Revolutionizing Fluid Power: Harnessing the Power of ChatGPT in Technology
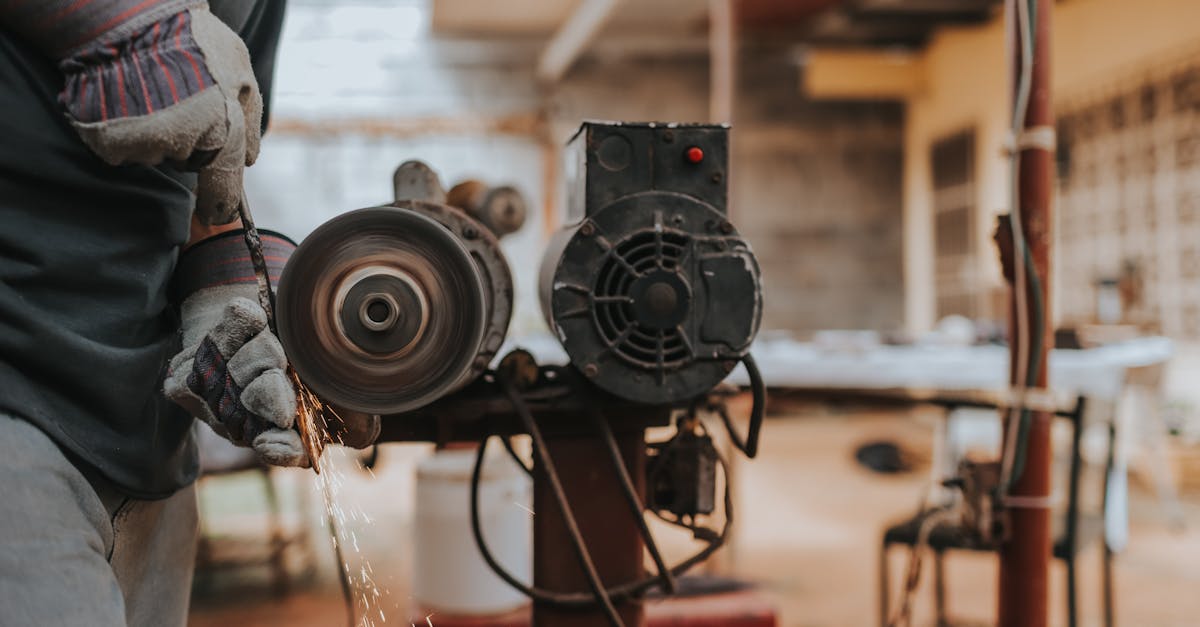
Fluid power technology, which includes hydraulic and pneumatic systems, is a vital part of many industries, including manufacturing, automotive, and aerospace. This technology relies on the transfer and control of fluids in a system to generate, control and transmit power. However, these systems can be prone to faults, malfunctions, or declines in performance over time due to wear and tear, leaks, operational stresses, among other factors. Consequently, there is a growing need for efficient and effective fault detection methodologies that can monitor fluid power systems and predict possible failures based on historical operational data.
Enter ChatGPT-4, developed by Open AI. This advanced model of the transformer-based machine learning technique has exhibited profound prowess in processing unstructured text data. But how does one utilize this language model in a seemingly unrelated domain, you may ask? Through data. Every fluid power system operation produces abundant data. This data, often regarded as just mere byproducts of operations, holds enormous potential in predictive maintenance, particularly in fault detection.
ChatGPT-4 and Fault Detection: A Technological Convergence
ChatGPT-4 can analyze vast amounts of data incredibly quickly, identifying patterns and transformations that would be impossible or exceptionally time-consuming for a human analyst. The idea behind its application in fault detection of fluid power technology is to allow the systems to continually learn from the data they generate. This learning process subsequently enables the systems to recognize when their factors deviate from the 'normal' patterns and flag potential issues in real-time, thereby minimizing catastrophic failures, derailing costly repairs, and enhancing operational efficiency.
The predictive maintenance powered by ChatGPT-4 revolves around running an algorithm on historical data collected from sensors embedded in the fluid power systems. This algorithm trains the ChatGPT-4 model to distinguish between normal operations and malfunctions or faults. Eventually, the model garners the capability to predict future system performance and potential anomalies using the most recent data.
Integrating ChatGPT-4 into Fluid Power Systems
Integrating ChatGPT-4 into fluid power systems for fault detection involves the following steps:
- Collect Data: The first step is continuous and comprehensive data collection from the fluid power system. This data lays a sturdy foundation for the training of the ChatGPT-4 model.
- Preprocess the Data: The collected data needs preprocessing to enhance the efficiency of the training process. Preprocessing may involve noise reduction, scaling, normalization, among other methods.
- Train the Model: The next step is to feed the preprocessed data into the ChatGPT-4 model for training, which involves exposing the model to different scenarios and learning to identify discrepancies in the system operations.
- Test the Model: Post-training, the model is tested on data unseen during training to assess its ability to predict faults accurately.
- Deploy in Real-Time: Assuring the reliability of the model in fault detection, it is finally integrated into the system to monitor the operation in real-time, detecting faults and anomalies.
Conclusion
Fluid power systems are complex, with various elements that could potentially fail. Incorporating machine learning techniques like ChatGPT-4 in the fault detection processes not only enhances these systems' longevity but also saves resources and maximizes efficiency. As technology unceasingly advances, the ability to monitor and predict system failures becomes ever more crucial in optimizing operations and safeguarding against unexpected breakdowns.
Comments:
Thank you all for joining this discussion! I'm excited to hear your thoughts on revolutionizing fluid power using ChatGPT in technology.
This article is fascinating! ChatGPT's potential to revolutionize fluid power is incredible. It could lead to more efficient systems and improved industrial processes.
I agree, Emily! The ability of ChatGPT to optimize fluid power systems through intelligent algorithms can bring significant advancements to various industries. It's an exciting prospect.
While the idea is intriguing, we should also consider potential risks and limitations. How do we ensure the safety and reliability of these AI-driven fluid power systems?
Great point, Sophia. Safety is indeed a critical aspect. The implementation of AI-driven fluid power systems should go hand in hand with rigorous testing, regulations, and fail-safe mechanisms to ensure reliability and minimize risks.
That's impressive, David. It would be fantastic if the article could delve deeper into such case studies, showcasing the tangible benefits achieved by integrating ChatGPT and AI into fluid power systems.
Absolutely, Sophia. I appreciate the suggestion. In future articles, I'll make sure to include more practical examples and case studies to highlight the real-world impact of AI in fluid power systems.
I agree, David. While AI can greatly enhance fluid power systems, having human oversight ensures a fail-safe in critical situations where AI algorithms may encounter unforeseen challenges.
Algorithmic bias is a critical consideration, David. We should ensure the models are trained on diverse datasets to avoid potential biases that could impact decision-making processes.
Absolutely, Sophia. Bias mitigation and transparency are crucial for building fair and inclusive AI systems that contribute positively to fluid power applications.
I completely agree, Sophia and David. Ethical considerations should be at the forefront of AI integration, taking steps to combat biases and ensure fairness in system outcomes.
I share the concern, Sophia. We must ensure that AI-driven fluid power systems are not vulnerable to malicious attacks or manipulation. Cybersecurity measures should be a top priority throughout the development and implementation process.
Absolutely, Oliver! Cybersecurity should be an integral part of integrating AI into fluid power systems. It's crucial to preemptively address potential vulnerabilities to protect against malicious activities and unauthorized control of these systems.
I totally agree, Oliver and Sophia. Cybersecurity measures should be robust and continuously updated to stay one step ahead of potential threats to AI-integrated fluid power systems.
This article fails to mention the potential impact of ChatGPT on hydraulic systems. Can AI algorithms also contribute to enhancing hydraulic performance?
Great question, Nathan! I believe AI algorithms can indeed improve the performance of hydraulic systems by optimizing fluid dynamics, reducing energy wastage, and increasing overall efficiency.
Absolutely, Nathan! AI-powered algorithms can analyze complex hydraulic systems, identify areas for improvement, and optimize control parameters, leading to enhanced performance and energy efficiency.
Is there any research showing real-world implementations of AI in fluid power systems? It would be interesting to see practical examples and their outcomes.
Great question, Michael! There have been several successful real-world implementations of AI in fluid power systems. One notable example is the optimization of hydraulic machinery in manufacturing plants, resulting in improved efficiency and reduced downtime.
That's great to hear, David! Highlighting the positive environmental impact of AI-integrated systems will play a crucial role in adoption and garnering support.
To further enhance safety, is it necessary to have a human operator in control of AI-driven fluid power systems, ensuring a failsafe in case of unforeseen circumstances or algorithmic errors?
An excellent point, Oliver. Having human operators overseeing AI-driven fluid power systems can provide an essential failsafe and added layer of safety. Proper training and clear protocols should be established to ensure effective human-AI collaboration.
Thanks for the response, David. Human supervision is vital to maintaining safety and instilling public trust in AI-driven fluid power systems.
You're welcome, Oliver. Trust and safety should be the pillars of AI-driven fluid power systems. Human supervision promotes accountability and confidence in the technology.
Could ChatGPT also assist in predictive maintenance for fluid power systems? By analyzing performance data, we may be able to identify potential issues and address them before they cause significant problems.
Absolutely, Mark! ChatGPT and other AI algorithms can leverage predictive analytics to monitor fluid power systems and predict maintenance needs. This can help prevent costly breakdowns and optimize maintenance schedules.
I believe AI-driven fluid power systems can also contribute towards sustainable practices, optimizing energy consumption and reducing environmental impact.
Absolutely, Daniel! By leveraging AI algorithms, we can achieve more sustainable fluid power systems that maximize energy efficiency, minimize waste, and support eco-friendly practices.
Predictive maintenance powered by AI can enhance the longevity of fluid power systems while reducing downtime. It's an exciting prospect!
Absolutely, Sophia. Predictive maintenance can help us transition from reactive to proactive maintenance strategies, saving resources and optimizing system performance.
ChatGPT's potential in fluid power systems is remarkable, but we should also be cautious about relying too heavily on AI. Human expertise and intuition must complement these technological advancements.
I completely agree, John. AI should augment human expertise rather than replace it. The combination of human knowledge and AI capabilities can lead to more innovative and efficient fluid power systems.
Well said, John. The synergy between AI and human expertise can unlock the full potential of fluid power systems while ensuring the necessary human touch and adaptability in complex scenarios.
I couldn't agree more, Daniel. It's important to strike the right balance between AI advancements and human involvement to maximize the benefits and mitigate potential risks.
While the focus is on AI, it's essential not to overlook the importance of ongoing research and development in traditional fluid power systems. Improvements in the core technology should go hand in hand with integrating AI.
Well said, Emily. Groundbreaking advancements in AI shouldn't overshadow the significance of continuous innovation in fluid power engineering itself.
Indeed, Emily. Predictive maintenance can significantly reduce downtime and maintenance costs by enabling proactive measures based on AI-driven insights.
Are there any challenges or limitations in implementing ChatGPT and AI algorithms in fluid power systems? I'd be interested to know more about the potential hurdles.
That's an excellent question, Lisa. While AI brings tremendous potential, challenges can include training data availability, algorithmic bias, and the need to continually update models. It's vital to address these challenges to ensure effective implementation.
Predictive maintenance can also lead to cost savings for businesses by eliminating unnecessary maintenance and repairs, optimizing resource allocation.
That's crucial, Michael. Cost savings associated with predictive maintenance can make a compelling business case for adopting AI-driven fluid power systems.
Could ChatGPT and AI algorithms help in developing more efficient pumps, valves, and actuators for fluid power systems? These components play a vital role in overall performance.
Absolutely, Lisa! ChatGPT and AI algorithms can assist in optimizing the design and performance of pumps, valves, actuators, and other critical components, resulting in more efficient and reliable fluid power systems.
I completely agree, David. AI algorithms can revolutionize the design and parameters of hydraulic systems, improving the efficiency and effectiveness of fluid power mechanisms.
That's fantastic, David! Optimized components will lead to enhanced overall performance, making fluid power systems more reliable and efficient. Exciting advancements!
Indeed, Lisa! A holistic approach to AI integration can improve every aspect of fluid power systems, from individual components to overall system design and functionality.
Sustainable fluid power systems are the need of the hour, given environmental concerns. AI can indeed play a significant role in optimizing energy consumption and reducing carbon footprints.
Human operators should be the ultimate decision-makers when it comes to AI-driven fluid power systems. Their expertise and ability to assess the broader context are crucial for safety and responsible usage.
Predictive maintenance can also help businesses improve the utilization of their assets by maximizing uptime and identifying opportunities for process optimization and efficiency gains.
Absolutely, Daniel. Enhanced asset utilization through predictive maintenance has a direct impact on productivity and cost-effectiveness, creating a competitive advantage for businesses.
Would incorporating ChatGPT and AI algorithms require significant infrastructure changes for existing fluid power systems? The cost implications might be a deciding factor for adoption.
That's a valid concern, Oliver. While there might be some infrastructure changes required, the long-term benefits, including improved performance, energy efficiency, and cost savings, can outweigh the initial implementation costs.
Human operators should indeed have the final say in AI-driven fluid power systems. Their expertise and decision-making capabilities are invaluable, especially in scenarios where unforeseen circumstances arise.
Absolutely, Michael. Human expertise and AI-driven fluid power systems together can propel us towards a greener and more sustainable future while leveraging the latest technological advancements.
Human operators can act as a safeguard, intervening when necessary, and maintaining control in critical situations where AI algorithms may lack context or face challenges.
Agreed, Sophia. Human operators play a vital role in ensuring safety and providing the necessary adaptability where AI algorithms alone may fall short.
Trust and confidence in AI-driven fluid power systems can be built by transparently communicating their capabilities, limitations, and the presence of human oversight.
Absolutely, Oliver. Transparency and communication are key in establishing trust and fostering acceptance of AI-driven technology in fluid power systems.
It's great to see how AI and ChatGPT can enhance not only the performance but also the sustainability and cost-effectiveness of fluid power systems. Exciting possibilities!
Indeed, Lisa! The integration of AI technologies like ChatGPT opens up a wide range of possibilities for advancing fluid power systems in various industries.
Apart from safety concerns, another challenge would be the scalability and compatibility of AI-driven solutions with existing fluid power systems. It might require significant modifications.
You're right, Nancy. Scalability and compatibility are crucial considerations for broader adoption. AI integration should aim for seamless integration and easy adaptability within existing systems.
Ensuring robust cybersecurity measures would also be imperative in protecting AI-driven fluid power systems from potential hacking attempts and unauthorized control.
Definitely, Daniel. Strong cybersecurity measures must be in place to safeguard AI-integrated fluid power systems against threats and ensure uninterrupted and secure operations.
The combination of AI optimization and efficient core components is a recipe for significant advancements in fluid power systems. I'm excited to witness their practical implementation.
Absolutely, Emily. The convergence of AI and traditional fluid power engineering holds immense promise for transforming industries and driving innovation.
It's essential to strike a balance between leveraging AI and continually investing in R&D to enhance the core technology itself. Both aspects contribute to the growth of fluid power systems.
You're right, Michael. AI should complement and enhance existing fluid power research and development, enabling us to push the boundaries of what's possible in the field.
The integration of AI optimization and efficient fluid power components paves the way for significant advancements in various sectors, including manufacturing and renewable energy.
Absolutely, Sophia. The sustainable development of fluid power systems demands a balance between technological progress and human expertise, ensuring environmental responsibility and safety.
Well said, Oliver. The synergy between technological advancements like AI and human judgment will be the key to unlocking the full potential of fluid power systems in addressing societal challenges.
Case studies highlighting the tangible benefits of AI-integrated fluid power systems would be valuable for various industries considering adoption. Practical examples create confidence.
Absolutely, Mark. Case studies provide concrete evidence on how AI integration can drive transformative outcomes in fluid power systems, helping stakeholders make informed decisions.
Addressing algorithmic bias and constantly updating the models is essential to ensure equitable outcomes. Diversity in datasets feeding these algorithms is crucial.
You're absolutely right, Lisa. Diversity in datasets and regular model updates are vital considerations to mitigate biases and ensure fair decision-making in AI-powered fluid power systems.
The continuous monitoring and auditing of AI algorithms used in fluid power systems can also help identify and rectify bias or unwarranted outcomes.
Absolutely, Nancy. Regular monitoring, audits, and transparency are necessary to maintain accountability and address any concerns arising from AI algorithmic decisions.
The integration of human judgment and AI capabilities can help us tackle complex challenges effectively while fostering a more sustainable and inclusive future for fluid power systems.
Indeed, Oliver. The collaboration between human operators and AI-driven fluid power systems can lead to remarkable advancements, supporting sustainable practices and driving positive change.
A combination of human expertise and AI's problem-solving capabilities can significantly improve fluid power system maintenance and optimize resource allocation.
Well said, John. The collaboration between human technicians and AI-driven predictive maintenance can create a more efficient and cost-effective approach to managing fluid power systems.
Absolutely, Daniel. The amalgamation of human and AI strengths can revolutionize fluid power maintenance, maximizing productivity and optimizing resource allocation for businesses.
Highlighting the potential cost savings through reduced maintenance and optimized asset utilization will be crucial in convincing businesses to adopt AI-driven fluid power systems.
You're absolutely right, Sophia. Demonstrating the economic benefits and long-term cost savings associated with AI-driven fluid power systems can be a compelling incentive for adoption.
Human operators bring contextual understanding, adaptability, and ethical judgment to fluid power systems. Their presence alongside AI safeguards against unwanted risks and ensures responsible usage.
Well said, Nancy. Fluid power systems encompass critical applications, and human operators play a vital role in ensuring ethical and responsible utilization of AI technologies alongside the necessary safety measures.
Addressing cybersecurity concerns will be pivotal in building trust in AI-driven fluid power systems. Continuous evaluation and adaptation to emerging threats are essential.
Absolutely, Lisa. The ever-evolving threat landscape demands ongoing cybersecurity assessments and measures to protect AI-integrated fluid power systems from potential vulnerabilities.
Definitely, Sophia. Cybersecurity must remain a top priority, and fluid power systems need to incorporate proactive measures to identify, prevent, and respond to potential threats.
The sustainable and optimized fluid power systems made possible by AI can contribute significantly to addressing climate change and achieving global environmental goals.
You're absolutely right, Oliver. AI-driven fluid power systems can play a key role in promoting sustainability and driving positive environmental impact across various sectors.
The responsible adoption of AI technologies in fluid power systems, aligned with sustainability objectives, can help us move towards a greener and more energy-efficient future.
Well said, John. The integration of AI in fluid power systems should prioritize sustainability as a core objective, enabling us to transition to a more environmentally conscious and efficient paradigm.
Human operators' ability to adapt and respond to unexpected circumstances adds an extra layer of safety and reassurance in critical fluid power operations.
Absolutely, Sophia. Human adaptability and quick decision-making remain crucial in critical scenarios, where AI algorithms may face challenges or encounter unprecedented situations.
Human operators can provide the necessary intuition and judgment to fluid power systems, ensuring they remain responsive and can adapt to changing circumstances.
Thank you all for your valuable insights and engaging in this discussion. Your contributions have been thought-provoking and highlight the exciting possibilities of AI in revolutionizing fluid power systems.
Thank you, David, for initiating this discussion. It has been a pleasure exchanging ideas and learning from other participants.
Thank you, David, for moderating this discussion and for providing informative responses. It was great to hear diverse perspectives on the potential of AI in the fluid power domain.
Thanks, David, for facilitating this engaging conversation. It has been enlightening to hear different viewpoints and delve into the intricacies of AI in fluid power systems.
This article on harnessing the power of ChatGPT in revolutionizing fluid power is truly fascinating! It's amazing how technology continues to advance and improve various fields.
Absolutely, Michael! The potential applications of ChatGPT in fluid power are vast. It could lead to more efficient systems and exciting innovations.
I agree with both of you. The integration of AI language models like ChatGPT can greatly enhance the capabilities and performance of fluid power systems.
As an engineer working with fluid power, I'm eager to explore how ChatGPT can optimize our systems. It's always exciting to embrace technological advancements in our field.
Thank you all for your positive feedback! As the author of this post, I'm thrilled to see the enthusiasm for integrating ChatGPT into fluid power technology.
I'm not sure how AI language models can be applied in fluid power. Can anyone provide concrete examples?
Sure, Ryan! AI language models like ChatGPT can analyze vast amounts of data to optimize fluid power efficiency. They can assist in predictive maintenance, fault diagnosis, and even suggest system improvements based on analyzed patterns.
Ryan, consider this—by leveraging ChatGPT, engineers can develop virtual prototypes and run simulations to test different fluid power system designs quickly and cost-effectively, providing valuable insights before physical implementation.
I'm concerned about the reliability and safety of incorporating AI into critical systems like fluid power. Can AI models like ChatGPT handle complex scenarios with certainty?
Valid concern, Olivia. While AI language models can excel in specific tasks, they still require human supervision. In fluid power, AI can aid decision-making, but human expertise ensures safety measures and resolves unforeseen circumstances.
I think this integration presents exciting opportunities, but we should also be cautious of potential biases in AI models like ChatGPT. How can we ensure fairness and avoid reinforcing existing discrimination?
Great point, Sophia! Bias mitigation is crucial in AI development. It's essential to have diverse datasets and continuous monitoring while training models like ChatGPT to minimize biases and promote fairness.
As a fluid power enthusiast, I'm curious about the feasibility of integrating ChatGPT into existing systems. Will it require substantial modifications or can it be easily implemented?
Tyler, implementing ChatGPT in existing systems may vary. Some integration may require modifications in data interfaces or minor hardware adjustments, while others might require more substantial changes to leverage its full potential.
I find this discussion intriguing. Can ChatGPT also contribute to energy efficiency in fluid power systems?
Absolutely, Joseph! ChatGPT can optimize fluid power systems by identifying energy-saving opportunities, recommending control strategy improvements, and assisting in the design of efficient components.
With the integration of AI language models like ChatGPT, do you think it will reduce human employment opportunities in the fluid power industry?
Emma, while AI can automate certain tasks, it also creates new opportunities. It can augment human capabilities, allowing engineers to focus on more complex challenges. Human expertise will always remain relevant.
This article overlooks cybersecurity concerns. Implementing AI in fluid power systems could potentially introduce vulnerabilities and expose risks. How can we address this issue?
Great point, William! Cybersecurity is of utmost importance. Incorporating AI should go hand in hand with robust security measures. Regular audits, threat modeling, and data encryption are essential to mitigate potential risks.
I wonder if ChatGPT will be accessible to small-scale companies in the fluid power industry. Will the cost of implementation be reasonable for them?
Sophie, as AI technology progresses, the cost of implementation tends to decrease. While large-scale implementation might be costly initially, it's possible that affordable alternatives or specialized services will be available for small-scale enterprises.
ChatGPT can be a valuable tool in fluid power, but we should also consider the ethical implications. Who should be held accountable if ChatGPT leads to a system failure or decision with negative consequences?
Liam, accountability is crucial. Responsibility ultimately lies with the engineers and organizations implementing AI like ChatGPT. Strict testing, effective regulations, and clear protocols are necessary to ensure safety and assign accountability.
I can see the potential of AI in fluid power, but what kind of collaboration would be required between fluid power experts and AI developers to optimize its integration?
Grace, close collaboration between fluid power experts and AI developers is paramount. Clear communication channels, shared domain knowledge, and interdisciplinary teamwork will contribute to the successful integration and optimization of AI in fluid power.
While AI seems promising, I'm concerned about the learning curve for engineers to effectively use ChatGPT for fluid power systems. Will extensive training be required?
Valid concern, Daniel. Engineers will need to become familiar with AI concepts and tools specific to fluid power. Training programs, workshops, and online resources can help bridge the learning gap for effective utilization of ChatGPT.
ChatGPT in fluid power systems could bring tremendous benefits, but what about the privacy of sensitive data? How can we ensure the confidentiality of such information?
Privacy is crucial, Olivia. Implementing data protection measures like anonymization, access controls, and encrypted communication can ensure the confidentiality of sensitive information processed by ChatGPT.
AI integration in fluid power is exciting, but we also need to consider user acceptance. How can we ensure that engineers trust and embrace AI solutions wholeheartedly?
Sophia, fostering trust is vital. Transparent explainability, gradual integration, user feedback incorporation, and demonstrating success through pilot projects can help gain engineers' trust in AI systems like ChatGPT.
The idea of integrating AI like ChatGPT into fluid power seems promising, but what challenges might arise during the development or deployment stages?
Ethan, several challenges can arise. These may include data availability, reliability, interpretability of AI decisions, technical complexities, and time required for system integration. Addressing them necessitates collaborative efforts and comprehensive planning.
It's intriguing to consider how AI like ChatGPT can assist in fluid power innovation. However, what potential legal or regulatory hurdles might need to be overcome?
Natalie, legal and regulatory aspects require attention. Adhering to data privacy laws, industry standards, certification requirements, and ensuring compliance with existing regulations are essential factors during the development and deployment of AI technology like ChatGPT.
This article has piqued my interest! I'd love to learn more about successful real-world implementations of ChatGPT in fluid power. Are there any notable examples?
Oliver, examples of ChatGPT implementation in fluid power are emerging. Many companies are exploring AI for predictive maintenance, energy optimization, and dynamic control. However, specific case studies are limited at this early stage.
Will the use of AI like ChatGPT in fluid power require significant computational resources? Will it be feasible for smaller companies with limited infrastructure?
Emma, while AI models like ChatGPT are computationally demanding, cloud-based solutions and specialized hardware options are increasingly accessible. Smaller companies can leverage these services without massive infrastructure investments.
I appreciate the potential benefits of using ChatGPT in fluid power, but are there any specific limitations or drawbacks engineers should be mindful of?
Good question, Benjamin. AI models like ChatGPT may encounter limitations such as data biases, inability to handle certain edge cases, and potential over-reliance on AI solutions. Engineers should strive for a balanced approach and augment AI with human expertise.
This discussion has been enlightening. ChatGPT has tremendous potential, but how can companies ensure a smooth transition during the adoption of AI in fluid power systems?
Emily, a smooth transition requires careful planning, starting with identifying areas where AI can add value in fluid power systems. Gradual integration, employee training, pilot projects, and a supportive company culture all play a crucial role.
I'm curious about the potential cost savings by integrating AI in fluid power with ChatGPT. Can it lead to substantial economic benefits?
Matthew, AI integration can indeed lead to significant cost savings. Enhanced system efficiency, reduced maintenance costs, optimized energy consumption, and improved overall productivity can positively impact the economics of fluid power systems.
While the integration of AI like ChatGPT in fluid power offers immense possibilities, what steps can be taken to ensure the technology is accessible to all, even in developing regions?
Sophia, ensuring accessibility requires equitable distribution of resources and knowledge transfer. Collaborations, open-source initiatives, and capacity building can help make AI technology like ChatGPT accessible in developing regions.
The potential of ChatGPT in fluid power sounds transformational, but are there any foreseeable risks that engineers should be mindful of?
Daniel, potential risks can include data privacy breaches, reliability concerns, challenges in interpreting AI decisions, and dependency on technology. Engineers should be mindful of these risks to ensure responsible and safe adoption of ChatGPT in fluid power.