures can be taken to handle such scenarios and maintain user safety?
Emily, to handle scenarios where users exploit weaknesses or generate unsafe instructions, a combination of pre-training on a diverse range of data and fine-tuning with reinforcement from human reviewers is crucial. Implementing safety checks, user validation processes, and context-aware response filtering can also help maintain user safety and prevent potential misuse.
Rene, collaborations with multiple cities and continuous evaluation of training data are crucial for data accuracy. How can we ensure these collaborations are effectively established and maintained?
Emily, effective collaborations require proactive communication, community building, and establishing data-sharing agreements among cities and entities involved in traffic management. Encouraging knowledge exchange, hosting workshops and conferences, and providing incentives for participation can help maintain long-term collaborations. Having a dedicated team that oversees the collaborations can ensure ongoing relationships and data quality.
Really enjoyed reading this, Rene! Traffic analysis is crucial for efficient city planning. Do you think ChatGPT can accurately predict traffic congestion patterns compared to traditional methods?
Thank you, Daniel! ChatGPT shows promise in predicting traffic congestion patterns accurately. However, it still requires large datasets and continuous fine-tuning to improve prediction accuracy. It's an ongoing research area.
Rene, considering the potential of ChatGPT in predicting traffic congestion, how does its accuracy compare to traditional traffic models that rely on historical data and statistical analysis?
Daniel, ChatGPT has shown promising results in predicting traffic congestion patterns. However, comparing accuracy with traditional models can be challenging, as it depends on the specific datasets, underlying statistical models, and analysis techniques used. Further research and experimentation are needed to provide conclusive insights into the comparative accuracy of ChatGPT and traditional traffic models.
Rene, thank you for your response! It's indeed a complex comparison to make. As ChatGPT continues to improve, it has the potential to outperform traditional models in accurately predicting traffic congestion patterns.
Impressive work, Rene! It's incredible how AI advancements can revolutionize emergency response planning. How does ChatGPT handle real-time data and adapt to changing traffic conditions?
Sophia, great question! ChatGPT can integrate real-time data feeds into its analysis, allowing it to adapt to changing traffic conditions. This can include weather updates, accidents, road closures, and more to provide up-to-date information for emergency response planning.
Rene, it's impressive how ChatGPT can incorporate real-time data feeds. How frequently does the model update its analysis based on new data?
Sophia, the frequency of model updates can be customized based on the specific use case and available computational resources. Ideally, the model can update its analysis in near real-time, leveraging continuous streams of incoming data. However, the exact update frequency depends on multiple factors, including processing speed, data volume, and resource allocation.
Thank you for clarifying, Rene! Considering the varying computational resources available, how can cities with limited resources benefit from incorporating ChatGPT in their traffic analysis systems?
Sophia, cities with limited resources can still benefit from ChatGPT. Optimizing computational resources, leveraging cloud computing, and adopting scalable infrastructure can help ensure efficient utilization. Collaborative efforts, such as sharing datasets and pooling resources among multiple cities, can also alleviate resource constraints while enabling the benefits of ChatGPT for traffic analysis.
Rene, thank you for your insights on transparency. In terms of computational resource allocation, are there any strategies to optimize resource usage while maintaining ChatGPT's performance?
Sophia, optimizing resource usage can be achieved through efficient model architectures, model compression techniques, and leveraging distributed computing frameworks. Prioritizing critical tasks and optimizing resource allocation based on traffic analysis requirements can also help ensure ChatGPT's performance while minimizing computational costs.