Revolutionizing Frame Relay: Harnessing the Power of ChatGPT in Technology
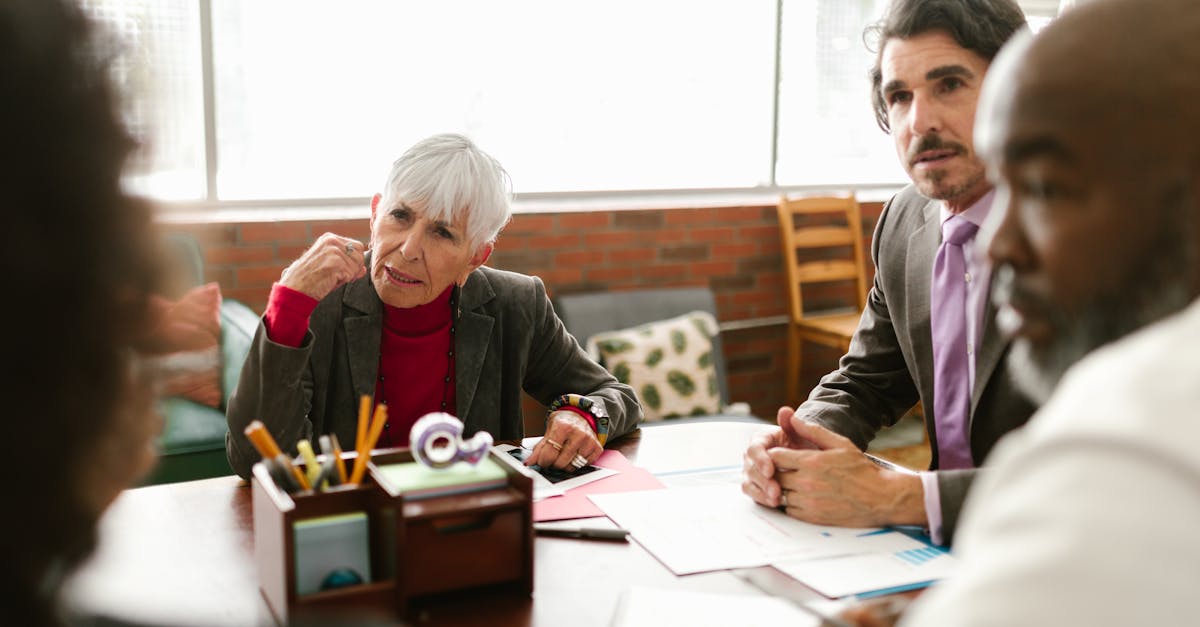
Frame Relay is a highly efficient data transmission technique used in telecommunication networks. As a packet-switching protocol, Frame Relay is instrumental in connecting remote Local Area Networks (LANs) over a Wide Area Network (WAN) framework. Despite the advent of advanced technologies, Frame Relay remains a staple for businesses due to its relative simplicity, cost effectiveness, and reliability. When it comes to effective network monitoring and analysis, ChatGPT-4, powered by the advanced language Artificial Intelligence (AI) model of OpenAI, presents immense potential.
Why ChatGPT-4 for Network Monitoring?
ChatGPT-4 utilizes Machine Learning (ML) techniques to understand, learn, and predict network patterns. What sets it apart is its natural language processing capabilities that can provide easy-to-understand reports, improve decision-making processes, and subsequently enhance network monitoring.
Frame Relay Network Monitoring through ChatGPT-4
The use of ChatGPT-4 for monitoring Frame Relay networks would involve capturing and analyzing the Frame Relay parameters like Frames, Logical Connection Identifier (LCI), Forward Explicit Congestion Notification (FECN), and Backward Explicit Congestion Notification (BECN).
1. Frame Analysis
A frame is the central component of Frame Relay defined by the starting and ending flags, with the frame header and payload in between. ChatGPT-4 can count the number of frames flowing through the network in a specific time frame, providing valuable data about the network load.
2. Logical Connection Identifier (LCI) Pattern Recognition
LCI is a unique identifier for data frames. Successful monitoring involves identifying potential LCI collisions. Collision occurs when two devices inadvertently use the same LCI, causing data transmission failure. ChatGPT-4 can help prevent such collisions by identifying patterns and suggesting changes.
3. Forward Explicit Congestion Notification (FECN) Monitoring
The FECN is a bit in the Frame Relay frame header that warns about potential network congestion. ChatGPT-4 can monitor and understand these signals and provide alerts if there are continuous FECN indications, helping to recognize and resolve potential network latency.
4. Backward Explicit Congestion Notification (BECN) Monitoring
Like FECN, the BECN warns about network congestion, but it does so in the opposite direction. Continuous BECN suggests congestion at the receiving end, which ChatGPT-4 can monitor and alert about to facilitate network optimization.
Improving Network Efficiency with ChatGPT-4
By incorporating ChatGPT-4's AI predictive analysis in monitoring Frame Relay parameters, businesses can significantly improve their network efficiency. Continuous monitoring and predictive maintenance can reduce network downtime and congestion, enhance data flow, and ultimately improve business operations.
Conclusion
With the power of AI through ChatGPT-4, businesses have the unique opportunity to leverage the prowess of real-time monitoring for their Frame Relay network. As this AI model evolves, its accuracy and usefulness in maintaining optimal network health and efficiency will only continue to grow, thereby redefining the landscape of network management.
Comments:
Great article! ChatGPT has indeed revolutionized the way we communicate and collaborate. Its potential in technology is immense.
Absolutely, Sarah! ChatGPT has opened up exciting possibilities in various fields, including technology advancement.
Thank you both for your kind words! It's amazing to witness the impact ChatGPT is having on technology. Feel free to share any personal experiences or thoughts you have.
I have mixed feelings about ChatGPT. While it has potential, there are concerns about its ability to generate misleading or biased information. We need to be cautious when relying on AI for important tasks.
I agree, Kevin. AI advancements like ChatGPT require responsible use and thorough scrutiny. It's crucial to address the limitations and potential risks associated with such technologies.
Valid concerns, Kevin and Emily. AI models like ChatGPT still have certain limitations that need to be addressed. Continued research and responsible use can help mitigate potential risks.
I've been using ChatGPT for my technology projects, and it has been a game-changer. It greatly assists in ideation and problem-solving. Highly recommend it!
Oliver, do you find any challenges while working with ChatGPT? I'm curious about its practical implications in real-world scenarios.
Hey Natalie! While ChatGPT is impressive, it sometimes generates responses that require manual filtering. Also, it's important to validate the information it provides as it can occasionally be inaccurate.
Thanks for sharing your experience, Oliver. It's vital to maintain a critical approach when using AI models. Proper validation and filtering can help ensure accurate and reliable results.
The potential of AI in technology is fascinating. With ongoing advancements in models like ChatGPT, we're witnessing a revolution that will reshape industries and enhance efficiency.
Indeed, Jason! AI-powered tools like ChatGPT have the power to transform business processes, automate tasks, and drive innovation in technology.
Jason and Christina, your insights are spot on! The transformative impact of AI in technology is just the beginning. We can expect even more advancements to revolutionize various industries.
While AI like ChatGPT brings numerous benefits, it's also crucial to address the potential ethical implications. We need to ensure AI is developed and utilized in a responsible and unbiased manner.
Absolutely, Irene! Ethical considerations should remain at the forefront of AI development and deployment. Ensuring fairness, transparency, and inclusivity are essential for responsible use.
Can someone explain how ChatGPT can specifically benefit the technology industry? I'd love to understand the practical use cases.
Brian, one prominent application of ChatGPT in the technology industry is customer support. It can assist in providing efficient and accurate responses to customer queries, enhancing the overall experience.
Excellent point, Alice! ChatGPT can significantly improve customer interactions by offering instant assistance and resolving common queries, freeing up human support agents for complex issues.
That's interesting, Alice and Dena. It seems like ChatGPT can not only enhance customer support but also improve productivity by automating routine tasks.
Absolutely, Brian! By automating repetitive tasks, ChatGPT allows professionals in the technology industry to focus on more complex and strategic initiatives, thereby boosting overall productivity.
I believe AI models like ChatGPT have immense potential, but we should ensure they don't replace human creativity and critical thinking. Balancing AI assistance with human expertise is crucial.
Well said, Liam! AI models like ChatGPT should always be seen as tools that augment human capabilities rather than replace them. Combining AI's efficiency with human creativity can lead to remarkable outcomes.
As an AI enthusiast, I find the continuous evolution of models like ChatGPT incredibly exciting. It sparks curiosity and brings about endless possibilities in technology innovation.
Grace, I share your excitement! The dynamic nature of AI advancements keeps pushing the boundaries of what we can achieve in technology. The potential for innovation and positive change is immense.
While there's much to celebrate about AI advancements, we must also address the potential job displacement it could cause. Striking a balance between automation and employment opportunities becomes crucial.
Valid concern, Daniel. The impact of automation on employment is an ongoing discussion. However, it's important to note that AI can also create new jobs and open up opportunities in emerging fields.
You're right, Dena. While some jobs may be replaced, AI advancements also stimulate the creation of new industries and job roles, requiring a shift in skillsets and continuous learning.
AI models like ChatGPT have the potential to enhance accessibility in technology. By providing real-time assistance and information, it can bridge gaps and make tech more inclusive.
Well said, Sophia! Accessibility is a crucial aspect, and AI can play a significant role in breaking barriers and ensuring technology is accessible to a wider range of people.
While AI models have their limitations, the progress made with ChatGPT is undeniable. It's exciting to think about the future possibilities and discoveries it can enable in the field of technology.
Indeed, Emma! The advancements AI models like ChatGPT bring pave the way for groundbreaking research, innovation, and new possibilities. The future of technology holds immense promise.
I appreciate the practical applications of ChatGPT discussed in this article. It's reassuring to know that AI models like this are actively contributing to the advancement of technology.
Thank you, David! It's a pleasure to see how ChatGPT and similar AI models are making a positive impact in various technological areas. The contributions they bring are truly exciting.
As AI continues to evolve, it's crucial to prioritize ethical development and user privacy. While ChatGPT offers great potential, maintaining trust and ensuring responsible practices are imperative.
I fully agree, Rachel. Incorporating ethical guidelines and privacy protection must be at the forefront when leveraging AI models like ChatGPT. Maintaining user trust is paramount for sustained adoption.
Absolutely, Rachel and Ethan! Ethical considerations, user privacy, and data protection are integral aspects that must be addressed to build reliable and trusted AI systems.
The application of AI models like ChatGPT in training and education is also promising. It can assist learners by providing immediate feedback and personalized guidance.
Great point, Mark! AI-based educational tools can enhance learning experiences, adapt to individual needs, and offer valuable insights, taking education to new heights.
I find it fascinating how AI models like ChatGPT can assist researchers by analyzing vast amounts of data quickly. It speeds up the discovery process and enables new breakthroughs.
Indeed, Robert! AI's ability to process and analyze enormous data sets is instrumental in accelerating scientific research and facilitating discoveries that would otherwise be time-consuming or even impossible.
While the potential for AI is immense, we must ensure we build AI models like ChatGPT with diverse, representative datasets, to avoid reinforcing biases and promoting inclusivity.
Spot on, Sophie! The quality and diversity of training data are crucial to address biases. By actively focusing on inclusivity and diversity, we can create AI models that benefit everyone.
I've been using ChatGPT for brainstorming and idea generation, and it has been incredibly helpful. It offers new perspectives and sparks creativity.
That's wonderful to hear, Megan! ChatGPT's ability to assist in generating ideas is undoubtedly an invaluable asset for professionals across various fields.
I have concerns about the energy consumption of AI models like ChatGPT. The environmental impact of large-scale AI deployments needs careful consideration.
Anthony, you raise a valid point. Minimizing the energy consumption of AI models, exploring energy-efficient alternatives, and promoting sustainable practices are important factors to mitigate environmental impact.
As technology evolves, addressing cybersecurity concerns becomes even more critical. While AI models offer immense possibilities, they can also be vulnerable to attacks and require robust defenses.
Absolutely, Amy! Bolstering cybersecurity measures and staying ahead of potential risks are vital in safeguarding AI models like ChatGPT and maintaining data integrity.
AI advancements like ChatGPT are undoubtedly exciting, but we must remain vigilant in preserving privacy rights. Striking the right balance between innovation and data protection is crucial.
Well said, William! Privacy considerations must be at the core of AI advancements, and proactive measures should be taken to protect user data and ensure privacy rights are respected.
The ethical implications of AI development, such as the potential for deepfakes, should also be considered. The responsible use of AI models like ChatGPT is crucial in preventing misuse.
Absolutely, Lauren! Addressing the ethical implications of AI, including the threat of deepfakes, requires collaboration between researchers, developers, and policymakers to establish guidelines and practices that prevent misuse.
Although AI models have come a long way, there's still a need for ongoing research to improve their interpretability and explainability. Transparency is key for building trust.
Well said, Sophie! Advancing AI models' interpretability and ensuring they provide transparent explanations for their output will enhance trust and adoption in various domains.
ChatGPT has undoubtedly made significant strides in natural language processing, but at times, it can produce responses that lack context or fail to address the user's intent accurately.
You're right, Max. While AI models like ChatGPT have made remarkable progress, challenges in understanding nuanced context and accurately addressing user intent still remain. Continued research is necessary to improve these aspects.
To truly harness the power of AI models like ChatGPT, we need to ensure access and affordability. Widening access and reducing barriers will empower more individuals and organizations to benefit from such technologies.
Absolutely, Ava! Promoting accessibility and affordability of AI models like ChatGPT is essential to unlock their potential benefits for a more diverse range of users and amplify their impact.
I've heard concerns about the potential for AI models to reinforce existing biases. It's essential to address this issue and ensure fairness in both model development and real-world applications.
You're absolutely right, Henry. AI models should be developed with a focus on fairness, eliminating biases, and promoting inclusivity. Continual evaluation and improvement are necessary to minimize any unintended reinforcement of biases.
AI models like ChatGPT can be powerful tools, especially in research and data analysis. They help uncover patterns and insights that may not be immediately apparent to humans.
Well said, Lily! AI models excel at analyzing vast amounts of data and spotting patterns that humans might miss. Leveraging their capabilities can lead to impactful discoveries and insights in research.
One aspect to consider with AI models like ChatGPT is their potential to learn from biased data. Efforts must be made to ensure AI systems are trained on diverse and representative datasets.
Absolutely, Anna! Addressing biases in training data and promoting diversity and inclusivity are crucial factors to prevent AI models from perpetuating harmful stereotypes or biased information.
ChatGPT's natural language processing capabilities are impressive. It can understand and respond to queries in a conversational manner, making it a valuable tool for numerous applications.
Indeed, Leo! The ability of ChatGPT to engage in natural language conversations makes it highly versatile and applicable across a wide range of domains and industries.
AI models like ChatGPT have immense potential in healthcare. They can assist in clinical decision support systems, analyzing medical data, and offering insights for improved diagnostics and treatment.
That's a great point, Grace! ChatGPT's applications in healthcare hold significant promise, from supporting medical professionals in decision-making to aiding in data analysis for more accurate diagnoses and personalized treatment plans.
The progress made with AI models like ChatGPT is remarkable. It's exciting to think about the endless possibilities and potential discoveries that lie ahead in technology.
Absolutely, Elliot! The advancements in AI models are truly awe-inspiring. The future holds immense potential for groundbreaking discoveries and transformative developments across various technological domains.
While AI models can automate tasks and improve efficiency, their limitations must be acknowledged. They are not substitutes for human judgment, creativity, or empathy.
Well said, Benjamin. AI models like ChatGPT are tools that enhance human capabilities, but they should never replace the unique qualities humans bring: empathy, intuition, and critical thinking.
AI advancements need to be accompanied by responsible governance frameworks and regulations. This will ensure that AI models like ChatGPT are deployed ethically, protecting user rights and minimizing harm.
Absolutely, Emma! Responsible governance and appropriate regulations play a crucial role in promoting ethical and safe deployment of AI models like ChatGPT, safeguarding the interests of both users and society at large.
The collaboration between humans and AI models like ChatGPT can unlock innovation and efficiency. Striking a balance and leveraging the strengths of both can lead to remarkable outcomes.
Well said, Sophia! The collaboration between humans and AI models offers huge potential. By combining human expertise with AI's capabilities, we can achieve outcomes that were once unimaginable.
The ability of AI models like ChatGPT to assist in documentation and content generation is truly remarkable. It saves time and streamlines workflows.
Absolutely, Oliver! AI models like ChatGPT can significantly enhance productivity by automating aspects of content generation and documentation, allowing professionals to focus their time and energy on other critical tasks.
AI models like ChatGPT can facilitate language translation and overcome communication barriers, making it easier to connect and collaborate across diverse cultures and languages.
You're absolutely right, Sophia! The language translation capabilities of AI models like ChatGPT have immense potential in fostering cross-cultural understanding, global collaboration, and breaking language barriers.
While AI models can assist humans, it's important to ensure transparent decision-making processes. Understanding how AI reaches its conclusions is crucial, especially in critical domains like healthcare.
Well said, Ella! In domains where AI models impact critical decisions, interpretability and transparency are vital. Users must have a clear understanding of how the model arrives at its conclusions.
The potential applications of AI models like ChatGPT in the gaming industry are also intriguing. They can enhance virtual characters, improve game narratives, and offer dynamic player experiences.
Absolutely, Leo! AI models like ChatGPT can revolutionize the gaming industry by creating more immersive and interactive experiences, enriching game narratives, and enhancing virtual characters with lifelike interactions.
The possibilities of ChatGPT in content personalization are fascinating. It can help tailor information and recommendations to individual preferences, improving user experiences.
Indeed, Eva! AI models like ChatGPT can enable personalized content delivery, ensuring users receive information and recommendations that align with their preferences and interests, enhancing overall user experiences.
I'm impressed by the progress AI models have made in natural language understanding. ChatGPT's ability to comprehend human queries and generate relevant responses showcases its potential.
Thank you, Aaron! Natural language understanding is a critical aspect of AI models like ChatGPT. Their ability to grasp human queries and provide contextually appropriate responses indeed showcases the progress made in this field.
The ongoing development and fine-tuning of AI models like ChatGPT are crucial. It ensures these models remain relevant, accurate, and capable of meeting evolving user needs.
Absolutely, Julia! Continued research, development, and refinement of AI models like ChatGPT are essential to address user requirements, incorporate improvements, and keep up with the ever-changing technological landscape.
Completely agree, Dena. The ability of ChatGPT to learn and adapt to network behavior has the potential to streamline network management and optimize resource allocation.
The potential for AI models like ChatGPT to assist in creative endeavors, such as art and music, is intriguing. They can inspire and collaborate with humans, unlocking new artistic possibilities.
Well said, Daniel! AI models like ChatGPT can collaborate with human artists and musicians to drive creativity, offering new perspectives and expanding the boundaries of artistic expression.
AI models like ChatGPT have the potential to aid in data analytics, helping businesses gain valuable insights from their vast datasets, leading to data-driven decision-making.
Absolutely, Lucy! AI models like ChatGPT can extract valuable insights from large datasets, empowering businesses to make informed decisions based on data analysis, boosting efficiency and competitiveness.
The evolution of AI models like ChatGPT is truly fascinating. The advancements we witness in technology today will shape the world we live in tomorrow.
Thank you, Jack! The rapid evolution of AI models like ChatGPT brings us closer to a future where technology acts as a catalyst for positive change, empowering individuals and advancing societies.
AI models like ChatGPT have immense potential in research areas like drug discovery and genomics. They can assist scientists in analyzing complex data to accelerate scientific breakthroughs.
Absolutely, Samantha! AI models like ChatGPT can be valuable collaborators for researchers in domains like drug discovery and genomics, helping analyze vast amounts of data and accelerating scientific advancements.
AI models like ChatGPT have already made significant advancements, and I look forward to seeing how they will continue to shape the future of technology.
Thank you, Ethan! AI models like ChatGPT have indeed made great strides, and the possibilities that lie ahead continue to be exciting. The future of technology is full of promise.
Thank you all for reading my article on Revolutionizing Frame Relay! I'm excited to hear your thoughts and insights.
Great article, Dena! I've always been curious about the potential of ChatGPT. It's amazing to see how it can revolutionize technologies like Frame Relay.
I agree, Michael. I work in the networking field, and the integration of ChatGPT with Frame Relay could have significant impacts on efficiency and speed.
Absolutely, Sarah! The ability of ChatGPT to optimize routing decisions in Frame Relay by analyzing real-time data can lead to improved network performance.
Sarah, as someone who manages network operations, I can see ChatGPT streamlining the troubleshooting process. Identifying and resolving issues could be faster and more efficient.
While it sounds promising, I wonder how ChatGPT handles complex network configurations. Can it adapt to challenging scenarios?
That's a valid concern, James. ChatGPT has shown promising adaptability by utilizing deep learning techniques and being trained on diverse network configurations.
Thanks for clarifying, Dena. It's impressive to see how ChatGPT can adapt to complex network environments. Exciting times for the networking industry!
I appreciated how you explained the potential cost savings through ChatGPT's predictive analysis. It's a convincing argument for incorporating it into existing network infrastructures.
The security implications of integrating ChatGPT into Frame Relay also need to be considered. How can we ensure data privacy and prevent potential vulnerabilities?
Great question, Jason. Data privacy is crucial, and ChatGPT's integration with Frame Relay needs robust encryption methods to ensure the protection of sensitive information.
Dena, I'm curious about the scalability of ChatGPT. Can it handle large-scale networks without compromising performance?
Good point, Dena. Robust encryption is a prerequisite to ensure secure integration. It would also be wise to conduct thorough penetration testing to identify any potential vulnerabilities.
Penetration testing is indeed crucial, Jason, to identify any potential vulnerabilities that could arise from integrating ChatGPT into Frame Relay networks.
Great article, Dena! I'm thrilled to see the potential of ChatGPT for Frame Relay. It could truly revolutionize the way we approach network optimization.
Absolutely, Joseph. The optimization capabilities of ChatGPT could lead to significant improvements in network efficiency and reduce maintenance costs.
Dena, could you provide more details on the potential cost savings? It's an intriguing aspect of incorporating ChatGPT into network infrastructures.
Certainly, Michael. By using predictive analysis and leveraging historical data, ChatGPT can identify patterns, predict network behaviors, and optimize resource allocation, resulting in potential cost savings.
Thank you, Dena! The potential for cost savings makes ChatGPT an even more compelling solution for network optimization.
Thanks for explaining, Dena. The potential cost savings are indeed a compelling aspect to consider when exploring the implementation of ChatGPT.
Absolutely, Dena. Penetration testing ensures the identification and mitigation of potential vulnerabilities, providing a more secure integration of ChatGPT.
Indeed, Jason. Robust security measures are vital when integrating ChatGPT into Frame Relay networks to maintain data privacy and protect against potential threats.
Precisely, Emily. A thorough security assessment should be conducted during the integration process to address any vulnerabilities and ensure robust data protection.
I found it fascinating how ChatGPT can use natural language processing to understand network configurations. A promising approach for simplifying network management.
I enjoyed reading about the real-world examples you provided, Dena. It helped me understand how ChatGPT can be practically applied in Frame Relay technology.
Is ChatGPT compatible with other networking protocols apart from Frame Relay? Could it be applied to technologies like Ethernet or MPLS?
Absolutely, Robert. ChatGPT's capabilities aren't limited to just Frame Relay. It can be trained on various networking protocols and applied accordingly.
That's great to hear, Daniel. It opens up possibilities for ChatGPT to enhance a wide range of networking technologies.
The potential of ChatGPT to learn from network behavior and proactively optimize Frame Relay is truly exciting. It could revolutionize network management!
Dena, I'm curious about the implementation process. How complex is it to integrate ChatGPT with existing Frame Relay networks?
Exactly, Megan. Understanding the integration complexity would be valuable in assessing the feasibility of implementing ChatGPT in existing networks.
Robert, I believe the adaptability of ChatGPT allows it to be utilized in various networking protocols, including Ethernet and MPLS.
Thanks, Emily. It's fascinating to see how ChatGPT can transcend specific protocols, making it a versatile solution in the networking industry.
Agreed, Robert. The complexity of integration can impact both the feasibility and cost-effectiveness of incorporating ChatGPT into existing networks.
Megan, I believe the implementation process can vary depending on the existing network infrastructure and the complexity of the Frame Relay setup.
I'm curious if there are any limitations to ChatGPT's capabilities in the context of Frame Relay. Are there scenarios where it may not be effective?
That's a good question, Lucy. While ChatGPT has shown promising adaptability, there may be niche scenarios where specialized solutions could be more effective.
Definitely, Dena. It's essential to analyze specific network requirements and limitations to determine if ChatGPT is the optimal choice for network optimization.
Thanks for addressing my question, Dena. It's important to evaluate specific use cases and consider alternative solutions where ChatGPT may not be a perfect fit.