Revolutionizing IR Spectroscopy: Unleashing the Power of ChatGPT in Technological Advancements
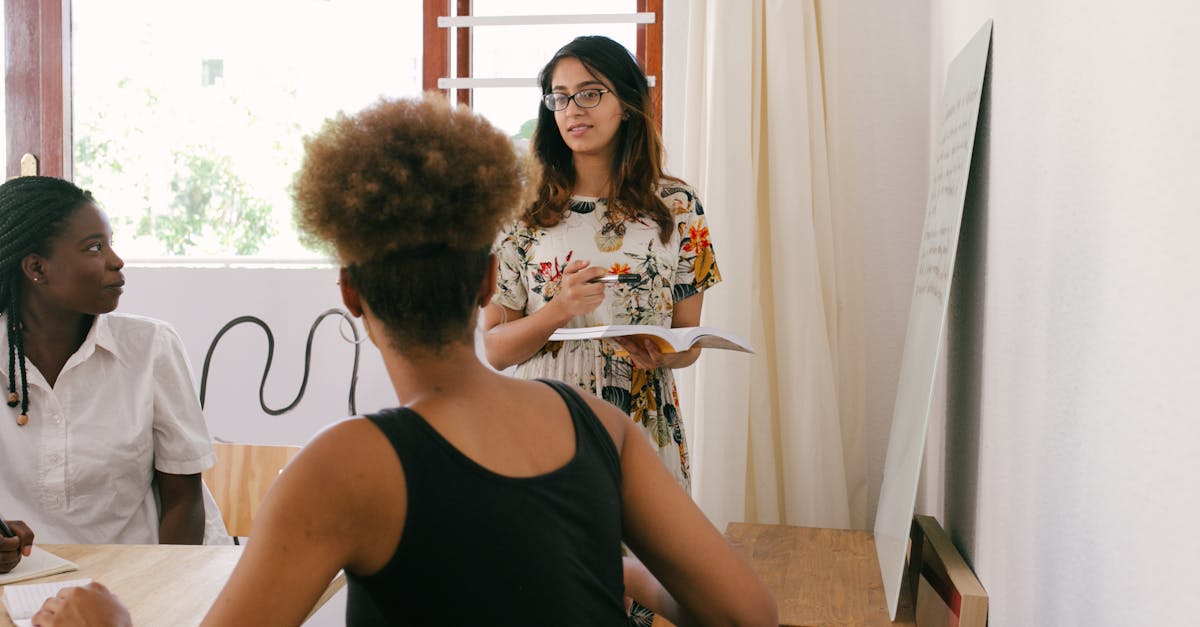
In the realm of analytical technology, Infrared (IR) spectroscopy has emerged as a powerful tool in identifying and quantifying molecular species. The precision, reliability, and efficiency brought by IR spectroscopy has expanded its application to varied sectors which includes pharmaceutical, petrochemical, environmental monitoring, and many others.
However, while IR spectroscopy is exceptional in producing rich and descriptive data, the complexity and sheer volume of the resulting datasets often pose significant challenges when it comes to interpretation and analysis. This is where tools like OpenAI’s ChatGPT-4 can be invaluable.
Understanding IR Spectroscopy
Harnessed primarily for molecular identification and quantification, IR Spectroscopy works on the principle of molecular vibrational modes. When infrared radiation is passed through a sample, certain wavelengths are absorbed by the molecules, causing them to vibrate, rotate or bend. The resulting absorption spectrum, which shows the absorption of radiation at specific wavelengths, creates a unique 'fingerprint' that can identify and quantify individual molecular components in the sample.
The Challenge of Data Interpretation
Interactive and rich as these IR spectra may be, they can also be incredibly complex. When dealing with large samples or mixtures, for example, the resulting spectra can contain a huge volume of overlapping peaks and patterns. Interpreting this data manually can be time-consuming and is prone to errors, even for the most experienced analysts.
Leveraging ChatGPT-4 for Data Interpretation
The recent development in AI technology offers an innovative solution to this problem. Specifically, ChatGPT-4, developed by OpenAI, is an advanced language model that can interpret and understand text at a human-like level. But not only that, it can also help in interpreting data, identifying patterns, and even making predictions based on the data it has analyzed. This makes it an excellent tool for interpreting and analyzing large spectral datasets produced by IR spectroscopy.
The process starts with feeding the spectral data into the AI model. Due to its sophisticated neural network architectures, ChatGPT-4 can learn to understand and predict the patterns inherent in the spectral data. It can identify overlaps and isolate specific molecular 'fingerprints', significantly speeding up the process and eliminating manual error.
Moreover, the AI's advanced language capabilities mean it can also present its findings in a comprehensible and accessible manner. This can range from a straightforward identification of molecular components to indications of probable errors, or likely ranges of concentration. As a result, using ChatGPT-4 could not only improve the efficiency and accuracy of analysis but could also help in decision-making and strategy formulation based on the interpreted data.
Conclusion
The potential for using AI models like ChatGPT-4 in data interpretation is immense. Its ability to handle complex data sets, determine patterns and present findings in an understandable manner could be a game-changer in fields that rely heavily on advanced analytical technologies like IR spectroscopy.
As we further refine and evolve these AI technologies, we'll be able to address—and conquer—even greater analytical challenges in the near future.
Comments:
Thank you all for your thoughtful comments and insights on my article! I'm glad to see such engaged discussion.
I found your article on Revolutionizing IR Spectroscopy fascinating, Galen! Can you elaborate more on how ChatGPT is being used in this field?
Certainly, Eleanor. ChatGPT is being leveraged in IR spectroscopy to enhance data interpretation and analysis. It can assist in real-time spectrum analysis and provide insights into sample composition and structural properties.
This new application of ChatGPT in IR spectroscopy opens up exciting possibilities! How reliable is the analysis provided by this AI model?
That's a great question, Rohan. The reliability depends on the training data and fine-tuning process. By incorporating a diverse range of spectra and extensive model validation, we can achieve high accuracy. However, it's important to note that human validation is still necessary for critical analysis.
I'm curious, Galen, what are some potential applications or industries that could benefit the most from this technology?
Excellent question, Amanda. The applications of ChatGPT in IR spectroscopy are vast. It can significantly benefit pharmaceutical research, environmental analysis, material characterization, and even forensics. It enables faster data interpretation and assists researchers in making more accurate decisions.
I can see how ChatGPT can speed up the analysis process, but could it potentially replace human expertise in this field?
You bring up an important point, Maria. While ChatGPT enhances the analysis process, it should not replace human expertise. The AI model serves as a powerful tool to assist scientists but cannot fully replicate the experience, knowledge, and critical thinking abilities of human researchers.
This technology sounds promising! Galen, what are some of the challenges faced during the integration of ChatGPT into the field of IR spectroscopy?
Indeed, Jeremy. We encountered challenges related to training data availability, ensuring model interpretability, and fine-tuning for various sample types. Addressing these challenges required collaboration between AI researchers and spectroscopy experts to develop optimized and reliable analyses.
Galen, I'm curious about the limitations of using ChatGPT in IR spectroscopy. Can you shed some light on that?
Absolutely, Sophia. While ChatGPT offers remarkable analysis capabilities, its performance might be limited when encountering rare or outlier sample spectra. Additionally, it is crucial to have a strong foundation of accurate training data for optimal results.
I wonder if ChatGPT could be integrated with other spectroscopic techniques to enhance their analysis as well. Any thoughts on that, Galen?
You're onto something, Nathan. Combining ChatGPT with other spectroscopic techniques could potentially yield more comprehensive analyses. It opens avenues for multi-modal analysis and synergistic insights into samples, leading to enhanced problem-solving capabilities.
Considering the vast amount of data generated in IR spectroscopy, how does ChatGPT handle data storage and processing requirements?
A valid concern, Emily. Handling large datasets requires robust computational resources. ChatGPT leverages modern hardware accelerators and cloud-based infrastructure to manage the storage and processing demands efficiently, enabling faster and scalable analyses.
Very exciting advancements! Galen, how does the interpretability of ChatGPT's analysis hold up compared to traditional IR spectroscopy methods?
Interpretability is crucial, Jason. While traditional IR spectroscopy methods offer well-established interpretive frameworks, ChatGPT brings additional insights that may require further validation. Understanding the underlying AI model's decisions and potential biases is an ongoing area of research and development.
Galen, do you think ChatGPT will eventually become a standard tool in IR spectroscopy laboratories?
It's an interesting prospect, Lily. As technology advances and its reliability improves, ChatGPT could become a valuable tool in IR spectroscopy laboratories. However, its integration and adoption would depend on the specific requirements, resources, and preferences of individual research groups.
Thank you all once again for your engaging comments and questions! I hope this article has sparked your interest in the potential of ChatGPT in revolutionizing IR spectroscopy.
Galen, thank you for shedding light on the use of ChatGPT in IR spectroscopy. The future possibilities sound incredibly promising!
You're very welcome, Kyle! I'm glad you found the possibilities exciting. ChatGPT has tremendous potential to reshape the field of IR spectroscopy and drive breakthroughs in various scientific disciplines.
Thank you, Galen, for sharing your insights. It's fascinating to see how AI is advancing various scientific fields.
You're welcome, Olivia! AI indeed holds enormous potential in advancing scientific research, and it's always exciting to witness its contributions across different domains.
Great article, Galen! It's impressive to see how ChatGPT is being employed in such innovative ways.
Thank you, Richard! ChatGPT's versatility allows it to be applied across various disciplines, bringing new possibilities and advancements.
Fantastic article! I'm eager to learn more about the practical implementation of ChatGPT in IR spectroscopy.
I appreciate your enthusiasm, Lucy! The practical implementation involves training the AI model on a diverse range of spectra, validating its accuracy, and integrating it into existing spectroscopy workflows.
Galen, do you foresee any ethical considerations or challenges with the use of ChatGPT in this field?
Ethical considerations are essential, Isaac. Ensuring transparency, avoiding bias in training data, and addressing potential ethical implications are all critical aspects. The responsible development and deployment of AI in IR spectroscopy must always be a priority.
ChatGPT's applications in IR spectroscopy are truly intriguing! Galen, how do you envision this technology evolving in the next few years?
It's an exciting prospect, Thomas. In the coming years, I envision ChatGPT evolving to handle more complex spectra analysis, improving interpretability, and further augmenting researchers' capabilities beyond just IR spectroscopy. The potential for advancements is immense.
Thank you, Galen, for this enlightening article! It showcases a new dimension of AI's impact on scientific advancements.
You're welcome, Emma! AI's impact on scientific advancements is indeed multi-faceted, and I'm thrilled to explore its potential in the context of IR spectroscopy.
Really fascinating read, Galen! ChatGPT's application in IR spectroscopy is an exciting development for researchers in the field.
I appreciate your kind words, Nolan! ChatGPT's application in IR spectroscopy brings advancements that can significantly benefit researchers, opening up new avenues of exploration.
Great article, Galen! The integration of AI in scientific research never fails to amaze me.
Thank you, Zara! The integration of AI undeniably has the potential to revolutionize scientific research across numerous disciplines, including IR spectroscopy.
Galen, how does ChatGPT handle the analysis of complex chemical structures in IR spectroscopy?
Complex chemical structures can be challenging, Aiden. While ChatGPT offers insights into structure analysis, its performance may vary depending on the available training data specific to those structures. Incorporating a comprehensive range of spectra during model training is vital for better results.
I'm amazed by the potential of ChatGPT in IR spectroscopy! How widely has it been adopted in research labs so far?
The adoption of ChatGPT in research labs is progressing, Grace. While it's difficult to provide an exact figure, its applications are garnering significant interest, and several labs have already started exploring its implementation. Its full-scale adoption will depend on further advancements, validations, and specific research needs.
Galen, what are some of the key advantages of using ChatGPT over traditional techniques in IR spectroscopy?
ChatGPT offers several advantages, Clara. Its real-time analysis capabilities, ability to handle vast data volumes, and assistance in decision-making make it an invaluable tool. Furthermore, it complements traditional techniques by bringing novel perspectives and more efficient analysis methods.
Fantastic article, Galen! The potential of ChatGPT in IR spectroscopy is truly remarkable.
I appreciate your kind words, Harrison! The potential of ChatGPT in IR spectroscopy has already shown promising results and paves the way for exciting advancements in the field.
This article has sparked my curiosity, Galen! Are there any specific research papers or studies that delve deeper into this topic?
Certainly, Ellie! I can provide you with a list of research papers and studies that dive deeper into the integration of ChatGPT in IR spectroscopy. I'll share the references as a follow-up to this discussion.
It's incredible to see how AI technology like ChatGPT is reshaping the world of scientific research! Great article, Galen.
Thank you, Aaron! The transformative potential of AI in scientific research is indeed awe-inspiring, and I'm glad you found the article insightful.
Galen, I'm curious about the limitations of using an AI model like ChatGPT. Can you shed some light on that?
Absolutely, Maya. ChatGPT's limitations lie in its reliance on the training data it's exposed to. It may struggle with rare or outlier spectra, and the quality of results is strongly influenced by the diversity and accuracy of the data it's trained on.
Great article, Galen! I'm thrilled to see how AI is contributing to advancements in scientific research.
Thank you, Levi! AI's contributions to scientific research are undoubtedly remarkable, and it's exciting to witness the positive impact it brings to various fields.
ChatGPT's role in revolutionizing IR spectroscopy is truly impressive! What are the major challenges you anticipate during its widespread adoption?
Widespread adoption presents challenges, Sarah. Ethical considerations, meeting diverse research requirements, and ensuring cross-platform compatibility are some of the key challenges. Addressing these will require collaboration between AI developers, spectroscopists, and regulatory bodies.
Galen, how long does it take to train ChatGPT for IR spectroscopy and fine-tune its performance?
Training and fine-tuning ChatGPT for IR spectroscopy is a time-consuming process, Leo. It typically requires weeks, or even months, to train the model using a vast dataset of spectroscopic information. Fine-tuning its performance involves multiple iterations and evaluations.
Thank you, Galen, for sharing this insightful article! The potential of ChatGPT in IR spectroscopy is truly fascinating.
You're welcome, Hannah! I'm delighted that you found the potential of ChatGPT in IR spectroscopy fascinating. It's an exciting time for advancements in scientific research.
Fascinating read, Galen! The utilization of AI models in scientific fields is paving the way for groundbreaking discoveries.
Thank you, Henry! AI models like ChatGPT are undoubtedly driving groundbreaking discoveries in scientific research, opening up new pathways for exploration.
Galen, what are some of the potential implications of incorporating ChatGPT in real-time IR spectroscopic analysis?
Incorporating ChatGPT in real-time IR spectroscopic analysis can have significant implications, Eva. It enables faster decision-making, enhanced accuracy, and the ability to analyze large volumes of data more efficiently. This can lead to faster scientific discoveries and aid in applications requiring rapid analysis.
Great article, Galen! It's intriguing to see how AI is being applied in innovative ways across scientific fields.
Thank you, Alex! The innovative applications of AI in scientific fields like IR spectroscopy are indeed captivating, and we've only scratched the surface of its potential.
This article has expanded my understanding of AI's impact, Galen! What role does human validation play in ChatGPT's analysis?
Human validation is crucial, Elizabeth. While ChatGPT can provide insightful analysis, it's important to have human experts validate the results for critical decision-making. Combining the power of AI with human expertise ensures a more accurate and reliable outcome.
Galen, do you anticipate any potential challenges in integrating ChatGPT with existing spectroscopy instruments or software platforms?
Integration can present challenges, Natalie. Ensuring compatibility with existing instruments and software platforms, and developing seamless interfaces, are among the primary challenges. Collaborative efforts between AI and spectroscopy experts are key to overcoming these challenges.
Intriguing article, Galen! I'm curious about the computational resources required to implement ChatGPT in IR spectroscopy labs.
Implementing ChatGPT in IR spectroscopy labs necessitates robust computational resources, Lucas. High-performance hardware accelerators, such as GPUs, and cloud-based infrastructure are typically employed to handle the substantial data processing requirements efficiently.
Thank you, Galen! Your article sheds light on the immense potential of AI in scientific research. It's a thrilling time for advancements.
You're welcome, Elena! It's indeed a thrilling time, with AI's potential revolutionizing scientific research across various domains. I'm glad you enjoyed the article!
The integration of ChatGPT in IR spectroscopy is groundbreaking! Galen, do you envision this technology being applied beyond lab settings?
The possibilities are exciting, Nathan. While lab settings initially benefit from ChatGPT's integration, there's potential for its application beyond labs. However, it would need careful consideration, validation, and adaptation to different environments before widespread adoption in non-lab settings.
This article has ignited my curiosity, Galen! What are the primary factors considered during the fine-tuning process for ChatGPT's performance?
Fine-tuning ChatGPT's performance involves iteratively optimizing several factors, Evelyn. Some key considerations include dataset diversity, model architecture tuning, hyperparameter optimization, and ensuring a range of performance metrics meet acceptable thresholds. It's an intricate process that requires rigorous experimentation and evaluation.
Galen, do you foresee any challenges in gaining acceptance and trust for AI-assisted analysis among the scientific community?
Building acceptance and trust is indeed a challenge, Julian. Demonstrating rigorous validation, the reliability of results, and transparency in the analysis process will be crucial. Collaborative efforts between AI researchers and spectroscopists in showcasing the value of AI-assisted analysis can help gain acceptance and trust within the scientific community.
Intriguing article, Galen! What steps do you recommend for scientists who are interested in incorporating ChatGPT into their spectroscopic research?
For scientists interested in adopting ChatGPT in their spectroscopic research, I recommend starting with understanding the model's capabilities and limitations, exploring available training data, and validating the results against known spectra. Collaborating with experts who have experience in AI integration can also be invaluable for a successful implementation.
Great article, Galen! How do you envision ChatGPT's potential impact on the field of IR spectroscopy in terms of speeding up research processes?
ChatGPT's potential impact on speeding up research processes is significant, Dylan. By assisting with real-time analysis, automated decision-making, and data interpretation, it accelerates the overall research workflow. This enables researchers to focus more on advanced analyses and experimental design.
The integration of AI models like ChatGPT in scientific research is truly transformative, Galen! How can scientists approach the validation process for AI-assisted analyses?
Validation is a crucial step, Sophie. Scientists can approach it by comparing AI-assisted results with independently obtained spectroscopic data, validating against established spectra information, and conducting blind tests with known samples. Collaborating with expert spectroscopists can provide valuable insights and ensure robust validation.
Impressive article, Galen! ChatGPT's role in revolutionizing IR spectroscopy is a testament to the power of AI in scientific advancements.
Thank you, Isla! AI's role in scientific advancements, exemplified by ChatGPT's application in IR spectroscopy, holds immense potential to reshape various fields and accelerate discoveries.
Galen, what are some of the key criteria to consider when selecting and preparing training data for ChatGPT in IR spectroscopy?
Selecting and preparing training data is crucial, Asher. Some key criteria include data diversity, capturing various sample types and environmental conditions, ensuring accurate labels and metadata, avoiding biased training sets, and incorporating spectroscopic expertise to curate comprehensive datasets that align with the research goals.
This article has broadened my knowledge, Galen! What role does ChatGPT play in the education and training of spectroscopists?
ChatGPT can have a role in educating and training spectroscopists, Skylar. It can serve as an interactive tool for students, provide quick access to expert-level insights, and aid in the training of beginner spectroscopists. However, it must be supplemented with traditional learning methods and real-world laboratory experience.
Impressive article, Galen! How does ChatGPT handle multi-modal analysis when dealing with IR spectra?
Multi-modal analysis with IR spectra can be handled by ChatGPT, Rowan. By integrating information from different spectroscopic techniques, such as mass spectrometry or Raman spectroscopy, ChatGPT can provide enhanced insights into samples, enabling a more comprehensive understanding of their characteristics.
Galen, do you foresee any potential impact of ChatGPT's implementation in IR spectroscopy on developing countries' scientific research capabilities?
The potential impact of ChatGPT's implementation in IR spectroscopy on developing countries' scientific research capabilities is substantial, Brooklyn. By providing access to advanced analysis capabilities, even in resource-constrained settings, it levels the playing field to some extent and fosters scientific advancements worldwide.
I'm excited about the potential of ChatGPT in IR spectroscopy, Galen! How can researchers overcome the challenges of limited training data for specific sample types?
Limited training data for specific sample types can be addressed through collaboration and data sharing, Caleb. Researchers can pool resources, create data-sharing initiatives, and work in interdisciplinary teams to amass sufficient training data for diverse sample types. A collective effort aids in overcoming the challenge and realizing the optimum potential of AI in IR spectroscopy.
Thank you all for reading my article on Revolutionizing IR Spectroscopy with ChatGPT! I'm excited to hear your thoughts and opinions.
Great article, Galen! The potential of incorporating ChatGPT in IR Spectroscopy sounds promising. It could revolutionize the way we analyze and interpret IR spectra.
Thank you, Natalie! I completely agree. By leveraging the power of ChatGPT, we can expect significant advancements in the field of IR Spectroscopy.
Interesting article, Galen. While I understand the potential benefits, I'm curious about the challenges and limitations ChatGPT might face in the context of IR Spectroscopy.
That's a great question, Adam. ChatGPT, like any AI model, can face limitations in accurately understanding context-specific scientific jargon. Ensuring an extensive training dataset with domain-specific knowledge will be crucial to overcome this.
I see immense possibilities in incorporating ChatGPT into IR Spectroscopy. It could assist researchers in identifying complex molecular structures more efficiently!
Absolutely, Erica! ChatGPT's natural language processing capabilities combined with its ability to learn from vast amounts of data can indeed aid researchers in molecular structure identification.
I have some concerns about the potential biases ChatGPT might introduce into the analysis. How can we ensure accurate and unbiased results?
Valid point, Alexis. Bias in AI models is a critical concern. It is crucial to train ChatGPT on diverse and representative datasets while regularly monitoring and mitigating any biases that might arise during its application in IR Spectroscopy.
It's fascinating how AI technologies are being integrated into various scientific fields. Galen, do you think ChatGPT can also be used in other spectroscopic techniques?
Absolutely, Natalie! The principles behind ChatGPT can be applied to various spectroscopic techniques, opening doors for advancements and improved analysis across different domains.
While ChatGPT offers exciting possibilities, how can we ensure the privacy and security of sensitive research data while using this AI model?
Excellent question, Andrew. To ensure data privacy and security, it is crucial to implement robust encryption protocols and adhere to strict data handling practices when utilizing ChatGPT in scientific research.
The potential of combining AI and spectroscopy is intriguing! Galen, are there any ongoing research projects or practical implementations leveraging ChatGPT in IR Spectroscopy?
Indeed, Emily! While practical implementations are still emerging, several research groups are exploring the application of ChatGPT in automating spectral analysis and interpretation, ultimately improving research efficiency.
Galen, do you have any thoughts on the computational resources required to deploy ChatGPT effectively for IR Spectroscopy?
Great question, Emma. ChatGPT can be resource-intensive, especially when handling large datasets. However, with advancements in cloud computing and efficient hardware, deploying ChatGPT for IR Spectroscopy can be achieved effectively.
This article has piqued my interest! Galen, what are the potential implications of incorporating ChatGPT in IR Spectroscopy for drug discovery?
Drug discovery relies heavily on accurate analysis and interpretation of spectroscopic data. By leveraging ChatGPT, scientists can potentially accelerate the drug discovery process by generating insights and predictions from complex spectral information.
Galen, have there been any comparative studies exploring the accuracy and efficiency of ChatGPT when compared to traditional methods in IR Spectroscopy?
Indeed, Natalie. Comparative studies are vital to assess the superiority of ChatGPT over traditional methods. Several research teams are actively investigating this aspect and initial results show promising performance gains with ChatGPT.
ChatGPT integration in IR Spectroscopy seems exciting! Galen, how can researchers incorporate ChatGPT in their existing analysis pipelines?
Great question, Michael. Integrating ChatGPT into existing analysis pipelines requires developing robust interfaces that allow seamless interaction between researchers and the AI model. By designing user-friendly tools, we can enhance usability and adoption.
I wonder if ChatGPT can be used to predict chemical reactions based on IR spectra. Galen, what are your thoughts on this?
Predicting chemical reactions from IR spectra is an exciting possibility, Erica. While it requires extensive research and model training, ChatGPT's ability to learn patterns in data could assist in making predictions and enhancing reaction analysis.
Considering the potential widespread use, are there any plans to optimize ChatGPT specifically for IR Spectroscopy to maximize its accuracy and utility?
Absolutely, Adam. Many researchers and organizations are actively working towards optimizing ChatGPT for specific scientific applications, including IR Spectroscopy. Tailoring the model's training and fine-tuning will play a crucial role in maximizing its accuracy.
I'm worried about potential biases that could be learned by ChatGPT from training data. Galen, how can we prevent biased predictions while training the model?
Bias is a significant concern, Alexis. Researchers must carefully curate diverse and representative datasets to ensure fair and unbiased training. Additionally, continuously monitoring and evaluating the model's output can help detect and address potential biases.
Galen, how can we overcome potential communication barriers when using ChatGPT for collaborations across different scientific domains?
Excellent question, Andrew. Interdisciplinary collaborations can indeed face communication challenges. By investing in proper documentation, shared understanding, and fostering open conversations, researchers can bridge the gap and enable effective cross-domain collaborations using ChatGPT.
I'm excited about the future possibilities. Galen, what are your thoughts on the integration of ChatGPT as a standard tool in IR Spectroscopy labs?
The integration of ChatGPT as a standard tool holds immense potential, Emily. However, it requires further research, development, and addressing several considerations such as data privacy, model interpretability, and the need for continuous model improvement.
I hope ChatGPT can assist researchers in overcoming common challenges faced in IR Spectroscopy, such as peak identification and deconvolution.
Indeed, Erica! ChatGPT's ability to learn from vast amounts of spectral data can help researchers tackle these challenges by providing automated tools for peak identification and deconvolution.
Galen, can we expect open-source implementations or pre-trained models of ChatGPT specifically tailored for IR Spectroscopy?
Open-source implementations and pre-trained models are valuable contributions to the scientific community. While it's an active area of research, offering specialized pre-trained ChatGPT models for IR Spectroscopy can greatly benefit researchers and foster collaboration.
Galen, what are the possibilities of integrating ChatGPT with experimental instrument software to enable real-time analysis?
Integrating ChatGPT with experimental instrument software can indeed empower real-time analysis, Michael. By combining spectral data acquisition with automated interpretation using ChatGPT, researchers can get rapid insights, accelerating their experiments and analysis.
I'm curious to know if there are any limitations or trade-offs when using ChatGPT for IR Spectroscopy. Galen, could you shed some light on this?
Certainly, Adam. While ChatGPT offers numerous advantages, the model's dependence on data means that it may struggle with providing accurate predictions for rare or unusual spectra. Additionally, computational resources required for training and deployment should be considered.
Galen, it's fascinating how AI is transforming scientific research. Do you see any ethical concerns associated with the widespread use of AI models like ChatGPT in IR Spectroscopy?
Great question, Alexis. Ethical considerations are crucial. Researchers using ChatGPT in IR Spectroscopy must be vigilant about the potential biases, data privacy, and transparency issues associated with AI models. Ensuring responsible and informed use is paramount.
Galen, are there any efforts to develop ChatGPT variants that can handle real-time, dynamic spectral data for continuous analysis?
Real-time analysis of dynamic spectral data is an important area of exploration, Andrew. Researchers are actively working towards developing ChatGPT variants that can handle continuous streams of spectral data and adapt to changing conditions.
How can we ensure the reproducibility of results obtained using ChatGPT in IR Spectroscopy? Galen, your insights would be valuable.
Ensuring reproducibility is crucial, Emma. Researchers should openly document their methodologies, publish code when possible, and provide access to trained models and related datasets. Promoting transparency and sharing knowledge helps foster reproducibility and scientific progress.
Galen, do you have any recommendations for researchers looking to incorporate ChatGPT into their IR Spectroscopy projects for the first time?
Certainly, Emily! Researchers new to incorporating ChatGPT should familiarize themselves with the model's strengths and limitations. It's essential to start with suitable training data, gradually evaluate its performance, and systematically fine-tune the model based on task-specific requirements.
Thank you, Galen, for providing insights into the exciting potential of ChatGPT in IR Spectroscopy. I look forward to witnessing the advancements in this field!