Revolutionizing Graph Databases: Harnessing the Power of ChatGPT
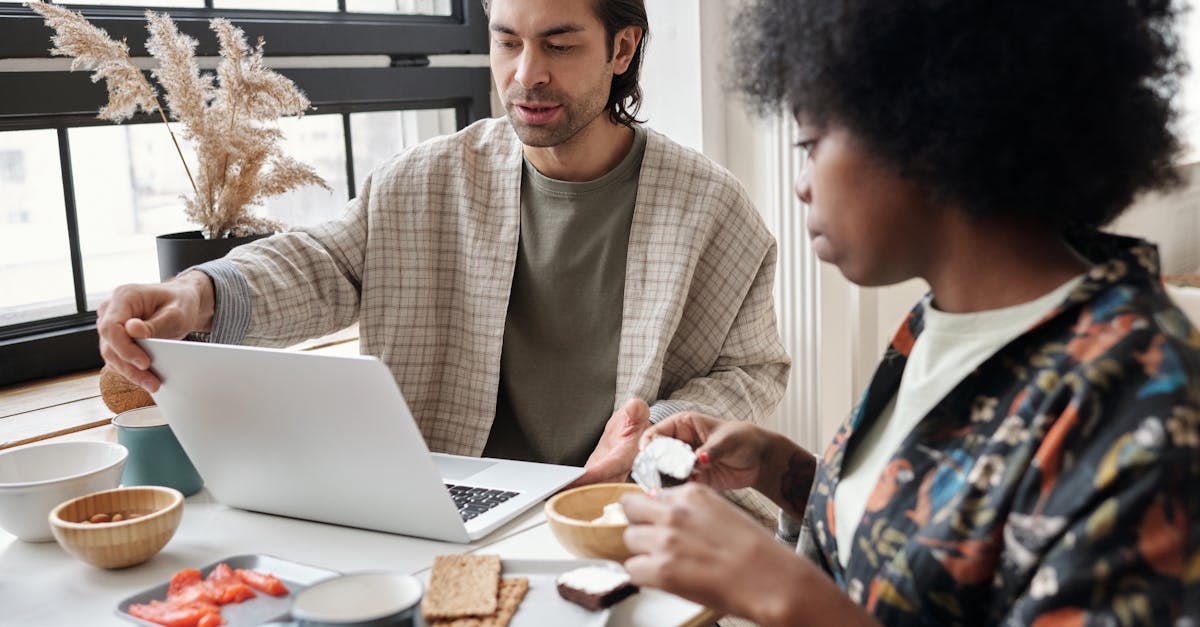
Understanding proprietary information is a crucial aspect of any business. With the advent of technology, much data has been digitized, accumulating vast amounts of complex data about various entities. Chatbots: amongst the buzz the word has created, is an AI-based software designed to interact with humans in their natural languages. These chatbots are usually converse via auditory or textual methods, and they can effortlessly mimic human languages to communicate with human beings in a human-like manner.
Graph Databases
The leap into digitization calls for appropriate tech transactions, such as moving from a traditional database to a graph database, which can accommodate and process this complex data efficiently. A Graph Database stores data in a graph, the most efficient possible format for connectivity queries. It employs nodes to store data entities and edges to store relationships between entities. The culmination of nodes and edges forms a graph. All nodes are directly linked to all its adjacent nodes, and an edge also contains details of a relationship, enabling faster data-exploration speed. A Graph Database is built from the ground up to support the challenging requirements of modern business critical applications.
Data Visualization
Data Visualization refers to the graphical representation of information and data. By employing visual elements such as charts, graphs, and maps, data visualization tools provide an accessible way to see and comprehend trends, outliers, and patterns in data. In the context of decision-making, this makes data-driven arguments easier to understand and usually more persuasive.
Graph Databases in Data Visualization
Graph Databases offer a mode of illustrating complex associations which would be challenging with other types of databases. They enable an increased and improved visualization of data and the relationships between different sets of data. The visualization of data in graph databases takes places via nodes and edges. This means that data entities (nodes) and the relationship between them (edges) are visually represented, providing a clear and simplified perspective to complex, unstructured data.
Usage of Graph Databases in ChatGPT-4
ChatGPT-4, similar to its predecessors, uses machine learning to generate responses to input data. It can parse vast amounts of data, identify patterns, and generate appropriate conversational responses. When paired with a Graph Database, ChatGPT-4 can receive complex structured data, deduce the relationships between different sets of data, and provide responses based on its interpretations. Although this application could be found in many sectors, from e-commerce to healthcare, data analytics stands out amongst the best applications.
ChatGPT-4, when integrated with graph databases, has an ability to interpret complex data from Graph Databases and provide simplified, understandable visual representations of the data. This could help non-technical professionals access the Graph Database, converse with ChatGPT-4 and quickly get simplified visual summaries of the complex data just by asking. This ability of ChatGPT-4 can revolutionize the way data is accessed and interpreted in organizations, and open up vast opportunities for businesses to leverage data more effectively.
Conclusion
In summary, Graph Databases' integration with ChatGPT-4 opens up new possibilities in the area of data visualization. It makes complex, unstructured data interpretable and accessible, democratizing data and making it easier for any individual in an organization to make data-driven decisions. In a world where data is king, such advancements present vast opportunities for businesses, providing them the tools necessary to stand out in a data-driven economy.
Comments:
Thank you all for taking the time to read my article on Revolutionizing Graph Databases with ChatGPT. I'm excited to hear your thoughts and engage in a discussion on this topic!
Great article, Joe! I've been following the advancements in graph databases, and integrating ChatGPT seems like a game-changer. Do you think it has potential drawbacks, such as scalability issues with large datasets?
Hi Sara, thanks for your kind words! You bring up an important point. While ChatGPT is powerful in its ability to handle complex queries, scalability might be a concern when dealing with massive datasets. However, there are optimizations being explored to mitigate these challenges. It's an exciting area of research!
I'm impressed by the potential of ChatGPT in revolutionizing graph databases. It seems like an intuitive way to query and explore interconnected data. Are there any specific industries where this technology could have a significant impact?
Hi Mark! Absolutely, there are several industries where the integration of ChatGPT and graph databases can create significant impact. Some examples include healthcare for analyzing patient data, finance for fraud detection, and e-commerce for personalized recommendations. The possibilities are vast!
Interesting article, Joe! I'm curious about the potential security implications of using ChatGPT in graph databases. How can we ensure the privacy and integrity of data, especially in sensitive industries?
Hi Natalie, great question! Privacy and data security are crucial when working with sensitive data. Access controls, encryption, and secure communication channels are some of the measures that can be implemented to protect data integrity and confidentiality. It's vital to prioritize these aspects while implementing ChatGPT in graph databases.
Joe, I've read about potential biases in language models like GPT. How can we ensure these biases don't impact the results and decision-making in graph databases?
Hi Ravi, that's an important concern. Bias mitigation is an ongoing area of research in natural language processing. It's crucial to develop robust strategies to identify and address biases during the training and deployment of language models. By implementing unbiased training data and fine-tuning processes, we can minimize the impact of biases on graph database results and decision-making.
Great article, Joe! I'm particularly interested in the performance aspect. How does ChatGPT integration affect query response times in graph databases?
Hi Oliver, glad you found it interesting! The performance impact can vary depending on the complexity of queries and the scale of the graph database. While ChatGPT introduces additional computational overhead, optimizations like caching, indexing, and parallel processing can be employed to improve response times. Balancing performance and accuracy is crucial in such integrations.
Amazing work, Joe! I'm curious about the learning process of ChatGPT when dealing with a graph database. How does it become aware of the underlying connections and relationships?
Hi Sophia, thanks for your kind words! ChatGPT learns about underlying connections and relationships through a combination of training data and the structure of the graph database. By providing it with appropriate context and training examples, the model can understand the relationships between nodes, properties, and paths in the graph. It's a fascinating process!
Joe, excellent article! Can you elaborate on the potential challenges when integrating ChatGPT with existing graph database systems? Are there any compatibility concerns or major modifications required?
Hi Emma, thank you for your kind feedback! Integrating ChatGPT with existing graph database systems can pose challenges. Compatibility concerns may arise due to differences in data models, query languages, and APIs. Additionally, modifications might be required to handle the increased computational requirements of ChatGPT. It's important to assess the impact and plan accordingly for a smooth integration process.
Joe, your article is fascinating! Could you shed some light on the potential limitations of ChatGPT in graph databases? What are some scenarios where it may not be the ideal solution?
Hi William, glad you enjoyed the article! ChatGPT has its limitations, particularly when it comes to handling highly specialized or domain-specific graph databases. In such scenarios, more customized and focused models might be more suitable. Additionally, if real-time responsiveness is a critical requirement, the latency introduced by ChatGPT's language generation process might not be ideal. It's essential to consider these factors based on the specific use case.
Joe, I really liked your article! How can ChatGPT's performance be measured or benchmarked in the context of graph databases? Are there any established metrics for evaluation?
Hi Liam, thank you for your kind words! Measuring ChatGPT's performance in the context of graph databases can involve various metrics. Response time, accuracy of query results, and user satisfaction are some key metrics to consider. It's important to establish baselines, conduct thorough testing, and iterate based on user feedback to optimize performance. Continuous evaluation and improvement are vital!
Joe, your article is thought-provoking! Could ChatGPT integration help in identifying and analyzing patterns within graph databases, especially in research or scientific domains?
Hi Ella, I'm glad you found it thought-provoking! Absolutely, ChatGPT integration can aid in identifying and analyzing patterns within research or scientific graph databases. The model's ability to understand complex queries and provide insightful responses can help researchers uncover hidden correlations, propose hypotheses, and explore novel connections. It has the potential to enhance discovery in various fields!
Joe, superb article! What are your thoughts on the future of graph databases and their integration with models like ChatGPT? Any exciting advancements or trends to look out for?
Hi Henry, thanks for your kind words! The future of graph databases and their integration with models like ChatGPT looks promising. Advancements in natural language processing and graph database technologies will likely drive more seamless integrations. We can expect improved scalability, performance optimizations, and techniques to address biases and privacy concerns. The combination of graphs and language models opens up exciting possibilities for data exploration and knowledge discovery!
Joe, thank you for sharing your expertise through this article! How do you envision the user experience to evolve when interacting with graph databases through ChatGPT? Will it become more intuitive and user-friendly?
Hi Sophie, glad you found it valuable! The user experience when interacting with graph databases through ChatGPT is likely to evolve positively. As natural language processing models improve, users will enjoy a more intuitive and user-friendly interface. The ability to ask questions, receive informative responses, and explore data through conversational interactions can make the experience more accessible and engaging. It's an exciting evolution!
Joe, excellent article! How can ChatGPT help with semantic search and retrieval in graph databases?
Hi Lucas, thanks for your kind words! ChatGPT can be beneficial for semantic search and retrieval in graph databases. By understanding natural language queries, it can uncover relevant nodes, relationships, and paths within the graph. Its ability to interpret context and provide meaningful responses helps users find the information they seek more effectively. It adds a semantic layer to graph search capabilities, making it easier to navigate complex connections!
Joe, your article is inspiring! I'm curious about the training requirements for ChatGPT in the context of graph databases. Is it necessary to use domain-specific training data?
Hi Aaron, glad you found it inspiring! Training ChatGPT for graph databases benefits from domain-specific data to enhance query understanding and accuracy. While fine-tuning on domain-specific data can be helpful, it's not always mandatory. Pre-training on diverse datasets followed by fine-tuning on a smaller dataset relevant to the graph database domain can often yield satisfactory results. It's important to strike a balance!
Joe, I really enjoyed your article! How does ChatGPT handle cases where it encounters ambiguous queries or incomplete information in graph databases?
Hi Maria, glad you enjoyed it! When ChatGPT encounters ambiguous queries or incomplete information in graph databases, it aims to seek clarification or make assumptions based on the available context. It can prompt users for further clarification or offer responses based on the available information. Handling ambiguity is an active area of research, and models like ChatGPT constantly improve to enhance their ability to address such cases.
Joe, your article is enlightening! Are there any specific graph database query languages or APIs that work best when integrating with ChatGPT?
Hi Grace, thank you for your kind words! When integrating ChatGPT with graph databases, there isn't a one-size-fits-all answer regarding query languages or APIs. It depends on the specific database and its ecosystem. However, query languages that offer expressive power, support for graph traversals, and abstraction over the underlying data model can simplify interactions for ChatGPT. It's crucial to consider the compatibility and capabilities of the graph database system.
Joe, excellent insights in your article! How can ChatGPT handle recommendation systems within graph databases? Can it provide personalized recommendations?
Hi Max, glad you found the insights valuable! ChatGPT can be applied in recommendation systems within graph databases. By utilizing knowledge about the user, their preferences, and the graph structure, personalized recommendations can be generated. The model's ability to understand user queries, consider historical data, and leverage graph connections allows for more effective and personalized recommendations. It's an exciting application area!
Joe, your article is fascinating! How can we ensure the reliability and transparency of decision-making based on ChatGPT's responses in graph databases?
Hi Rebecca, thank you for your kind words! Ensuring reliability and transparency in decision-making based on ChatGPT's responses requires measures like explainability and accountability. Techniques such as attention mechanisms, explaining model outputs, and logging user interactions can aid in understanding and auditing the decision process. Additionally, providing feedback channels and continuous monitoring can help improve the reliability and transparency of ChatGPT's influence on decision-making in graph databases.
Joe, great article! I'm curious about the future research directions related to ChatGPT and graph databases. Are there any key challenges or opportunities that researchers are focusing on?
Hi Daniel, glad you liked the article! Future research directions related to ChatGPT and graph databases include optimizing performance by reducing response times, addressing biases, enhancing privacy measures, and exploring more specialized language models tailored for specific industry domains. Additionally, research on seamless integration with distributed graph databases, visual querying, and incorporating user feedback to improve the system are key areas of focus. There's plenty to look forward to!
Joe, impeccable article! How can ChatGPT handle evolving graph database schemas or structural changes?
Hi Ethan, thank you for your kind words! Handling evolving graph database schemas or structural changes is an important consideration. Maintaining a robust training pipeline that incorporates updated data and performs regular retraining allows ChatGPT to adapt to such changes. By being exposed to a variety of schema configurations during training, the model can better understand and handle evolving databases. Continuous updates and improvement cycles ensure compatibility and accuracy!
Joe, your article is enlightening! How can ChatGPT help in detecting anomalies or patterns of interest within graph databases?
Hi Zoe, glad you found it enlightening! ChatGPT can aid in detecting anomalies or patterns of interest within graph databases. By understanding natural language queries describing anomalies or interests, the model can leverage its ability to traverse the graph, analyze relationships, and uncover relevant patterns. This can assist users in identifying outliers, potential fraud, or interesting connections that might otherwise be challenging to discover. It expands the analytical capabilities in graph databases!
Joe, your article is fantastic! How can ChatGPT handle multi-hop reasoning or complex queries involving multiple graph traversal steps?
Hi Jasmine, thank you for your kind feedback! ChatGPT can handle multi-hop reasoning and complex queries involving multiple graph traversal steps. By maintaining context and leveraging its training on diverse examples, the model can understand and fulfill multi-step queries by navigating through the graph, considering relationships, and aggregating information across multiple hops. It's a powerful capability that contributes to ChatGPT's effectiveness in graph databases!
Joe, excellent insights in your article! How can ChatGPT help with data exploration and visualization in graph databases?
Hi Noah, glad you found the insights valuable! ChatGPT can aid in data exploration and visualization in graph databases. By understanding user queries and generating insightful responses, it can guide users in navigating and understanding complex graph structures. Additionally, by leveraging visualization tools that can interpret ChatGPT's outputs, it becomes possible to present the graph data in visually intuitive ways, enhancing the overall exploration experience. It's a powerful combination!
Joe, your article is intriguing! How can ChatGPT handle cases where users ask ambiguous or vague questions in graph database interactions?
Hi Isabella, glad you found it intriguing! When users ask ambiguous or vague questions in graph database interactions, ChatGPT aims to seek clarification by prompting the user for more specific details. By providing helpful suggestions or asking for more context, the model strives to improve the query understanding and offer more accurate and relevant responses. Handling ambiguity is a challenge, but ChatGPT continues to improve its ability to address such cases!
Joe, your article is insightful! What are the main considerations when selecting or designing a graph database for integration with ChatGPT?
Hi Thomas, thank you for your kind words! When selecting or designing a graph database for integration with ChatGPT, several considerations come into play. Some key factors include the data model's compatibility with expressive query languages, support for graph traversals, scalability options, and the ability to efficiently handle complex queries. Additionally, characteristics like data integrity, privacy features, and ease of integration are important aspects to evaluate. Finding the right balance based on the specific requirements is crucial!
Joe, great article! Can ChatGPT handle inference or reasoning on top of the graph database, supporting deductions or logical operations?
Hi Lucy, glad you liked the article! ChatGPT can handle inference or reasoning on top of the graph database by supporting deductions and logical operations. While it may not provide full-fledged logical reasoning capabilities, it can leverage its training on contextual information, graph traversals, and relationships to offer reasoned responses. This allows users to receive recommendations, insights, and explanations based on the underlying graph structure. It opens up opportunities for inferential reasoning!
Joe, your article is inspiring! How can ChatGPT handle queries involving temporal aspects in graph databases?
Hi Emily, thank you for your kind words! ChatGPT can handle queries involving temporal aspects in graph databases. By maintaining context and training on diverse examples, the model can understand and reason about time-related queries. It can track historical states, changes, or events within the graph, allowing users to inquire about specific time periods, patterns, or trends. This temporal understanding adds another dimension to the capabilities of graph-based interactions!
Joe, fantastic article! Is there any particular skill set or knowledge required to integrate ChatGPT with graph databases effectively?
Hi Nora, glad you found it fantastic! Integrating ChatGPT with graph databases effectively requires a combination of skills. Familiarity with natural language processing, graph database technologies, query optimization, and data modeling is beneficial. Understanding the nuances of both the language model and the graph database system helps in designing efficient integrations. Collaboration between domain experts, data scientists, and engineers contributes to successful implementations. A multidisciplinary skill set is advantageous!
Joe, your article is informative! How does ChatGPT handle cases where a query's response is uncertain or subjective in the context of graph databases?
Hi Alex, glad you found it informative! When a query's response is uncertain or subjective in the context of graph databases, ChatGPT aims to provide informative but nuanced responses. It can acknowledge the uncertainty and present multiple possibilities, highlight potential biases, or consider subjective factors that might influence the response. The model strives to help users make informed decisions when facing situations that warrant subjective or uncertain answers.
Joe, great article! How can ChatGPT accommodate user feedback and adapt to evolving user needs within graph database interactions?
Hi Emma, thank you for your kind words! Accommodating user feedback and adapting to evolving user needs is essential in graph database interactions. Continuous feedback loops and access to user suggestions help improve the model's responses over time. By monitoring user sentiment and analyzing user interactions, it becomes possible to iteratively refine ChatGPT's behavior, better align it with user expectations, and address any shortcomings. User-centric adaptation plays a key role in enhancing the effectiveness of the system!
Joe, your article is thought-provoking! Can ChatGPT help in data cleaning or identification of inconsistencies within graph databases?
Hi Charlie, glad you found it thought-provoking! ChatGPT can assist in data cleaning and identification of inconsistencies within graph databases. By understanding user queries describing data quality concerns, the model can interpret the intent and guide users towards potential inconsistencies or errors in the graph. ChatGPT's ability to analyze relationships and contextual information aids in the identification of data issues, supporting the data cleaning process and ensuring data integrity in the graph!
Joe, your article raises interesting points! How can ChatGPT handle graph databases with large-scale or distributed architectures?
Hi Samuel, thank you for your kind feedback! Handling large-scale or distributed graph databases is challenging but feasible with ChatGPT. Techniques like parallel processing, distributed computing, and efficient graph traversal algorithms can be utilized to manage the computational complexity and optimize performance. Additionally, integrating with distributed graph databases' architectures requires considering data partitioning, load balancing, and data consistency measures. Scaling ChatGPT to large-scale graphs is an exciting area of research!
Joe, your article is fascinating! Can ChatGPT support real-time decision-making in graph databases, or does it primarily suit offline or analytical use cases?
Hi Ruby, glad you found it fascinating! While ChatGPT is powerful in analyzing and providing insights in graph databases, its suitability for real-time decision-making might depend on the specific requirements. The language generation process introduces inherent latency, which might be a constraint for time-critical or highly dynamic scenarios. However, optimizing response times and integrating with real-time data pipelines can mitigate this limitation to some extent. It's important to evaluate the trade-offs based on the use case!
Joe, excellent article! Can ChatGPT handle reasoning and queries involving probabilistic or uncertain aspects in graph databases?
Hi Louise, thank you for your kind words! ChatGPT can handle reasoning and queries involving probabilistic or uncertain aspects in graph databases to some extent. By considering contextual information, available probabilities, or uncertainty measures in the graph, it can offer reasoned responses that take into account the uncertainty. This allows users to explore probabilistic relationships, evaluate potential outcomes, and make informed decisions based on the available probabilistic or uncertain data within the graph!
Joe, your article is enlightening! How do you envision the collaboration between researchers, industry, and open-source communities in advancing ChatGPT and graph database integration?
Hi Freya, glad you found it enlightening! Collaboration between researchers, industry, and open-source communities is crucial for advancing ChatGPT and graph database integration. Researchers can explore innovative techniques, address challenges, and improve the models based on real-world use cases. Industry players can provide valuable insights, infrastructure, and feedback to make the solutions practical and impactful. Open-source communities foster knowledge sharing, contribute to advancements, and ensure accessibility to a broader audience. Together, they drive progress, foster innovation, and bring the benefits to the community!
Joe, your article is superb! How can ChatGPT handle secure integration and access control mechanisms when interacting with graph databases?
Hi Georgia, thank you for your kind words! ChatGPT can handle secure integration and access control mechanisms when interacting with graph databases. By utilizing encryption techniques, secure communication channels, and proper authentication protocols, the model can ensure data privacy and access control. Employing authorization mechanisms, role-based access control, and data anonymization techniques further contribute to secure integration. It's vital to implement a robust security framework while enabling seamless interactions with the graph database through ChatGPT!
Joe, excellent insights in your article! How can ChatGPT handle complex nested queries or aggregations in graph databases?
Hi Adam, glad you found the insights excellent! ChatGPT can handle complex nested queries or aggregations in graph databases. By understanding the hierarchical structure of nested queries and leveraging its training on diverse examples, the model can navigate the graph and generate responses that involve nested operations or aggregations. It allows users to receive comprehensive insights or computed results in response to complex queries based on the nested structure of the graph data!
Joe, your article is thought-provoking! Can ChatGPT handle queries involving different languages or translations in a multilingual graph database context?
Hi Lucia, glad you found it thought-provoking! ChatGPT's ability to handle queries involving different languages or translations depends on the training data and the language model variants available. By training on diverse multilingual data and considering the context, it can offer responses for different languages in a multilingual graph database context. However, proficiency and accuracy might vary across languages based on the model's exposure and training data availability. It's an area where advancements in multilingual models with broader coverage are actively being researched!
Joe, your article is inspiring! How can ChatGPT handle user queries that involve both structured and unstructured data in graph databases?
Hi Ruby, glad you found it inspiring! ChatGPT can handle user queries that involve both structured and unstructured data in graph databases. By leveraging its training on diverse examples and understanding the underlying graph structure, it can integrate structured and unstructured data sources to provide meaningful responses. By interpreting the intent of the query and traversing the graph accordingly, it helps users explore the connections and uncover insights from a combination of structured and unstructured data. It's a powerful capability in graph-based interactions!
Joe, your article is fantastic! How can ChatGPT ensure accurate and reliable responses even when dealing with noisy or incomplete data in graph databases?
Hi Nathan, thank you for your kind words! Ensuring accurate and reliable responses when dealing with noisy or incomplete data in graph databases is challenging. ChatGPT's training on diverse examples helps it to understand the intent, overlook noise, and infer missing information to some extent. By providing informative suggestions, handling incomplete queries gracefully, and offering explanations for the limitations, the model strives to maintain accuracy and reliability even in the presence of noisy or incomplete data. It's an ongoing area of research to further enhance these capabilities!
Joe, excellent article! Can ChatGPT support exploratory querying and visualization of the graph database structure itself?
Hi Callum, glad you liked the article! ChatGPT can support exploratory querying and visualization of the graph database structure itself to some extent. By understanding the user's intent, interpreting the query, and leveraging its training on diverse examples, the model helps users explore and understand the underlying graph structure. Coupled with appropriate visualization tools, it enables users to interactively explore connections, navigate the graph, and gain insights into the graph database structure. It enhances the exploratory capabilities in graph-based interactions!
Joe, your article is enlightening! How does ChatGPT deal with user queries that involve domain-specific jargon or technical terms in graph databases?
Hi Anna, thank you for your kind words! ChatGPT's ability to handle user queries involving domain-specific jargon or technical terms depends on its exposure to the relevant training data during fine-tuning. By training the model with data that covers the desired domain or by leveraging domain-specific resources, it can better understand and respond to queries involving jargon or technical terms in the context of graph databases. It's essential to fine-tune the language model based on the specific domain needs for optimal performance!
Joe, great article! How does ChatGPT handle queries that require reasoning over long paths or chains of nodes in graph databases?
Hi Liam, glad you found the article great! When queries require reasoning over long paths or chains of nodes in graph databases, ChatGPT uses its training on diverse examples and understanding of context to navigate through the graph. By considering relationships, traversing the path, and leveraging contextual information, it offers responses that involve reasoning over long paths or chains of nodes. This allows users to uncover insights, traverse through complex connections, and explore relationships spanning extended paths in the graph!
Joe, your article is insightful! Can ChatGPT handle user queries that involve temporal aspects within the graph database structure?
Hi Lucas, thank you for your kind words! ChatGPT can handle user queries that involve temporal aspects within the graph database structure. By understanding and reasoning about time-related queries, the model can assist users in exploring temporal patterns, historical trends, or time-dependent relationships within the graph. It leverages its training on diverse examples to provide appropriate responses based on the temporal context, allowing for deeper insights and analysis in graph-based interactions!
Joe, your article is fascinating! How can ChatGPT support natural language generation functionality when interacting with graph databases?
Hi Isabel, glad you found it fascinating! ChatGPT supports natural language generation functionality when interacting with graph databases. By considering the context, understanding the intent of the user query, and leveraging its training on diverse examples, the model generates responses that convey meaningful information in a human-like manner. This natural language generation capability enhances the user experience, making interactions with graph databases more intuitive, informative, and accessible. It's a key aspect of ChatGPT's utility in graph-based applications!
Joe, superb article! How can ChatGPT handle cases where queries involve complex logical operations or expressions in graph databases?
Hi Thomas, thank you for your kind words! ChatGPT can handle cases where queries involve complex logical operations or expressions in graph databases. By understanding the logical structure, interpreting the intent, and leveraging its training on diverse examples, the model is capable of providing responses that involve complex logical operations or expressions. It supports users in constructing intricate queries, exploring complex conditions, and evaluating logical expressions within the graph. It enhances the query capabilities when dealing with complex logical constructs!
Joe, excellent article! Can ChatGPT facilitate collaborative exploration or knowledge sharing within graph databases?
Hi Joshua, glad you liked the article! ChatGPT can facilitate collaborative exploration and knowledge sharing within graph databases. By enabling conversational interactions, it enhances the collaborative experience by providing a platform for multiple users to ask questions, obtain insights, and share knowledge. Users can collaboratively navigate the graph, discuss findings, and collectively explore the data space, fostering a collaborative environment that leads to increased knowledge sharing and discovery in graph databases. It adds a collaborative dimension to data exploration!
Joe, your article is informative! How can ChatGPT handle cases where users have diverse or evolving needs in graph databases?
Hi Sophia, glad you found it informative! When users have diverse or evolving needs in graph databases, ChatGPT's flexibility in understanding natural language queries and leveraging its training data allows it to adapt to varying user requirements. By handling a wide range of queries, accommodating user feedback, and continuously improving its responses over time, the model can address diverse and evolving needs. It empowers users to explore the graph, derive insights, and uncover valuable information based on their specific requirements as they evolve!
Joe, great article! Are there any concerns regarding reliability or quality of responses when using ChatGPT with graph databases as compared to traditional query languages?
Hi Callum, thank you for your kind words! Concerns regarding the reliability or quality of responses when using ChatGPT with graph databases can arise due to the probabilistic nature of language models. While ChatGPT offers contextual understanding and insightful responses, there may be cases where the generated answers lack accuracy or precision compared to traditional query languages. Balancing the benefits of conversational interactions with accuracy requirements becomes crucial. It's important to appropriately assess the trade-offs and ensure the reliability and quality of responses align with the specific graph database use case!
Joe, your article is thought-provoking! Can ChatGPT assist in identifying potential biases or inconsistencies within graph databases?
Hi Olivia, glad you found it thought-provoking! ChatGPT can aid in identifying potential biases or inconsistencies within graph databases. By understanding user queries that explore data integrity concerns or potentially biased patterns, the model can assist in identifying inconsistencies, highlighting potential biases, and recommending actions to address them. The model's capabilities coupled with rigorous evaluation techniques contribute to ensuring data reliability and fairness within graph databases. It's important to foster responsible and unbiased data exploration in graph-based interactions!
Thank you all for taking the time to read my article on 'Revolutionizing Graph Databases: Harnessing the Power of ChatGPT'! I'm looking forward to hearing your thoughts and insights.
Great article, Joe! I've been using graph databases for a while now, and the idea of incorporating ChatGPT into it sounds fascinating. It opens up a lot of possibilities for more intelligent and context-aware querying.
I agree with Michael. The ability to have human-like conversations with a graph database can greatly improve its usability. It could make querying and exploring relationships much more intuitive.
Thank you, Michael and Karen, for your positive feedback! Indeed, by leveraging the power of ChatGPT, graph databases can become more user-friendly and provide a natural way to interact with complex data.
I'm not sure about this. While it may enhance usability, won't incorporating ChatGPT introduce additional complexity and potential biases in data interpretation?
Valid concern, Sarah. Bias in data interpretation is a challenge in AI systems. When using ChatGPT with graph databases, it's crucial to carefully curate the training data to minimize biases and ensure robustness. Transparency and accountability are paramount in this context.
I've had experience with graph databases, and while ChatGPT integration sounds intriguing, I'm curious about the scalability. Will incorporating a language model like ChatGPT impact the performance of graph query execution?
Good question, Jonathan. Incorporating ChatGPT would introduce additional computational overhead, but steps can be taken to mitigate this impact. For example, optimizing the implementation and leveraging distributed computing can help maintain satisfactory performance even with increased complexity.
I can see the potential benefits, but I worry about the learning curve for non-technical users. Would using ChatGPT significantly increase the complexity of querying for those without a deep understanding of graph databases?
That's a valid concern, Amy. One approach is to provide intuitive interfaces and natural language query options alongside ChatGPT integration. This way, non-technical users can still benefit from its power while maintaining usability for all levels of expertise.
As someone who works extensively with graph databases, I see immense potential in combining them with ChatGPT. It could take knowledge discovery and exploratory analysis to a whole new level.
I completely agree, Maxime. The combination of graph databases and ChatGPT opens up exciting possibilities for advanced knowledge discovery and data exploration.
While I see the benefits, I can't help but think about the ethical implications. With a system like ChatGPT integrated, how can we ensure responsible use of the data and prevent misuse?
Ethics is a critical aspect, Lisa. By implementing robust data privacy measures, user consent mechanisms, and regular audits, we can strive for responsible use of the integrated system. It's crucial to address ethical concerns proactively.
I'm excited about the potential here, but I'm also wondering about the impact on query speed. Will incorporating ChatGPT slow down the response times significantly?
Response time is an important consideration, David. While there may be a slight impact on query speed, optimizing the implementation and distributing the workload can help minimize any noticeable delays. The goal is to strike a balance between functionality and performance.
I like the idea of making graph databases more user-friendly, but I'm concerned about the potential security vulnerabilities introduced by integrating an AI language model. How would you address this, Joe?
Addressing security vulnerabilities is crucial, Isabella. Implementing robust security protocols, regular code audits, and rigorous testing can help minimize the risks associated with the integration. By following best practices, we can prioritize both usability and security.
I can see how ChatGPT integration would enhance knowledge sharing and collaboration in graph databases. It could facilitate discussions and help users make more informed decisions based on the insights derived.
Absolutely, Sophie! Collaboration and knowledge sharing within graph databases can be greatly enhanced by incorporating ChatGPT. It turns the querying process into a more interactive and insightful experience.
Graph databases are already powerful tools, but adding ChatGPT gives it a whole new dimension. Really excited about the possibilities it offers.
Thanks, Kevin! The combination of graph databases and ChatGPT does indeed open up a new dimension of possibilities, empowering users to leverage richer and more intuitive interactions with their data.
I'm curious about the implementation details. How challenging is it to integrate ChatGPT with existing graph databases, both from a technical and maintenance perspective?
Integrating ChatGPT with existing graph databases can pose technical challenges, Michelle. It requires expertise in natural language processing, graph database APIs, and efficient data synchronization. Maintenance-wise, regular updates, performance monitoring, and prompt issue resolution are vital to ensure a smooth integration experience.
I'm curious if ChatGPT can handle complex queries involving multiple relationships and attributes. Is it capable of extracting insights from complex graph structures effectively?
Great question, Emily! ChatGPT can be trained on complex graph database structures, allowing it to handle queries involving multiple relationships and attributes. By designing appropriate training data and providing context-aware interactions, it can effectively extract insights from complex graph structures.
This integration sounds amazing, but what about the learning curve for developers? Will they need to learn additional skills to work effectively with ChatGPT and graph databases?
Learning curves are an important consideration, Oliver. Developers may need to familiarize themselves with the specific integration techniques and refine their knowledge of graph database APIs. However, comprehensive documentation, example code, and developer resources can assist in the transition, making the learning process more accessible.
I wonder if there are any use cases or industries where the integration of ChatGPT and graph databases would be particularly valuable.
Absolutely, Ryan! Industries such as healthcare, finance, logistics, and social networks can greatly benefit from the integration. Use cases could include smarter patient diagnosis, fraud detection, optimized routing, and personalized recommendations, just to name a few!
While the concept sounds interesting, I'm concerned about the potential biases in the responses generated by ChatGPT. How can we ensure that the information retrieved from graph databases is accurate and unbiased?
Addressing biases is a critical aspect, Jennifer. Ensuring a diverse and representative training dataset for ChatGPT is essential. Additionally, implementing mechanisms for user feedback and continuous model evaluation can help identify and rectify potential biases promptly, ensuring the accuracy and reliability of the information retrieved from graph databases.
This integration could be a game-changer for data exploration and research. It could empower researchers to dive deeper into complex relationships and gain valuable insights more efficiently.
Absolutely, Sophia! In the research domain, the integration of ChatGPT and graph databases offers researchers a powerful tool for exploring intricate relationships and accelerating the process of knowledge discovery.
How do you envision the future development and adoption of ChatGPT within graph databases, Joe?
Great question, Robert! I believe that the adoption of ChatGPT within graph databases will continue to grow as the technology matures. As research and real-world use cases expand, we'll see advancements in the integration techniques and improved user experiences, leading to broader adoption across various industries and domains.
What steps can be taken to ensure that incorporating ChatGPT into graph databases doesn't compromise data privacy?
Data privacy is crucial, Laura. Encryption of sensitive data, implementing access controls, and adhering to data protection regulations can ensure that incorporating ChatGPT into graph databases upholds the highest standards of privacy and data security.
I've been exploring the potential of graph databases, and ChatGPT integration seems like a logical next step. It could be a great way to enhance user experiences and drive innovation in the data industry.
Thank you, Samuel! The integration of ChatGPT with graph databases indeed holds significant promise for enhancing user experiences, enabling new discoveries, and driving innovation in various data-driven domains.