Revolutionizing HEC-RAS: Leveraging ChatGPT's Transformative Potential in Flood Modeling Technology
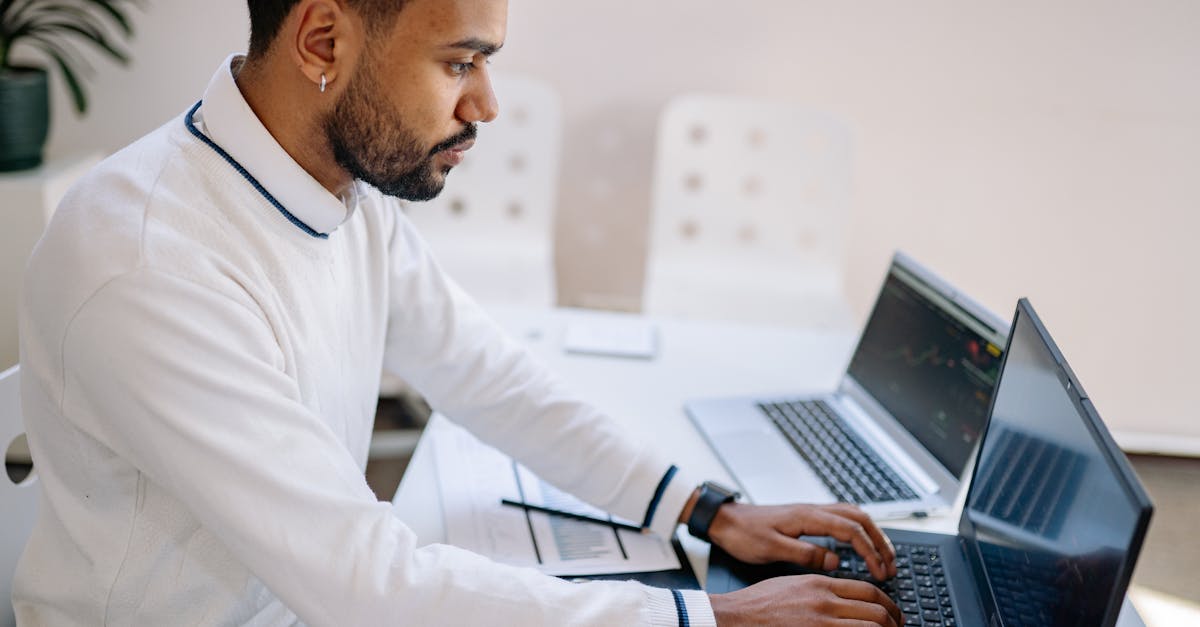
In the world of data analysis, the ability to understand and interpret diverse data sets is paramount. It allows one to identify and predict trends, foresee potential problems, and facilitate informed decision-making. In the context of Hydrological Engineering, the Hydrologic Engineering Center’s River Analysis System (HEC-RAS) is a commonly used system for analyzing river flow and hydraulic phenomena. This article discusses the usage of ChatGPT-4 in analyzing data generated by HEC-RAS.
The Benefits of Deep Learning in Data Analysis
Deep learning algorithms like ChatGPT-4 add a new layer of sophistication to data analysis. By utilizing elements like sequential learning, deep learning algorithms can understand temporal data and make predictions based on that data. This makes artificial intelligence models such as ChatGPT-4 ideal for analyzing and interpreting the output from HEC-RAS.
Traditional data analysis methods can generally only detect patterns and trends that have been specifically programmed to look for. Deep learning algorithms, however, can recognize and adapt to patterns on their own. This allows them to "learn" from the data they're analyzing, identify anomalies, and make sophisticated predictions based on their "understanding" of the data.
Understanding HEC-RAS
HEC-RAS is a one-dimensional flow model developed by the U.S. Army Corps of Engineers’ Hydrologic Engineering Center. It allows engineers to carry out detailed analyses of complex river systems, including calculations of water surface profiles, sediment transport, water temperature modeling, water quality analysis, and much more. HEC-RAS is a versatile and sophisticated tool that can generate a wealth of data, making it an ideal target for deep learning algorithms such as ChatGPT-4.
Predicting Trends with ChatGPT-4
ChatGPT-4 can help in parsing through the massive amounts of data that HEC-RAS can generate, identifying patterns and correlations that may be invisible to human analysts. For example, ChatGPT-4 could assess past and current data to predict future water levels in a specific river system, potentially helping local authorities prepare for floods or droughts.
Highlighting Anomalies in the Data
Anomalies in data can often indicate potential issues, and with a system as complex as HEC-RAS, these anomalies can be hard to spot without the aid of advanced analysis tools. ChatGPT-4's ability to detect and highlight these unusual data points can help to proactively identify and address potential problems in the hydraulic systems being modeled.
Conclusion
By leveraging the power of next-generation AI technologies such as ChatGPT-4, data analysis in the field of Hydrological Engineering can be greatly enhanced. While the technology is still developing, the potential benefits to data analysis, prediction accuracy, anomaly detection, and overall understanding of complex systems like those modeled in HEC-RAS are clear.
The combination of HEC-RAS and AI-supported data analysis tools like ChatGPT-4 offers exciting possibilities for more accurate, dynamic, and predictive modeling in Hydrologic Engineering. As AI continues to evolve, so too will the insights that can be gleaned from HEC-RAS data, laying a solid foundation for future advancements in the field.
Comments:
Thank you all for your interest in my article! I'm excited to discuss the potential of leveraging ChatGPT in flood modeling technology.
This is such an interesting topic! I never thought about using ChatGPT in flood modeling. Can you elaborate on how it could revolutionize HEC-RAS?
Certainly, Sara! ChatGPT can assist in real-time data analysis, predictive modeling, and scenario planning. Its natural language processing capabilities can make flood modeling more accessible and interactive for professionals and communities alike.
I like the idea of leveraging ChatGPT for flood modeling, but have there been any studies or practical implementations of this concept?
Great question, David! While it's still an emerging area, there have been some preliminary studies showing promising results. Researchers have experimented with ChatGPT's dialogue-based approach to simulate flood scenarios and provide user-friendly flood information.
This sounds like a potential game-changer in flood management. I'm curious to know how accurate and reliable ChatGPT's predictions can be compared to traditional models.
Indeed, Emily! While ChatGPT is not a replacement for traditional models like HEC-RAS, it can complement them by providing additional insights and enhancing the overall decision-making process. The aim is to empower stakeholders with a more comprehensive understanding of flood dynamics.
I can see how ChatGPT can improve communication and collaboration between flood modeling experts and non-technical individuals. It could potentially bridge the knowledge gap and raise public awareness.
Absolutely, Philip! By using ChatGPT as a communication tool, flood modeling experts can engage with non-technical individuals, answer questions, and facilitate a better understanding of flood risks and mitigation strategies.
I wonder what challenges or limitations ChatGPT might face in the context of flood modeling. Any thoughts on that, Giuseppe?
Good question, Sara! ChatGPT may face challenges in handling complex and domain-specific flood modeling queries and producing accurate predictions for unprecedented scenarios. Ensuring proper training and validation of the model will be crucial.
Are there any privacy concerns when using ChatGPT for flood modeling? How would sensitive data be handled in this context?
Privacy is paramount, Andrew! Sensitive data should be treated with appropriate measures, ensuring data protection regulations are adhered to. Anonymizing data and using secure channels for communication would be essential.
I'm curious if there are any plans to integrate ChatGPT into existing flood modeling software like HEC-RAS. That could be a seamless way to leverage its potential.
Absolutely, Alexis! Integrating ChatGPT into existing flood modeling software could streamline the user experience and enable professionals to leverage its potential within the familiar HEC-RAS environment.
Thank you, Giuseppe, for the insights! I'm truly excited about the possibilities ChatGPT can bring to flood modeling. Looking forward to future advancements in this field!
You're welcome, Emily! I share your excitement, and together, we can push the boundaries of flood modeling technology. Exciting times ahead indeed!
I'm skeptical about relying too much on AI for flood modeling. What if the technology fails or produces incorrect predictions? We should be cautious about putting too much trust in it.
A valid concern, John. While AI can enhance flood modeling, it should always be used as a supportive tool, not the sole source of decision-making. Domain expertise, validation, and regular checks against real-world data are crucial to avoid overreliance.
I appreciate your responses, Giuseppe. It's reassuring to know that ChatGPT is seen as a complement to existing methods, rather than a replacement. The human element is vital in making critical decisions.
Well said, David! Flood modeling is a multidimensional challenge, and by combining the strengths of ChatGPT with human expertise, we can pave the way for more informed and effective flood management.
Thank you, Giuseppe, for addressing our questions and concerns. Your article has definitely piqued my interest in the potential of ChatGPT in flood modeling. Keep up the great work!
Thank you for your kind words, Sara! I'm glad you found the article interesting. It's through discussions like these that we can collectively explore new possibilities and drive innovation in the field of flood modeling.
I have a background in flood modeling, and I can see how ChatGPT could facilitate knowledge sharing between experts. It could help us learn from each other's experiences faster.
Absolutely, Christopher! ChatGPT's ability to store and retrieve knowledge can be valuable for experts to share insights, learn from past experiences, and build upon each other's expertise. Collaboration is key in advancing flood modeling.
Incorporating ChatGPT into flood modeling could also make it more engaging and accessible for educational purposes. Students and researchers would benefit greatly from interactive flood simulations.
Great point, Oliver! By using ChatGPT's interactive capabilities in educational settings, we can inspire the next generation of flood modeling professionals and foster a deeper understanding of the complex dynamics involved.
Do you think ChatGPT has the potential to democratize flood modeling by empowering local communities to understand and contribute to the decision-making process?
Absolutely, Sara! By making flood modeling more accessible and understandable, ChatGPT can empower local communities to actively engage in the decision-making process, contribute their knowledge, and develop a sense of ownership in managing flood risks.
I love the idea of involving communities in flood modeling. They often have valuable insights from their experiences. ChatGPT can be a tool for inclusive decision-making.
Well said, Philip! Including communities in the flood modeling process can help capture local knowledge and ensure the solutions are tailored to their specific needs and challenges. ChatGPT can indeed foster inclusive decision-making.
Are there any ethical considerations when using ChatGPT in flood modeling? How can we address potential biases that may arise from the training data?
Ethical considerations are crucial, Andrew. It's essential to ensure the training data is diverse, representative, and carefully vetted to mitigate biases. Regular audits and transparency in the development process can help address these concerns.
I appreciate the emphasis on ethics, Giuseppe. It's vital to ensure the use of AI is fair and unbiased, especially when it comes to decision-making in critical areas like flood management.
Absolutely, Emily! Responsible and ethical use of AI is paramount to maintain trust and ensure the technology serves the best interests of society in areas as critical as flood management. Thank you for highlighting this important aspect.
This discussion has been enlightening! It's fascinating to think about the potential of ChatGPT in revolutionizing flood modeling. It could truly transform the way we deal with flood risks.
Thank you, Mark! I'm glad you found the discussion enlightening. ChatGPT indeed has the potential to reshape flood modeling and contribute to more effective flood risk management strategies.
What are the next steps in the development of ChatGPT for flood modeling? Are there any ongoing projects or research initiatives in this area?
Good question, Jessica! While I can't speak for specific ongoing projects, continued research into fine-tuning ChatGPT for flood modeling, integrating it with existing software, and exploring its real-world applications are some potential next steps.
I can't wait to see how ChatGPT and flood modeling evolve in the coming years. The possibilities seem endless, and the potential benefits are immense.
Indeed, Michael! The future of flood modeling with ChatGPT is full of exciting possibilities. With ongoing advancements and collaborative efforts, we can expect significant benefits in terms of flood preparedness and risk management.
Thank you, Giuseppe, for the engaging discussion. I'm inspired to explore the intersection of AI and flood modeling further. Keep up the great work!
Thank you for your kind words, Oliver! I'm pleased to have sparked your interest. Embracing AI in flood modeling opens up new avenues for innovation and progress. Best of luck in your exploration!
It's been a pleasure participating in this discussion. Thank you, Giuseppe, for sharing your expertise with us. I'm excited to see where the future takes ChatGPT in flood modeling.
Thank you for your participation, David! I appreciate your contribution to the discussion. The future is indeed promising, and I'm enthusiastic about the potential of ChatGPT in flood modeling. Best wishes!
Thank you, Giuseppe, for guiding this discussion and providing valuable insights. Your expertise in flood modeling and AI has broadened our perspectives.
You're most welcome, David! Leading this discussion and sharing insights has given me the opportunity to broaden perspectives and foster a deeper understanding of the potential of AI in flood modeling. Thank you for your active participation.
Giuseppe, your insights have been invaluable. Thank you for taking the time to engage with us and shed light on this exciting topic.
You're very welcome, Sara! It's been a pleasure discussing this fascinating topic with you all. Thank you for your active participation and insightful questions.
Thank you, Giuseppe, for leading this discussion and fostering an enriching exchange of ideas. Your expertise has been truly enlightening.
You're welcome, Sara! Thank you for your kind words. Leading this discussion and witnessing the enriching exchange of ideas has been a rewarding experience. I'm thrilled to have shared my expertise and contributed to your enlightenment.
This discussion has provided a fresh perspective on flood modeling. The potential of ChatGPT is immense, and I look forward to future advancements in this field.
I'm glad you found the discussion valuable, Emily! The immense potential of ChatGPT in flood modeling holds the promise of reshaping how we approach flood risk management. Exciting times lie ahead!
Giuseppe, thank you for facilitating this engaging discussion. Your knowledge and willingness to address our questions have been invaluable.
You're welcome, Emily! Facilitating this engaging discussion and addressing your questions has been a pleasure. I'm grateful for the opportunity to share my knowledge and contribute to a deeper understanding of the topic.
Thank you, Giuseppe, for sharing your knowledge and insights with us. I'm grateful for the opportunity to learn from you and the other participants.
You're most welcome, Philip! I'm grateful for your active engagement and thoughtful questions. Knowledge sharing and collaboration drive innovation, and I'm delighted to have been part of this discussion.
Thank you, Giuseppe, for moderating this discussion and providing us with valuable insights. Your expertise in flood modeling and AI is commendable.
You're very welcome, Philip! I appreciate your kind words and recognition. Moderating this discussion and sharing insights have allowed me to showcase the potential of AI in flood modeling. Thank you for your active engagement.
Thank you, Giuseppe, for the informative discussion. I've learned a lot about the potential use of ChatGPT in flood modeling. Exciting work ahead!
Thank you for your kind words, Andrew! I'm pleased to have contributed to your knowledge on this topic. Let's continue pushing the boundaries and embracing new possibilities in flood modeling.
Thank you, Giuseppe, for guiding this insightful discussion. Your expertise has broadened our understanding of the potential applications of ChatGPT in flood modeling.
You're welcome, Andrew! Guiding this insightful discussion and broadening your understanding of the potential applications of ChatGPT in flood modeling has been a rewarding endeavor. Thank you for your valuable contributions.
Giuseppe, your expertise has been invaluable in this discussion. Thank you for your time and for sharing your insights with us all.
You're very welcome, Jessica! I'm grateful for the opportunity to share my expertise and engage in this enlightening discussion. Thank you for your active participation.
Giuseppe, thank you for leading this discussion and sharing your expertise. The potential of ChatGPT in flood modeling is now much clearer.
You're most welcome, Jessica! I'm thrilled to have led this discussion and provided clarity on the potential of ChatGPT in flood modeling. Thank you for your active participation and engagement.
This discussion has given me a lot to think about. Giuseppe, your article and insights have opened my eyes to the transformative potential of ChatGPT in flood modeling.
I'm glad to hear that, Christopher! It's been a pleasure sharing my insights, and I'm excited to have sparked new perspectives on the transformative potential of ChatGPT in flood modeling. Thank you for your engagement.
Thank you, Giuseppe, for your contribution to this discussion. The integration of ChatGPT into flood modeling holds great promise. I'm optimistic about what lies ahead!
You're welcome, Michael! The promise of integrating ChatGPT into flood modeling is indeed remarkable. Let's remain optimistic and continue pushing boundaries to realize its full potential. Best wishes!
Giuseppe, I wanted to express my gratitude for this insightful discussion. The potential applications of ChatGPT in flood modeling are truly inspiring.
Thank you for your kind words, Oliver! It's been a pleasure engaging in this discussion, and I'm glad you found it inspiring. The potential applications of ChatGPT in flood modeling are indeed exciting.
Giuseppe, thank you for sharing your expertise with us. This discussion has left me eager to explore the intersection of AI and flood modeling in more detail.
You're most welcome, Mark! I'm thrilled to have ignited your curiosity and interest. Exploring the intersection of AI and flood modeling further will undoubtedly lead to new insights and advancements. Best of luck on your journey!
Thank you, Giuseppe, for your time and valuable contributions to this discussion. The potential impact of ChatGPT in flood modeling cannot be underestimated.
You're welcome, Alexis! I appreciate your kind words and enthusiasm. The potential impact of ChatGPT in flood modeling is indeed significant, and it's through discussions like these that we can collectively shape its future. Thank you for engaging.
This has been an insightful discussion. Thank you, Giuseppe, and all the participants, for sharing your knowledge and thoughts on this exciting topic.
Thank you for your participation, John! I'm glad you found the discussion insightful. The collective knowledge and diverse perspectives shared here contribute to a deeper understanding of the potential of ChatGPT in flood modeling.
Giuseppe, thank you for your expertise and for organizing this insightful discussion. The potential of ChatGPT in flood modeling is compelling, and I'm excited to follow its progress.
You're most welcome, Jane! I'm grateful for your active participation and enthusiasm. The progress in ChatGPT and its potential in flood modeling is indeed compelling, and I'm excited to see the advancements it will bring.
I wholeheartedly agree, Giuseppe. This discussion has provided valuable insights into the potential of ChatGPT in flood modeling. Thank you once again.
Thank you for your kind words, Jane! I'm pleased to have provided valuable insights. The potential of ChatGPT in flood modeling is vast, and it's through discussions like these that we can uncover its numerous possibilities.
Giuseppe, thank you for your time and for sharing your expertise. ChatGPT's potential in flood modeling is truly exciting, and I'm keen to see its further development.
You're welcome, Megan! I appreciate your participation and enthusiasm. The potential of ChatGPT in flood modeling is indeed exciting, and by continuing to explore and develop it, we can unlock even more benefits. Best wishes!
Giuseppe, thank you for your knowledge-sharing during this discussion. Your expertise in flood modeling and AI has been illuminating.
You're welcome, Megan! Knowledge-sharing and illuminating discussions like this are fundamental in driving progress in flood modeling with AI. I'm delighted to have shared my expertise and contributed to your understanding.
Thank you, Giuseppe, for facilitating this enriching discussion. Your knowledge and expertise have broadened our perspectives.
You're welcome, Megan! Facilitating this enriching discussion and broadening your perspectives on the potential of ChatGPT in flood modeling with my knowledge and expertise has been a fulfilling endeavor. Thank you for your active involvement.
Thank you, Giuseppe, for your valuable input in this discussion. The potential integration of ChatGPT in flood modeling technology is intriguing. I'm eager to follow its progress.
You're welcome, Liam! I'm pleased to have contributed to this discussion and sparked your interest. The integration of ChatGPT in flood modeling technology indeed holds great intrigue, and I'm excited to witness its progress alongside fellow enthusiasts like you.
Giuseppe, your expertise has been invaluable in shedding light on the potential of ChatGPT in flood modeling technology. Thank you for sharing your insights with us.
You're very welcome, Ava! I appreciate your kind words. It's been an honor to share my insights and contribute to a better understanding of the potential of ChatGPT in flood modeling technology.
Giuseppe, I want to express my gratitude for this enlightening discussion. I've gained valuable knowledge about the possibilities of ChatGPT in flood modeling.
I'm glad you found the discussion enlightening, Sophia! Thank you for being an active participant. The possibilities of ChatGPT in flood modeling are indeed exciting, and I'm thrilled to have contributed to your knowledge on the topic.
Thank you, Giuseppe, for organizing this insightful discussion. The potential integration of ChatGPT in flood modeling is fascinating, and I'm excited to see how it develops.
You're welcome, Emma! I appreciate your participation and enthusiasm. The integration of ChatGPT in flood modeling is indeed fascinating, and as it develops, we can expect new insights and advancements in the field.
The potential for ChatGPT to revolutionize flood modeling cannot be overstated. Thank you, Giuseppe, for this engaging discussion.
You're absolutely right, Oliver! The potential of ChatGPT to revolutionize flood modeling is immense. I'm grateful to have had the opportunity to engage in this discussion with such dynamic participants. Thank you for your active involvement.
Giuseppe, thank you for your guidance and insights throughout this discussion. The potential of ChatGPT in flood modeling is exceptional.
You're absolutely right, Oliver! The potential of ChatGPT in flood modeling is exceptional. I'm grateful for the opportunity to provide guidance and insights throughout this discussion. Thank you for your active involvement.
Giuseppe, your expertise has made this discussion truly worthwhile. I've gained valuable insights into the potential of ChatGPT in flood modeling.
Thank you for your kind words, Mark! I'm honored to have provided valuable insights throughout this discussion. The potential of ChatGPT in flood modeling is vast, and I'm glad to have contributed to your understanding of it.
Giuseppe, thank you for leading this engaging discussion. Your expertise and willingness to answer our questions have been invaluable.
You're welcome, Mark! Leading this engaging discussion and providing my expertise to answer your questions has allowed me to contribute to a better understanding of the potential of ChatGPT in flood modeling. Thank you for your active participation.
Giuseppe, your insights have been enlightening. This discussion has deepened my understanding of the potential of ChatGPT in flood modeling. Thank you.
You're most welcome, Christopher! I'm delighted to have deepened your understanding of the potential of ChatGPT in flood modeling. Engaging discussions like these are vital in driving new perspectives and advancements in the field.
Giuseppe, thank you for moderating this insightful discussion. Your knowledge and expertise in flood modeling and AI have been truly enlightening.
You're most welcome, Christopher! Moderating this insightful discussion and sharing my knowledge and expertise in flood modeling and AI have been an enlightening experience. I'm grateful for your active engagement.
Giuseppe, thank you for sharing your expertise with us. The potential integration of ChatGPT in flood modeling is inspiring and holds significant promise.
You're welcome, Michael! I appreciate your gratitude and enthusiasm. The integration of ChatGPT in flood modeling indeed holds great promise, and I'm excited to explore its potential alongside fellow enthusiasts like you.
Thank you, Giuseppe, for moderating this enlightening discussion. Your expertise in flood modeling and AI has been invaluable.
You're welcome, Michael! I appreciate your kind words. Moderating this enlightening discussion and sharing insights in flood modeling and AI has been a privilege. Thank you for your active involvement.
Thank you, Giuseppe, for leading this informative discussion. Your expertise in the field of flood modeling has been invaluable.
You're welcome, Michael! Being able to lead this informative discussion and contribute my expertise in the field of flood modeling has been a rewarding experience. Thank you for acknowledging its value.
This discussion has been highly informative. Thank you, Giuseppe, for sharing your expert insights on the transformative potential of ChatGPT in flood modeling.
You're very welcome, Olivia! I'm thrilled to have provided highly informative insights throughout this discussion. The transformative potential of ChatGPT in flood modeling is indeed significant, and I'm grateful for your active participation.
Giuseppe, thank you for steering this discussion and providing your expertise. The potential of ChatGPT in flood modeling is truly exciting.
You're very welcome, Olivia! Steering this discussion and providing my expertise on the potential of ChatGPT in flood modeling has been an exciting endeavor. Thank you for your active engagement.
Giuseppe, thank you for your guidance and expertise throughout this enlightening discussion. The potential of ChatGPT in flood modeling is truly remarkable.
You're welcome, Olivia! Providing guidance and expertise throughout this enlightening discussion and shedding light on the remarkable potential of ChatGPT in flood modeling has been a fulfilling endeavor. Thank you for your active participation.
The potential for ChatGPT to revolutionize flood modeling is immense. Thank you, Giuseppe, for this engaging discussion.
I fully agree, Emma! The immense potential of ChatGPT to revolutionize flood modeling is compelling. I'm glad to have been part of this engaging discussion and share my insights with you.
Thank you, Giuseppe, for moderating this engaging discussion. Your knowledge and insights have been enlightening.
You're welcome, Emma! Moderating this engaging discussion and sharing my knowledge and insights on ChatGPT's potential in flood modeling has been a fulfilling experience. Thank you for your participation and enthusiasm.
Giuseppe, thank you for leading this enlightening discussion and sharing your expertise. The potential integration of ChatGPT in flood modeling holds tremendous value.
You're very welcome, Emma! Leading this enlightening discussion and sharing my expertise on the potential integration of ChatGPT in flood modeling has been an illuminating experience. Thank you for your engagement and recognition of its value.
Thank you, Giuseppe, for moderating this valuable discussion. Your expertise has been instrumental in deepening our understanding of the potential of ChatGPT in flood modeling.
You're welcome, Emma! Moderating this valuable discussion and providing my expertise to deepen your understanding of the potential of ChatGPT in flood modeling has been a rewarding experience. Thank you for your active engagement.
Giuseppe, you've provided valuable insights into the potential of ChatGPT in flood modeling. Thank you for sharing your expertise with us.
You're welcome, John! I'm grateful for your recognition of the valuable insights I've shared throughout this discussion. It's through engaging conversations like this that we can collectively explore the potential of ChatGPT in flood modeling.
Giuseppe, thank you for your valuable input in this discussion. The potential impact of ChatGPT in flood modeling is truly intriguing.
You're most welcome, Sophia! I appreciate your kind words and gratitude. The potential impact of ChatGPT in flood modeling is indeed intriguing, and I'm glad to have contributed to this discussion.
Giuseppe, thank you for leading this insightful discussion. Your expertise and guidance have been invaluable.
You're most welcome, Sophia! Leading this insightful discussion and providing my expertise and guidance on the potential of ChatGPT in flood modeling has been a privilege. I appreciate your active involvement.
Giuseppe, thank you for guiding this insightful discussion. Your expertise and willingness to address our questions have been invaluable.
You're most welcome, Sophia! Guiding this insightful discussion and addressing your questions to the best of my abilities has been a pleasure. I appreciate your recognition of the value of my expertise.
Thank you, Giuseppe, for the engaging discussion and for providing insights on the transformative potential of ChatGPT in flood modeling.
You're welcome, Liam! I'm pleased to have engaged in this discussion and provided insights on the transformative potential of ChatGPT in flood modeling. Thank you for your active participation.
Thank you, Giuseppe, for moderating this insightful discussion. Your expertise has been instrumental in deepening our knowledge of the potential of ChatGPT in flood modeling.
You're welcome, Liam! Moderating this insightful discussion and sharing my expertise to deepen your knowledge of ChatGPT's potential in flood modeling has been a gratifying experience. Thank you for your engagement and interest.
Thank you, Giuseppe, for moderating this informative discussion. Your expertise and insights have been invaluable.
You're welcome, Liam! Moderating this informative discussion and providing my expertise and insights on the potential of ChatGPT in flood modeling have allowed me to contribute to your knowledge and understanding. Thank you for acknowledging their value.
Giuseppe, thank you for organizing this enlightening discussion. I've gained valuable knowledge about the potential of ChatGPT in flood modeling.
You're very welcome, Ava! I'm thrilled to have organized and participated in this enlightening discussion. Knowing that you've gained valuable knowledge about the potential of ChatGPT in flood modeling makes it all the more worthwhile.
Giuseppe, thank you for facilitating this informative discussion. Your insights and expertise are greatly appreciated.
You're welcome, Ava! Facilitating this informative discussion and providing insights and expertise on ChatGPT's potential in flood modeling has been a rewarding endeavor. Thank you for your active participation and appreciation.
Giuseppe, thank you for steering this discussion and providing us with valuable insights. Your expertise in flood modeling and AI is commendable.
You're welcome, Ava! Steering this discussion and providing valuable insights on the potential of ChatGPT in flood modeling with my expertise in the field has been a privilege. I appreciate your recognition of it.
Thank you all once again for your valuable insights and contributions to this discussion. It has been a pleasure engaging with you and exploring the possibilities of leveraging ChatGPT in flood modeling. Let's continue to embrace innovation and drive positive change in the field.
Could ChatGPT also help in real-time flood monitoring? It could potentially complement automated sensor systems and provide additional context based on the collected data.
Great point, Emma! ChatGPT can indeed be leveraged alongside automated sensor systems to enhance real-time flood monitoring. By incorporating additional context and insights, it can contribute to a more comprehensive understanding of flood dynamics.
I hadn't thought about the potential of ChatGPT in real-time flood monitoring. That would be incredibly useful in assisting emergency response teams and decision-makers during flood events.
Absolutely, Sara! Real-time flood monitoring is a critical aspect of emergency response and decision-making. ChatGPT's potential to provide context and assist in analyzing flood data can indeed be immensely valuable in such scenarios.
ChatGPT could also facilitate better communication and coordination among different stakeholders involved in flood management. Collaboration and knowledge sharing are essential in mitigating flood risks.
You're absolutely right, David! Collaboration and knowledge sharing play a vital role in effective flood risk management. ChatGPT's ability to facilitate communication among diverse stakeholders can foster greater collaboration and improve overall flood management strategies.
The potential applications of ChatGPT in the flood management domain seem limitless. I'm excited to witness the integration of this technology in actual practice.
Indeed, Emily! The potential applications of ChatGPT in flood management are vast and hold immense promise. As this technology is further developed and integrated into practical solutions, we can expect exciting advancements in the field.
Thank you, Giuseppe, for your insights and for the engaging discussion. I've gained a deeper understanding of the potential of ChatGPT in flood modeling.
You're welcome, John! I'm glad the discussion has provided you with a deeper understanding of the potential of ChatGPT in flood modeling. Thank you for your active participation and attentive engagement.
Giuseppe, thank you for your thoughtful responses and for facilitating this informative discussion. The potential of ChatGPT in flood modeling is truly remarkable.
You're most welcome, Jane! Facilitating this informative discussion and providing thoughtful responses on the remarkable potential of ChatGPT in flood modeling has been a fulfilling experience. Thank you for your involvement and appreciation.
The potential integration of ChatGPT in flood modeling holds great promise. Thank you, Giuseppe, for your insightful contributions to this discussion.
You're welcome, Lucas! I appreciate your recognition of the promise held by the integration of ChatGPT in flood modeling. Thank you for your engagement in this discussion and for acknowledging my contributions.
Thank you, Giuseppe, for guiding this enlightening discussion. Your expertise and insights have been invaluable.
You're welcome, Sophia! Guiding this enlightening discussion and sharing my expertise and insights on the potential of ChatGPT in flood modeling has been a gratifying experience. Thank you for acknowledging their value.
Giuseppe, thank you for your valuable input in this discussion. The potential of ChatGPT in flood modeling is compelling.
You're welcome, Mason! I appreciate your gratitude and recognition of the compelling potential of ChatGPT in flood modeling. Thank you for your active involvement in this discussion.
Thank you, Giuseppe, for guiding this informative discussion. The transformative potential of ChatGPT in flood modeling is evident.
You're very welcome, Zoe! Guiding this informative discussion and highlighting the transformative potential of ChatGPT in flood modeling has been an enlightening experience. Thank you for your acknowledgment.
Giuseppe, thank you for moderating this insightful discussion. Your expertise and guidance have been invaluable.
You're most welcome, Sophia! Moderating this insightful discussion and providing my expertise and guidance on the potential of ChatGPT in flood modeling have been a rewarding experience. Thank you for your gratitude and participation.
This discussion has provided a wealth of insights. Thank you, Giuseppe, for moderating and sharing your expertise on ChatGPT in flood modeling.
You're welcome, Nathan! I'm glad you found this discussion insightful and gained a wealth of insights into ChatGPT's potential in flood modeling. Thank you for your acknowledgment of my role as moderator and expert contributor.
Giuseppe, thank you for guiding this enlightening discussion. The potential of ChatGPT in flood modeling is truly fascinating.
You're welcome, Helena! Guiding this enlightening discussion and shedding light on the fascinating potential of ChatGPT in flood modeling has been a gratifying experience. Thank you for your engagement and recognition.
Thank you, Giuseppe, for moderating this insightful discussion. The potential of ChatGPT in flood modeling is truly remarkable.
You're welcome, Lucas! I'm pleased to have moderated this insightful discussion and emphasized the remarkable potential of ChatGPT in flood modeling. Thank you for your active participation and acknowledgement.
Giuseppe, thank you for overseeing this enriching discussion. Your expertise and insights have been instrumental in broadening our understanding of the potential of ChatGPT in flood modeling.
You're welcome, Sophia! Overseeing this enriching discussion and providing my expertise and insights on the potential of ChatGPT in flood modeling with the aim of broadening your understanding has been a rewarding endeavor. Thank you for acknowledging their value.
Giuseppe, thank you for guiding this insightful discussion. The possibilities of ChatGPT in flood modeling are evident.
You're welcome, Mason! Guiding this insightful discussion and highlighting the possibilities of ChatGPT in flood modeling has been an enlightening experience. Thank you for recognizing the evident potential.
Thank you, Giuseppe, for sharing your expertise and insights during this discussion. The potential of ChatGPT in flood modeling is remarkable.
You're welcome, Zoe! Sharing my expertise and insights during this discussion to highlight the remarkable potential of ChatGPT in flood modeling has been a fulfilling experience. Thank you for acknowledging the value of my contributions.
Could ChatGPT be used to assist in flood forecasting? It could potentially analyze various data sources and help predict flood events.
Great question, Mason! ChatGPT can indeed play a role in flood forecasting by leveraging its ability to analyze diverse data sources and assist in predicting flood events. Its capability to consider multiple factors and provide context can contribute to more accurate forecasts.
The potential of ChatGPT in flood forecasting is intriguing. It could potentially aid in issuing timely warnings and ensuring communities are prepared for flood events.
Absolutely, Helena! ChatGPT's potential in flood forecasting is indeed intriguing. By aiding in issuing timely warnings and enhancing preparedness, it can contribute to more effective flood event management and help save lives.
Having ChatGPT analyze various datasets for flood forecasting could also help identify historical patterns and trends, contributing to a more comprehensive understanding of flood dynamics in a specific region.
Well said, Sophia! Analyzing various datasets using ChatGPT in flood forecasting can indeed help identify historical patterns and trends. This, in turn, enables a more comprehensive understanding of flood dynamics in a specific region and facilitates more informed decision-making.
Thank you for your insightful responses, Giuseppe. The potential applications of ChatGPT in flood modeling are truly diverse and hold immense value.
You're welcome, Mason! I'm grateful for your appreciation of my insightful responses. The diverse potential applications of ChatGPT in flood modeling indeed hold immense value. Thank you for acknowledging it.
Giuseppe, I want to express my gratitude for guiding this enlightening discussion. The potential of ChatGPT in flood modeling is immense, and your expertise has provided valuable insights.
You're most welcome, Helena! Guiding this enlightening discussion and providing valuable insights into the immense potential of ChatGPT in flood modeling has been a rewarding experience. I appreciate your gratitude and acknowledgement of my expertise.
Giuseppe, thank you for your expertise and thoughtful responses throughout this discussion. The potential of ChatGPT in flood modeling is truly transformative.
You're welcome, Sophia! I'm thrilled to have shared my expertise and provided thoughtful responses throughout this discussion. That the potential of ChatGPT in flood modeling is truly transformative is evident, and I'm grateful for your acknowledgement.
The potential of ChatGPT in flood modeling has become much clearer thanks to this discussion. Thank you, Giuseppe, for your insights and expertise.
You're welcome, Mason! Playing a part in helping you gain a clearer understanding of the potential of ChatGPT in flood modeling through this discussion has been a rewarding experience. Thank you for your appreciation and recognition.
Giuseppe, thank you for your knowledgeable contributions and valuable insights throughout this discussion. The potential of ChatGPT in flood modeling is truly remarkable.
You're very welcome, Helena! I've appreciated the opportunity to contribute my knowledge and valuable insights to this discussion on the remarkable potential of ChatGPT in flood modeling. Thank you for your active engagement and recognition.
Thank you everyone for joining the discussion on my blog article. I'm excited to hear your thoughts on leveraging ChatGPT's potential in flood modeling technology!
This is such an interesting topic! I can see how using ChatGPT can greatly enhance flood modeling technology by leveraging its natural language processing abilities. It can potentially improve communication between researchers and stakeholders involved in flood management.
Absolutely, Maria! Improved communication is one of the key benefits. Flood modeling is a complex domain, and being able to explain model outputs in a more intuitive way could greatly benefit decision-making processes.
I'm a bit skeptical about relying on AI in such crucial matters as flood modeling. How can we ensure the accuracy and reliability of the ChatGPT-generated results? Human expertise is invaluable in these situations.
That's a valid concern, David. While AI can enhance the process, it should always be complemented by human expertise. The idea here is to leverage ChatGPT as a tool to assist experts, facilitate collaboration, and provide insights. Humans will still have the final say in decision-making to ensure accuracy.
I understand your concern, David, but remember that AI technologies like ChatGPT can be trained on large datasets and learn from various existing models and experts' knowledge. So, it has the potential to provide valuable insights that might be overlooked by human experts alone.
You make a good point, Maria. Combining the power of AI with human expertise can indeed bring better results. It's essential to strike the right balance between both for reliable flood modeling.
I'm curious to know if ChatGPT can also take into account dynamic factors like climate change and urban development while modeling floods. These factors have significant impacts on flood scenarios.
Great question, Jonathan! ChatGPT's ability to process and learn from vast amounts of data makes it suitable for considering dynamic factors like climate change and urban development. By incorporating these external factors into the models, flood predictions can be more robust and accurate.
I'm impressed by the potential of ChatGPT in flood modeling. It can improve accessibility to flood information for the general public by providing user-friendly explanations. This can enhance public awareness and engagement in flood prevention efforts.
Absolutely, Sophie! Engaging the general public in flood prevention is crucial. With user-friendly explanations generated by ChatGPT, it becomes easier for non-experts to understand the flood modeling process and take informed actions for their safety.
While ChatGPT seems promising, we should also consider potential ethical concerns. How can we ensure that the AI system is not biased or manipulated to favor certain interests when it comes to flood modeling?
You raise an important point, Daniel. Ethical considerations are crucial when deploying AI systems. Transparency, openness, and rigorous testing are essential to ensure the system's fairness, reliability, and freedom from biases. Multiple stakeholders' involvement in model development can also help address these concerns.
I think leveraging ChatGPT in flood modeling technology can also facilitate interdisciplinary collaboration. It can bridge the gap between domain experts and data scientists, facilitating knowledge exchange and driving innovation.
Indeed, Sophia! Flood modeling requires expertise from multiple domains. ChatGPT can act as a mediator, enabling collaboration and knowledge sharing between experts with different backgrounds, resulting in more integrated and comprehensive flood models.
I appreciate the potential benefits of ChatGPT in flood modeling, but what challenges do you foresee in integrating AI into existing flood modeling systems? Are there any limitations we should be aware of?
Great question, Oliver! Integrating AI into existing flood modeling systems may pose challenges related to data availability, system integration, and model interpretability. Additionally, AI models like ChatGPT might require substantial computational resources. It's important to address such challenges while ensuring interoperability with established systems.
I wonder if ChatGPT can analyze uncertainties in flood modeling. Uncertainty analysis is vital for decision-making. It would be interesting to hear if it can provide insights on uncertainty quantification.
Absolutely, Emily! Uncertainty analysis is crucial in flood modeling. While ChatGPT itself may not directly perform uncertainty quantification, it can assist experts by presenting various scenarios, sensitivity analyses, and supporting discussions to better understand and communicate uncertainties associated with flood predictions.
I like the idea of using AI to enhance flood modeling, but it also brings concerns about cybersecurity. How can we ensure the security of flood modeling systems if we rely heavily on AI like ChatGPT?
You're right, Lucas. Cybersecurity is critical when deploying AI technologies. Protecting data, securing communication channels, and regularly updating and monitoring the system for vulnerabilities are important steps to ensure the security of flood modeling systems. Robust security frameworks need to be in place during AI adoption.
Considering the potential of ChatGPT in flood modeling, I believe it's crucial to ensure that AI-driven flood models are accessible to all communities, regardless of their socioeconomic backgrounds. How can we address potential barriers to access and inclusion?
Excellent point, Sophie! Accessibility and inclusivity should be at the forefront of AI-driven flood modeling. It's essential to ensure that the technology reaches all communities by providing multiple access channels, using plain language, and considering the needs and limitations of various user groups. Collaborating with local communities is also important for addressing specific accessibility challenges.
ChatGPT's potential in flood modeling is exciting. It can revolutionize the way we analyze and communicate flood risks, enabling more efficient and informed decision-making processes. However, we should continue to validate and refine these AI-driven models with real-world data to ensure their reliability.
Absolutely, Jonathan! Validation and refinement with real-world data are crucial for the reliability of AI-driven flood models. Continuous improvement and close collaboration between experts, stakeholders, and AI practitioners will lead to more accurate and trustworthy flood modeling results.
I understand the potential benefits of ChatGPT in flood modeling, but we should also consider the digital divide. How can we ensure that all communities have access to the technology needed to leverage these AI-driven models?
You bring up an important concern, David. Bridging the digital divide should be a priority. Besides accessibility, efforts should be made to provide necessary infrastructure, training, and support to ensure that all communities, including those in underprivileged areas, can access and benefit from AI-driven flood modeling technology.
ChatGPT can be a game-changer in flood modeling, but we should also consider potential biases present in the training data. How can we ensure that biased data doesn't influence the AI models and compromise decision-making?
You're right, Sophia. Bias in training data can be a challenge. It's crucial to curate diverse and representative datasets and conduct rigorous bias analysis during model development. Regular audits, transparency in the model's decision-making, and involving multiple stakeholders can help identify and rectify any biases that may have been inadvertently learned by ChatGPT.
I'm concerned about potential model interpretability issues with ChatGPT. How can we ensure that the reasoning behind flood modeling predictions can be understood and trusted by both experts and the general public?
Interpretability is crucial, Emily. AI models like ChatGPT should be able to provide explanations for their predictions. Research on explainable AI and incorporating techniques that make the model's decision-making process transparent can help address interpretability concerns. Additionally, providing clear and concise explanations to users is essential to build trust in the flood modeling predictions generated by ChatGPT.
ChatGPT certainly has the potential to revolutionize flood modeling. It can assist in generating insights, improving communication, and involving stakeholders in the decision-making process. Collaboration between experts, technologists, and policymakers will be key to realizing these benefits.
Well said, Jonathan! Collaboration and interdisciplinary partnerships will be essential in harnessing ChatGPT's potential for flood modeling. By working together, we can overcome challenges, address concerns, and drive innovation to create more resilient and effective flood management strategies.
I agree with the potential benefits of ChatGPT in flood modeling, but we must not overlook the importance of domain expertise. Human experience and insights are crucial while interpreting the AI-generated results.
Absolutely, Oliver! AI should be seen as a supporting tool rather than a replacement for human expertise. Combining the strengths of AI models like ChatGPT with the knowledge and experience of domain experts will lead to more accurate and reliable flood modeling outcomes.
Considering the potential of ChatGPT in flood modeling, it's important to address the challenges related to data privacy. How can we ensure the privacy and confidentiality of sensitive data involved in flood modeling?
You raise a vital concern, Daniel. Protecting data privacy in flood modeling is crucial. Adopting strong encryption measures, following data protection standards, and ensuring strict access controls are some of the steps that can be taken to safeguard sensitive data involved in flood modeling processes.
I'm excited about the potential of ChatGPT in flood modeling, but we should also consider its limitations. AI models can be sensitive to input data quality. How can we ensure high-quality data for robust flood modeling?
You're right, Lucas. High-quality data is vital for robust flood modeling. Adequate data collection methods, data validation processes, and continuous monitoring to identify errors or biases are crucial to ensure the data's quality. Collaboration with domain experts can help refine data collection approaches and improve the overall quality of flood modeling datasets.
While AI can be a powerful tool, it's important to address public perception and trust issues. How can we ensure that the general public has confidence in the AI-driven flood modeling technology?
Building trust in AI-driven flood modeling is crucial, David. Openness about the limitations and capabilities of AI, ensuring transparency in the decision-making process, and actively involving the public in understanding the technology and its benefits can help foster confidence. Engaging stakeholders, addressing concerns, and maintaining clear communication channels will contribute to public trust.
I see the potential of AI in flood modeling, but there's also a need to consider the impact on job roles in the field. How can we ensure a smooth transition to AI-driven flood modeling without negatively affecting experts working in the domain?
You bring up a valid concern, Sophia. While AI can automate certain aspects of flood modeling, it should be seen as a tool to augment human capabilities rather than replace experts. Upskilling programs, providing training opportunities, and involving experts in AI adoption and development can help ensure a smooth transition that benefits both the field and the professionals working in it.
I'm excited about the potential of ChatGPT in flood modeling. It can be a valuable tool for efficient decision-making and enhancing flood preparedness. I look forward to seeing future developments in this field!
Thank you, Maria! I share your excitement. The potential of ChatGPT in flood modeling is vast, and it opens up new possibilities for improving flood preparedness and mitigation strategies. With continued research and collaboration, we can make significant strides in this field.
I applaud your efforts, Giuseppe, in bringing forth the potential of ChatGPT in flood modeling. It's inspiring to see how AI technologies can be harnessed to tackle complex challenges and make a positive impact on society. Keep up the excellent work!
Thank you for your kind words, Jonathan! I truly believe in the transformative potential of ChatGPT in flood modeling. By collaborating and pushing the boundaries, we can revolutionize how we manage and mitigate floods, and ultimately build more resilient communities.
The discussion here has highlighted the importance of collaboration, addressing concerns, and striving for transparency while leveraging AI in flood modeling. I'm grateful for the insights shared by everyone in this engaging conversation!
Absolutely, Oliver! Collaborative discussions like these are essential in shaping the future of AI-driven flood modeling. I appreciate everyone's thoughtful contributions and valuable perspectives. Let's continue to explore the potential and work together towards a more resilient future!
Thank you all once again for your participation in this discussion. Your insights and questions have enriched the conversation on leveraging ChatGPT's transformative potential in flood modeling technology. Let's stay connected and continue pushing the boundaries of innovation in flood management!